Abstract
Besides differential methylation, DNA methylation variation has recently been proposed and demonstrated to be a potential contributing factor to cancer risk. Here we aim to examine whether differential variability in methylation is also an important feature of obesity, a typical non-malignant common complex disease. We analyzed genome-wide methylation profiles of over 470,000 CpGs in peripheral blood samples from 48 obese and 48 lean African-American youth aged 14–20 y old. A substantial number of differentially variable CpG sites (DVCs), using statistics based on variances, as well as a substantial number of differentially methylated CpG sites (DMCs), using statistics based on means, were identified. Similar to the findings in cancers, DVCs generally exhibited an outlier structure and were more variable in cases than in controls. By randomly splitting the current sample into a discovery and validation set, we observed that both the DVCs and DMCs identified from the first set could independently predict obesity status in the second set. Furthermore, both the genes harboring DMCs and the genes harboring DVCs showed significant enrichment of genes identified by genome-wide association studies on obesity and related diseases, such as hypertension, dyslipidemia, type 2 diabetes and certain types of cancers, supporting their roles in the etiology and pathogenesis of obesity. We generalized the recent finding on methylation variability in cancer research to obesity and demonstrated that differential variability is also an important feature of obesity-related methylation changes. Future studies on the epigenetics of obesity will benefit from both statistics based on means and statistics based on variances.
Introduction
Recently, it has been reported that increased methylation variability may be an important feature of some malignant human diseases, such as cancer.Citation1 These increased epigenetic variances may reflect adaptation to the exposure to environmental risk factors. Hansen et al. were the first to propose that cancer tissues present increased methylation variation in regions that are differentially methylated between cancer and normal tissues.Citation2 Another genome-wide methylation study by Teschendorff et al. in cervical cancer further demonstrated the added value of differential variability by showing its ability of significantly improving the sensitivity and detection of cervical cancer risk.Citation3,Citation4 These studies gave novel insights and impetus to epigenetic research indicating that, in addition to differentially methylated CpG sites (DMCs), differentially variable CpG sites (DVCs) may also play an essential role in human disease development and progression.
The epidemic of obesity has imposed a huge burden on human health worldwide.Citation5,Citation6 Obesity is an important risk factor for various diseases, including cardiovascular diseases,Citation7,Citation8 type 2 diabetes (T2D)Citation9 and certain types of cancer,Citation10 such as breastCitation11 and colon cancer.Citation12 As a typical common complex disease, obesity is the result of the interplay between external (environmental) and internal (genetic) factors.Citation13 Epigenetics has been suggested as the molecular mechanism mediating this interplay. The recent epigenome-wide association studies (EWAS) have identified several DMCs or differentially methylated CpG regions related to obesity.Citation14,Citation15 However, the potential role of DVCs in obesity has never been explored.
Based on the genome-wide methylation profiling from 48 obese cases and 48 lean controls, in this study we aim to examine whether differential variability is also an important feature of obesity related methylation changes. DVCs using statistics based on variances and DMCs using statistics based on means were first identified. Independent prediction of obesity status was then tested to demonstrate the importance of DVCs and DMCs. Gene ontology analysis was also performed to provide some functional interpretations of these CpG sites. Finally, to demonstrate their roles in the etiology and pathogenesis of obesity, we tested whether the genes harboring the DVCs or DMCs showed significantly enrichment of genes identified by genome-wide association studies on obesity and its related diseases. This is the first study exploring the contribution of methylation variance to a non-malignant common complex disease.
Results
Obesity related DMCs and DVCs
For both the analyses on DMCs and DVCs between obese cases and lean controls, histograms of P-values ( and ) indicated a substantial number of CpG sites that were associated with obesity status. In total, we found 23,305 DMCs and 28,653 DVCs with FDR < 0.05. There were 2,360 CpG sites that overlapped between DMCs and DVCs (), a significant enrichment [odds ratio (OR) 1.82 with 95% confidence interval (CI) 1.74–1.90, Fisher’s exact P-value < 2.2E-16, P.permutation < 0.001], indicating there are some common features between DMCs and DVCs. These CpG sites were defined as differentially methylated and variable CpG sites (DMVCs).
Figure 1. DMC, DVCs and DMVCs. (A) Density histograms of DMCs (differentially methylated CpG sites). These P-values were derived from linear regression based on the Limma package comparing differences in means between lean and obese subjects. (B) Density histograms of DVCs (differentially variable CpG sites). These P-values were derived from Bartlett’s test comparing differences in variances between lean and obese. (C) Venn diagram illustrating DMCs, DVCs and DMVCs (differentially methylated and variable CpG sites). The overlapping DMVCs were significantly enriched with P.Fisher < 2.2E-16 and P.permutation (1000 times) < 0.001. (D) Top ranked DMC cg08339189. Y-axis shows the β value, x-axis the sample. Phenotypes were indicated as lean (black, n = 48) and obese (red, n = 48). The dashed lines show the mean levels in lean (0.15) and obese (0.18) separately. (E) Top ranked DVC cg24570070. The mean levels were not significantly different (0.88 in obese vs. 0.91 in lean). However, obese cases showed a large methylation variance. (F) Top ranked DMVC cg00033915. The mean levels (dashed line) were significantly different between the two groups (0.92 in obese vs. 0.93 in lean, raw p = 1.1E-5 and FDR = 4.2E-3). Furthermore, the obese group also showed significantly larger variance.
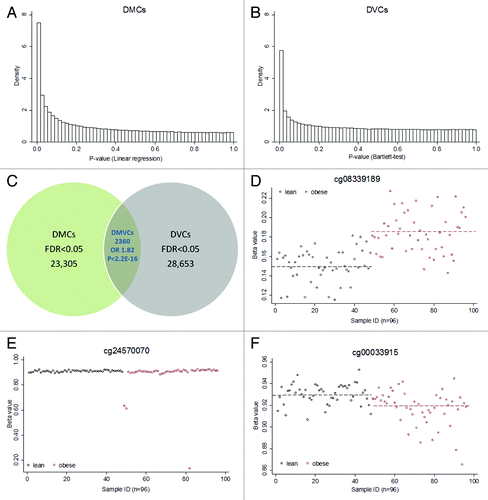
Examples of typical DMCs, DVCs and DMVCs were presented in , and , respectively. In contrast with DMCs, which showed more homogenous differential methylation changes, DVCs generally exhibited an outlier structure with the increased or decreased variability caused by large changes in DNA methylation present in only a small number of “outlier” samples. About 9.45% of DVCs (2,707 out of 28,653) were driven by single outliers (defined as only one sample displaying > 20% change and all the other samples displaying < 5% change in DNA methylation).Citation3 As expected, DMVCs showed features of both DMCs and DVCs with relatively homogeneous differential methylation changes but larger variance in the obese group.
Distributions of obesity related DMCs, DVCs and DMVCs
showed the distributions of the three types of CpG sites across their average β values () and across their genomic locations (). Similar to the overall distribution of DNA methylation measured by the Illumina 450K chip in peripheral blood leukocytes, the average methylation levels of obesity related DMCs, DVCs and DMVCs were primarily present in hypomethylated (β-values < 20%) and hypermethylated (β-values > 80%) categories. However, there was a significant difference (p < 2.2E-16) in the percentage of the CpG sites within these two categories for these three types, with more DMCs in the hypomethylated category and more DVCs and DMVCs in the hypermethylated category. Similarly, we observed the genomic distributions of DMCs, DVCs and DMVCs were significantly different (p < 2.2E-16). CpG sites within CpG island and promoter region including TSS1500, TS200, 1st exon were more likely to be differentially methylated, while CpG sites within the open sea and gene body regions were more likely to be differentially variable sites. This is consistent with the distributions of the three types of CpG sites across average β values because CpG sites in the open sea and gene body regions tend to be hypermethylated while CpG sites in CpG islands and promoter regions tend to be hypomethylated.
Figure 2. Distributions of DMCs, DVCs and DMVCs. (A) Distributions across average methylation levels. The Y-axis represents the density, x-axis the average methylation β value. The orange line presented the kernel density curve of overall CpG sites across β 0–1, the green line presented DMCs, the gray line DVCs and the blue line DMVCs. The percentages of each of these four types of CpG sites with betas below 0.2 or above 0.8 are also listed in the plot. (B) Distribution across genomic locations. Chi-square test found significant difference among their distributions across the genome with P-value < 2.2E-16. TSS200, CpG sites within 200bp from the transcription starting site (TSS); TSS1500, CpG sites within 200–1500bp from the transcription starting site (TSS); body, gene body.
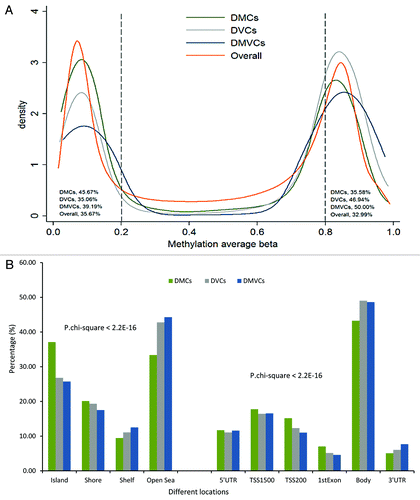
Increased methylation variance in obese cases
Next, we explored whether the DMCs would be more hypermethylated and DVCs would be more variable in obese cases than in lean controls. These two characteristics have been shown in previous cancer studies. showed the scatterplot of the mean difference and the variance difference for the DMCs, DVCs and DMVCs. In agreement with previous cancer studies, 68.3% of DVCs (left upper quadrant 42.4% plus right upper quadrant 25.9%) were more variable in obese cases. The DMVCs presented an even stronger trend with 84.0% (57% plus 27%) being more variable in obese cases. These DMVCs, which showed more variable in obese cases, were defined as hyper-DMVCs. However, we did not observe a more hypermethylated DMCs profile in obese cases with 50.3% of the DMCs (31.8% plus 18.5%) being more hypermethylated in cases and 49.7% (31.1% plus 18.6%) being more hypermethylated in controls. To exclude the possibility that this different feature we observed in obesity was caused by the different methylation platforms used in this study (Illumina 450K) compared with previous cancer studies (Illumina 27K), we redid this analysis limited to the CpG sites on the Illumina 27K chip (). For the CpG sites on the 27K chip, it clearly showed that obesity related DMCs were more hypermethylated in obese cases with 78.8% (55.2% plus 23.6%) of the DMCs being more hypermethylated. Since most CpG sites in the 27K chip are located within promoter regions, we repeated the analysis according to genomic regions to test whether this DMC skew toward hypermethylation in cases for 27K chip data will also display in promoter regions of the 450K chip. As is shown In Table S2, we did not observe that the DMCs in the promoter regions including TSS1500, TS200 and 1st exon in the 450K were more hypermethylated in cases than in controls. On the other hand, DVCs on the 27K chip () and DVCs in different genomic regions (Table S3) showed a consistent pattern of more variability in obese cases. This indicates that increased methylation variance in cases is a common feature for obesity and cancer, but that the increased methylation levels seem to be platform dependent.
Figure 3. Increased methylation variance in obese. (A) Scatter plot of mean methylation difference (x-axis) against methylation variance ratio (y-axis) comparing lean and obese. The percentage of DMCs, DVCs and DMVCs within each of the four quadrants was also listed. (B) Scatter plot of mean methylation difference (x-axis) against methylation variance ratio (y-axis) comparing lean and obese limited to the CpG sites on the illumina 27K chip. The percentage of DMCs, DVCs and DMVCs within each of the four quadrants was also listed.
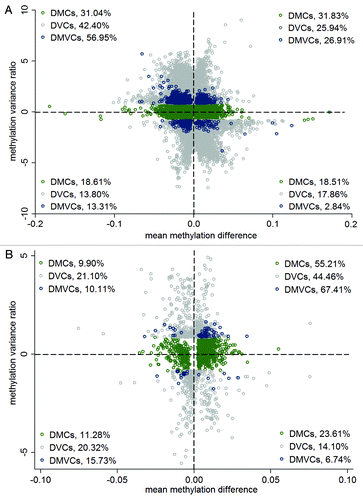
Predictive ability of DMCs and DVCs
To demonstrate the importance of differential variability and differential methylation in obesity, we split our sample into 2 and tested whether the DMCS and DVCs identified from the 1st sample can independently predict the obesity status of the 2nd sample. ROC analysis was used to test the predictive ability of DMCs and DVCs and the results are shown in . Both types of CpG sites significantly predicted obesity case-control status in the independent validation sample. The AUC of DMCs was 0.69 with 95% CI: 0.54 to 0.81, while this was 0.70 (0.56–0.85) for DVCs. There was no statistical difference between their prediction abilities. It suggested that both DMCs and DVCs are important features of obesity.
Figure 4. Predictive ability of DMCs and DVCs. (A) Predictive ability of DMCs. The receiver operating characteristic (ROC) analysis showed the area under curve (AUC) and its 95% confidence interval (CI) in a randomly split testing set (24 obese vs. 24 lean) from the whole data set. (B) Predictive ability of DVCs. AUC and 95%CI was presented in a randomly split testing set (24 obese vs. 24 lean) from the whole data set.
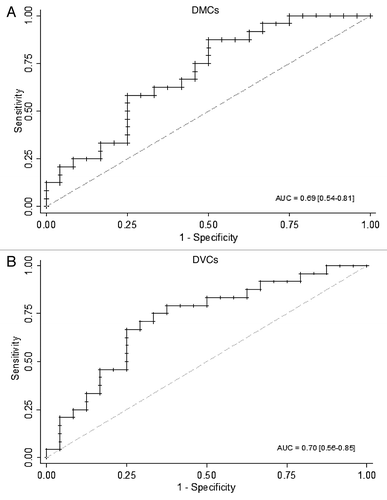
Gene ontology analysis of DMCs, DVCs and DMVCs
To demonstrate their unique contributions as well as to provide some functional interpretations of DMCs and DVCs, genes in the top 500 DMCs, the top 500 DVCs and all the DMVCs were selected for gene ontology analysis. The reason that the top 500 DMCs and DVCs were selected is that we were interested in the unique feature of DMCs and DVCs and there was no overlapped between the top 500 CpGs of these two lists (a significant underenrichment, P.permutation < 0.001). shows the top ten pathways for the three lists. DNA binding (9.0E-6), development (p = 5.4E-5), regulation of neurogenesis (p = 1.1E-4), cell differentiation (p = 1.6E-4) and transcription regulation (p = 1.4E-4) were among the top list for DMCs, while DVCs were significantly enriched in polymorphisms (protein for which there is at least one variant within the same species, that is not directly responsible for a disease) (p = 2.3E-5) and alternative splicing (p = 7.1E-5). DMVCs were enriched in both alternative splicing (p = 3.6E-18) and transcription regulation (p = 6.0E-7). It further showed the strongest enrichment in the phosphoprotein pathway (p = 1.7E-29).
Figure 5. Gene ontology enrichment analysis of DMCs, DVCs and DMVCs. Gene ontology analysis was performed using DAVID (http://david.abcc.ncifcrf.gov). The human genome was used as background. The top 500 DMCs (A), the top 500 DVCs (B) and all DMVCs (n = 1608) (C) were selected for analysis. The top ten enriched pathways are listed here together with their enrichment P-values, which are derived from a modified Fisher’s exact test.
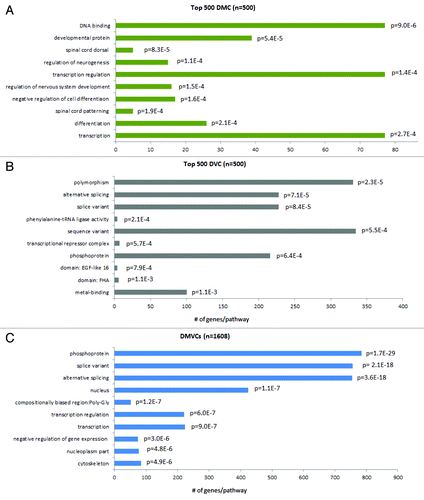
Enrichment of GWAS genes for obesity and comorbidities
Based on recent genome-wide methylation studies on T2D which observed significant excesses of differentially methylated sites in genomic regions previously identified through GWAS,Citation16 we explored whether genes in obesity related DMCs and DVCs would show significant enrichment of GWAS genes for obesity. The results were shown in . Both the DMCs (OR = 2.70, p = 2.9E-6) and DVCs genes (OR = 1.97, p = 0.001) showed significant enrichment of obesity GWAS genes. The enrichment was even stronger in the DMVCs (OR = 3.38, p = 2.9E-6) and hyper-DMVCs genes (OR = 5.1E-7). The significant overlap between GWAS and EWAS signals indicates that these genes are very important with either their sequence variants or methylation changes contributing to the risk of obesity. The even stronger enrichment of GWAS genes in the hyper-DMVCs group suggests that increased DNA methylation variability in combination with differential methylation is an important feature of key genes related to obesity.
Table 1. Enrichment analysis of DMCs, DVCs, DMVCs and Hyper-DMVCs in obesity and its comorbidities
As obesity is a major risk factor for T2D, dyslipidemia, hypertension and certain types of cancer including breast cancer and colon cancer, we further explored whether obesity related DMCs and DVCs genes would show significant enrichment of GWAS identified genes for these diseases. As shown in , both the DMCs and DVCs genes showed enrichment of GWAS genes for these diseases to a certain degree. This indicates that either the differential methylation or the differential variability of these genes may be involved in the development of obesity related diseases. To provide some clues of the roles of obesity related DMCs and DVCs genes played in the mechanisms of obesity related cancer risk, we also explored whether these genes would show significant enrichment of tumor suppressor genes and oncogenes and observed a significant enrichment of these genes in both the list of DMCs, DVCs, DMVCs and hyper-DMVC genes ().
Discussion
Recent evidence has shown that epigenetic variance may be an important contributor to cancer risk. In this study, we generalize this finding from the cancer field to another typical complex disease, obesity. Here we demonstrate for the first time, in the context of genome-wide DNA methylation profiling on obesity cases and lean controls, that differential variability is also an important feature of obesity related methylation changes. Similar to DMCs, DVCs can independently predict obesity status. Similar to previous cancer studies, DVCs show more variability in cases than in controls. Furthermore, the genes harboring these CpG sites showed significant enrichment of genes identified by GWAS on obesity and obesity related diseases, supporting their roles in the etiology and pathogenesis of obesity.
DVCs, which may reflect the adaption to changing environments,Citation1 presented increased variability in obese cases in our study. In Hansen’s study,Citation2 which focused on 384 CpG sites covering 139 differentially methylated regions between colon cancer and normal tissues, the vast majority of CpGs showed larger methylation variance in cancer samples than in normal samples. This is a common feature across several human cancer types including colon, lung, breast, thyroid and Wilms’ tumors. Teschendorff’s studyCitation3 on different stages of cervix cancer extended this finding to the genome-wide level and observed that DMCs were more hypermethylated and DVCs were more variable in cancer cases. This skew may reflect the choice of the Infinium 27K methylation platform used in which most CpG sites are located within promoter regions and are usually unmethylated in the normal state. In this study which used the 450K Infinium methylation platform (a much denser methylation array with probes across the genome including the gene body and open sea regions), we still observed a substantial skew toward hypervariability in obese cases, indicating that increased methylation variance in cases is a common feature of cancer and obesity and this feature is independent of the platforms used. This increased variation has been suggested to contribute to tumor heterogeneity or as an index of earlier stage of carcinogenesis.Citation2-Citation4 The increased methylation variability in obese may also contribute to its pathogenesis heterogeneity.
In this study, we firmly demonstrated that the statistics based on variability could identify true positives as reliably as the statistics based on differential methylation. Both DVCs and DMCs independently predicted obesity status in a second set of samples. The importance of DVCs was further demonstrated by the enrichment analysis of obesity GWAS genes. Similar to the recent genome-wide methylation studies on T2DCitation16 which observed significant excesses of DMCs in genomic regions previously identified through GWAS, we observed this feature for both DMCs and DVCs in obesity, this is, genes harboring DMCs as well as genes harboring DVCs displayed significantly enrichment of obesity genes identified by GWAS. There are several possible explanations for this enrichment. First, these DMCs or DVCs may be allele-specific methylation sites (ASM) which represent methylation changes that purely result from obesity associated SNPs. Second, these DMCs or DVCs may be under genetic control of obesity associated SNPs but their levels and variances are not solely determined by DNA sequence [methylation QTLs (mQTLs)]. In this case, the DMCs or DVCs may act as the interplay between DNA sequence variants and environmental factors (gene-environment interaction) and have the potential to amplify the genetic signals. This is supported by a recent study on rheumatoid arthritis by Liu et al.Citation17 in which they observed 9 DMC in MHC regions (a major genetic risk region for rheumatoid arthritis), which potentially mediate the relationships between SNPs and rheumatoid arthritis disease risk. Five out of the nine DMCs also showed a significant association between genotype and variance of methylation. Third, these DMCs or DVCs may purely represent environmental exposures. If this is the case, the over-representation of these DMCs or DVC in obesity GWAS genes will indicate that these genes are very important for the etiology of obesity in which either sequence variants or epigenetic variations may change the gene functions and contribute to obesity. All the 3 scenarios suggest that these DMCs or DVCs in obesity GWAS genes are likely to represent causes rather than consequences of obesity. Obesity induced methylation changes (i.e., consequences) might be enriched in genes responsible for its comorbidities. This is also the case for both DMCs and DVCs with genes harboring DMCs or DVCs showing significant enrichment of GWAS genes for obesity related diseases such as hypertension, dyslipidemia, type 2 diabetes and certain type of cancers. This indicates that DMCs and DVCs are important players in both obesity etiology and pathogenesis of its comorbidities.
The overlap of DMCs and DVCs identified a set of important CpG sites (DMVC). This set showed even stronger enrichment of obesity GWAS genes. Additionally, the DMVCs presented a more noticeable trend of increased methylation variability in obesity cases compared with DVCs. This part of DMVCs was defined as hyper-DMVCs, which contains the majority feature of DMVCs and exhibits the strongest enrichment of obesity GWAS genes. Another advantage of DMVCs is that this set of CpGs does not have a single outlier structure with only one of the DMVCs driven by single outliers. Similar to Teschendorff’s 3 strategy of using the intersection of age related CpG sites and DVCs to perform the biomarker selection, the DMVCs in the current study may help to find the more relevant obesity markers. In a recent GWAS study,Citation18 one SNP in the FTO gene, which has been associated with mean BMI and obesity in previous studies, displayed significant association with BMI variability. DNA methylation has been suggested as the potential mediator of this gene-environment interaction. In the current study, the hyper-DMVCs include one CpG site in the FTO gene (cg02642561 located in gene body region, with linear regression FDR 0.03 and Bartlett’s test FDR 0.03) and this CpG site or other CpG sites closely linked to this CpG site may explain the observed SNP’s effect on both the means and the variance of BMI. This speculation needs to be tested in a data set including both SNP and methylation information.
Several limitations of this study need to be recognized. First, we used the DNA from leukocytes, which represent different cell populations with distinct epigenetic profiles. Data on white blood cell counting with 5-part differential (neutrophils, eosinophils, basophils, monocytes and lymphocytes) as well as data on flow cytometry of CD4+ cells and CD8+ cells are available for some of the cases (n ranges from 27–42) and some of the controls (n ranges from 41–45) of the current study participants. Based on these data, we did not observe differences in the proportions of these available cell types between obese cases and lean controls (Table S4). Therefore, it is highly unlikely that our findings were biased by shifts in these leukocyte subpopulations although we cannot exclude the possibility that other unmeasured cell types might have this effect. Furthermore, although there is a possibility that some of the DMCs are driven by leukocyte subset composition, it seems unlikely that the DVCs are driven by the cell population differences because of their outlier structure. Second, all the participants in this study are youth and young adults aged 14–20 y old. The advantage of focusing on youth is that the results will not be confounded by obesity comorbidities or medication use, both of which are very common in adult subjects with obesity. However, in consideration of the strong effect of age on DNA methylation, generalization of the current findings to adult or older population needs to be cautious. Third, we used two independent statistical methods to identified DMCs and DVCs, which may not be very efficient. A novel approach combining the mean and variance statistics will be more helpful to identify epigenetic risk loci.
In conclusion, we generalized the recent finding on methylation variability in cancer research to obesity and demonstrated that differential variability is also an important feature of obesity related methylation changes. Future studies on epigenetics of obesity will benefit from both statistics based on means and statistics based on variability.
Materials and Methods
Subjects
We selected 48 obese (24 males and 24 females) and 48 age- and gender-matched lean African-American (AA) participants from the EpiGO (EpiGenetic Basis of Obesity Induced Cardiovascular Disease and Type 2 Diabetes) study. The general characteristics of these 96 samples are listed in Table S1. The EpiGO study was established in 2011 with the goal of identifying methylation changes involved in the pathogenesis of obesity and its related co-morbidities. Currently it is still ongoing and will in total enroll 400 obese and 400 lean youth aged 14–20 y with roughly equal number of AAs and European Americans (EA) as well as males and females. All the subjects will be recruited from the southeastern United States.
The Institutional Review Board at the Georgia Health Science University had given approval for this study. Written informed consent was provided by all subjects or by parents if subjects were less than 18 y. This study is performed in accordance with the principles expressed in the Declaration of Helsinki.
For all the participants in the EpiGO study, height and weight were measured by standard methods using a wall-mounted stadiometer and a scale, respectively. Body mass index (BMI) was calculated as weight/height.Citation2 The inclusion criteria are as follows: (1) age ≥ 14 but < 21; (2) BMI ≥ 30kg/m2 or BMI ≥ 9 5th percentile for age and sex if age ≤ 20 for obese cases and BMI < 25kg/m2 or BMI < 50th percentile for age and sex if age ≤ 20 for lean controls; (3) free of any acute or chronic illness; (4) no daily medication controls for diseases; (5) EAs or AAs with both parents of the subjects reporting being of European or African ancestry, respectively.
Fasting peripheral blood samples were collected. DNA was extracted from the peripheral leukocytes using the QIAamp DNA Mini Kit (QIAGEN).
Genome-wide methylation assay
Genome-wide methylation analysis was performed by Illumina Infinium Human Methylation 450K Beadchip (Illumina Inc.). This chip quantitatively measures more than 450,000 CpG sites at single nucleotide resolution with 99% coverage of RefSeq Gene and 96% coverage of CpG islands. It covers regions across the whole genome with probes distributed in CpG islands, CpG shores, CpG shelves, open sea, 5′UTR, promoter regions, first exon, gene body and 3′UTR. After bisulfite treatment, 200ng converted whole genome amplification DNA was purified, applied and hybridized to the BeadChips. Illumina HiScan was used to scan the assays at the Genomic Facility of the University of Chicago. The intensity of the image was extracted with the Genome Studio Methylation Software Module (Illumina Inc.) according to the manufacturer’s recommendation. Initial array processing and quality control were also performed with BeadStudio software. The methylation β (β) values are constrained to lie between 0 (completely unmethylated) and 1 (completely methylated), which represents the ratio of the intensity of the methylated bead type to the combined locus intensity. To minimize any batch effect, each chip, which can accommodate 12 samples, included 3 samples from each group of the following 4 groups: obese males, obese females, lean males and lean females.
Statistical analysis
The database of basic characteristics of the participants was managed by Stata SE version 12 (StataCorp). For the genome-wide methylation data analysis, R-based open source software packages were used including Limma (Linear Models for Microarray Data),Citation19 PAMR (popular shrunken centroid predication algorithm),Citation20 and EVORA (Epigenetic variable outliers for risk prediction analysis).Citation4
Quality control and normalization
CpG sites on the X and Y chromosomes and CpG sites with detection P-value ≥ 0.01 in more than 25% of the samples were excluded prior to data analysis. All these 96 samples had at least 99% of CpG sites with detection P-value < 0.01, thus there were no samples removed. After these quality checks, there were a total of 473,778 CpG sites from all the 96 samples imported into data analysis. Quartile normalization was performed before analysis. About 18.7% of the 450K probes contain SNPs and the presence of these SNPs may trigger some of the observed effect. However, when these probes were excluded from the analyses, the results were virtually unchanged. So results for all the qualified probes are reported here.
Differentially methylated CpG sites
To find the DMCs, the Limma package was used under the design matrix of a two group test. A raw P value was assigned to each CpG site based on the empirical Bayes shrinkage from the designed linear model output. Raw P-values were converted to false discovery rates (FDR) based on Benjamini and HochbergCitation21 to correct for multiple testing. A FDR value of 0.05 was used as the threshold in the current study.
Differentially variable CpG sites
Bartlett’s test in the EVORA package was used to find the DVCs. A raw P value was assigned to each CpG site, as a means of selecting differentially variable features where the differential variability is driven by a potentially small number of outliers. Benjamini and Hochberg based FDR values were also used to correct for multiple testing. To be consistent with DMCs, we chose the same FDR threshold of 0.05 for DVCs selection.
Predictive ability of DMCs and DVCs
To test the predictive ability of DMCs and DVCs, we sought to develop a classifier using the DMCs or DVCs identified in the discovery (or training) set followed by the validation of this classifier in an independent validation (or testing) set. To do so, we randomly split the whole data set (48 obese vs. 48 lean) into equally sized training (24 obese vs. 24 lean) and testing (24 obese vs. 24 lean) sets.
To select the classifier from DMCs in the training set, PAMR was used, which was based on differences in means. Similarly, an adaptive index perdition algorithm called EVORA was performed to find the feature classifier from DVCs, which was based on variance difference. Before using EVORA, the β value was transformed into a COPA (Cancer Outlier Profile Analysis) value to better identify outlier induced differential variation. For both PAMR and EVORA, a 10-fold internal cross-validation procedure was used to build the feature classifiers. The prediction performances of the identified classifiers were examined in the independent testing set using the area under curve (AUC) of ROC (Receiver Operating Characteristic) analysis.
Gene ontology enrichment analysis
Gene ontology analysis was performed using DAVID (the Database for annotation visualization and Integrated Discovery v6.7) (http://david.abcc.ncifcrf.gov). The human genome was used as background and the enrichment P-values were derived from a modified Fisher’s exact test. The top 500 DMCs genes, the top 500 DVCs genes and all the DMVCs genes (differentially methylated and variable CpG sites) were imported into the analysis. The top ten enriched pathways were exported from the output.
Enrichment analysis in genes responsible for obesity and its comorbidities
Genome-wide associated studies (GWAS) genes for obesity and its related diseases including type 2 diabetes, hypertension, dyslipidemia, breast cancer and colon cancer were identified from publications as well as the GWAS catalog (http://www.genome.gov/gwastudies). The list of tumor suppressor genes and oncogenes were taken from the Memorial Sloan-Kettering Cancer Center (MSKCC) cancer database (http://cbio.mskcc.org/CancerGenes/Select.action). The gene lists were provided on Table S5.
The valid genes covered by 450K (n = 19,751) were used as the reference for the enrichment analysis. Fisher’s exact test was used to assign raw P-values. A test based on 1000 permutations was further performed to get a precise P-value under the null hypothesis.
Abbreviations: | ||
AUC | = | area under curve |
CI | = | confidence interval |
DMCs | = | differentially methylated CpG sites |
DMVCs | = | differentially methylated and variable CpG sites |
DVCs | = | differentially variable CpG sites |
EPIGO | = | Epigenetic Basis of Obesity Induced Cardiovascular Disease and Type 2 Diabetes study |
EVORA | = | epigenetic variable outliers for risk prediction analysis |
EWAS | = | epigenome-wide association study |
GWAS | = | genome-wide association study |
hyper-DMVCs | = | DMVCs presented hyper variable in obese |
OR | = | odds ratio |
PAMR | = | popular shrunken centroid predication algorithm |
ROC | = | receiver operating characteristic |
SNP | = | single nucleotide polymorphism |
T2D | = | type 2 diabetes |
Additional material
Download Zip (182.9 KB)Acknowledgments
This study was supported by grants HL105689 from National Institute of Health (NIH).
Disclosure of Potential Conflicts of Interest
No potential conflicts of interest were disclosed.
References
- Feinberg AP, Irizarry RA. Evolution in health and medicine Sackler colloquium: Stochastic epigenetic variation as a driving force of development, evolutionary adaptation, and disease. Proc Natl Acad Sci U S A 2010; 107:Suppl 1 1757 - 64; http://dx.doi.org/10.1073/pnas.0906183107; PMID: 20080672
- Hansen KD, Timp W, Bravo HC, Sabunciyan S, Langmead B, McDonald OG, et al. Increased methylation variation in epigenetic domains across cancer types. Nat Genet 2011; 43:768 - 75; http://dx.doi.org/10.1038/ng.865; PMID: 21706001
- Teschendorff AE, Jones A, Fiegl H, Sargent A, Zhuang JJ, Kitchener HC, et al. Epigenetic variability in cells of normal cytology is associated with the risk of future morphological transformation. Genome Med 2012; 4:24; http://dx.doi.org/10.1186/gm323; PMID: 22453031
- Teschendorff AE, Widschwendter M. Differential variability improves the identification of cancer risk markers in DNA methylation studies profiling precursor cancer lesions. Bioinformatics 2012; 28:1487 - 94; http://dx.doi.org/10.1093/bioinformatics/bts170; PMID: 22492641
- Danaei G, Ding EL, Mozaffarian D, Taylor B, Rehm J, Murray CJ, et al. The preventable causes of death in the United States: comparative risk assessment of dietary, lifestyle, and metabolic risk factors. PLoS Med 2009; 6:e1000058; http://dx.doi.org/10.1371/journal.pmed.1000058; PMID: 19399161
- Misra A, Khurana L. Obesity and the metabolic syndrome in developing countries. J Clin Endocrinol Metab 2008; 93:Suppl 1 S9 - 30; http://dx.doi.org/10.1210/jc.2008-1595; PMID: 18987276
- Poirier P, Giles TD, Bray GA, Hong Y, Stern JS, Pi-Sunyer FX, et al, American Heart Association, Obesity Committee of the Council on Nutrition, Physical Activity, and Metabolism. Obesity and cardiovascular disease: pathophysiology, evaluation, and effect of weight loss: an update of the 1997 American Heart Association Scientific Statement on Obesity and Heart Disease from the Obesity Committee of the Council on Nutrition, Physical Activity, and Metabolism. Circulation 2006; 113:898 - 918; http://dx.doi.org/10.1161/CIRCULATIONAHA.106.171016; PMID: 16380542
- El-Atat F, Aneja A, Mcfarlane S, Sowers J. Obesity and hypertension. Endocrinol Metab Clin North Am 2003; 32:823 - 54; http://dx.doi.org/10.1016/S0889-8529(03)00070-7; PMID: 14711064
- Rathmann W, Giani G. Global prevalence of diabetes: estimates for the year 2000 and projections for 2030. Diabetes Care 2004; 27:2568 - 9, author reply 2569; http://dx.doi.org/10.2337/diacare.27.10.2568; PMID: 15451946
- Anderson AS, Caswell S. Obesity management--an opportunity for cancer prevention. Surgeon 2009; 7:282 - 5; http://dx.doi.org/10.1016/S1479-666X(09)80005-X; PMID: 19848061
- Morimoto LM, White E, Chen Z, Chlebowski RT, Hays J, Kuller L, et al. Obesity, body size, and risk of postmenopausal breast cancer: the Women’s Health Initiative (United States). Cancer Causes Control 2002; 13:741 - 51; http://dx.doi.org/10.1023/A:1020239211145; PMID: 12420953
- Larsson SC, Wolk A. Obesity and colon and rectal cancer risk: a meta-analysis of prospective studies. Am J Clin Nutr 2007; 86:556 - 65; PMID: 17823417
- Obesity: preventing and managing the global epidemic. Report of a WHO consultation. World Health Organ Tech Rep Ser 2000; 894:i - xii, 1-253; PMID: 11234459
- Wang X, Zhu H, Snieder H, Su S, Munn D, Harshfield G, et al. Obesity related methylation changes in DNA of peripheral blood leukocytes. BMC Med 2010; 8:87; http://dx.doi.org/10.1186/1741-7015-8-87; PMID: 21176133
- Feinberg AP, Irizarry RA, Fradin D, Aryee MJ, Murakami P, Aspelund T, et al. Personalized epigenomic signatures that are stable over time and covary with body mass index. Sci Transl Med 2010; 2:49ra67; http://dx.doi.org/10.1126/scitranslmed.3001262; PMID: 20844285
- Toperoff G, Aran D, Kark JD, Rosenberg M, Dubnikov T, Nissan B, et al. Genome-wide survey reveals predisposing diabetes type 2-related DNA methylation variations in human peripheral blood. Hum Mol Genet 2012; 21:371 - 83; http://dx.doi.org/10.1093/hmg/ddr472; PMID: 21994764
- Liu Y, Aryee MJ, Padyukov L, Fallin MD, Hesselberg E, Runarsson A, et al. Epigenome-wide association data implicate DNA methylation as an intermediary of genetic risk in rheumatoid arthritis. Nat Biotechnol 2013; 31:142 - 7; http://dx.doi.org/10.1038/nbt.2487; PMID: 23334450
- Yang J, Loos RJ, Powell JE, Medland SE, Speliotes EK, Chasman DI, et al. FTO genotype is associated with phenotypic variability of body mass index. Nature 2012; 490:267 - 72; http://dx.doi.org/10.1038/nature11401; PMID: 22982992
- Smyth GK. Linear models and empirical bayes methods for assessing differential expression in microarray experiments. Stat Appl Genet Mol Biol 2004; 3:e3; http://dx.doi.org/10.2202/1544-6115.1027; PMID: 16646809
- Tibshirani R, Hastie T, Narasimhan B, Chu G. Diagnosis of multiple cancer types by shrunken centroids of gene expression. Proc Natl Acad Sci U S A 2002; 99:6567 - 72; http://dx.doi.org/10.1073/pnas.082099299; PMID: 12011421
- Hochberg Y, Benjamini Y. More powerful procedures for multiple significance testing. Stat Med 1990; 9:811 - 8; http://dx.doi.org/10.1002/sim.4780090710; PMID: 2218183