Abstract
The ornate and diverse patterns of seashells testify to the complexity of living systems. Provocative computational explorations have shown that similarly complex patterns may arise from the collective interaction of a small number of rules. This suggests that, although a system may appear complex, it may still be understood in terms of simple principles. It is still debatable whether shell patterns emerge from some undiscovered simple principles, or are the consequence of an irreducibly complex interaction of many effects. Recent work by Boettiger, Ermentrout and Oster on the biological mechanisms of shell patterning has provided compelling evidence that, at least for this system, simplicity produces diversity and complexity.
The diversity and intricacy of the patterns on seashells have long been symbolic of the creative beauty of the natural world. Suggestively similar patterns, produced by simple equations, have become an icon for the aesthetic and power of abstract math. Now, recent work investigating the mechanism of shell patterning has demonstrated that nature’s technique has the same simplicity inherent in those mathematical abstractions. A simple neural circuit with a connection pattern of short-range excitatory synapses and long-range inhibitory synapses can generate almost all the shell patterns found in nature. Simple differences in the sensitivity of the neurons to stimulation along with the firing response characteristics account for the differences in the pigmentation patterns and shell shapes found amongst different species. This work highlights an emerging role for neural control in understanding self-organization and spatial patterning in the growth and development of higher organisms. Furthermore, it reveals a common biological framework capable of executing many of the models used to demonstrate “emergent complexity”—a growing field interested in how a few simple rules can generate unpredictably complex and intricate behavior.Citation1–Citation3
Here, we review briefly the development of the emergent complexity, illuminating the role shell patterns have played as both an example and an inspiration. We then review the neural patterning model that reproduces shell patterns, highlighting the connection between this model and earlier mathematical abstractions, which capture particular elements of its behavior. Finally we consider what the diversity of emergent patterning behaviors found in aquatic mollusks might teach us about other neural systems.
An Ancient Symbol for a New Field: Seashells and Emergent Complexity
Simple abstract systems with a few rules governing interaction between individual components give rise to complex, behavior of the system as a whole. This discovery launched a new era in both popular and academic sciences. Whole departments and research institutes have sprung up dedicated to the study of dynamical systems and complexity. Yet this observation is not new to science. Newton’s laws for the interactions of massive bodies provided explanations for as diverse phenomena as the falling of an apple to the motions of the heavens. Poincaré first noticed the chaotic trajectories that Newton’s deterministic laws of motion could produce. The power of relatively simple, concise models has been a defining feature of physics. Yet with the advent of personal computers, simple systems of arbitrary rules with no foundation in the natural world could be rapidly explored. These studies took another step forward when explorers like Stephen Wolfram recognized familiar patterns from nature could be reproduced by these entirely abstract simplified models.Citation3 These models appeared to capture features once thought too intricate to explain—like the dendritic branching of trees, the meandering curves of coastlines, and the ornate patterns of seashells. These mathematical and computational forays provided provocative—if rather circumstantial—evidence that complex patterns of nature may yet be understood in terms in a few fundamental concepts.
One example of such emergent complexity is the fractal patterns that are self-similar at all levels of resolution generated from simple recursive rules. The “Sierpinski triangles”, first described by the Polish mathematician Waclaw Sierpinski in 1915, seem remarkably similar to the pigmentation patterns of the shells of Cymbiola innexa (see ). The parallel has been noticed by Wolfram, Kusch and others.Citation4,Citation5
Cellular automata (CA) programs—discreet fields of units whose state depends on the states of their neighbors—provided another field of investigation where simple rules give rise to complex emergent behaviors. In the most common of automata models a unit is either on or off, and its state is determined during each iteration by a simple function of the states of its immediate neighbors in the previous iteration. For example, Conway’s “Game of Life,” the best known of automata models, a unit turns on if three of its neighbors were on, and turns off if fewer than two or more than three neighbors were on in the previous cycle. With these simple rules a tremendous variety of behaviors can arise depending on the initial configuration of the system. This includes traveling “glider” patterns, self regenerating patterns, or an array which can function as a universal Turing machine.Citation6 Waddington and CoweCitation7 were among the first to demonstrate that a variety of apparently intricate, complicated shell patterns could be produced by a small collection of rules governing a CA model with some added stochastic fluctuations to the values of the units. Kusch and colleagues subsequently demonstrated that a CA with seven rules and seven parameters can reproduce a much broader array of patterns, with and without periodic structure.Citation5 Many of these patterns are examples of “irreducible systems,” whose global behavior cannot be described by computational rule aside from a step by step application of the CA rules (see Binder 2009 for an excellent recent discussion).Citation1
The best-known work demonstrating emergent order was initiated by Alan Turing, describing the properties of certain reaction diffusion systems. In the 1950s Turing captured the imagination of chemists and biologists by demonstrating that simple diffusion-reaction (DR) processes with uniform initial distributions of components could self-organized into spatially structured stable states.Citation8 This suggested a possible natural mechanism to explain, for example, how a collection of once identical cells in a developing multicellular organism could adopt separate fates. Meanwhile the apparently spontaneous generation of structured patterns from a simple, initially homogeneous system became a classical example in the growing field of emergent complexity. Further development of this work earned Ilya Prigogine the Nobel Prize in chemistry in 1977, which Turing would likely have shared had he lived. Meinhardt, Gierer and Murray explored these systems in much greater depth and demonstrated how a variety of further elaborations and additional reacting species could produce many more complex spatial patterns.Citation12–Citation14 Once again, the diverse patterns of seashells became the iconic reference—the evidence that these patterns arise in nature and the motivation for understanding better the properties of the algorithms that produced them.Citation9–Citation16
The Mollusk’s Thoughts on Shell Patterns
Meanwhile experimental malacologists were turning high powered electron microscopes and new tissue specific labeling techniques on mollusk’s shell-secreting organ, its mantle. These experiments revealed a dense network of sensory and stimulatory neurons distributed throughout the mantle. Inhibition of these neurons with neural blockers led to defects or arrest of shell growth and patterning.Citation17–Citation19
Concurrent with Meinhardt’s reaction diffusion modeling, Ermentrout, Campbell and Oster proposed a neural model, arguing that the long range correlations in patterns required a non-local patterning mechanism.Citation20 This model still posited the build up of a chemical refractory substance, and it lacked any direct connection to the physiological evidence of neural control that was just emerging at the time. Although the model reproduced far fewer patterns than Meinhardt’s subsequent work, it set the groundwork for the current model. Aided by a much improved picture of the neural physiology behind shell growth and patterning, Boettiger, Ermentrout and Oster used a purely neurosecretory model to reproduce a great diversity of patterns. This demonstrated that nature seems to generate its complex pigmentation patterns using the behavioral properties of neurons. Thus the long-sought ‘morphogens’ turn out to be simply neurotransmitters. Since this neural activity determines both the shape and patterning of the shell, one might metaphorically refer to that pattern as a record of the mollusk’s “thoughts”.
The neural equations that describe the biological interactions determining shell growth have the analogous form of a simple ‘rule’ in the form of an integral-difference equation with nine parameters. This rule generates complex structured patterns as did the other abstractions discussed previously. Moreover, the model demonstrates that the abstract, data-independent models that have solutions reminiscent of shell patterns can indeed be interpreted as particular types of neural models. For example, by discretizing the neural equations and choosing hard thresholds for the input-firing rate response kernels, a CA type model can be created from the neural model (see Boettiger 2009 SOM for further details). Similarly, if the neural activity is near the steady state firing rate, the convolution can be expanded in harmonics to give DR like equations.Citation16,Citation20
A New Model System for Neural Interactions?
The success and tractability of the neural model of shell patterning and shape suggests that species exhibiting neural controlled spatial patterning may provide model systems to investigate the properties of neural networks empirically. Visible spatial patterns provide a clear readout of the activity state of the underlying network and the range of stable states it can adopt without requiring invasive measurements. Understanding how spatial patterns of pigment interact and propagate to create new patterns, we gain insight into how the underlying waves of neural activity interact. For example, the tear-drop patterns on the shell of Conus marblus result from the slowing down of converging traveling waves of neural activity. Similar effects have been observed in converging waves of neural activity across the cortex of rat brains.Citation21 Thus, understanding the neural interactions responsible for creation of spatial and temporal patterns in mollusks may provide new insights into the propagation and interactions of neutral signals in higher organisms. One near cousin of the shell building mollusks, the Cephalopods, may prove more tractable ground for such explorations. Cephalopods (octopus, squid and cuttlefish) use a neural network to control the exposure of chromatophores to change their skin color and patterns for camouflage and social behaviors. Phylogenetically, the Cephalopods are also mollusks and their skin is the homologue of the mantle in shell building mollusks. Each individual organism is able to produce a wide variety of static and dynamic patterns depending on its social and environmental context. Understanding how simple interactions of this neuromuscular network give rise to such diverse skin patterns may provide insight into how neural interactions following similar rules can give rise to the types of complex interactions we call consciousness.
Figures and Tables
Figure 1 Simple models create complex patterns of triangles. (A) Sierpinski triangles: generated by simply drawing an upside down triangle inside the original triangle, then drawing a new triangle in each of the triangles this generates, as indicated in the inset. (B) A cellular automata model, specified by “Rule 90”, illustrated at left. This produces a pattern much like that in (A) if started from a single point. With random initial conditions it generates patterns like those seen here. (C) Neural model of Cymbolia innexa (Boettiger et al. 2009). (D) Photo of Cymbolia innexa.
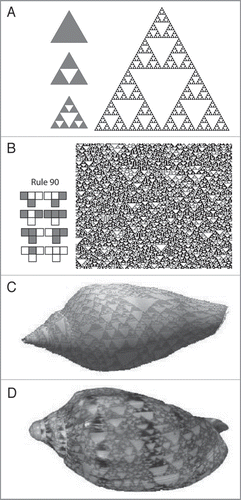
Addendum to:
References
- Binder PM. The edge of reductionism. Nature 2009; 459:332 - 334
- Wolfram S. Undecidability and intractability in theoretical physics. Phys Rev Letts 1985; 54:735 - 738
- Wolfram S. A New Kind of Science. Wolfram Media 2002;
- Wolfram S. Cellular automata as models of complexity. Nature 1984; 311:419 - 424
- Kusch I, Markus M. Mollusc shell pigmentation: cellular automaton simulations and evidence for undecidability. J Theor Biol 1996; 178:333 - 340
- Dennett D. Darwin’s Dangerous Idea 1995; London Penguin Books
- Waddington CH, Cowe J. Computer simulations of a molluscan pigmentation pattern. J Theor Biol 1969; 25:219 - 225
- Turing A. The chemical basis of morphogenesis. Phil Trans Roy Soc B 1952; 237:37 - 72
- Meinhardt H. Models of Biological Pattern Formation 1982; New York Academic Press
- Meinhardt H. Models for positional signalling, the threefold subdivision of segments and the pigmentation pattern of molluscs. J Embr Exp Morph 1984; 83:289 - 311
- Meinhardt H, Gierer A. Pattern formation by local self-activation and lateral inhibition. Bioessays 2000; 22:753 - 760
- Meinhardt H, Klingler M. Pattern formation by coupled oscillations: the pigmentation patterns on the shells of mollusces. Lecture Noth Biomath 1986; 71:184 - 198
- Meinhardt H, Klingler M. A Model for pattern formation on shells of molluscs. J Theor Biol 1987; 126:63 - 89
- Meinhardt H, Prusinkiewicz P, Fowler D. The Algorithmic Beauty of Sea Shells 2003; 3rd ed New York Springer
- Gierer A, Meinhardt H. A theory of biological pattern formation. Kybernetik 1972; 12:30 - 39
- Murray JD. Mathematical biology 2003; 3rd ed New York Springer
- Dillaman RM, Saleuddin ASM, Jones GM. Neurosecretion and shell regeneration in Helisoma duryi. Can J Zool 1976; 54:1771 - 1778
- Dogterom AA, Loenhout H, Schors RC. The effects of growth hormone of Lymnaea stagnalis on shell calcification. Gen Comp Endocrinol 1979; 39:63 - 678
- Wilbur K, Saleuddin ASM. The Mollusca 1983; New York Academic Press Inc
- Ermentrout B, Campbell J, Oster G. A Model for Shell Patterns Based on Neural Activity. The Veliger 1986; 28:369 - 388
- Xu W, et al. Compression and reflection of visually evoked cortical waves. Neuron 2007; 55:119 - 129