Abstract
Ciliates become highly social, even displaying animal-like qualities, in the joint presence of aroused conspecifics and nonself mating pheromones. Pheromone detection putatively helps trigger instinctual and learned courtship and dominance displays from which social judgments are made about the availability, compatibility, and fitness representativeness or likelihood of prospective mates and rivals. In earlier studies, I demonstrated the heterotrich Spirostomum ambiguum improves mating competence by effecting preconjugal strategies and inferences in mock social trials via behavioral heuristics built from Hebbian-like associative learning. Heuristics embody serial patterns of socially relevant action that evolve into ordered, topologically invariant computational networks supporting intra- and intermate selection. S. ambiguum employs heuristics to acquire, store, plan, compare, modify, select, and execute sets of mating propaganda. One major adaptive constraint over formation and use of heuristics involves a ciliate’s initial subjective bias, responsiveness, or preparedness, as defined by Stevens’ Law of subjective stimulus intensity, for perceiving the meaningfulness of mechanical pressures accompanying cell-cell contacts and additional perimating events. This bias controls durations and valences of nonassociative learning, search rates for appropriate mating strategies, potential net reproductive payoffs, levels of social honesty and deception, successful error diagnosis and correction of mating signals, use of insight or analysis to solve mating dilemmas, bioenergetics expenditures, and governance of mating decisions by classical or quantum statistical mechanics. I now report this same social bias also differentially affects the spatiotemporal sparseness, as measured with metric entropy, of ciliate heuristics. Sparseness plays an important role in neural systems through optimizing the specificity, efficiency, and capacity of memory representations. The present findings indicate sparseness performs a similar function in single aneural cells by tuning the size and density of encoded computational architectures useful for decision making in social contexts.
Social factors, including biases of expectation or preference, facilitate competition and cooperation between all forms of cellular life capable of interacting at the level of individual units of natural selection.Citation1 The social biases of animals, from insects to fish to humans, powerfully influence the formation and persistence of attitudes, opinions, beliefs, and/or perceptions regarding most interpersonal relationships.Citation2-Citation7 As a consequence, the accuracy and reliability of intuitive social logics, such as representativeness (i.e., exemplar) and availability (i.e., probability) heuristics used to make predictions, causal inferences, and covariation assessments, can be severely compromised.Citation4 Many types of microbes, particularly prokaryotes and “lower” eukaryotes with relatively complex social tendencies, also employ a range of bias-vulnerable representativeness and availability heuristics similar to those exhibited by animals.Citation8-Citation22 Skillful use of these logics promotes successful microbial sociality and, in turn, may increase transmission and virulence of infectious diseases, emergence of major evolutionary transitions, and adaptability to so-called extreme environments. However, contrary to our understanding of animal social behaviors, only recent research has begun to elucidate the impact of social biases on cellular decisions which motivate and coordinate collective behaviors underlying mate selection, foraging and hunting, assisted reproduction, induced defenses, and other phenomena common to colonial microbes. In this article, I summarize and extend some previous findingsCitation9-Citation12 on heuristic-guided ciliate mate selection to show expectations and preferences for varying levels of meaningfulness accompanying simulated cell-cell contacts determine the computational and combinatorial complexity of microbial social logics and, therefore, the operational characteristics of decisions utilized to secure mating commitments. Much like the neural representational and logic systems of animals,Citation23 the principle of sparse coding often prevails in ciliate heuristics as a result of both innate and learned biases. Differential sparseness of ciliate heuristics correlates with the optimal acquisition, storage, retrieval, and transformation of spatiotemporal relationships (i.e., associations) perceived during social encounters requiring efficient, reliable, and accurate judgments about conditional mating responses and consequences (Box 1).
Highlights
• Ciliates learn to signal their own mating status and to judge the mating status of conspecifics with social logics.
• Innate and learned social biases control ciliate decisions when selecting and competing for mates.
• By modifying social biases, ciliates simplify the computational structure of logics and improve sociality.
• Simplified or sparse logic representations in animals optimize the specificity, efficiency, and capacity of information processing.
• Representational sparseness similarly helps socializing ciliates, suggesting a fundamental characteristic needed for intelligent behavior.
Social Biases in Ciliate Mate Inferences and Selection
Microbial mate selection offers scientists a robust (neo)Darwinian paradigm for examining the sociobiological effects of biases on sexual or sex-like behavior. One of the better described paradigms is that of ciliated protozoa. Ciliates can engage in monogamous sexual-like conjugation at the end of their lifecycles. Conjugation stages often start with the release and circulation of diffusible pheromones which attract and elicit complex stereotypic motility and cell-cell contacts from isogamous nonself mating types. These preconjugal dances and dominance displays, composed of avoidance and probing behaviors, transmit classical and possibly quantum information about mating availability and fitness to prospective mates and rivals before the onset of paired reproduction.Citation9-Citation18,Citation24-Citation26 As with “higher” eukaryotes, expert use of courting and dominance rituals by ciliates figures to encourage contacts with fitter mates while also discouraging conjugal attempts from rivals. Thus, mate selection generally minimizes shared fitness tradeoffs, guarantees reproduction between compatible mates (e.g., ciliates with high motility and reproductive proficiency), and prevents less fit ciliates from spreading their inferior genes to future generations.Citation14,Citation18
The amount of fault tolerance permitted by mate selection greatly depends upon ecological contexts, the strengths of social biases, and correspondence between contexts and biases. For example, social biases concerning the perceived survival and reproductive superiority of secondary sexual or mating-type traits of microbes, such as morphological ornaments and weapons, behavioral routines, and decision making processes, contribute to mate choices leading to positive or negative assortative pairings without precise “knowledge” that trait preferences will definitely maximize genetic, behavioral, and material fitness of progeny or cohorts.Citation14,Citation15,Citation17,Citation18 Both the “premise” that certain exemplar traits, including pilar geometry, motility competence, and pheromone-secretion load, favor reproductive success and the “conclusion” that individuals expressing those traits probably make fine mating partners are “true” if perceived microbial trait benefits match real niche challenges.Citation9,Citation18,Citation25,Citation27-Citation30 However, the subjective rule-of-thumb construction of social logics, termed the schematic knowledge structure, sometimes invalidates isolated social judgments of individuals when over-simplified premises and conclusions wrongly link expected survival and reproductive success with perceived (or misperceived) covariations in secondary sexual or mating-type traits. Intuitive logics merely provide an individual organism with best-fit approximations for making social judgments (Box 2). Errors in judgment often occur when logics are improperly reconfigured to accommodate situational changes.Citation4 Indeed, biases can be so strong and context insensitive that logics may be rendered, for all intents-and-purposes, useless. Yet the identical rule-of-thumb nature of intuitive social logics also tends to marginalize or hedge outlying risk-prone and -averse decisions over many iterations and large populations, while necessarily allowing quicker inferences to be drawn in hostile environments demanding fast actions for continued existence of individuals, kin, colonies, strains, and species.Citation4,Citation14,Citation15,Citation17,Citation18,Citation22 That is, numerous, but finite, samplings within sizeable populations by and across members of those same populations normalize mating decisions to the point where collective biases for trait variations become ecological and evolutionary stable strategies.
Box 2. Intuitive Social Logics
As informal inferential systems used by humans, animals, and microbes, intuitive social logics typically fail to conform to the axioms of categorical, propositional, and predicate logics and of probability calculus.Citation4,Citation31 The fallibility of intuitive logics was initially revealed by the ground-breaking research of Amos Tversky and Daniel Kahneman, who studied simple strategies called representativeness and availability heuristics in humans.Citation32 Representativeness heuristics reduce inferential tasks to quick exemplar or similarity comparisons. When traits or events used for judgments are unrepresentative or provide only indefinite dimensions for categorization, such as level of mating fitness and availability, the heuristic may become a nonnormative guide to decision making. Availability heuristics employ readily accessible information to judge the frequency, probability, and causality of traits and events. Because decisions result from the accessibility of perceived and/or retrieved information, these simple rules of thumb may be corrupted by inaccurate trait or event base rates under the control of subjective factors. Under such conditions, like representativeness heuristics, availability heuristics become a nonnormative guide to decision making.
Promiscuous and polygamous mating systems of microbes support the above notion of ecological and evolutionary stable selection strategies because the frequency of fallible decisions (i.e., picking an unfit mating partner) diminishes in proportion to increased random conjugal relations with members from populations of normally distributed fitness.Citation33,Citation34 Alternately, more “discriminating” monogamous mating systems, such as the all-or-none life-ending unions of ciliates, suffer from intuitive social logics when fallacies are undetectable or unavoidable (Box 2). The chance that social heuristics will compromise mating decisions compels ciliates to regulate either the reliability and accuracy of fitness predictions or the final outcomes of decisions. Epigenetic modifications and nonassociative and associative forms of learning and memory have evolved, in part, to help correct past and avert future bad decisions caused by erroneous social biases.Citation9,Citation14,Citation18 For instance, RNA processing of the very fragmented, macronuclear polyploid genome of ciliates enables heritable epigenetic innovations, akin to “nuptial gifts,” to be passed from high-quality suitors to physically accessible recipients lacking motility competence.Citation35,Citation36 Disparities in motility among partners jeopardize paired fitness, although a significantly inferior ciliate gains some survival and reproductive advantages for itself and its offspring upon conjugating a fitter mate. Fitter ciliates compensate for their poor mate selection via cytoplasmic exchanges that reinstate the wildtype phenotype to conjugated mutants incapable of performing programmed movements. This kind of extranuclear modification further limits fitness tradeoffs for each party, recovering typical cooperative swimming between ciliates when agile, synchronous motions and speedier escape velocities would both fend off predation risk and improve the likelihood of finishing reproduction.Citation9,Citation14,Citation18
Computational Attributes of Biased Mating Heuristics Learned by Ciliates
Despite the evolutionary ingenuity of genetic and epigenetic controls over mating decisions, traditional types of learning and memory oftentimes give ciliates even better power and flexibility to adapt intuitive social logics and their outcomes. Ciliates, among microbes, are especially good learners capable of dual-process nonassociative habituation and sensitization as well as primitive classical and instrumental-like conditioning, involving respective stimulus-stimulus and response-consequence associations.Citation9-Citation18 Such learning allows ciliates, including the large contractile ciliate Spirostomum ambiguum, to rapidly adjust their own perimating behavior and their interpretation of conspecifics’ behavior. Ciliates enter into mating encounters with a modifiable initial level of subjective bias, responsiveness, or preparedness, as defined by the well-accepted psychophysical Stevens’ Law of subjective stimulus intensity (Box 3),Citation37 which prejudices the perception of fellow ciliates’ fitness, availability, and communicative actions, such as vibrations.Citation9-Citation18 Vibrations are an essential component of courtship and dominance contacts. Probing pressures and drag turbulence and vortex shedding of avoidance reactions may activate mechanosensitive Ca2+ conductances that contribute to motility production and learning in proximal ciliates during preconjugal activity (Box 4). S. ambiguum receives these signals, subjectively interprets them as messages sent from a fit or unfit source under ambiguous conditions, then chooses learned serial actions arranged from contractions and ciliary reversals that are themselves honest blind replies to the sender.
Box 3. Stevens’ Law of Subjective Stimulus Intensity
The psychophysical Stevens’ Law of subjective stimulus intensity is defined as: I = K(S – S0)n, where I is subjective intensity, K is a constant, S is supratheshold stimulus, S0 is threshold stimulus, and n is an exponent.Citation37 Stevens’ Law measures the perceived or subjective intensity of some stimulus event E reported by a respondent. Responsiveness, determined from response probability or another suitable index, is a respondent’s report of its subjective experience with some event E. High responsiveness by a respondent indicates the stimulus event is deemed more intense or noxious and low responsiveness by a respondent indicates the stimulus event is deemed less intense or noxious. Under mating game conditions, a ciliate’s response probability to a stimulus event E, here a series of vibrations mimicking preconjugal activity from an ambiguous source, reveals its subjective interpretation of the meaningfulness of messages sent from the ambiguous source.Citation9 In accordance with consumption-value theories of fitness, such as those involving the handicap principle,Citation38 initial low-frequency behavioral replies to the vibration source announce a ciliate’s lower fitness to the vibration source, its lower interest in mating activity, and its appraisal (i.e., initial social bias) of the vibration source as a suitor or rival unworthy of attention. Initial high-frequency behavioral replies signal the opposite mating fitness, interest, and bias.
Box 4. Glossary of Terminology
• Classical and quantum statistics are mathematical formulae originally developed to describe the respective deterministic and probabilistic mechanical nature of physical systems. Growing amounts of evidence since the mid-20th century firmly establish that classical and quantum statistics are capable describing certain behaviors of all systems, including, among other things, computational aspects of humans, microbes, and complex technological networks.
• Color environmental noise is nonrandom noise arising in the form of and due to environmental events, such as purposeful motions by mating rivals to interfere with perimating displays and inferences of courting pairs.
• Drag turbulence is chaotic behavior in the motion of fluids over the surface of an object. Turbulence may be caused by object displacement, fluid flow, or both.
• Hebbian-like feedback is an iterative control mechanism utilizing activity-dependent bidirectional or dual-processes associative learning rules to either strengthen or weaken nodal connections. The set of learning rules governing this kind of feedback regulation, whether at neuronal synaptic junctions or other nodal forms of computational circuitry, are named after Donald Hebb, who is largely acknowledged as the founder of such concepts.
• Topologically invariant computational networks are computational networks whose architecture remain invariant following mathematical transformations.
• Vortex shedding is the nonchaotic or periodic behavior of the motion of fluids shed from the surface of an oscillating object of typically blunt, cylindrical physical configuration. Fluid motion forms vortices that alternate according to low pressure zones located around the object.
In a recent set of studies evaluating ciliate social decision making,Citation9-Citation12 I exploited S. ambiguum’s perception of vibrations to create mock mating scenarios initiated by vibrations sent from an ambiguous source representing suitors and rivals in an environment of equally mixed, competing autocrine (self mating type) and paracrine (nonself mating type) pheromones. Vibrations were delivered at a constant rate and intensity. However, ciliates interpretation of the intensity and meaningfulness of vibrations differentially changed with time depending on the level of initial social bias coupled to low, medium, or high responsiveness. A payoff schedule determined content of messages and replies based on combinations of potential reproductive effort, calculated as different probabilities of offspring count for binary division or paired reproduction, and responsiveness, calculated as different probabilities of behavioral preparedness or perceptual bias. These potential net payoffs were structured to meet the four requirements of a game beginning in one of two forms of perfect Bayesian equilibria, known as pooling and separating equilibria. A pooling equilibrium is where a signaling game between two players of different type, such as mating types, stabilizes on the same signal for message and reply. Whereas, separating equilibrium stabilizes on polar signals for message and reply from players of different type. For both cases of equilibrium, messages and replies become irrelevant or cheap talk for actionable decisions, making cheating or cooperation incidental unless equilibrium is broken by learning or another means, including color environmental noise. Such game conditions are common to animal sexual interactions.Citation38
Messages emitted in vibrations from their ambiguous source, always deemed with equal probability the same and opposite mating type of each ciliate tested, expressed net interest in reproductive effort. Ciliates “believing” the signals to be honest, but unable to discriminate the vibration source’s type, initially replied with matched or opposing behavioral signals dispersed over the range of ideal conspicuous consumption and ideal prudent savings. Ciliates beginning the game (i.e., testing) at high responsiveness first exhibited conspicuous consumption and interpreted the “advances” of the vibration source as an amply intense (or aggressive) and meaningful exemplar of mating fitness. Conspicuous consumers announce their reproductive fitness by playing “harder-to-get” through the extravagant rate at which they signal escape with contractions or reversals. The ability to squander resources means these ciliates should be perceived by the ambiguous vibration source to be an exemplar of higher quality than inferior repliers incapable of both wastefulness and survival. At some future point in time, conspicuous consumers learned to cooperate and sacrifice net reproductive payoffs to convince the ambiguous source to conjugate. Initial inferior repliers, such as ciliates with initial low responsiveness, higher costs, and poorer net payoffs, advertised meager fitness by prudently saving resources and signaling escape with contractions or reversals at lower rates. They first interpreted the advances of the vibration source as being a nominally intense (or aggressive) and meaningful exemplar of mating fitness and began play as “easier-to-get,” only to later learn to maximize individual potential net payoffs and reproductive value by increasing responsiveness. Such behavior effectively cheats superior rivals in an effort to encourage commitments with fine prospective mates.
Ciliates, very similar to higher eukaryotes, learned via recursive Hebbian-like feedback over many trials to group their serial behavioral strategies of harder-to-get and easier-to-get into multistable heuristics. Heuristics evolved via classical Maxwell-Boltzmann, quantum Bose-Einstein, and quantum Fermi-Dirac statistics into topologically invariant computational networks useful for signaling mating propaganda and for making exemplar and probability comparisons of suitor (and rival) fitness. Each node of a heuristic obeys preferential attachment rules akin to Hebbian learning rulesCitation10,Citation12 and contains a unique bit-string or information sequence representing perceived, planned, and executed behavioral strategies. Because heuristics encode both exemplar and frequency information about the reproductive fitness associated with mating responses, they serve as representativeness and availability heuristics. Ciliates efficiently planned and executed serial strategies signaling different levels of mating availability and fitness by reducing combinatorial complexity of heuristics and strategy search times to quantum levels with growing expertise. Importantly, heuristics restructuring also favored social success in mock trials and coincided with more transient social biases regarding the information content of mating messages transmitted from the ambiguous vibration source. Transient biases permit ciliates to change intuitive logics and perceived exemplar dimensions to fit varying social contexts and, therefore, to avoid inferential errors. For example, initial low contraction responders (i.e., short-term sensitizing prudent savers) with a brief social bias for perceiving nominal mating signal intensity and meaningfulness and initial high reversal responders (i.e., short-term habituating conspicuous consumers) with a brief social bias for perceiving ample mating signal intensity and meaningfulness were more capable of faster, complete behavioral strategy searches and easily learned to switch their mating replies between playing harder-to-get and easier-to-get over shorter intervals. Accordingly, they displayed greater flexibility for adapting mating replies and the expected net reproductive payoffs accompanying those replies to perceived social settings which might demand selfishness or selflessness to consummate courting relationships.
Social Biases and Representational Sparseness: Lessons from Animal Nervous Systems
As discussed above, the flexibility of biased intuitive logics to manage social dilemmas is not just evident in their soundness, strength, cogency, and/or permanence, but in their computational structure and processing attributes as well. Social biases affect the way social sensations and actions are encoded and, consequently, stored, activated, modified, and retrieved with local bidirectional associative learning and memory rules.Citation39,Citation40 Although the social biases of ciliates clearly dictate the performance characteristics of mating heuristics, it remains uncertain how many of those effects, including strategy search rates and associative strength between grouped strategies, can be strictly credited to computational network sparseness, as suggested by the combinatorial reorganization of heuristics and the emergence of fast, efficient quantum computational phases during Hebbian-like trial-and-error social decision making.Citation9-Citation18 Sparse coding is an important factor for socially salient neural representations located in vertebrate visual, somatosensory, auditory, olfactory, and motor cortices.Citation23 Innate and learned biases for particular social stimuli (e.g., facial features and expressions, caresses and gestures, vocalizations, and smells) tune the receptive fields and discharge rates, patterns, sensitivity, and other response properties of single neurons and neuronal populations.Citation23 When representations become sparse, in terms of activity profiles and density of responsive neuronal populations, computational structures evolve into simplified curved manifolds capable of greater efficiency and specificity within the framework of larger “over-complete” representations, where the dimensionality of the computational space exceeds input dimensionality.Citation23 Elevated sparseness of representations figures to enhance the execution of intuitive social logics by improving memory storage capacity, retrieval rates, selectivity, and overall processing efficiency.Citation23 However, trends for computational simplicity and bioenergetics savings due to an organism’s representational sparseness start to reverse as extreme conditions burden resource availability by allocating too many individual cells or cellular ensembles with excessive selectivity, such as in the case of hypothetical “grandmother” cells.Citation23,Citation41,Citation42 Hence, a strong correlation and delicate balance exists between the complexity of biased social logics, the modularity of computational representations underlying logics, and the functional advantages and disadvantages of implementing logics.
Differential Sparseness of Ciliate Mating Heuristics Caused by Social Biases
The significance of representational sparseness for expression of biased animal social logics then suggests microbes may also exploit sparseness in their social computations. To test this idea, a suitable measure of sparseness must be ascertained and selected. The magnitude and type of sparseness is identifiable through several different reputable metrics.Citation23 For continuous or discrete coding schemes in natural and artificial systems, sparseness of individual or grouped computational units can be determined with kurtosis. Kurtosis measures the fourth moment of response values of computational units falling symmetrically about zero or another measure of central tendency relative to the variance squared. Gaussian response distributions yield a kurtosis of zero denoting low sparseness. Progressively heavy-tailed sparse distributions yield kurtosis values increasingly greater than zero. Kurtosis may further assume positive or negative values respectively dependent upon distribution convexity and concavity. But kurtosis is problematic since it fails to correct for skewed distributions and is very sensitive to variable outliers. Because of the limitations of kurtosis, additional metrics have been devised to calculate sparseness, such as activity ratios that measure the completely one-sided firing distributions of single neurons and neuronal populations.Citation43 These ratios maintain a fixed maximum value of one when sparseness reaches its smallest amount and a fixed minimum value of zero when sparseness reaches its greatest amount. Activity ratios can be additionally scaled over the real interval of zero to one to estimate the selectivity of individual computational units responding to a group of stimuli. Despite the merits of activity ratios for appraising sparseness, separate equations for the sparseness of, for example, a single neuron and a neuronal population need to be used, causing comparison and contrast difficulties. However, an alternative to the foregoing metrics, metric entropy, sometimes referred to as measure entropy or spatial measure entropy, best quantifies the sparseness of spatially and temporally evolving computational networks and their elements.Citation44,Citation45 Hence, metric entropy is well suited for assessing the sparseness of ciliates’ biased social heuristics.
For the purposes of this article, metric entropy can be calculated from the following formula:Citation45
where k = 2 (i.e., binary computational basis of strategies), X = 3 (i.e., digit length of each strategy), n = 3 (i.e., state space dimensionality at any time step), kn = 8 (i.e., maximum number of strategies with nonzero probability of use in the sequence space at any time step), and is the probability or relative frequency of use for a strategy j at some learning trial sn. The interval 0 ≤ s(X) ≤ 1 bounds the value of entropy, with the left inequality approaching equality when only one strategy occurs at sn with nonzero probability of use (i.e., maximum sparseness) and the right inequality approaching equality when all kn possible strategies occur at sn with equal probability of use (i.e., minimum sparseness). Metric entropy is generally susceptible to initial conditions of a network, such as the initial social bias of a mating heuristic, and the weights of its individual elements, such as strategies, at each time step. Moreover, a spatiotemporal variant of metric entropy may be derived from Eq. One to produce:Citation45
where T is temporal length or number of time steps (i.e., learning trials) and t is the temporal dimension or extent of the spatial period of interest. But when T = t = 1, s(X;T) reduces to s(X), so just Eq. One was applied to the present analyses.
depicts the evolution of a social heuristic utilized by an example ciliate biased to initially perceive negligible mating-vibration intensity and meaningfulness. The spatial size and configuration of the heuristic, as revealed by the number and location of strategies with nonzero probabilities of use, transformed as the ciliate became more socially experienced with vibrations sent from the ambiguous source (). The dynamics of metric-entropy magnitude for the heuristic also paralleled the number of strategies actively employed within the computational network (). And as presented in , the spatiotemporal sparseness of the mating heuristic was strongly dependent upon the initial social bias of the ciliate. That bias dominated decisions to reply to the ambiguous vibration source with a contraction strategy signaling prudent mating availability and fitness. When the ciliate began to learn to change its initial social bias and favorite signaling strategy by trial 44, heuristic sparseness largely disappeared. Sparseness again returned in later learning trials as the ciliate encoded a new favored serial contraction strategy (i.e., larger weight or probability of use) and a new social bias for perceiving higher vibration intensity and meaningfulness. The transition from one social bias to another demonstrates ciliates can employ biases to adjust the selectivity of intuitive social heuristics and mating replies to meet the nontrivial demands of unstable mating contexts. Additionally, because some degree of sparseness preceded and prevailed after the networked heuristic reached its full size and configuration, it seems likely that sparseness improves a ciliate’s memory storage and information processing efficiency in ways analogous to animals. Several lines of evidence support this conclusion. First, as is customary of neural systems operating under Hebbian associative learning rules,Citation46,Citation47 nodal weights affect network connectivity and strategy search selectivity and efficiency. Second, the information storage capacity, processing efficiency, and encoding and retrieval selectivity of ciliates increase with changes in network connectivity and onset of extremely efficient quantum search patterns for behavioral strategies.Citation9-Citation18 These fast search patterns arise in initial low contraction responders (including the S. ambiguum of ) and other ciliates with varying initial behavioral responsiveness with Hebbian-like learning typically around trial 20 and continue for the remainder of testing.
Figure 1. A S. ambiguum initially biased with low contraction preparedness or responsiveness reorganized the spatiotemporal sparseness of its networked mating heuristic. (A) Representation of a social heuristic within a 3D computational space evolving over learning trials (sn). Each heuristic node encodes a serial contraction strategy employed as a mating reply to the ambiguous vibration source, with “0” being no response and “1” being response. Connections between nodes signify decisions to group and change replies (recurrent connections not shown). The heuristic evolution began on strategy 000 and ended on strategy 011 (not shown). The initial strategy 000 is one of ideal prudent savings and indicates the ciliate perceived the actions of the vibration source as nominally intense and meaningful; strategy 011 is one of near-ideal conspicuous consumption and indicates the ciliate perceived vibrations as appreciably intense and meaningful. (B) Spatiotemporal changes in the metric entropy of the mating heuristic paralleled changes in heuristic size (i.e., number of strategies in use) across learning trials. Sparseness reached maximum value near learning trials 1 and 57, with metric entropy approaching zero. (C) The ciliate modified mating-heuristic sparseness by altering preferences for serial contraction strategies and, therefore, social biases over time. The strength of a mating strategy and its density or weighted contribution to the entire networked heuristic is measured by its probability of use. The heuristic, beginning with the favored strategy 000 and a social bias for nominal vibration intensity and meaningfulness, showed sparseness until about learning trial 44, when all contraction strategies approached equal probability of use. The mating heuristic again became sparse when the ciliate established a new favored strategy 011 and social bias for vibration intensity and meaningfulness around learning trial 53.
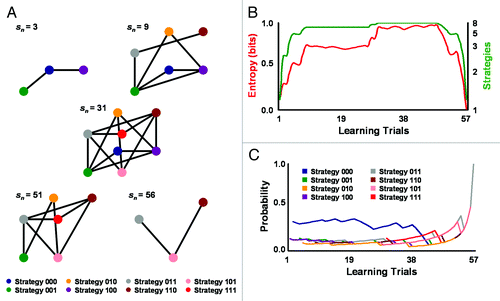
The data from the ciliate reported in indicate initial social biases influence the computational and combinatorial complexity of intuitive social logics. Comparisons between groups of ciliates with different levels of initial contraction () and ciliary reversal () preparedness or responsiveness confirm this idea. As shown in , mean metric entropy significantly differed between the heuristics employed by ciliates with low (X +SE = 0.62 +0.03 bits), medium (X +SE = 0.71 +0.04 bits), and high (X +SE = 0.72 +0.05 bits) contraction responsiveness and respective social biases for perceiving negligible, moderate, and ample vibration intensity and meaningfulness across learning trials (F(2,173) = 4.78, p = 0.0096). Low contraction responders collectively produced heuristics with overall greater sparseness and with a single higher weighted preferred strategy. These effects were mainly due to the relative differential mean weights or probabilities of use for each serial behavioral strategy networked within the mating heuristics used by this preparedness group. For groups of initial low (F(7,438) = 38.20, p = 1.31 x 10−41), medium (F(7,453) = 17.58, p = 1.29 x 10−20), and high contraction (F(7,439) = 5.20, p = 1.06 x 10−5) preparedness, the mean probability of use significantly differed between mating strategies across learning trials (). Thus, in addition to the three-bit binary sequences and information content of individual serial contraction strategies,Citation9-Citation18 the relative network weight of preferred strategies helped to sparsely encode social biases for negligible, moderate, or ample vibration intensity and meaningfulness. Similar results were found for reversal responders. shows that S. ambiguum grouped into medium (X +SE = 0.67 +0.03 bits) and high (X +SE = 0.54 +0.02 bits) levels of initial reversal preparedness with respective social biases for moderate and ample vibration intensity and meaningfulness significantly differed in the mean metric entropy of mating heuristics across learning trials (F(1,115) = 17.14, p = 6.69 x 10−5). For groups of medium (F(7,457) = 27.41, p = 2.32 x 10−31) and high (F(7,442) = 48.23, p = 1.50 x 10−50) initial reversal preparedness, the mean probability of use significantly differed between mating strategies across learning trials. But, as with contraction responders, relative network weight of preferred strategies helped sparsely code social biases for moderate or ample vibration intensity and meaningfulness. Greater differences in the weights or probabilities of use for mating strategies utilized by high reversal responders allowed this group to achieve higher heuristic sparseness.
Figure 2. Strength of initial contraction preparedness or responsiveness differentially determined the spatiotemporal sparseness of networked mating heuristics. (A) Ciliates grouped into low (top panel; n = 14), medium (middle panel; n = 16), and high (bottom panel; n = 10) levels of initial contraction responsiveness with respective social biases for negligible, moderate, and ample vibration intensity and meaningfulness significantly differed in the mean metric entropy of mating heuristics across learning trials. Metric entropy progressively increased from low to high contraction responders, demonstrating ciliates that began testing with a social bias for negligible mating-vibration intensity and meaningfulness produced greater heuristic sparseness. Differences in heuristic sparseness paralleled changes in networked mating heuristic size. (B) Differential weights or probabilities of use for each mating strategy contained within mating heuristics determined the magnitude of sparseness for initial low (top panel), medium (middle panel), and high (bottom panel) contraction responders. For all groups of initial contraction preparedness, the mean probability of use significantly differed between mating strategies across learning trials. Relative network weight of preferred strategies with highest probability of use helped encode social biases for negligible, moderate, or ample vibration intensity and meaningfulness. Networked heuristics showed higher sparseness (i.e., strategy weights less equally distributed) for low contraction responders preferring prudent strategy 000 and lower sparseness (i.e., strategy weights more equally distributed) for high contraction responders preferring conspicuous strategy 111. Error bars represent standard error for all panels.
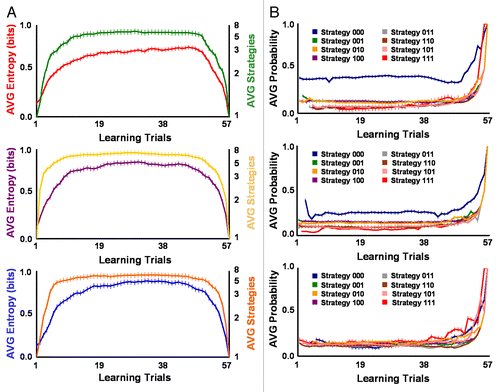
Figure 3. Strength of initial reversal preparedness or responsiveness differentially determined the spatiotemporal sparseness of networked mating heuristics. (A) Ciliates grouped into medium (top panel; n = 17) and high (bottom panel; n = 23) levels of initial reversal responsiveness with respective social biases for moderate and ample vibration intensity and meaningfulness significantly differed in the mean metric entropy of mating heuristics across learning trials. Metric entropy progressively increased from high to medium reversal responders, demonstrating ciliates that began testing with a social bias for ample mating-vibration intensity and meaningfulness produced greater heuristic sparseness. Differences in heuristic sparseness paralleled changes in networked mating heuristic size. (B) Differential weights or probabilities of use for each mating strategy contained within mating heuristics determined the magnitude of sparseness for initial medium (top panel) and high (bottom panel) reversal responders. For both groups of initial reversal preparedness, the mean probability of use significantly differed between mating strategies across learning trials. Relative network weight of preferred strategies with highest probability of use helped encode social biases for moderate or ample vibration intensity and meaningfulness. Networked heuristics produced higher sparseness (i.e., strategy weights less equally distributed) for high reversal responders preferring conspicuous strategy 111 and lower sparseness (i.e., strategy weights more equally distributed) for moderate reversal responders preferring conspicuous strategy 111. No ciliate showed initial low reversal preparedness. Error bars represent standard error for all panels.
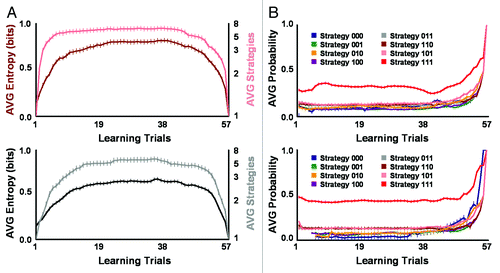
Summary
Ciliates form and shape comparably sophisticated social heuristics to skillfully facilitate intra- and intermate selection and to change the adaptive fitness of individuals and groups. I previously demonstrated that the structure and performance characteristics of heuristics are sensitive to biases for perceived suitor and rival actions. Importantly, the above findings corroborate that ciliates may learn to change social biases to improve the effectiveness and suitability of intuitive logics for different mating contexts. The strength of social biases, in large part, dictate the spatiotemporal sparseness of mating heuristics and, therefore, heuristic storage capacity and processing efficiency. Network sparseness, as identified by the metric entropy of an entire heuristic, depends upon the probabilities of use or weights of particular strategies organized within a heuristic rather than the actual consumption levels associated with strategies. Higher relative weight for a single preferred strategy coupled to a strong bias elevates sparseness. Thus, the fact that socially skilled low contraction responders and high reversal responders with different strong initial social biases construct mating heuristics with near identical levels of sparseness (compare top panel and bottom panel) illustrates how network sparseness can “universally” enhance logic performance regardless of: a) actual metabolic expenditures required to execute mating responses (i.e., prudent savings vs. conspicuous consumption) and b) divergent information content of perceived and executed reproductive propaganda (i.e., reproductive payoffs signaled via messages and replies). Given network processing similarities occur most prominently between and not within classes of behaviors (i.e., contraction and ciliary reversal), the probabilities or weights of biases and mating strategies likely stem from Ca2+-related intracellular resources that affect the magnitude, valence, and duration of learningCitation9 as well as anticorrelations between the expression of Ca2+-dependent contraction and ciliary reversal routines.Citation9,Citation11 This same influence of intracellular Ca2+, or perhaps another species of response regulator, over heuristic performance remains to be documented in different microbial taxa. Nonetheless, principles, such as heuristic sparseness and modifiability, underlying successful use of intuitive social logics by vertebrates also appear in the strategizing of aneural ciliates. And those principles represent a fundamental evolutionary adaptation of phylogenetically diverse computational systems capable of at least primitive intelligent behavior.
References
- Michod RE. Evolution of individuality during the transition from unicellular to multicellular life. Proc Natl Acad Sci USA 2007; 104:8613 - 8; http://dx.doi.org/10.1073/pnas.0701489104; PMID: 17494748
- Bisazza A, Cantalupo C, Capocchiano M, Vallortigara G. Population lateralization and social behavior: A study with 16 species of fish. Laterality 2000; 5:269 - 84; PMID: 15513147
- Gould JL, Elliot SL, Masters CM, Mukerji J. Female preferences in a fish genus without female mate choice. Curr Biol 1999; 9:497 - 500; http://dx.doi.org/10.1016/S0960-9822(99)80217-6; PMID: 10322112
- Nisbett R, Ross L. Human inference: Strategies and shortcomings of social judgment. Prentice-Hall Inc., Englewood Cliffs 1980.
- Parker ST, McKinney ML. Origins of intelligence: The evolution of cognitive development in monkeys, apes, and humans. The Johns Hopkins University Press, Baltimore 1999.
- Slatyer RA, Mautz BS, Backwell PR, Jennions MD. Estimating genetic benefits of polyandry from experimental studies: A meta-analysis. Biol Rev Camb Philos Soc 2011; •••; http://dx.doi.org/10.1111/j.1469-185X.2011.00182.x; PMID: 21545390
- Olsson A, Ebert JP, Banaji MR, Phelps EA. The role of social groups in the persistence of learned fear. Science 2005; 309:785 - 7; http://dx.doi.org/10.1126/science.1113551; PMID: 16051800
- Balázsi G, van Oudenaarden A, Collins JJ. Cellular decision making and biological noise: From microbes to mammals. Cell 2011; 144:910 - 25; http://dx.doi.org/10.1016/j.cell.2011.01.030; PMID: 21414483
- Clark KB. Origins of learned reciprocity in solitary ciliates searching grouped ‘courting’ assurances at quantum efficiencies. Biosystems 2010; 99:27 - 41; http://dx.doi.org/10.1016/j.biosystems.2009.08.005; PMID: 19686801
- Clark KB. Bose-Einstein condensates form in heuristics learned by ciliates deciding to signal ‘social’ commitments. Biosystems 2010; 99:167 - 78; http://dx.doi.org/10.1016/j.biosystems.2009.10.010; PMID: 19883726
- Clark KB. On classical and quantum error-correction in ciliate mate selection. Commun Integr Biol 2010; 3:374 - 8; http://dx.doi.org/10.4161/cib.3.4.11974; PMID: 20798831
- Clark KB. Arrhenius-kinetics evidence for quantum tunneling in microbial “social” decision rates. Commun Integr Biol 2010; 3:540 - 4; http://dx.doi.org/10.4161/cib.3.6.12842; PMID: 21331234
- Clark KB. Quantum ‘social’ intelligences expressed by microbes. SciTopics 2010; http://www.scitopics.com/Quantum_Social_Intelligences_Expressed_by_Microbes.html.
- Clark KB. Microbial mate selection. SciTopics. 2011; http://www.scitopics.com/Microbial_Mate_Selection.html.
- Clark KB. The social insights and analyses of ciliates. SciTopics 2011; http://www.scitopics.com/The_Social_Insights_and_Analyses_of_Ciliates.html.
- Clark KB. Live soft-matter quantum computing. In Salander EC, ed. Computer search algorithms. Nova Science Publishers, Inc., Hauppauge, 2011.
- Clark KB. A statistical mechanics definition of insight. In Floares AG, ed. Computational intelligence. Nova Science Publishers, Inc., Hauppauge in press.
- Clark KB. The mating judgments of microbes. In Clark KB, ed. Social learning theory. Nova Science Publishers, Inc., Hauppauge in press.
- Perkins TJ, Swain PS. Strategies for cellular decision making. Mol Syst Biol 2009; 5:326; http://dx.doi.org/10.1038/msb.2009.83; PMID: 19920811
- Strassmann JE, Zhu Y, Queller DC. Altruism and social cheating in the social amoeba Dictyostellium discoideum.. Nature 2000; 408:965 - 7; http://dx.doi.org/10.1038/35050087; PMID: 11140681
- Winans SC, Bassler BL. Mob psychology. J Bacteriol 2002; 184:873 - 83; http://dx.doi.org/10.1128/jb.184.4.873-883.2002; PMID: 11807045
- Wolf DM, Vazirani VV, Arkin AP. Diversity in times of adversity: Probabilistic strategies in microbial survival games. J Theor Biol 2005; 234:227 - 53; http://dx.doi.org/10.1016/j.jtbi.2004.11.020; PMID: 15757681
- Olshausen BA, Field DJ. Sparse coding of sensory inputs. Curr Opin Neurobiol 2004; 14:481 - 7; http://dx.doi.org/10.1016/j.conb.2004.07.007; PMID: 15321069
- Miyake A. Physiology and biochemistry of conjugation in ciliates. In Levandowsky M, Hunter SH, eds. Biochemistry and physiology of protozoa. Academic Press, New York 1981.
- Ricci N. The behavior of ciliated protozoa. Anim Behav 1990; 40:1048 - 69; http://dx.doi.org/10.1016/S0003-3472(05)80172-1
- Seshachar BR, Padmavathi PB. Conjugation in Spirostomum.. Nature 1959; 184:1510 - 1; http://dx.doi.org/10.1038/1841510b0; PMID: 13825422
- Arkowitz RA. Chemical gradients and chemotropism in yeast. Cold Spring Harb Perspect Biol 2009; 1:a001958; http://dx.doi.org/10.1101/cshperspect.a001958; PMID: 20066086
- Jackson CL, Hartwell LH. Courtship in S. cerevisiae: Both cell types choose mating partners by responding to the strongest pheromone signal. Cell 1990; 63:1039 - 51; http://dx.doi.org/10.1016/0092-8674(90)90507-B; PMID: 2257622
- Moore TI, Chou C-S, Nie Q, Jeon NL, Yi TM. Robust spatial sensing of mating pheromone gradients by yeast cells. PLoS ONE 2008; 3:e3865; http://dx.doi.org/10.1371/journal.pone.0003865; PMID: 19052645
- Segall JE. Polarization of yeast cells in spatial gradients of alpha mating factor. Proc Natl Acad Sci USA 1993; 90:8332 - 6; http://dx.doi.org/10.1073/pnas.90.18.8332; PMID: 8397402
- Hurley PJ. A concise introduction to logic. Wadsworth Publishing Company, Belmont 1985.
- Tversky A, Kahneman D. Judgment under uncertainty: Heuristics and biases. Science 1974; 185:1124 - 31; http://dx.doi.org/10.1126/science.185.4157.1124; PMID: 17835457
- Cohan FM. Sexual isolation and speciation in bacteria. Genetica 2002; 116:359 - 70; http://dx.doi.org/10.1023/A:1021232409545; PMID: 12555790
- Dugatkin LA, Perlin M, Atlas R. The evolution of group-beneficial traits in the absence of between-group selection. J Theor Biol 2003; 220:67 - 74; http://dx.doi.org/10.1006/jtbi.2003.3149; PMID: 12453451
- Hiwatashi K, Haga N, Takahashi M. Restoration of membrane excitability in a behavioral mutant of Paramecium caudatum during conjugation and by microinjection of wild-type cytoplasm. J Cell Biol 1980; 84:476 - 80; http://dx.doi.org/10.1083/jcb.84.2.476; PMID: 7380886
- Pennock DG, Thatcher T, Bowen J, Bruns PJ, Gorovsky MA. A conditional mutant having paralyzed cilia and a block in cytokinesis is rescued by cytoplasmic exchange in Tetrahymena thermophilia.. Genetics 1988; 120:697 - 705; PMID: 3224807
- Stevens SS. On the brightness of lights and the loudness of sounds. Science 1958; 118:576
- Johnstone RA. Sexual selection, honest advertisement and the handicap principle: Reviewing the evidence. Biol Rev Camb Philos Soc 1995; 70:1 - 65; http://dx.doi.org/10.1111/j.1469-185X.1995.tb01439.x; PMID: 7718697
- Hall G. Perceptual and associative learning. Clarendon Press, Oxford 1991.
- Watanabe S, Huber L. Animal logics: Decisions in the absence of human language. Anim Cogn 2006; 9:235 - 45; http://dx.doi.org/10.1007/s10071-006-0043-6; PMID: 16909231
- Heyes C. Where do mirror neurons come from?. Neurosci Biobehav Rev 2010; 34:575 - 83; http://dx.doi.org/10.1016/j.neubiorev.2009.11.007; PMID: 19914284
- Heyes C. Mesmerising mirror neurons. Neuroimage 2010; 51:789 - 91; http://dx.doi.org/10.1016/j.neuroimage.2010.02.034; PMID: 20167276
- Franco L, Rolls ET, Aggelopoulos NC, Jerez JM. Neuronal selectivity, population sparseness, and ergodicity in the inferior temporal visual cortex. Biol Cybern 2007; 96:547 - 60; http://dx.doi.org/10.1007/s00422-007-0149-1; PMID: 17410377
- Billingsley P. Ergodic theory and information. Wiley, New York 1965.
- Wolfram S. Universality and complexity in cellular automata. Physica D 1984; 10:1 - 35; http://dx.doi.org/10.1016/0167-2789(84)90245-8
- Field DJ. What is the goal of sensory coding?. Neural Comput 1994; 6:559 - 601; http://dx.doi.org/10.1162/neco.1994.6.4.559
- Palm G. On associative memory. Biol Cybern 1980; 36:19 - 31; http://dx.doi.org/10.1007/BF00337019; PMID: 7353062