Abstract
Epigenetic mechanisms, including DNA methylation, are important determinants in development and disease. There is a need for technologies capable of detecting small variations in methylation levels in an accurate and reproducible manner, even if only limited amounts of DNA are available (which is the case in many studies in humans). Quantitative methylation analysis of minute DNA amounts after whole bisulfitome amplification (qMAMBA) has been proposed as an alternative, but this technique has not been adequately standardized and no comparative study against conventional methods has been performed, that includes a wide range of methylation percentages and different target assays. We designed an experiment to compare the performance of qMAMBA and bisulfite-treated genomic (non-amplified) DNA pyrosequencing. Reactions were performed in duplicate for each technique in eight different target genes, using nine artificially constructed DNA samples with methylation levels ranging between 0% and 100% with intervals of 12.5%. Cubic polynomial curves were plotted from the experimental results and the real methylation values and the resulting equation was used to estimate new corrected data points. The use of the cubic regression-based correction benefits the accuracy and the power of discrimination in methylation studies. Additionally, dispersion of the new estimated data around a y = x line (R2) served to fix a cutoff that can discriminate, with a single 9-point curve experiment, whether whole bisulfitome amplification and subsequent qMAMBA can produce accurate methylation results. Finally, even with an optimized reagent kit, DNA samples subjected to whole bisulfitome amplification enhance the preferential amplification of unmethylated alleles, and subtle changes in methylation levels cannot be detected confidently.
Introduction
DNA methylation plays a crucial role in normal development and has relevant effects on gene regulation, X chromosome inactivation and genomic stabilization through transcriptional silencing of repetitive elements.Citation1 In humans, DNA methylation occurs mostly in cytosines within CpG dinucleotides, which are underrepresented in the genome because of their high mutation rate, but are especially abundant in 5′ regulatory regions of genes.Citation2
Common complex diseases with strong genetic and environmental risk determinants are excellent targets for the study of gene methylation, which is known to link environmental factors and the genome. Moreover, most common complex diseases have a progressive and quantitative nature that could be partly explained by accumulating epigenetic variation.Citation3 For instance, differences in DNA methylation levels have been identified in the frontal cortex of patients diagnosed with major psychotic symptoms, in genomic regions that had been previously linked to disease etiology.Citation4
Studies in monozygotic twins have demonstrated purely epigenetic variation underlying complex traits, as is the case of the decrease in methylation in several genes associated with systemic lupus erythematosus.Citation5 SNP associations detected by genome wide association (GWA) studies have been revisited in order to identify parent of origin effects or differentially methylated transcription factor binding sites and have been successful in the case of different diseases such as type 1 and type 2 diabetes.Citation3 It is now believed that the integration of epigenomic and genomic analyses will help reveal novel functional determinants within associated loci and several associations between epigenomic perturbations and human diseases have been reported.Citation6
On the other hand, several studies have provided evidence on the existence of hundreds of genes with differential methylation of their promoters in various types of cancers, and such genes have been shown to be consistent and reproducible across different data sets and publications.Citation7 Global hypomethylation of the genome and hypermethylation at specific gene promoters are known to be partly responsible for important events in carcinogenesis, including chromosomal instability and transcriptional silencing of tumor suppressor genes.Citation8,Citation9 Although robustly replicated across studies, these methylation changes are often quantitatively subtle, as in breast cancer, where the absolute differences between tumoral and normal tissues are below 5%.Citation10 Thus, it is necessary to develop technologies capable of determining slight variations in methylation levels in an accurate and reproducible manner.
Clinical tissue specimens from particular stages of a disease are limited and often irreplaceable, making those DNA samples very valuable material for research. Quantitative methylation analysis of minute DNA amounts after whole bisulfitome amplification (qMAMBA) has been proposed as a solution for limited sample availability (for example, in the case of DNA extracted from body fluids).Citation11,Citation12 Taking into account that acceptable methods for epigenetic studies should ensure that subtle methylation differences are robustly detected, we designed a study to compare qMAMBA and bisulfite-treated DNA pyrosequencing in terms of accuracy and reproducibility.
In this work, we validate the previously proposed cubic polynomial regression correction methodCitation13 and use it to fix a cutoff, based on a single 9-point dilution curve experiment that can determine whether whole bisulfitome amplification and subsequent qMAMBA technology can be performed without producing an artifactual result.
Results and Discussion
Following the experimental design outlined in , we compared the performance of conventional genomic DNA pyrosequencing and qMAMBA in a methylated DNA dilution curve (0%, 12.5%, 25%, 37.5%, 50%, 62.5%, 75%, 87.5% and 100% methylated human DNA) for eight Pyromark methylation assays located in different genes (MALT1, MAP3K7, MAP3K7IP1, MAP3K14, NFKBIA, RELA, TNFAIP3 and TRADD), that were selected because of their central regulatory functions in NFκB and Toll-like receptor responses, and their interest in studies on inflammation and autoimmunity. A 9-point cubic polynomial curve was plotted with the results from each of the target genes, with real methylation levels in the x axis and experimentally obtained, Pyromark Q24 software derived values for each point of the curve in the y axis. Curves for all genes fitted very well a polynomial cubic equation (R2 > 0,975) (Table S1). For each experimental result, x was solved to correct for PCR bias, and estimated data presented b values very close to 1, confirming the high level of correction of PCR bias that is obtained with the cubic equation based correction,Citation13 compared with the raw pyrosequencing results (). When these estimated values were plotted against the real methylation levels, linear regression showed very high coincidence with a y = x straight line () and data-points fitted very well to the function, as shown by correlation coefficients (), especially for the conventional pyrosequencing experiments.
Table 1. Raw and corrected b values, sums of squared errors of the differences between estimated and real methylation levels, and coefficients of variation between replicates are shown for each gene and methodology, expressed as average values of the 9 points of the curve and standard deviations
Figure 2. Degree of bias and correction in each experiment. In all graphs, the x axis represents the real methylation levels, while the experimental (black diamonds and squares) and estimated (gray triangles) values are on the y axis. The derived cubic polynomial curve is shown for one of the sets of experimental results (diamonds), presenting in most cases a preferential amplification of unmethylated alleles (b < 1, except for MAP3K7 with conventional pyrosequencing). Gray triangles plot the real values vs. the levels estimated after the cubic equation-based correction and are fitted to a y = x linear regression (discontinuous line). Linear correlation coefficients are shown for each experiment.
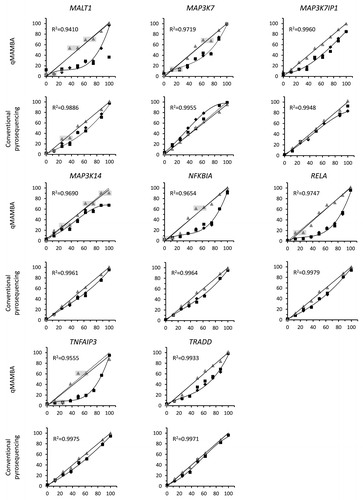
Assays with a correlation coefficient higher than 0.99 in the estimated vs. real value linear regression () were able to discriminate clearly between all adjacent points of the 9-point methylation curves. This was the case in 7 out of 8 target genes analyzed with the conventional pyrosequencing method but only in 2 of the genes assayed with qMAMBA. In the plots with lower correlation values, several adjacent points of the dilution curve (differing in 12.5% methylated DNA) were scored with similar estimated values and could not be discriminated, as highlighted in .
Sum of squared errors were calculated in order to quantify the accumulated difference between real and estimated methylation values with different target genes and techniques. In general, sum of squared error values were notably higher in the qMAMBA results. On the other hand, variation coefficients and their standard deviations across the different curve points were also calculated and showed more variation between replicates in the case of qMAMBA results ().
As previously mentioned, in order to be able to assess the contribution of variation in DNA methylation to human disease, subtle changes in methylation levels must be robustly detected in the wider range of disorders as possible using small amounts of DNA. Technical approaches such as qMAMBA could be very useful in methylation analyses, but they should prove to be reliable enough. Previous analyses of whole bisulfitome amplified samples addressed only extreme differences in methylation levels, which indeed are correctly discriminated.Citation11,Citation12 The present work is the first study to compare qMAMBA and bisulfite-treated genomic DNA pyrosequencing in terms of accuracy and reproducibility in a systematic way, covering a wide range of methylation percentages and different target assays.
In general, our study shows that the performance of assays is worse when bisulfitome amplification precedes PCR and pyrosequencing reactions, suggesting an inflation of PCR bias in this technique, due to a very strong preferential amplification of unmethylated alleles in qMAMBA. The only exception is MAP3K7IP1, which shows a very similar performance by both conventional pyrosequencing and qMAMBA. In this work, we demonstrate that qMAMBA method can only be satisfactorily applied if the R2 value derived from the linear regression for the corrected vs. the real methylation values is very close to 1 (> 0.99) and thus, dispersion of estimations from the straight line is small enough to avoid stretches of overlapping values in which methylation differences cannot be accurately calculated (). Although less frequent, the same precautions should also be taken with conventional pyrosequencing.
In conclusion, we use the previously proposed cubic polynomial regression correction method and provide evidence on its efficiency in correcting the PCR bias. Moreover, we show that b values depart much more from 1 when calculated with uncorrected observed methylation values compared with corrected estimations, so that systematic use of the cubic regression-based correction enormously benefits both the accuracy and the power of discrimination in methylation studies.
Finally, we fix a cutoff that allows distinguishing between those assays that will have a satisfactory performance from those unable to discriminate subtle changes in methylation levels, by performing a single 9-point curve experiment. This threshold establishes that in order to avoid artifactual results, the correlation coefficient (R2) should be higher than 0.99 when a linear regression between the estimated and the real methylation values is plotted, following cubic polynomial equation correction of the raw results.
Methods
DNA samples
Methylation curves were constructed combining different amounts of commercially available HeLa Genomic DNA (used as unmethylated DNACitation14) and CpG Methylated HeLa Genomic DNA (representing totally methylated human genomic DNA), both from New England Biolabs (cat. nos. N4006S and N4007S, respectively) (). The resulting methylation levels of the curve where the following: 0%, 12.5%, 25%, 37.5%, 50%, 62.5%, 75%, 87.5% and 100%, all in a final volume of 20 µl and a DNA concentration of 100 ng/µl.
Sodium bisulfite conversion of unmethylated cytosines was performed in each sample of the curve using the commercially available Epitect Bisulfite Kit (Qiagen cat. no. 59104) as indicated by the manufacturer, and column-purified into 40 µl. An aliquot (5 µl) of each sample was used to amplify the bisulfitome with the Epitect Whole Bisulfitome Amplification Kit (Qiagen cat. no. 59205) following the manufacturer’s instructions. Briefly, around 250 ng bisulfite treated DNA were amplified using 1 µl of REPLI-g Midi Polymerase in a final volume of 40 µl. Reactions were incubated at 28°C for 8h, followed by a 95°C step of 5 min and then kept overnight at 4°C. Finally, 30 µl each of bisulfite treated genomic and amplified samples were diluted 1:2 in ddH2O and used for PCR reactions.
Selection and pyrosequencing of the CpG islands
Eight predesigned, commercially available Pyromark CpG Assays (Qiagen) located in eight different genes (MALT1, MAP3K7, MAP3K7IP1, MAP3K14, NFKBIA, RELA, TNFAIP3 and TRADD) were selected. Each of the assays covered 4–6 CpG islands located in the 5′ non coding end and/or in the first exon of each target, regions known to be involved in gene expression regulation and thus, interesting candidates for subsequent disease related studies ().
Table 2. Pyromark CpG Assay list
Each of the pyrosequencing experiments was performed in duplicate, so that two sets of results were available for each target gene and approach. Amplifications of the loci of interest were performed in a C1000 Thermal Cycler (BioRad) using 1.5 µl of DNA in 25-µl PCR reactions with the Pyromark PCR Kit (Qiagen cat. no. 978703) and the forward and the biotinylated reverse specific primers provided with each Pyromark CpG Assay, following the manufacturer’s protocol. Thermocycling conditions were as follows: 15 min at 95°C for enzyme activation followed by 45 cycles of denaturation for 30 sec at 95°C, annealing for 30 sec at 56°C and extension for 30 sec at 72°C, with a final extension of 10 min at 72°C. PCR reactions were tested by agarose gel electrophoresis.
After PCR amplification, 20 µl of each biotinylated PCR product were bound to streptavidin coated sepharose beads (GE Healthcare cat. no. 17-5113-01) in 24-well plates. PCR products were denatured and non-biotinylated strands were removed using the Pyromark Q24 vacuum workstation. The beads were then resuspended in 25 μl of annealing buffer containing 0.3 μM of the specific sequencing primer from each CpG Assay. Pyrosequencing with the Pyromark Q24 system was performed using the Pyro Gold Q24 reagents. Nucleotide, enzyme and substrate dispensation order and volumes, as well as the duration of each step, were planned using the Pyromark Q24 software v2.0 (all from Qiagen) based on the target sequence. Following the runs, raw data files were imported into the software for standard quality controls and preliminary analyses.
Data analysis and Statistics
For each target gene and experimental approach (direct pyrosequencing of genomic DNA or qMAMBA), results were fitted into a polynomial cubic equation (y = ax3 + cx2 + dx + e), with the real methylation levels plotted on the x axis and the observed, experimental values (extracted from de Pyromark Q24 software) on the y axis, using Microsoft Excel v.10.0. With this equation, methylation levels were estimated for each experimental result by solving the x with Cardano’s method, as previously described.Citation15 Adjustment and accuracy of these estimations with respect to the real methylation levels were measured by linear correlation coefficients (R2).
The value of b, a measure of amplification bias that reflects the efficiency of primer binding and polymerase elongation in amplicons with differential composition of methylated and unmethylated DNA, was calculated for each curve point with the following equation: b = [y × (100 - x)]/[x × (100 - y)], where y is the uncorrected experimental or estimated and x is the real value. As described previously,Citation13 b = 1 indicates unappreciable PCR bias, while preferential amplification of unmethylated alleles results in b < 1.
Average b values (representing an overall b value of each particular gene), sums of squared errors of the differences between estimated methylation values and real levels, and coefficients of variation between replicates and their standard deviations were calculated for each gene and approach using Prism v5.0 (GraphPad Software Inc.).
Additional material
Download Zip (103.5 KB)Acknowledgments
This work was partially funded by Research Project grants from the Instituto de Salud Carlos III of the Spanish Ministry of Economy and Competitiveness (PI10/0310) and from the Basque Department of Industry (SAIO-PE08BF03). NF-J and LP-I are predoctoral fellows supported by FPI grants from the Basque Department of Education, Universities and Research (BIF-2009-099 and BIF-2010-189, respectively). The funders had no role in study design, data collection and analysis, decision to publish, or preparation of the manuscript.
Disclosure of Potential Conflicts of Interest
No potential conflicts of interest were disclosed.
Supplemental Materials
Supplemental materials may be found here: www.landesbioscience.com/journals/epigenetics/article/22846
References
- Bestor TH. The DNA methyltransferases of mammals. Hum Mol Genet 2000; 9:2395 - 402; http://dx.doi.org/10.1093/hmg/9.16.2395; PMID: 11005794
- McCabe MT, Brandes JC, Vertino PM. Cancer DNA methylation: molecular mechanisms and clinical implications. Clin Cancer Res 2009; 15:3927 - 37; http://dx.doi.org/10.1158/1078-0432.CCR-08-2784; PMID: 19509173
- Bell CG, Beck S. The epigenomic interface between genome and environment in common complex diseases. Brief Funct Genomics 2010; 9:477 - 85; http://dx.doi.org/10.1093/bfgp/elq026; PMID: 21062751
- Mill J, Tang T, Kaminsky Z, Khare T, Yazdanpanah S, Bouchard L, et al. Epigenomic profiling reveals DNA-methylation changes associated with major psychosis. Am J Hum Genet 2008; 82:696 - 711; http://dx.doi.org/10.1016/j.ajhg.2008.01.008; PMID: 18319075
- Javierre BM, Fernandez AF, Richter J, Al-Shahrour F, Martin-Subero JI, Rodriguez-Ubreva J, et al. Changes in the pattern of DNA methylation associate with twin discordance in systemic lupus erythematosus. Genome Res 2010; 20:170 - 9; http://dx.doi.org/10.1101/gr.100289.109; PMID: 20028698
- Rakyan VK, Down TA, Balding DJ, Beck S. Epigenome-wide association studies for common human diseases. Nat Rev Genet 2011; 12:529 - 41; http://dx.doi.org/10.1038/nrg3000; PMID: 21747404
- Yao C, Li H, Shen X, He Z, He L, Guo Z. Reproducibility and concordance of differential DNA methylation and gene expression in cancer. PLoS One 2012; 7:e29686; http://dx.doi.org/10.1371/journal.pone.0029686; PMID: 22235325
- Yoo CB, Jones PA. Epigenetic therapy of cancer: past, present and future. Nat Rev Drug Discov 2006; 5:37 - 50; http://dx.doi.org/10.1038/nrd1930; PMID: 16485345
- Yang AS, Estécio MR, Doshi K, Kondo Y, Tajara EH, Issa JP. A simple method for estimating global DNA methylation using bisulfite PCR of repetitive DNA elements. Nucleic Acids Res 2004; 32:e38; http://dx.doi.org/10.1093/nar/gnh032; PMID: 14973332
- Wang S, Dorsey TH, Terunuma A, Kittles RA, Ambs S, Kwabi-Addo B. Relationship between tumor DNA methylation status and patient characteristics in African-American and European-American women with breast cancer. PLoS One 2012; 7:e37928; http://dx.doi.org/10.1371/journal.pone.0037928; PMID: 22701537
- Vaissière T, Cuenin C, Paliwal A, Vineis P, Hoek G, Krzyzanowski M, et al. Quantitative analysis of DNA methylation after whole bisulfitome amplification of a minute amount of DNA from body fluids. Epigenetics 2009; 4:221 - 30; PMID: 19458486
- Paliwal A, Vaissière T, Herceg Z. Quantitative detection of DNA methylation states in minute amounts of DNA from body fluids. Methods 2010; 52:242 - 7; http://dx.doi.org/10.1016/j.ymeth.2010.03.008; PMID: 20362673
- Moskalev EA, Zavgorodnij MG, Majorova SP, Vorobjev IA, Jandaghi P, Bure IV, et al. Correction of PCR-bias in quantitative DNA methylation studies by means of cubic polynomial regression. Nucleic Acids Res 2011; 39:e77; http://dx.doi.org/10.1093/nar/gkr213; PMID: 21486748
- Qian ZR, Sano T, Yoshimoto K, Yamada S, Ishizuka A, Mizusawa N, et al. Inactivation of RASSF1A tumor suppressor gene by aberrant promoter hypermethylation in human pituitary adenomas. Lab Invest 2005; 85:464 - 73; http://dx.doi.org/10.1038/labinvest.3700248; PMID: 15711568
- Jacobson N. Basic Algebra. Dover Pubn Inc 2009; Mineola, NY.