Abstract
Migration is a hallmark life history strategy of a diverse range of organisms, and also ubiquitous in ontogenic processes including normal embryonic development as well as tumor progression. In such scenarios, individual organisms/cells typically respond to long range (and often noisy) environmental cues. In addition, individuals may interact socially with one another leading to emergent group-level navigational abilities. Although much progress has been made in understanding the mechanisms of taxis, there is a lack of theoretical and quantitative understanding of how individuals trade-off information obtained through their own migratory ability and that via social interactions. Here, we discuss results and insights from a recent computational model developed to investigate the evolution of leadership and collective motion in migratory populations. It is shown that, for a broad range of parameter values, only a small proportion of the population gather directional information while the majority employ social cues alone. More generally, ecological conditions for the evolution of resident, solitary and collective migratory strategies are obtained. We discuss how consideration of both proximate and ultimate factors within the same framework may provide insights into preserving migratory patterns that are in grave danger due to anthropogenic pressures.
Modeling the Evolution of Migration
Migration is a spectacular and widespread phenomenon in the natural world occurring in myriad forms spanning a range of spatiotemporal scales; from the solitary migration of germ cellsCitation1 and blackcaps (Sylvia atricapilla),Citation2 to collective migration of neural crest cellsCitation3 and wildebeest (Connochaetes taurinus).Citation4 A key feature that characterizes migratory individuals is relatively long-term directionality of motion to reach habitats that offer safety from predation, better grazing grounds, and/or enhanced opportunities for breeding.Citation5 This process is often facilitated by individuals sensing and following directional cues from the environment. For example, micro-organisms and cells typically use chemical or thermal cuesCitation6 whereas many migratory birds use magnetic, visual and olfactory information.Citation5
In addition to responding to environmental cues, migratory organisms may interact socially with one another. In some cases social interactions during migration result in the formation of very large mobile groups, the mass migration of wildebeest of the Serengeti being an iconic example. Previous mathematical models have shown that although each individual may, itself, be error prone in determining correctly the direction in which to move, combining information with others (through relatively simple local interactions such as a tendency to align direction of travel with near neighborsCitation7) reduces this noise, facilitating effective information transfer about resources and migratory routes over large spatial scales.Citation8–Citation12 In this above scenario one can consider the pooling of information through social interactions to be a public good.Citation13,Citation14 It is unclear, however, if or how such strategies evolve in natural systems. This is because we lack a fundamental understanding of how such simple yet contrasting features of individuality and collectivity originate in the first place and how they can be maintained through natural selection.
Here, we discuss recent results of a model developed to provide an integrated framework for the study of leadership, collective animal behavior and migratory strategies based on fundamental tenets of evolutionary biology.Citation15 One of the challenges in developing such a model is that in order to accurately represent the dynamics of individual motion and interactions they must be simulated at a suitably fine timescale. Furthermore, we need to maintain individual identities within generations (to represent genetically and/or phenotypically distinct individuals) and to consider a sufficiently large population to prevent potential artifacts such as fixation of strategies by drift. Finally, these computations must be performed over ecological and evolutionary timescales where the statistical relationship between (sometimes changing) strategies and fitness (which itself is frequency-dependent) can be accurately determined.
To achieve these goals we developed a massively-parallel computational framework entirely coded and implemented on programmable graphics processing units (GPUs).Citation16,Citation17 Whereas a typical contemporary desktop computer has two or four processing cores, GPUs have hundreds of cores, and thus are capable of performing orders of magnitude more operations per unit time than CPUs. We were therefore able to simulate 16,000 interacting individuals with a code that is hundreds of times faster than on a CPU.Citation16 Whereas architectures that double or triple computational power provide valuable advances in scientific research, those that can offer tens to hundreds of times more performance are transformative, changing the way in which we do scientific computing and allowing us to address entirely new questions.
Leadership, Sociality and Evolving Migration
Individuals in our model possess two evolvable traits.Citation15 First, they have the ability to detect environmental gradients/cues, which we refer to as their “gradient detection ability.” Secondly, individuals can evolve to interact socially with others, specifically a tendency to move towards and to align direction of travel with near neighbors. These behavioral traits are not discrete and individuals may exhibit a continuum of possible strategies depending on the degree to which they rely on (and invest in) each. It is assumed that those who travel farther along the environmental gradient accumulate more resources or reach better breeding habitats, and thus accrue fitness benefits. However, individuals may also incur a cost that is proportional to the level of investment in their traits of gradient detection ability and/or sociality. Such costs may arise, for example, due to energetic expenditure involved in detecting migratory information in a noisy environment. In addition, there could be associated costs such as reduced predator vigilance while processing directional cues or increased competition for local resources when individuals aggregate with others. We show that even such relatively simple trade-offs between the costs and benefits of migration can account for a wide range of observed migratory phenomenon.Citation15
In a simple scenario, when the costs of both traits are assumed to be negligible, all individuals in the evolved population rely heavily on environmental cues but still possess moderate sociality traits. While gradient detection ability helps in accurate determination of migratory route, social tendencies result in formation of stable and coherent groups. Such groups navigate more accurately than solitary individuals due to spontaneous averaging of erroneous individual measurements, as described above. This emergent collective property is often referred to as the “many wrongs principle.”Citation10 Owing to its spatiotemporal dynamics, we call this evolved strategy “collective migration of cohesive groups” ().
Including even relatively small costs for leadership, however, dramatically alters the evolutionary outcome. In this scenario individuals in the evolved population no longer all play the same role.Citation14,Citation15 Only a relatively small proportion of the population follow environmental cues, and these individuals exhibit reduced sociality. The majority, however, employ social cues alone (“social individuals”). This population self-organizes into groups of different sizes where the relatively few gradient climbing individuals act spontaneously as “leaders,” guiding those that employ a social strategy. Strikingly, the proportion of leaders in migratory groups reduces with the group size.Citation8 In addition, leaders dynamically self-sort to frontal or edge, positions of groups while social individuals form rest of the group (). These multitudes of groups merge and split (“fission-fusion”) and, at the level of populations, we find collective migration (). This strategy of “collective migration with fission-fusion” occurs over a very broad range of parameter values.
Costs of sociality may have profound implications on migratory strategies. A general prediction of our model is that if the costs of sociality are greater than those of leadership, the population evolves a homogeneous strategy of leaders with no, or very weak, sociality, resulting in “solitary migration.”Citation15 To an observer, even a collection of solitarily migrating individuals may appear to move collectively (). But, the apparent cohesion and coordination are only due to individuals independently detecting, and following, similar environmental cues.
In an extreme scenario when the costs of gradient detection are very high, it is better to obtain benefits locally than to migrate to farther areas; therefore, no individuals possess leadership, resulting in a “resident population.”Citation15 Specifically, if the costs of sociality are zero, populations will evolve sociality trait leading to “resident swarms,” which are cohesive groups with their center of mass moving randomly (). On the other hand, if the social costs are even modest, no individuals possess either of the traits thus resulting in a “solitary resident strategy” of non-social individuals ().
Memory Effects in the Collapse and Recovery of Migration
Our entire biosphere is under severe threat due to increasing anthropogenic influences, and as a consequence many migrations around the world are at risk.Citation18,Citation19 Our computational and evolutionary approach may offer potentially useful insights into the influence of human activities such as hunting or habitat fragmentation on animal migration. We note that the greater the habitat fragmentation, the smaller the frequency of suitable stop-over or refueling sites, thus larger the distance required to migrate to accumulate nutrients (i.e., migratory benefits). Furthermore, genetically coded migratory behavior can undergo rapid changes on relatively short ecological time scales such as decades, as seen in wild populations of blackcaps.Citation2 In such scenarios, where environmental deterioration and evolutionary processes occur over comparable time scales, our model predicts that number of leaders in the population reduces to zero leading to a collapse of migration.
Changes in migratory patterns have been recorded in many natural populations; for example, migration has disappeared in bison (Bison bison) of North America and wildebeest of the Kalahari.Citation19,Citation20 Wildebeest in the Serengeti may face a similar fate due to a proposed road that bisects the national park in Tanzania.Citation21 Our model predicts a potentially bleak future for such migrants; it suggests that it may be extremely difficult to recover lost migrations. This is because leader mutants are not as favorable, and occur infrequently, in highly fragmented habitats. Much greater habitat restoration is required to recover such lost migrationCitation15 (in the parlance of physical sciences, this is known as the hysteresis effect).
Collective Motion and Phases of Migration: A Complex Adaptive System
In summary, we present a novel approach to the study of leadership, collective motion and migration by looking at plausible origins of these processes. We show how simple trade-offs between individuality (i.e., leadership) and collectivity, result in a wide range of migratory strategies, including resident populations, solitary migrants and those who migrate collectively. Among these, collective migration occurs over a very wide range of ecological assumptions, and within such populations there typically exist a relatively small proportion of leaders who can sense and follow environmental cues, and a majority who migrate by following social cues.
Our model predictions could provide potentially useful insights into conservation and management of migratory species. That leaders are often relatively few in number could mean that collective migrations may be prone to sudden, and practically irreversible, collapse in the event of leader populations undergoing extinction due to inherent fluctuations, habitat fragmentation, disease or through harvesting by humans. It is worth noting that indigenous peoples in the Canadian Arctic hold the belief that migratory caribou herds have few leaders.Citation22 Furthermore, their traditional hunting practices allow the migratory front to pass before beginning the hunt and then they take those from the middle of the herds since this is considered to reduce chances of eliminating leaders, and thus of disrupting migratory routes.Citation23 In light of our model predictions, a rigorous scientific test of this traditional knowledge may aid in designing sound management practices for preserving populations of caribou herds, and to limit their hunting to within sustainable levels. More generally, our work highlights that an approach that considers both proximate and ultimate factors will be crucial for developing strategies for long term conservation of migratory populations.
Broadly speaking, our study integrates the fields of migration and collective motion with that of evolutionary game theory. The population behaviors observed bear comparison with those of physical systems that are composed of a large number of interacting entities. Specifically, migratory states of populations may be considered analogous to distinct macroscopic phases of physical systems such as solids and liquids, or phases of magnets, all of which arise as a consequence of interactions among their microscopic constituents as well as the influence of external conditions. Likewise, we find, and can characterize, distinct “phases of migration” (see above) that correspond to the way in which evolved traits scale to the higher order spatiotemporal dynamics of groups and populations. In our model we can reveal how these collective states relate to key ecological conditions such as the costs of leadership and sociality, and the overall population density.
Although the analogy of macroscopic phases and properties of physical matter to classify collective motion has been widely employed in the literature,Citation7,Citation24,Citation25 we emphasize a key distinguishing feature of biological systems; that the individual level interactions can modify over time as a consequence of mutation and natural selection. Thus, the resulting macroscopic pattern is a consequence of both changes in external conditions as well as adaptation of lower-scale interactions. In other words, biological systems are complex adaptive systemsCitation26 and our results show the importance of this approach to the study of both fundamental and applied aspects of migratory behavior.
Figures and Tables
Figure 1 Spatial organization of evolved migratory populations. We assume positive x-axis (i.e., from left to right) to be the direction of environmental gradients. Individuals are represented by triangles. Green (light grey in black and white) color indicates relatively high gradient detection ability while the blue (dark in black and white) indicates lack of gradient detection ability.
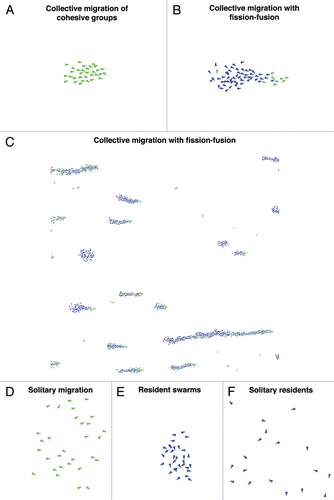
Acknowledgements
We acknowledge support from a Searle Scholar Award 08-SPP-201 to I.D.C., Defense Advanced Research Projects Agency Grant HR0011-05-1-0057 to Princeton University. I.D.C. also acknowledges support from National Science Foundation Award PHY-0848755 and Office of Naval Research Award N00014-09-1-1074. We thank Simon Garnier for the help in producing the figure.
Addendum to:
References
- Kunwar PS, Siekhaus DE, Lehmann R. In vivo migration: a germ cell perspective. Annu Rev Cell Dev Biol 2006; 22:237 - 265
- Pulido F. The Genetics and evolution of avian migration. Bioscience 2007; 57:165 - 174
- Friedl P, Hegerfeldt Y, Tusch M. Collective cell migration in morphogenesis and cancer. Int J Dev Biol 2004; 48:441 - 449
- Fryxell JM, Greever J, Sinclair ARE. Why are migratory ungulates so abundant?. Am Nat 1998; 131:781 - 798
- Alerstam T, Hedenstrom A, Akesson S. Long-distance migration: evolution and determinants. Oikos 2003; 103:247 - 260
- Adler J. Chemotaxis in bacteria. Annu Rev Biochem 1975; 44:341 - 356
- Vicsek T, Czirók A, Ben-Jacob E, Cohen I, Shochet O. Novel type of phase transition in a system of self-driven particles. Phys Rev Lett 1995; 75:1226 - 1229
- Couzin ID, Krause J, Franks NR, Levin SA. Effective leadership and decision making in animal groups on the move. Nature 2005; 433:513 - 516
- Grunbaum D. Schooling as a strategy for taxis in a noisy environment. Evol Ecol 1998; 12:503 - 522
- Simons A. Many wrongs: The advantage of group navigation. Trends Ecol Evol 2004; 19:453 - 455
- Couzin ID. Collective minds. Nature 2007; 445:715
- Torney CJ, Neufeld Z, Couzin ID. Context-dependent interaction leads to emergent search behavior in social aggregates. Proc Natl Acad Sci USA 2009; 106:22055 - 22060
- Dall SRX, Giraldeau LA, Olsson O, McNamara JM, Stephens DW. Information and its use by animals in evolutionary ecology. Trends Ecol Evol 2005; 20:187 - 193
- Torney CJ, Levin SA, Couzin ID. Specialization and evolutionary branching in migratory populations. Proc Natl Acad Sci USA 2010; 107:20394 - 20399
- Guttal V, Couzin ID. Social interactions, information use and the evolution of collective migration. Proc Natl Acad Sci USA 2010; 107:16172 - 16177
- Green S. Particle simulation using CUDA. NVidia Corporation 2007;
- Li H, Kolpas A, Petzold L. Parallel simulation for a fish schooling model on a general-purpose graphics processing unit. Concurrency and Computation: Practice and Experience 2009; 21:725 - 737
- Wilcove DS, Wikelski M. Going, Going, Gone: Is animal migration disappearing?. PLoS Biol 2008; 6:188
- Harris G, Thirgood S, Hopcraft JGC, Cromsigt JPGM, Berger J. Global decline in aggregated migrations of large terrestrial mammals. Endang Species Res 2009; 7:55 - 76
- The decline of the Kalahari wildebeest. Oryx 1992; 26:147 - 150
- Dobson AP, Borner M, Sinclair AR, Hudson PJ, Anderson TM, Bigurube G, et al. Road will ruin Serengeti. Nature 2010; 467:272 - 273
- Padilla E. Defining caribou leaders in traditional knowledge of the Porcupine Caribou herd. Caribou leadership: A study of traditional knowledge, animcal behavior and policy 2010; Fairbanks University of Alaska Masters Thesis
- Gunn A, Arlooktoo G, Kaomayak D. Freeman MMR, Carbyn LN. The contribution of the ecological knowledge of Inuit to wildlife management in the Northwest Territories. Traditional Knowledge and Renewable Resource Management in Northern Regions. Traditional Knowledge and Renewable Resource Management. Boreal Institute for Northern Studies, Edmonton, Alberta 1988; 23:Spinage, CA Occasional Publication 22 - 29
- Ramaswamy S. The Mechanics and statistics of active matter. Ann Rev Cond Mat Phys 2010; 1:323 - 345
- Cavagna A, Cimarelli A, Giardina I, Parisi G, Santagati R, Stefanini F, et al. Scale-free correlations in starling flocks. Proc Natl Acad Sci USA 2010; 107:11865 - 11870
- Levin SA. Complex adaptive systems: exploring the known, the unknown and the unknowable. Bull Am Math Soc 2003; 40:3 - 19