Abstract
Women with advanced stage ovarian cancer (OC) have a five-year survival rate of less than 25%. OC progression is associated with accumulation of epigenetic alterations and aberrant DNA methylation in gene promoters acts as an inactivating “hit” during OC initiation and progression. Abnormal DNA methylation in OC has been used to predict disease outcome and therapy response. To globally examine DNA methylation in OC, we used next-generation sequencing technology, MethylCap-sequencing, to screen 75 malignant and 26 normal or benign ovarian tissues. Differential DNA methylation regions (DMRs) were identified, and the Kaplan–Meier method and Cox proportional hazard model were used to correlate methylation with clinical endpoints. Functional role of specific genes identified by MethylCap-sequencing was examined in in vitro assays. We identified 577 DMRs that distinguished (p < 0.001) malignant from non-malignant ovarian tissues; of these, 63 DMRs correlated (p < 0.001) with poor progression free survival (PFS). Concordant hypermethylation and corresponding gene silencing of sonic hedgehog pathway members ZIC1 and ZIC4 in OC tumors was confirmed in a panel of OC cell lines, and ZIC1 and ZIC4 repression correlated with increased proliferation, migration and invasion. ZIC1 promoter hypermethylation correlated (p < 0.01) with poor PFS. In summary, we identified functional DNA methylation biomarkers significantly associated with clinical outcome in OC and suggest our comprehensive methylome analysis has significant translational potential for guiding the design of future clinical investigations targeting the OC epigenome. Methylation of ZIC1, a putative tumor suppressor, may be a novel determinant of OC outcome.
Introduction
Ovarian cancer (OC) causes more deaths than any other gynecologic malignancy.Citation1 Five-year survival rates have only marginally improved over the past three decades, and women with advanced stage OC have a five-year survival rate of less than 25%.Citation1 Although most patients respond to platinum-based chemotherapy, relapses are common, leading to platinum-resistant OC, which is uniformly fatal.Citation2,Citation3
Similar to other malignancies, OC progression is associated with a redistribution of DNA methylation, characterized by genomic hypomethylation with localized CpG island (CGI) hypermethylation.Citation4-Citation6 Such changes are associated with both OC initiation and progression to chemotherapy resistance.Citation7 Hypermethylation of specific CGI-containing genes in OC has been shown to repress the expression of tumor suppressors, such as RASSF1A, BRCA1, DAPK and OPCML, and development-associated transcription factors, such as HOXA10 and HOXA11.Citation6,Citation8,Citation9 Individual methylated genes with possible prognostic value in OC include HOXA11, linked with post-surgical residual tumor and overall poor prognosis,Citation9 MCJ, strongly associated with chemoresistance,Citation10 and 14–3-3-σ, significantly correlated with elevated serum CA-125 levels and high grade malignancy.Citation11 In addition, panels of hypermethylated loci, identified using various approaches, have been shown to represent possible biomarkers for disease detection and prognosis in advanced OC.Citation12-Citation16
In the present study, we used a next generation sequencing approach, methyl-CpG binding domain of the MBD2 protein to capture double-stranded DNA followed by high-throughput next- generation sequencing (MethylCap-seq) to assess the methylation status of malignant and benign ovarian tumors and normal ovarian tissues. We identified differential DNA methylation profiles as well as candidate hypermethylated loci. We validated the functional consequence of hypermethylation-mediated silencing of the Hedgehog (Hh) signal transduction pathway which has been shown to be involved in human ovarian carcinogenesis and chemotherapy drug sensitivity.Citation17,Citation18 In addition, we have generated perhaps the most comprehensive methylome analysis to date in OC, which we believe has strong translational potential for guiding the design of future clinical investigations targeting the epigenome of this deadly female malignancy.
Results
MethylCap-seq analysis of the ovarian tumor methylome
To investigate methylation signatures associated with poor outcome in OC, genome-scale DNA methylation profiles were obtained for 75 tumors, 20 benign ovarian samples (unmatched) and 6 normal (unmatched) ovarian tissues (; Table S1). A sequencing depth of ten million uniquely mapped reads provided sufficient coverage for methylation mapping (, histogram). In addition, repeated sequencing was performed on two samples (Fig. S1A), and the data were highly (R2 > 0.9) correlated.
Figure 1. Strategy for discovering novel methylated genes in ovarian cancer using MethylCap-seq. (A) Flowchart of the experimental design. (B) Histograms show the number of mapped reads in 101 ovarian samples. At least 10 million uniquely mapped reads per sample were used in the analysis. Pie charts illustrating the percentage of differential methylation regions (DMRs) of promoter CpG islands (CGI) and non-promoter CGI. (C) Methylation profiles of 577 DMRs locate at ± 4 kb spanning from transcription start site (TSS) in malignant, benign and normal ovarian samples. Each row shows a DMR region overlapping a TSS. Average of the methylation levels was calculated for 75 malignant (left), 20 benign (middle) and 6 normal (right) ovarian tissues. Each 200 base pairs is a bin to show the methylation profiles in an 8K region. (D) Area under the receiver operating curves (AUC) shows the accuracy for distinguishing malignant samples and non-malignant samples (containing benign and normal). The average methylation level of 577 DMRs and top 10% DMRs (n = 57) shows higher 87% accuracy for distinguishing malignant and non-malignant ovarian tissue.
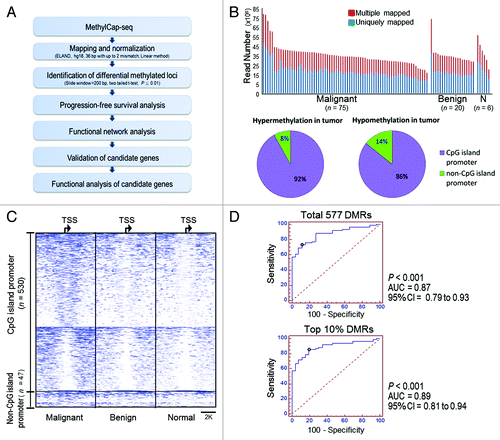
To identify differentially methylated regions (DMRs) in malignant vs. non-malignant (20 benign and 6 normal ovarian tissues) ovarian tissues, an area spanning 4 kb upstream or downstream of the nearest transcription start site (TSS) was used. This approach yielded 577 DMRs in the tumors, and these DMRs represented the vast majority hypermethylated CGIs (92%) and 86% of hypomethylated tumor CGIs (, pie charts). Methylation profiles of the 577 DMRs were more similar in benign and normal tissues compared with tumor (; Fig. S1B and C). Furthermore, as shown in , malignant from non-malignant samples could be distinguished with 87% accuracy by using the average of 577 normalized methylation levels (AUC = 0.87, 95%CI = 0.79 to 0.93, p < 0.001), and with 89% accuracy when the top 10% of significantly methylated DMRs were used (AUC = 0.89; 95% CI = 0.81 to 0.94, p < 0.001).
To examine the association between DMRs and progression-free survival (PFS), methylation regions closer to the TSS (2 Kb upstream or downstream in the 577 DMRs) were used. This analysis yielded 63 DMRs significantly associated with PFS (), as determined by the Cox regression model (Table S2, adjusted for stage, grading, age and histological type). The relationship between survival probability and methylation frequency was then examined using Kaplan-Meier estimates (; Fig. S2A). DMRs were first dichotomized into high- or low- methylation using the 60th percentile cut-off value (described above), and the number of high methylation DMRs (HM-DMRs) was calculated for each patient. Next, as the area under the ROC curve (AUC) indicated that 17 HM-DMRs were highly accurate (p < 0.001) predictors of recurrence (Fig. S2B), HM-DMRs were dichotomized as high or low frequency. At 60 mo, high frequency HM-DMRs correlated (p < 0.001) with reduced PFS (17.5% survival probability), and low frequency HM-DMRs correlated (p < 0.001) with long PFS (87.3% survival probability) (, top panel). Only two HM-DMRs correlated (p < 0.05) with long PFS (Fig. S3). In addition, when only advanced stage tumors were assessed, high frequency HM-DMRs correlated (p < 0.001) with reduced PFS (11% PFS probability at 40 mo), compared with an 80% PFS probability at 40 mo for low frequency HM-DMRs (, bottom panel). A similar trend was observed in early stage tumors, as high frequency HM-DMRs tended to be associated with shorter PFS and low frequency HM-DMRs with longer PFS (Fig. S4); however, statistical significance was not achieved (p = 0.092) due to low rate of recurrence in Stage 1, 2 OC and a smaller sample size.
Figure 2. A panel of DMRs associated with prognostic outcome and pathogically functional pathways. (A) Heatmap illustrating the methylation levels of 63 DMRs at promoter regions (± 2Kb spanning the transcription start site (TSS). The DMRs are listed in Table S2. High methylation-DMR (HM-DMR) was defined by 60th percentile of each gene in 75 malignant samples for prognostic analysis. Malignant tissues derived from patients with recurrent disease were associated with HM-DMRs (black gradient). (B) High frequency HM-DMRs (high freq HM-DMRs) were associated with poor progression-free survival (PFS). freq, frequency. (C) Restored gene expression in ovarian cells after epigenetic drug treatments. Expression of each gene examined was restored in at least one of the ovarian cell lines. (D) Network analysis showing the closest functions for high methylated genes. The weight of the edge indicates the probability of genes to connect components (contained gene or proteins) in the functional pathways. Red squares represent proteins involved in signaling pathway and blue circles represent genes. The blue gradient indicates methylation level.
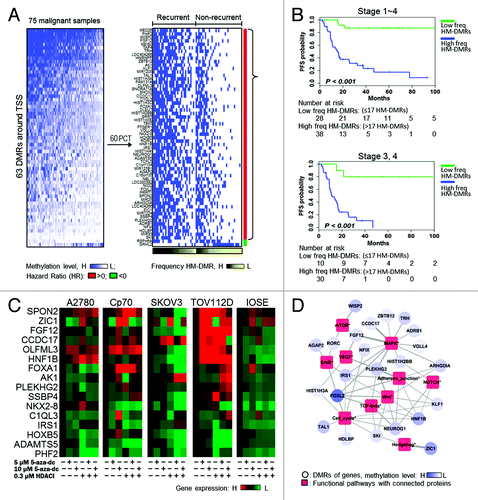
Identification and validation of functionally relevant DMR genes in ovarian cancer
Based on the MethylCap-seq results, we selected 14 genes not previously shown to be hypermethylated in ovarian tumors and two genes (HNF1B and HOXB5) reported to be methylated in OC. Epigenetic (5-aza-dc +/− HDACI) drug treatment restored expression of all 16 genes in at least one of the ovarian cell lines examined (), and SPON2 and ZIC1 reexpression was observed in five cells. In addition, functional roles of genes associated with the 53 DMRs (10 DMRs were eliminated because they corresponded to hypothetical proteins or noncoding RNA) and OC pathogenesis were investigated using the Protein Interaction Network Analysis (PINA) to search for DMR-pathway associations. As shown in , a significant number of genes (23/63 genes) were connected to functional networks involving cancer-relevant pathways such as mTOR, MAPK, VEGF, ErbB, Adherens Junction, NOTCH, Wnt, TGF-β, Cell Cycle and Hedgehog Signaling.
ZIC1 and ZIC4 methylation and clinical correlations
PINA network analysis identified a differentially methylated gene, the putative tumor suppressor ZIC1 (, lower right corner), as closely linked with hedgehog (Hh) signaling. Detailed analysis of the MethylCap-seq data showed that both ZIC1 and ZIC4 (another ZIC family member) were arranged in a “head-to-head” configuration () with overlapping CGIs (referred to as CpG islands 1–9). These regions were hypermethylated in OC, in contrast to modest methylation of ZIC family members ZIC2 and ZIC5 (located at 13q32 and overlapping CGIs 10–17) in benign and normal tissues (). A strong correlation (Pearson’s correlation r > 0.80) was observed between methylation of CGI 4 and CGIs 1, 2, 5, 6 and 8 (). Interestingly, CGI 4 overlapped the ZIC1 promoter and first exon, as well as the putative ZIC4 promoter, and CGIs 1, 2, 5 and 6 overlapped the ZIC1 and ZIC4 coding regions, with only CGI 8 located in an intergenic region ().
Figure 3. Analysis of ZIC family methylation patterns. (A) Methylation profiles of CpG islands located at regions around ZIC1, ZIC4, ZIC2 and ZIC5 on the genome browser. The left panel shows Chr3: 148,584,017 ~14,869,093 and contains nine CGIs (CGI 1~9); right panel shows Chr13: 99,401,912 ~99,450,871 and contains eight CGIs (CGI 10~17). Around ZIC1 and ZIC4, the region presents high methylation in malignant samples. However, some malignant samples showed low methylation (middle of the genome browser). (B) Estimating correlations between CGIs. High concordance of methylated CGI at chromosome 3 but not at chromosome 13. CGI 4 is highly concordant with CGI 1, 2, 5 and 6, and the CGIs related to exon loci. (C) Validation of methylation levels of ZIC1 promoter used bisulfite pyrosequencing. Validated regions were located 439 to 496 base pair upstream from the TSS (transcriptional orientation indicated by the arrow). The red boxes show that methylation levels of 86 malignant samples are higher (p < 0.001 by Mann-Whitney U test) than 35 non-malignant samples (containing 15 benign, 6 normal tissues and 14 normal paraffin tissues) and squares indicate outliers. (D) Area under the receiver operating curves (AUC) was used to estimate the accuracy of predicting malignant samples associated by methylation levels. The average of seven CG sites accurately distinguished 80% of malignant samples using a 41.7% methylation cutoff value.
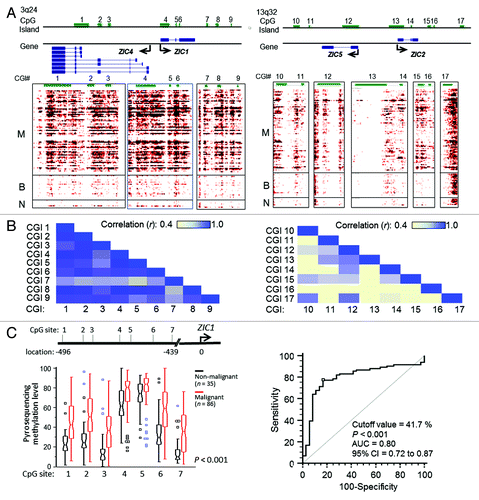
Because the sonic hedgehog pathway plays an important role in OC cell proliferationCitation18-Citation20 and the above results indicated that methylation-mediated silencing of ZIC1, a tumor suppressor,Citation21,Citation22 might result in loss of its inhibitory effects and promote more aggressive ovarian tumor behavior, we validated ZIC1 hypermethylation in ovarian tumors. Pyrosequencing was used to quantify methylation levels at seven CpG sites in the ZIC1 promoter. ZIC1 methylation was higher (p < 0.001 by Mann-Whitney U-test) in 86 malignant compared with 35 non-malignant (15 benign and 20 normal) ovarian tissues (). Furthermore, ZIC1 methylation accurately (p < 0.001; AUC = 0.80) discriminated malignant from non-malignant ovarian tissues (95%CI = 0.72 to 0.87; ), indicating that hypermethylated ZIC1 could serve as an OC biomarker.
ZIC1 expression negatively correlates with GLI methylation patterns and transcription
To broaden the scope of our findings, we analyzed gene expression and methylation array data from The Cancer Genome Atlas (TCGA; 406 ovarian tumors).Citation23 Sample groups (n = 5) based on increasing ZIC1 methylation levels also showed reduced (p < 0.001) ZIC1 expression (; Fig. S5). In addition, by treating ovarian cells with the demethylating agent 5-aza-dc and observing ZIC1 and ZIC4 re-expression, we further confirmed that ZIC1 and ZIC4 were silenced by DNA methylation (Fig. S6A).
Figure 4. Correlation of ZIC expression and hypermethylation in patient samples and GLI expression in ovarian cells. (A) Comparison of methylation and gene expression in 406 ovarian tumors from The Cancer Genome Atlas (TCGA).Citation23 The methylation level is according the AVG β-value (0 to 1 represents low to high methylation in the 27K methylation bead array). Using the same interval of AVG β divides the 5 groups. The gene expression directly used the level 3 data. ZIC1 methylation was negatively correlated (p < 0.001) with ZIC1 expression. (B) The association between expression of ZIC1, ZIC4, and GLIs in ovarian cells treated Hedgehog (Hh) and/or DNA methylation inhibitor. Repressed expression of GLIs in SKOV3 and Cp70 after treatment with Hh inhibitor. Restored ZIC1 and ZIC4 expression was associated with reduced GLI2 in SKOV3 and Cp70. Ctrl, no drug treatment. (C) GLIs expression changes after transient transfection of ZIC1 or ZIC4. High expression of exogenous ZIC1 and ZIC4 was significantly associated with reduced GLI2 in SKOV3 and Cp70. (*p < 0.05; **p < 0.01 and ***p < 0.001 by two-tailed t-test).
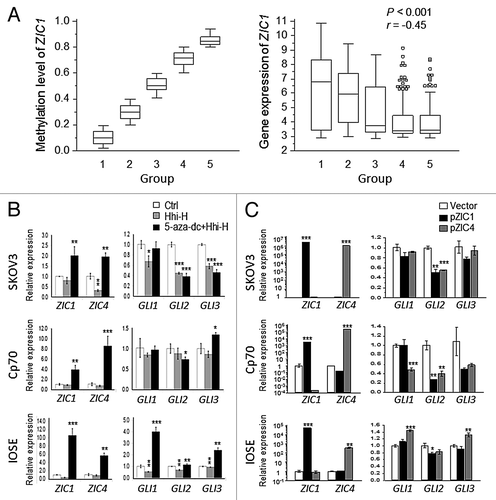
As ZIC and GLI proteins have been shown to transcriptionally regulate one other,Citation24 it was of interest to determine whether ZIC1 and ZIC4 regulate the expression of the Hh effectors GLI1, GLI2 and GLI3 in OC. Treatment of ovarian cell lines with the Hh inhibitor cyclopamine resulted in repressed GLIs expression but no corresponding change in expression of ZIC1 and ZIC4 (). However, the combined treatment of Hh inhibitor plus 5-aza-dc resulted in reexpression of ZIC1 and ZIC4 in all three cell lines examined (Cp70, SKOV3, IOSE), reduced GLI2 expression in SKOV3 and Cp70, and reduced GLI3 expression in SKOV3 (). Furthermore, transfection of ZIC1 or ZIC4 resulted in reduced expression of GLI2 in both OC cell lines as well as IOSE, albeit to a lesser extent (). Enforced ZIC1 or ZIC4 expression suppressed GLI1 and GLI2 levels in Cp70 (). Taken together, the data indicate that ZIC1and ZIC4 negatively regulate Hh signaling by suppressing the expression of GLI2 and possibly GLI1 in OC.
Functional consequences of ZIC1 and ZIC4 overexpression
We next investigated the effects of transient ZIC1 and ZIC4 overexpression on cell proliferation, migration and invasion. Overexpression of ZIC1 inhibited (p < 0.05) proliferation of both OC cell lines tested (SKOV3 and Cp70) compared with vector control, while ZIC4 only reduced Cp70 cell proliferation (Fig. S7). However, overexpressing either ZIC1 or ZIC4 in SKOV3 cells reduced (p < 0.001) both migration and invasion (). Taken together, the above data indicated that silencing of ZIC1 and ZIC4 in ovarian tumors, due to promoter methylation, results in loss of negative regulation and contributes the OC progression.
Figure 5.ZIC1 or ZIC4 overexpression modulates ovarian cancer cell migration/invasion and ZIC1 methylation is related to disease outcome. (A and B) Effect of overexpression of ZIC1 or ZIC4 on cell migration and invasion in SKOV3. Representative results of cells stained with Hochest33324 and results of quantitative migration and invasion assays are shown. The results of three independent experiments were analyzed by Mann-Whitney U test (p < 0.001). (C) High methylation CpG sites at ZIC1 promoter are related to poor progression free survival. The cutoff values used to dichotomize high- and low- methylation for CpG site 6 and 7 were 52.59 and 30.03, respectively (see Table S3).
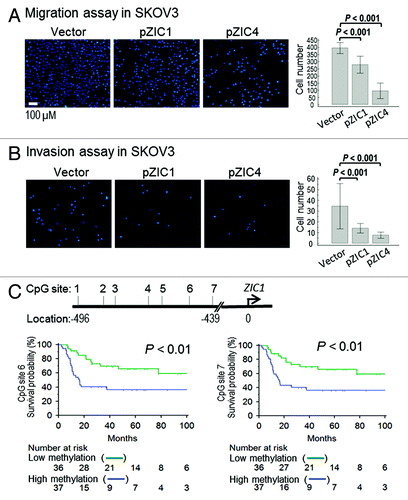
ZIC1 methylation is an independent PFS prognostic factor in ovarian cancer
The association between ZIC1 methylation and PFS was investigated using bisulfite pyrosequencing with optimal cutoff values determined by AUC. CpG sites 6 and 7 were the most accurate for classifying malignant ovarian samples (68.8% and 67.4% accuracy, respectively; Table S3). Univariate and multivariate Cox regression analysis were then conducted to determine whether these two CpG sites could serve as independent prognostic indicators of PFS. In the univariate analysis, hypermethylated ZIC1 was associated with short PFS (hazard ratio [HR] = 5.35, 95% confident interval [CI] = 1.31 to 5.35, p = 0.007), while the multivariate survival analysis showed both hypermethylated ZIC1 and advanced stage were independent risk factors for poor PFS (CpG site 6, HR = 2.37, 95% CI = 1.15 to 4.87, p = 0.019; CpG site 7, HR = 2.45, 95% CI = 1.17 to 5.13, p = 0.017; ; Table S4). In addition, a correlation (p < 0.05) between hypermethylated ZIC1 and early disease recurrence was observed (Table S5). Kaplan-Meier survival analysis showed that lower PFS probability correlated (p < 0.01 for CpG sites 6 and 7) with high ZIC1 methylation ().
Table 1. Univariate and multivariate Cox survival analysis for the association between the risk of high methylation of ZIC1 at CpG site 6, clinical variables and progression free survival
Discussion
In the current study, we used MethylCap-seq to carry out an unbiased, genome-wide DNA methylation analysis of 101 ovarian tissues. We identified 577 differentially methylated regions or DMRs that distinguished, with 87% accuracy, malignant from non-malignant (benign and normal) samples. We further identified the most significant DMRs (n = 57) that could, with 89% accuracy, classify the malignant samples, representing potential OC DNA methylation biomarkers. Although we and others have conducted genome-wide methylation studies on advanced stage samples to select methylation biomarkers,Citation25,Citation26 many of the resulting candidate genes have neither been validated using quantifiable methods nor verified in an independent patient cohort.Citation27 The present study is the first to perform next generation sequencing on ovarian tumors and validate the methylome findings in patient samples.
Based on 577 DMRs, we further conducted survival analysis to select outcome-associated markers. The association of high frequency HM-DMRs with poor outcome supports the notion of a CGI methylator phenotype (CIMP) in OC. CIMP has been reported to describe a subset of colorectal tumors with concordant, cancer specific methylation of a group of genes.Citation28 This form of epigenetic instability has also been reported in OC and other human neoplasms,Citation29-Citation34 but the mechanism is unclear. Aberrant methylation patterns have been associated with increased or altered activity of DNA methyltransferases (DNMT1, DNMT3a or DNMT3b). DNMT3b overexpression has been reported in OC cells, and overexpression of DNMT3a was reported in sporadic OC,Citation35,Citation36 although the relationship between gene hypermethylation and altered DNMT levels in OC cells is not straightforward.Citation37 A “poor prognosis-CIMP” has also reported in human neoplasms,Citation38-Citation42 and the current study shows a relationship between a high frequency HM-DMRs and poor PFS across all stages of OC (), and a trend for high frequency HM-DMRs and poor PFS of early stage disease (Fig. S4). Thus, the 53 DMRs identified in this study could serve as potential markers for evaluating OC outcome, but this would be need to be examined prospectively.
The zinc-finger domains in ZIC proteins bind with GLI proteins at Gli-binding sequence (GBS) to regulate gene transcription.Citation43 ZICs have been shown to be primarily involved in embryonic development,Citation44 but a recent paper reported a role for ZIC2 in OC.Citation45 In addition, GLI proteins have been shown to regulate several critical functions during embryonic development and activate downstream targets of the Hh signaling pathway in tumorigenesis, and Gli1 and Gli2 are the prime transcriptional effectors of Hh signaling in cancer.Citation46,Citation47 In OC, expression of Gli1/Gli2 has been reported, indicating an activated Hh pathway.Citation18 Gli2 inhibition resensitized drug resistant OC cells to taxanes,Citation17 and Gli1 was recently shown to play a role in OC cisplatin sensitivity.Citation48 Our results support a role for the GLI family in drug resistance, through hypermethylation-mediated silencing of ZIC1, a putative tumor suppressor.Citation21 SKOV3 and Cp70 were more responsive to cyclopamine-induced decreases in Gli2 expression compared with the less drug resistant A2780 and TOV112D cell lines (Fig. S6). Similarly, ZIC1 overexpression suppressed Gli2 in SKOV3 and Cp70 cells (). We suggest that high expression of Gli2 combined with low ZIC1 expression may contribute to drug resistance in OC.
Our comprehensive methylome analysis has identified functional DNA methylation biomarkers predictive of OC clinical outcome. Our findings underscore the importance of DNA methylation in OC, support the notion that therapies targeting specific pathways altered by this epigenetic mark may hold promise in OC, and as a whole the methylome data may represent a resource for continued development of epigenetic-based therapies for this devastating disease. We have shown that ZIC1 and ZIC4, related to the hedgehog pathway, a key signaling pathway in OC, are DNA methylation prognostic biomarkers and that loss of negative Hh signaling regulation may contribute to disease progression.
Materials and Methods
Samples
Samples from 86 malignant (75 cases for genome-wide methylation analysis), 20 benign and 6 normal epithelial ovarian tissues were collected during surgery, frozen immediately in liquid nitrogen and stored at -80°C or in liquid nitrogen until analysis. An additional 14 normal paraffin tissues were used to confirm methylation levels. Specimens were obtained from patients between 1991 and 2010 and acquired from Tri-Service General Hospital (Taipei, Taiwan). This study was conducted in accordance with the Helsinki human subjects’ doctrine and was approved by the Institutional Review Boards of the Tri-Service General Hospital. Informed written consent was obtained from each patient. The inclusion and exclusion criteria have been previously described.Citation49 The histological diagnosis of epithelial OC included Stage I, II, III and IV according to the International Federation of Gynecology and Obstetrics (FIGO). Normal epithelial ovarian tissue was collected by scraping the ovarian epithelium from patients diagnosed with uterine leiomyoma. All clinical information was recorded and reviewed from the patient hospital records. Patient demographics for genome-wide methylation analysis are listed in Table S1.
DNA isolation and MethylCap-seq assay
Genomic DNA was extracted from ovarian tissue or cell lines using the QIAmp DNA Mini Kit (QIAGEN GmbH). Methylated DNA was enriched from 2 µg genomic DNA using the MethylMiner Methylated DNA Enrichment Kit (Invitrogen) following the manufacturer’s instructions. Briefly, genomic DNA was sonicated to ~200-bp, captured by MBD proteins and precipitated using 1M salt buffer. Enriched methylated DNA was used to generate libraries for sequencing following the standard protocols from Illumina. MethylCap-seq libraries were sequenced using the Illumina Genome Analyzer IIx System. Image analysis and base calling were performed using the standard Illumina pipeline. Unique reads (up to 36-bp reads) were mapped to the human reference genome (hg18) using the ELAND algorithm, with up to two base-pair mismatches (see Table S6).
DNA methylation data production from next-generation sequencing
The uniquely mapped reads were used for additional linear normalization and differential methylation analysis.Citation50 The methylation level was calculated by accumulating the number of reads.(Equation 1)NRead,i is the number of normalized reads at the ith bin; URead,i is the number of uniquely mapped reads at the ith bin, and NU is the number of total uniquely mapped reads. “INT” rounds the element to the nearest integers toward minus infinity, “^” means the power operator. A region of methylation level is represented by the average of the normalized of uniquely reads. Comparison of group A and B (G = A or B), the average methylation level (AVGR,G) was calculated separately at two groups in a given region R (which includes m bin size, and start at the sth bin). The number of sample is SA for group A, and SB for group B.
(Equation 2) AVGR,G means the average methylation level of group G at the R region. MR,G is the methylation levels of each sample of group G at the R region. Then, we identified statistical significance of different methylation at the R region using two-tailed and paired t-test (p ≤ 0.001). Differentially methylated loci were analyzed between malignant and normal ovarian tissues.
Cell and culture conditions
Ovarian cancer cells (A2780, Cp70, SKOV3 and TOV112D) and immortalized normal ovarian cells (IOSE) were cultured at 37°C in an atmosphere of 5% (v/v) CO2 in air. The culture conditions for A2780 and CP70 cells have been described in detail.Citation49,Citation51,Citation52 SKOV3 were cultured in RPMI 1640 with 10% (w/v) fetal bovine serum, 100 U/ml penicillin, 0.1 mg/ml streptomycin, 1% MEM NEAA, 1mM MEM sodium pyruvate solution and 10mM HEPES. TOV112D, IOSE were cultured in 1:1 (v/v) ratio of MCDB-105/Medium-199 supplemented with 15% (w/v) fetal bovine serum, 100 U/ml penicillin, 100 μg/ml streptomycin. Epidermal growth factor (EGF) (5 μl, 1 mg/ml) and hydrocortisone (200 μl, 1 mg/ml) in 500 L were added to the culture medium for IOSE cells. Growth media used in cell culture were purchased from GIBCO (Invitrogen Corporation).
RNA extraction and QRT-PCR analysis of ovarian cell lines
Total RNA was isolated from cultured cells with the PureLink RNA Mini Kit (Ambion, Life Technologies) and reverse transcribed by using SuperScript® III Reverse Transcriptase (Invitrogen). Real-time PCR (qRT-PCR) was performed using the 7900HT Fast Real-Time PCR Systems (Applied Biosystems). The ΔCt and ΔΔCt was calculated using the housekeeping gene GAPDH and experimental condition controls as a reference, respectively. PCR reactions were performed in triplicate (see Table S7 for PCR primer sequences). Epigenetic regulation of gene expression was examined by using ovarian cell lines treated with the DNA demethylating agent 5-aza-2’-deoxycytidine (5-aza-dc, 5 μM or 10 μM; Sigma-Aldrich) alone or in combination with Trichostatin A (TSA, 0.3 μM; Sigma-Aldrich), a histone deacetylase inhibitor (HDACI). The transcriptional correlation between ZICs and GLIs examined by treating cells with the Hedgehog signaling inhibitor cyclopamine (8 μM; LC Laboratories) alone or in combination with 5-aza-dc (5 μM) for 3 d.
Cell transfection and viability assay
Ovarian cells (1.5 × 106) were transfected with pCMV6-ZIC1, pCMV6-ZIC4 and pCMV6 vector (OriGene) using Amaxa™ Cell Line Nucleofector™ Kit (Lonza), cultured for 24 h in a 6-well plate, trypsinized, counted and used in the assays described below. Exogenous ZIC1 or ZIC4 was confirmed by qRT-PCR. The MTS assay was used to determine cell viability, as per the manufacturer’s directions (Promega). Briefly, 2,000 cells were seeded in 96-well cell-culture plates in triplicate per experimental condition. After 0, 24, 48, 72 h incubation, CellTiter 96 Aqueous One Solution reagent (Promega) was added for 90 min and absorbance was measured at 490 nm.
Migration and invasion assay
For cell migration and invasion assay, 2 × 104 cells were seeded in BD BioCoat™ Control and Growth Factor Reduced BD Matrigel™ Invasion Chamber containing a 8.0 µm PET membrane insert (BD Bioscience). To determine the number of cells migrating through the pores or invading through the matrigel, after 22 h, inserts were washed, fixed using paraformaldehyde, and then incubated with 1 µg/mL Hoechst 333o42 (Life Technologies) for 15 min at 37°C. The stained cells were counted; 6–8 random images ( × 100) per well were obtained.
Survival analysis
Progression free survival (PFS) was defined as the interval from primary surgery or first line chemotherapy to recurrence. Sixty-six patients were included in the PFS analysis (nine patients were eliminated due to persistent disease). Persistent disease was based on increased serum CA125 titers (or plateaued > 35 U/mL) after chemotherapy. Recurrent disease (before follow-up) was observed in 32 (48%) patients and considered censored data for PFS. For methylation levels at each locus, we identified the 60th percentile as a cutoff value to dichotomize high and low methylation for each DMR. The PFS analysis used Kaplan-Meier survival curve and COX regression models. Univariate and multivariate Cox regression analysis were used to calculate hazard ratios (HR) and 95% confidence intervals (CI) for evaluating risks of disease progression associated with DNA methylation level. The multivariate Cox proportional hazards model was performed to determine the independent prognostic value of age, DNA methylation status, stage, grade, and histological subtype.
Statistical analysis
To evaluate the accuracy of the two groups (malignant and non-malignant samples), we calculated the optimal cutoff value using the most significant area under the receiver operating characteristic (ROC) curve (AUC). The relationship between gene expression and methylation was assessed by Pearson correlation coefficient. P-values less than 0.05 were considered statistically significant (assigned *, p < 0.05; **, p < 0.01; ***, p < 0.001). Two-tailed t-test was used to compare continuous variables in different treatment and control groups. Methylation levels in malignant and non-malignant samples were determined using bisulfite pyrosequencing and compared by two-tailed Mann-Whitney U test. All analyses were performed using the statistical package in R (R version 2.14.0) or MedCalc (version 9.0).
Additional material
Download Zip (790.4 KB)Acknowledgments
This work was supported by funds from the National Cancer Institute Awards U54 CA113001, The Integrative Cancer Biology Program, Centers for Cancer Systems Biology and CA85289, the Ovarian Cancer Research Fund (PPDIU01.2011), and Department of Health (DOH101-TD-PB-111-NSC008, National Research Program for Biopharmaceuticals to Hung-Cheng Lai).
Disclosure of Potential Conflicts of Interest
No potential conflicts of interest were disclosed.
Reference
- Bukowski RM, Ozols RF, Markman M. The management of recurrent ovarian cancer. Semin Oncol 2007; 34:Suppl 2 S1 - 15; http://dx.doi.org/10.1053/j.seminoncol.2007.03.012; PMID: 17512352
- Liu CM. Cancer of the ovary. N Engl J Med 2005; 352:1268 - 9, author reply 1268-9; http://dx.doi.org/10.1056/NEJM200503243521222; PMID: 15791705
- Sandercock J, Parmar MK, Torri V, Qian W. First-line treatment for advanced ovarian cancer: paclitaxel, platinum and the evidence. Br J Cancer 2002; 87:815 - 24; http://dx.doi.org/10.1038/sj.bjc.6600567; PMID: 12373593
- Watts GS, Futscher BW, Holtan N, Degeest K, Domann FE, Rose SL. DNA methylation changes in ovarian cancer are cumulative with disease progression and identify tumor stage. BMC Med Genomics 2008; 1:47; http://dx.doi.org/10.1186/1755-8794-1-47; PMID: 18826610
- Wei SH, Chen CM, Strathdee G, Harnsomburana J, Shyu CR, Rahmatpanah F, et al. Methylation microarray analysis of late-stage ovarian carcinomas distinguishes progression-free survival in patients and identifies candidate epigenetic markers. Clin Cancer Res 2002; 8:2246 - 52; PMID: 12114427
- Barton CA, Hacker NF, Clark SJ, O’Brien PM. DNA methylation changes in ovarian cancer: implications for early diagnosis, prognosis and treatment. Gynecol Oncol 2008; 109:129 - 39; http://dx.doi.org/10.1016/j.ygyno.2007.12.017; PMID: 18234305
- Balch C, Fang F, Matei DE, Huang TH, Nephew KP. Minireview: epigenetic changes in ovarian cancer. Endocrinology 2009; 150:4003 - 11; http://dx.doi.org/10.1210/en.2009-0404; PMID: 19574400
- Ibanez de Caceres I, Battagli C, Esteller M, Herman JG, Dulaimi E, Edelson MI, et al. Tumor cell-specific BRCA1 and RASSF1A hypermethylation in serum, plasma, and peritoneal fluid from ovarian cancer patients. Cancer Res 2004; 64:6476 - 81; http://dx.doi.org/10.1158/0008-5472.CAN-04-1529; PMID: 15374957
- Fiegl H, Windbichler G, Mueller-Holzner E, Goebel G, Lechner M, Jacobs IJ, et al. HOXA11 DNA methylation--a novel prognostic biomarker in ovarian cancer. Int J Cancer 2008; 123:725 - 9; http://dx.doi.org/10.1002/ijc.23563; PMID: 18478570
- Shridhar V, Bible KC, Staub J, Avula R, Lee YK, Kalli K, et al. Loss of expression of a new member of the DNAJ protein family confers resistance to chemotherapeutic agents used in the treatment of ovarian cancer. Cancer Res 2001; 61:4258 - 65; PMID: 11358853
- Akahira J, Sugihashi Y, Suzuki T, Ito K, Niikura H, Moriya T, et al. Decreased expression of 14-3-3 sigma is associated with advanced disease in human epithelial ovarian cancer: its correlation with aberrant DNA methylation. Clin Cancer Res 2004; 10:2687 - 93; http://dx.doi.org/10.1158/1078-0432.CCR-03-0510; PMID: 15102672
- Wei SH, Balch C, Paik HH, Kim YS, Baldwin RL, Liyanarachchi S, et al. Prognostic DNA methylation biomarkers in ovarian cancer. Clin Cancer Res 2006; 12:2788 - 94; http://dx.doi.org/10.1158/1078-0432.CCR-05-1551; PMID: 16675572
- Chan MW, Wei SH, Wen P, Wang Z, Matei DE, Liu JC, et al. Hypermethylation of 18S and 28S ribosomal DNAs predicts progression-free survival in patients with ovarian cancer. Clin Cancer Res 2005; 11:7376 - 83; http://dx.doi.org/10.1158/1078-0432.CCR-05-1100; PMID: 16243810
- Teodoridis JM, Hall J, Marsh S, Kannall HD, Smyth C, Curto J, et al. CpG island methylation of DNA damage response genes in advanced ovarian cancer. Cancer Res 2005; 65:8961 - 7; http://dx.doi.org/10.1158/0008-5472.CAN-05-1187; PMID: 16204069
- Makarla PB, Saboorian MH, Ashfaq R, Toyooka KO, Toyooka S, Minna JD, et al. Promoter hypermethylation profile of ovarian epithelial neoplasms. Clin Cancer Res 2005; 11:5365 - 9; http://dx.doi.org/10.1158/1078-0432.CCR-04-2455; PMID: 16061849
- Gloss BS, Patterson KI, Barton CA, Gonzalez M, Scurry JP, Hacker NF, et al. Integrative genome-wide expression and promoter DNA methylation profiling identifies a potential novel panel of ovarian cancer epigenetic biomarkers. Cancer Lett 2012; 318:76 - 85; http://dx.doi.org/10.1016/j.canlet.2011.12.003; PMID: 22155104
- Steg AD, Katre AA, Bevis KS, Ziebarth A, Dobbin ZC, Shah MM, et al. Smoothened antagonists reverse taxane resistance in ovarian cancer. Mol Cancer Ther 2012; 11:1587 - 97; http://dx.doi.org/10.1158/1535-7163.MCT-11-1058; PMID: 22553355
- Bhattacharya R, Kwon J, Ali B, Wang E, Patra S, Shridhar V, et al. Role of hedgehog signaling in ovarian cancer. Clin Cancer Res 2008; 14:7659 - 66; http://dx.doi.org/10.1158/1078-0432.CCR-08-1414; PMID: 19047091
- Bast RC Jr., Klug TL, St John E, Jenison E, Niloff JM, Lazarus H, et al. A radioimmunoassay using a monoclonal antibody to monitor the course of epithelial ovarian cancer. N Engl J Med 1983; 309:883 - 7; http://dx.doi.org/10.1056/NEJM198310133091503; PMID: 6310399
- Liao X, Siu MK, Au CW, Wong ES, Chan HY, Ip PP, et al. Aberrant activation of hedgehog signaling pathway in ovarian cancers: effect on prognosis, cell invasion and differentiation. Carcinogenesis 2009; 30:131 - 40; http://dx.doi.org/10.1093/carcin/bgn230; PMID: 19028702
- Gan L, Chen S, Zhong J, Wang X, Lam EK, Liu X, et al. ZIC1 is downregulated through promoter hypermethylation, and functions as a tumor suppressor gene in colorectal cancer. PLoS One 2011; 6:e16916; http://dx.doi.org/10.1371/journal.pone.0016916; PMID: 21347233
- Wang LJ, Jin HC, Wang X, Lam EK, Zhang JB, Liu X, et al. ZIC1 is downregulated through promoter hypermethylation in gastric cancer. Biochem Biophys Res Commun 2009; 379:959 - 63; http://dx.doi.org/10.1016/j.bbrc.2008.12.180; PMID: 19135984
- Network CGAR, Cancer Genome Atlas Research Network. Integrated genomic analyses of ovarian carcinoma. Nature 2011; 474:609 - 15; http://dx.doi.org/10.1038/nature10166; PMID: 21720365
- Mizugishi K, Aruga J, Nakata K, Mikoshiba K. Molecular properties of Zic proteins as transcriptional regulators and their relationship to GLI proteins. J Biol Chem 2001; 276:2180 - 8; http://dx.doi.org/10.1074/jbc.M004430200; PMID: 11053430
- Bauerschlag DO, Ammerpohl O, Bräutigam K, Schem C, Lin Q, Weigel MT, et al. Progression-free survival in ovarian cancer is reflected in epigenetic DNA methylation profiles. Oncology 2011; 80:12 - 20; http://dx.doi.org/10.1159/000327746; PMID: 21577013
- Dai W, Teodoridis JM, Zeller C, Graham J, Hersey J, Flanagan JM, et al. Systematic CpG islands methylation profiling of genes in the wnt pathway in epithelial ovarian cancer identifies biomarkers of progression-free survival. Clin Cancer Res 2011; 17:4052 - 62; http://dx.doi.org/10.1158/1078-0432.CCR-10-3021; PMID: 21459799
- Jones A, Lechner M, Fourkala EO, Kristeleit R, Widschwendter M. Emerging promise of epigenetics and DNA methylation for the diagnosis and management of women’s cancers. Epigenomics 2010; 2:9 - 38; http://dx.doi.org/10.2217/epi.09.47; PMID: 22122746
- Toyota M, Ahuja N, Ohe-Toyota M, Herman JG, Baylin SB, Issa JP. CpG island methylator phenotype in colorectal cancer. Proc Natl Acad Sci U S A 1999; 96:8681 - 6; http://dx.doi.org/10.1073/pnas.96.15.8681; PMID: 10411935
- Issa JP. Methylation and prognosis: of molecular clocks and hypermethylator phenotypes. Clin Cancer Res 2003; 9:2879 - 81; PMID: 12912930
- Strathdee G, Appleton K, Illand M, Millan DW, Sargent J, Paul J, et al. Primary ovarian carcinomas display multiple methylator phenotypes involving known tumor suppressor genes. Am J Pathol 2001; 158:1121 - 7; http://dx.doi.org/10.1016/S0002-9440(10)64059-X; PMID: 11238060
- Ueki T, Toyota M, Sohn T, Yeo CJ, Issa JP, Hruban RH, et al. Hypermethylation of multiple genes in pancreatic adenocarcinoma. Cancer Res 2000; 60:1835 - 9; PMID: 10766168
- Toyota M, Ahuja N, Suzuki H, Itoh F, Ohe-Toyota M, Imai K, et al. Aberrant methylation in gastric cancer associated with the CpG island methylator phenotype. Cancer Res 1999; 59:5438 - 42; PMID: 10554013
- Zhang QY, Yi DQ, Zhou L, Zhang DH, Zhou TM. Status and significance of CpG island methylator phenotype in endometrial cancer. Gynecol Obstet Invest 2011; 72:183 - 91; http://dx.doi.org/10.1159/000324496; PMID: 21968189
- Kolbe DL, DeLoia JA, Porter-Gill P, Strange M, Petrykowska HM, Guirguis A, et al. Differential analysis of ovarian and endometrial cancers identifies a methylator phenotype. PLoS One 2012; 7:e32941; http://dx.doi.org/10.1371/journal.pone.0032941; PMID: 22403726
- Ahluwalia A, Hurteau JA, Bigsby RM, Nephew KP. DNA methylation in ovarian cancer. II. Expression of DNA methyltransferases in ovarian cancer cell lines and normal ovarian epithelial cells. Gynecol Oncol 2001; 82:299 - 304; http://dx.doi.org/10.1006/gyno.2001.6284; PMID: 11531283
- Bai X, Song Z, Fu Y, Yu Z, Zhao L, Zhao H, et al. Clinicopathological significance and prognostic value of DNA methyltransferase 1, 3a, and 3b expressions in sporadic epithelial ovarian cancer. PLoS One 2012; 7:e40024; http://dx.doi.org/10.1371/journal.pone.0040024; PMID: 22768205
- Ehrlich M. DNA methylation in cancer: too much, but also too little. Oncogene 2002; 21:5400 - 13; http://dx.doi.org/10.1038/sj.onc.1205651; PMID: 12154403
- Abe M, Ohira M, Kaneda A, Yagi Y, Yamamoto S, Kitano Y, et al. CpG island methylator phenotype is a strong determinant of poor prognosis in neuroblastomas. Cancer Res 2005; 65:828 - 34; PMID: 15705880
- Shen L, Catalano PJ, Benson AB 3rd, O’Dwyer P, Hamilton SR, Issa JP. Association between DNA methylation and shortened survival in patients with advanced colorectal cancer treated with 5-fluorouracil based chemotherapy. Clin Cancer Res 2007; 13:6093 - 8; http://dx.doi.org/10.1158/1078-0432.CCR-07-1011; PMID: 17947473
- An C, Choi IS, Yao JC, Worah S, Xie K, Mansfield PF, et al. Prognostic significance of CpG island methylator phenotype and microsatellite instability in gastric carcinoma. Clin Cancer Res 2005; 11:656 - 63; PMID: 15701853
- Jo P, Jung K, Grade M, Conradi LC, Wolff HA, Kitz J, et al. CpG island methylator phenotype infers a poor disease-free survival in locally advanced rectal cancer. Surgery 2012; 151:564 - 70; http://dx.doi.org/10.1016/j.surg.2011.08.013; PMID: 22001634
- Ling Y, Huang G, Fan L, Wei L, Zhu J, Liu Y, et al. CpG island methylator phenotype of cell-cycle regulators associated with TNM stage and poor prognosis in patients with oesophageal squamous cell carcinoma. J Clin Pathol 2011; 64:246 - 51; http://dx.doi.org/10.1136/jcp.2010.082875; PMID: 21169275
- Koyabu Y, Nakata K, Mizugishi K, Aruga J, Mikoshiba K. Physical and functional interactions between Zic and Gli proteins. J Biol Chem 2001; 276:6889 - 92; http://dx.doi.org/10.1074/jbc.C000773200; PMID: 11238441
- Grinberg I, Millen KJ. The ZIC gene family in development and disease. Clin Genet 2005; 67:290 - 6; http://dx.doi.org/10.1111/j.1399-0004.2005.00418.x; PMID: 15733262
- Marchini S, Poynor E, Barakat RR, Clivio L, Cinquini M, Fruscio R, et al. The zinc finger gene ZIC2 has features of an oncogene and its overexpression correlates strongly with the clinical course of epithelial ovarian cancer. Clin Cancer Res 2012; 18:4313 - 24; http://dx.doi.org/10.1158/1078-0432.CCR-12-0037; PMID: 22733541
- Rubin LL, de Sauvage FJ. Targeting the Hedgehog pathway in cancer. Nat Rev Drug Discov 2006; 5:1026 - 33; http://dx.doi.org/10.1038/nrd2086; PMID: 17139287
- Ruiz i Altaba A, Mas C, Stecca B. The Gli code: an information nexus regulating cell fate, stemness and cancer. Trends Cell Biol 2007; 17:438 - 47; http://dx.doi.org/10.1016/j.tcb.2007.06.007; PMID: 17845852
- Kudo K, Gavin E, Das S, Amable L, Shevde LA, Reed E. Inhibition of Gli1 results in altered c-Jun activation, inhibition of cisplatin-induced upregulation of ERCC1, XPD and XRCC1, and inhibition of platinum-DNA adduct repair. Oncogene 2012; 31:4718 - 24; http://dx.doi.org/10.1038/onc.2011.610; PMID: 22266871
- Su HY, Lai HC, Lin YW, Liu CY, Chen CK, Chou YC, et al. Epigenetic silencing of SFRP5 is related to malignant phenotype and chemoresistance of ovarian cancer through Wnt signaling pathway. Int J Cancer 2010; 127:555 - 67; http://dx.doi.org/10.1002/ijc.25083; PMID: 19957335
- Huang TT, Gonzales CB, Gu F, Hsu YT, Jadhav RR, Wang CM, et al. Epigenetic deregulation of the anaplastic lymphoma kinase gene modulates mesenchymal characteristics of oral squamous cell carcinomas. Carcinogenesis 2013; http://dx.doi.org/10.1093/carcin/bgt112; PMID: 23568951
- Li M, Balch C, Montgomery JS, Jeong M, Chung JH, Yan P, et al. Integrated analysis of DNA methylation and gene expression reveals specific signaling pathways associated with platinum resistance in ovarian cancer. BMC Med Genomics 2009; 2:34; http://dx.doi.org/10.1186/1755-8794-2-34; PMID: 19505326
- Balch C, Yan P, Craft T, Young S, Skalnik DG, Huang TH, et al. Antimitogenic and chemosensitizing effects of the methylation inhibitor zebularine in ovarian cancer. Mol Cancer Ther 2005; 4:1505 - 14; http://dx.doi.org/10.1158/1535-7163.MCT-05-0216; PMID: 16227399