Abstract
Histone deacetylases (HDACs) have emerged as important targets for cancer treatment. HDAC-inhibitors (HDACis) are well tolerated in patients and have been approved for the treatment of patients with cutaneous T-cell lymphoma (CTCL). To improve the clinical benefit of HDACis in solid tumors, combination strategies with HDACis could be employed. In this study, we applied Analysis of Functional Annotation (AFA) to provide a comprehensive list of genes and pathways affected upon HDACi-treatment in prostate cancer cells. This approach provides an unbiased and objective approach to high throughput data mining. By performing AFA on gene expression data from prostate cancer cell lines DU-145 (an HDACi-sensitive cell line) and PC3 (a relatively HDACi-resistant cell line) treated with HDACis valproic acid or vorinostat, we identified biological processes that are affected by HDACis and are therefore potential treatment targets for combination therapy. Our analysis revealed that HDAC-inhibition resulted among others in upregulation of major histocompatibility complex (MHC) genes and deregulation of the mitotic spindle checkpoint by downregulation of genes involved in mitosis. These findings were confirmed by AFA on publicly available data sets from HDACi-treated prostate cancer cells. In total, we analyzed 375 microarrays with HDACi treated and non-treated (control) prostate cancer cells. All results from this extensive analysis are provided as an online research source (available at the journal’s website and at http://luigimarchionni.org/HDACIs.html). By publishing this data, we aim to enhance our understanding of the cellular changes after HDAC-inhibition, and to identify novel potential combination strategies with HDACis for the treatment of prostate cancer patients.
Introduction
An important mechanism of cells to epigenetically regulate gene expression is by acetylating and deacetylating histones.Citation1 Histone deacetylases (HDACs) are a class of enzymes that deacetylate lysine residues in the N-terminal tails of histones, thereby blocking gene transcription.Citation1 HDACs are frequently overexpressed in cancer; their overexpression leads among others to epigenetic silencing of tumor suppressor genes.Citation1 Therefore, various HDAC-inhibitors (HDACis) have been developed for cancer therapy, of which vorinostat (SAHA) and Romidepsin are approved by the United States Food and Drug Administration (US FDA) for the treatment of cutaneous T-cell lymphomas (CTCL). HDACis arrest cells in G0/G1 or G2/M phase dependent on the dose of HDACi and/or cell type used.Citation2 Despite pre-clinical data showing great promise and their success in liquid tumors, the potential of HDACis as single agents against solid tumors, specifically prostate cancer (PCa), seems to be limited in clinical studies.Citation2
It seems that improving DNA accessibility with HDACis is merely the first step in cancer treatment. Recent studies have therefore focused on combination strategies involving HDACis, with success. Valproic acid (VPA) in combination with epirubicin/FEC (5-fluorouracil, epirubicin, cyclophosphamide) resulted in an objective response in 64% of patients with solid advanced malignancies.Citation3 Combination therapy with the HDACi magnesium valproate and DNA demethylating agent hydralazine resensitized 80% of cancer patients to chemotherapy on which they had previously progressed.Citation4 This combination was successfully added to doxorubicin and cyclophosphamide therapy in breast cancer patients as well.Citation5 The addition of vorinostat to the mammalian target of rapamycin (mTOR) inhibitor temsirolimus improved anti-cancer activity against renal cell carcinoma in vitro and in vivo.Citation6 Other recent preclinical studies indicated that HDACis such as VPA may sensitize cancer cells, among others PCa cells, to radiotherapy.Citation7,Citation8 In non-small cell lung cancer studies it was found that cells may be sensitized for radiotherapy through acetyl p53-mediated downregulation of c-myc.Citation9 The rationale for such combination studies with HDACis was that HDACis may reverse epigenetic changes made by the tumor, downregulate gene expression involved in DNA damage repair and/or upregulate apoptosis in cancer cells.
In this study, we apply analysis of functional annotation (AFA) to HDACi-treated PCa cells, thereby providing a rationale for novel combination strategies with HDACis. AFA is a high-throughput bioinformatics approach to identify sets of genes that are differentially expressed between conditions, such as cancer cells pre- and post-treatment. It is conceptually similar to gene set enrichment analysis (GSEA).Citation10-Citation14 This unbiased method enables the interpretation of large amounts of gene expression data generated by microarray analysis through superimposition, selection, analysis and visualization of information encompassing distinct biological concepts, such as cellular signaling pathways, protein-protein interaction (PPI) networks, gene ontology (GO), gene expression regulation by transcription factors and microRNA targets. In our study AFA was used to detect cellular processes that are affected by HDACis in PCa cell lines. We analyzed data from an elaborate microarray experiment with an HDACi-sensitive (DU-145) and a (relatively) HDACi-insensitive PCa cell line (PC3) treated with the HDACis VPA and vorinostat (SAHA).Citation15 We further complemented this analysis with gene expression profiles from HDACi-treated LNCaP and PC3 cells using all available data from the public domain.Citation16,Citation17 Overall we analyzed 375 distinct microarray experiments involving HDACi-treated PCa cells, which to our best knowledge is the largest analysis of this kind to date. By creating an encyclopedia of expression changes induced by HDACis in PCa cell lines, we have implemented a resource publicly available to the research community for use in future research, both for improving our understanding of the cellular effects of HDACis on PCa cells, and for developing hypotheses to test drug combinations with HDACis in preclinical studies. Finally, based on the AFA results, we present a rationale for the combination of HDACis with mitotic spindle checkpoint inhibitors and immunotherapy. Further (pre-)clinical evaluation is necessary to validate these rationales.
Results
Analysis of functional annotation (AFA) after treatment with HDACis
We previously used a “multiple-loop, double-cube” design to identify genes differentially expressed in PCa cell lines upon HDAC-inhibition by VPA or SAHA.Citation15 In the current study, we applied AFA, as previously described, to enable the interpretation of these results in the context of relevant cancer biology.Citation10,Citation11,Citation18-Citation20 To this end, we selected Functional Gene Sets (FGS) from distinct databases, recapitulating different and complementary biological concepts: (1) cellular signaling pathways from Pathway Commons, (2) PPI networks from the National Center for Biotechnology Information (NCBI) Entrez Gene database, (3) downstream transcriptional responses, and (4) gene expression regulatory networks orchestrated by transcription factors and microRNA targets. These collections included, among others, the Human Protein Reference Database (HPRD), GO, Kyoto Encyclopedia of Genes and Genomes (KEGG), the Molecular Signature Database (MSigDB), the Pathway Commons and NCBI Entrez Gene databases.Citation14,Citation21-Citation30 FGS whose expression altered most significantly upon HDAC-inhibition in DU-145 and PC3 cells across all conditions after correction for multiple testing (adjusted p-value < 0.05, top five FGS or more in case of ties), were retrieved and are displayed in . This approach enabled the identification of biological themes that are differentially expressed upon HDAC-inhibition across all conditions irrespective of the gene expression direction change (), as well as biological processes that are selectively up- or downregulated across all conditions (, respectively). The complete lists with all AFA results, including FGS that were differentially expressed between cell lines, treatments and/or time-points, with all relevant statistics (e.g., fold-change, p-values, false discovery rates (FDR), log-odds), are provided as supplementary data available online at the journal’s website and at http://luigimarchionni.org/HDACIs.html.
Figure 1. Heat maps visualizing differentially expressed functional gene sets (FGS) as determined by analysis of functional annotation (AFA) performed on DU-145 and PC3 cells treated with HDAC-inhibitors (HDACis) VPA or vorinostat for 48 or 96 h. Each row represents a distinct FGS, while each column represents a distinct coefficient from our previous linear model analysis. The FGS that were most significantly differentially expressed across all comparisons performed are shown in the figure (top 5 FGS showing an adjusted p-value ≤ 5%, or more in case of ties). Color scales correspond to the absolute adjusted p-values obtained from our analysis after base 10 logarithmic transformations (i.e., the number on the color scale increases with decreasing adjusted p-values). Differentially expressed FGS were selected from different collections in order to encompass distinct biological concepts, as shown by the color bar on the left of each heat map. Cell signaling FGS are highlighted in red and yellow (Pathway Commons Reactome and NCI pathways, respectively), signaling pathway target gene sets in green (Human Protein Reference Database, HPRD), protein-protein-interaction networks in cyan (PPI, as compiled in the NCBI Entrez Gene database), FGS for shared transcriptional factor binding sites (TFBS) in blue, and microRNA (MIR) targets gene sets in pink (both from the Broad Institute Molecular Signature Database collections). For complete tables and heat maps visualizing all differentially expressed FGS, see supplementary data (available at the journal’s website and at http://luigimarchionni.org/HDACIs.html). (A) Differentially expressed FGS after ordering the genes based on absolute moderated t-statistics, thus irrespective of the direction of gene expression modulation upon HDAC-inhibition. (B) FGS differentially expressed due to upregulated genes, as determined by signed t-statistics. (C) FGS differentially expressed due to downregulated genes, as determined by signed t-statistics.
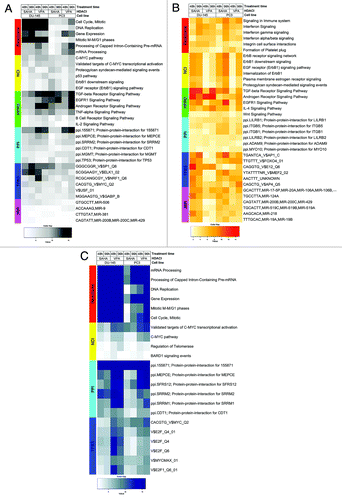
We further explored the AFA results by analyzing the relationship among identified FGS in terms of gene overlap, identifying common and distinct sets of genes driving the enrichment. To this end we assembled “gene to FGS” membership matrices for enriched FGS presented in , and performed hierarchical clustering and social network analysis to group them based on gene overlap (for details see Figs. S1-S6 and the supplementary methods section).Citation31 This revealed both FGS groups whose enrichment was driven by similar, if not identical, genes, as well as FGS groups with minimal gene overlap, whose enrichment was hence due to separate and distinctive genes. Even more interesting, such divergent FGS groups pointed to complementary, yet distinct, biological concepts. For instance, a number of different FGS corresponding to specific signaling pathways and to their downstream targets were enriched, with minimal overlap of the underlying genes, providing a stronger rationale for pathway modulation upon HDACi-treatment. An example of such findings is the epidermal growth factor signaling, for which the FGS corresponding to both the pathway machinery (e.g., “ErbB1 receptor signaling network” from National Cancer Institute (NCI)-Pathway Commons) and the downstream targets (e.g., “EGFR Signaling Pathway” targets from HPRD) proved to be enriched (; Figs. S2 and S5).
Overall, treatment with HDACis altered gene expression programs related to biological processes that are well known to be involved in tumor development and progression, such as cell adhesion and cell cycle related FGS. In addition, well-established potential drug targets were modulated, such as the c-myc, the androgen receptor signaling, and the Epidermal Growth Factor Receptor 1 (EGFR1) signaling pathways. Furthermore, the interpretation of the AFA results attracted attention to two groups of functionally connected FGS that were up- or downregulated after HDAC-inhibition: major histocompatibility complexes (MHC) genes and mitotic spindle checkpoint genes, respectively (). These FGS will be further discussed below.
Treatment of PCa cells with HDACis results in upregulation of major histocompatibility complex (MHC) genes
Interpretation of the AFA results indicated that HDACis may play a role in immuno-editing, as it transcriptionally modulated genes involved in this biological process. In fact, the “Signaling in Immune system” Reactome FGS proved to be most significantly upregulated across all studied conditions along with FGS related to IL-4 and Interferon signaling pathways (). Beta-2 microglobulin (B2M), Human Leukocyte Antigen (HLA) class I molecules, and Interferon induced proteins were among the most upregulated genes driving this upregulation (). Upregulation of FGS related to the immune system was observed in other collections as well: for example PPI networks with LILRB1 (Leukocyte immunoglobulin-like receptor B1) and LILRB2 were also upregulated upon HDAC-inhibition (). Furthermore, the cAMP Response Element Binding protein (CREB) and the interferon-stimulated response element (ISRE) site gene sets were differentially expressed upon HDACi-treatment (see supplementary data online available at the journal’s website and at http://luigimarchionni.org/HDACIs.html). Interestingly, CREB is part of an enhanceosome that increases transcription of MHC-I, B2M and MHC-II genes, and interferon is a major regulator of MHC-I transcription.Citation32,Citation33
Table 1. Differentially expressed genes from the “Signaling in Immune system” FGS
We validated our data by performing differential gene expression analysis and AFA on an additional 353 microarray experiments from three publicly available data sets (GSE8645 and GSE31620, and Connectivity Map) involving PCa cells treated with HDACis.Citation16,Citation17 We assessed the overall agreement of differential gene expression across all analyzed data sets using Correspondence At the Top (CAT) curves as described previously, which overall proved to be greater than expected by chance (Figs. S7-S16).Citation10,Citation18,Citation34 We also compared and validated AFA results identifying the biological themes and processes which were consistently differentially expressed across the data sets, treatment conditions, and data points analyzed (Figs. S17-S19). Overall, FGS related to the immune system were significantly upregulated across most of the analyzed conditions, spanning distinct HDACis, cell lines and treatment time points (Fig S18; the complete results in the Supplemental Material and at http://luigimarchionni.org/HDACIs.html).
Based on the finding that FGS related to the immune system are differentially expressed upon HDACi-treatment, we hypothesized that PCa cells may alter the expression of genes related to the immune system, a change that is reverted by HDACis. To further explore this hypothesis, we assessed whether the expression of MHC proteins in human PCa tissue is deregulated compared with normal prostate tissue. For this purpose, a PCa Tissue Microarray (TMA) was performed using specimens from 71 patients who had undergone a radical prostatectomy. Protein expression of HLA-A, HLA-B, HLA-DRA and B2M was scored in PCa tissue and normal surrounding tissue (). Repeated measurement analysis using the panel of all four proteins revealed a significant combined change in MHC protein expression in human PCa tissue compared with normal surrounding tissue (p < 0.001). B2M and HLA-B were primarily responsible for this deregulation. B2M protein expression was downregulated compared with normal surrounding tissue (p = 2.5·10−7), while HLA-B was upregulated (p = 0.009), as determined by Student’s t-tests (). There was no eminent change in HLA-A and HLA-DRA protein expression. As a final note, B2M expression of non-cancerous tissue tended to be decreased in patients with PSA recurrence (p = 0.06), possibly indicating that deregulation of B2M is correlated with PCa progression (data not shown).
Figure 2. IHC staining of human prostate tissue. A TMA performed with PCa tissue and normal surrounding tissue from 71 patients with primary PCa indicated deregulated MHC protein expression in human PCa tissue (p < 0.001). (A) Representative images of human PCa and normal prostate tissue stained for B2M (top) and HLA-B (bottom) via immunohistochemistry show decreased expression of B2M and increased expression of HLA-B in PCa tissue compared with normal surrounding tissue in a PCa patient. (B) B2M, HLA-A, HLA-B and HLA-DRA protein expression was scored by measuring the staining intensity of these proteins in immunohistochemistry samples from 71 PCa patients by two investigators independently (0, no staining; 1, light staining; 2, medium staining; 3, strong staining). The mean staining intensity of 71 cancerous and non-cancerous tissue samples is displayed in the bar graph. B2M and HLA-B protein expression was significantly different between PCa and normal surrounding tissue (p = 2.5·10−7 and p = 0.009, respectively), while there was no eminent change in HLA-A and -DRA protein expression (p > 0.05).
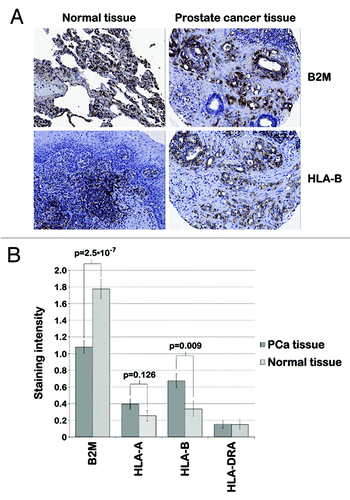
Treatment of PCa cells with HDACis results in downregulation of mitotic spindle checkpoint genes
Our AFA results indicated that HDACis changed the expression of genes relevant to mitosis as well (; Figs. S17 and S19). summarizes the genes contributing to altered expression of the “cell cycle, mitotic” Reactome FGS in DU-145 and PC3 cells treated with VPA or vorinostat. Treatment of DU-145 cells with 1 mM VPA or 1 μM vorinostat resulted in a transcriptional downregulation of genes coding for kinetochore and other mitotic proteins (adjusted p-value < 10−9). In contrast, the more resistant PC3 cells showed less downregulation (adjusted p-value < 10−9 only after 96h treatment with VPA) ( and ).
Table 2. Differentially expressed genes from the “Cell Cycle, Mitotic” FGS
Without proper kinetochore formation, chromosomes will not divide equally across the two daughter cells. To prevent aneuploidy, cells activate the spindle assembly checkpoint, which prevents progression through mitosis if metaphase is not completed properly.Citation35 We hypothesized that treatment with HDACis VPA or vorinostat could result in a functional spindle checkpoint defect by downregulation of kinetochore proteins and other proteins involved in this checkpoint. In PC3 cells we expected the spindle checkpoint to be intact, as there is less transcriptional downregulation of mitotic spindle checkpoint and kinetochore genes. To test this hypothesis, DU-145 and PC3 cells were synchronized in S-phase by a double thymidine block (), released and subsequently treated with colcemid—a spindle poison that depolymerizes microtubules and limits microtubule formation in mitosis. Cells with an intact spindle checkpoint will recognize a defect in microtubule attachment through kinetochore signaling and be arrested in mitosis. In the absence of a functioning kinetochore, the spindle checkpoint is not intact and cells progress through mitosis in the absence of proper chromosome segregation by microtubules. Both PC3 and DU-145 cells accumulated in G2/M-phase after 18h of treatment with colcemid; a small sub-G0 population was measured as well. Therefore, it can be assumed that these cells have an intact spindle assembly checkpoint (). Separate doses of colcemid were chosen for DU-145 and PC3 cells since the standard dose of 0.1µg/ml led to toxicity in DU-145 cells (). Next, we treated synchronized PC3 and DU-145 cells after release from S-phase with SAHA or simultaneously with HDACis and colcemid. During the 20 h after administration of 9 µM SAHA, most PC3 cells were initially in the G2/M phase, but later in G1-phase as well. Treatment of DU-145 cells with SAHA resulted in an increased G1- and (later) sub-G0 population. After combination treatment, PC3 cells mainly accumulated in G2/M-phase after ≥ 9 h of treatment, most likely these cells are mitotically arrested by activation of the spindle checkpoint (; Fig. S20, Supplemental Material and http://luigimarchionni.org/HDACIs.html). On the other hand, treatment of DU-145 cells for ≥ 9 h resulted in a time-dependent increase in a large sub-G0 population apart from a population in G2/M phase, the latter population getting smaller after 20 h of treatment (; Fig. S21 and http://luigimarchionni.org/HDACIs.html). This may indicate that part of the HDACi-sensitive cells (DU-145 cells) override the spindle checkpoint, leading to a G0/G1 arrest and eventually apoptosis, while cells that are relatively resistant to HDACis (PC3 cells) are arrested in mitosis by activation of the spindle assembly checkpoint.
Figure 3. Summary of the flow cytometry protocol. Cells were synchronized with a double thymidine block, which arrests cells at the beginning of the S-phase. For this purpose, cells were plated, and at 30–50% confluency incubated for 12 h in DMEM-10 media containing 2 mM thymidine. Subsequently cells were washed twice in PBS and incubated in DMEM-10. After 16 h, the media was replaced with DMEM-10/thymidine and cells were incubated for 12–14 h. Then cells were released from S-phase by removing the thymidine containing media. After three hours cells were treated with HDACis and/or colcemid, and for 17 h control and treated cells were harvested (†) every 2–3 h to monitor cell cycle progression by flow cytometry.
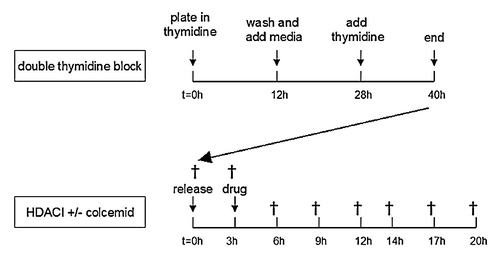
Figure 4. HDACis and spindle checkpoint activation in PCa cells. Simultaneous inhibition of PCa cells with HDACis and colcemid leads to accumulation of cells in mitosis in PC3 cells, but to a time-dependent increase of a sub-G0 population in DU-145 cells. (A) DU-145 and PC3 cells were treated for 18 h with colcemid at a final concentration of 0.1 µg/ml after being released from S-phase (M3). This resulted in mitotic accumulation of PC3 cells (M2), but DU-145 cells exhibited an increased sub-G0 population (M4), indicating cytotoxicity. DU-145 cells were successfully arrested in mitosis with low toxicity at a final concentration of 0.04 µg/ml colcemid. (B) PC3 cells were treated for variable periods with either 9 µM vorinostat alone or a combination of 0.1 µg/ml colcemid with 4 µM vorinostat or 4 mM VPA. Combining HDACis with colcemid resulted in mitotic accumulation of PC3 cells. (C) DU-145 cells were treated for variable periods with either 9 µM vorinostat alone or a combination of 0.04 µg/ml colcemid with 4 µM vorinostat or 4 mM VPA. Combining HDACis with colcemid resulted in a time-dependent increase of a sub-G0 population in DU-145 cells.
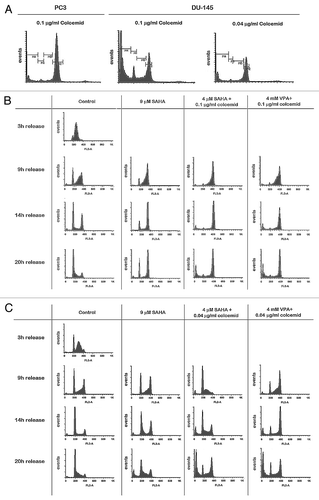
Discussion
In the past decade, a growing number of scientists have concluded that single agents, often targeting a single pathway, are insufficiently effective for cancer treatment, as many complex signaling pathways are involved in cancer development and progression. Therefore clinical trials have shifted focus to combination therapy to either target different pathways or target the same major pathway from different angles. However, many recent phase III studies have concluded that clinical benefit of these combination therapies is limited, while toxicity is increased.Citation36-Citation38 To speed up the implementation of combination therapies in clinic and to reduce costs of failing expensive clinical trials, there is a strong need to develop preclinical tools to identify combination strategies that will be successful in clinic.
In this study, we show that AFA allows unbiased hypothesis generation for combination therapies. This systems biology approach successfully unraveled the mechanisms of action of HDACis, without the bias of assumptions based on previous literature or the preference of the researcher. We show major FGS expression changes after HDACi-treatment, induced either directly through regulation of histones or indirectly by regulating (multiple) genes in pathways. A major advantage of AFA is that it is able to identify and visualize modest gene expression changes when occurring across a predefined category, without prior arbitrary cut-offs for fold change, p-value, and/or false discovery rates (FDR). This is particularly relevant since previous studies have indicated that fluctuations in such basal gene expression patterns at levels less than what is considered significant (i.e., FDR < 0.05) do have a functional relationship.Citation39 Furthermore, AFA combines biological information from different knowledge domains and different conditions and is therefore equal to GSEA done over multiple contrasts. FGS representing similar biological concepts proved to be in agreement with each other, supporting the strength of AFA. In summary, AFA enables us to identify specific biological and molecular processes affected by chemotherapeutic agents in greater detail, as subtle but consistent changes can be identified, providing a framework for further investigation.
We hence performed differential gene expression analysis and applied AFA to four distinct data sets, ultimately analyzing over 42,475 distinct FGS over a total of 375 different samples. This analysis, which we made publically available online, is the largest performed on PCa cells treated with HDACis. It therefore constitutes an invaluable resource for the identification of FGS that are targeted by HDACis. Finally, we have investigated two of the identified molecular concepts for which multiple FGS proved affected upon HDAC-inhibition more into detail, namely, MHC genes and mitotic spindle checkpoint genes.
The immune system plays a prominent role in the development, progression and treatment of cancers, among others PCa.Citation40 Recognition of cancer cells by the immune system leads to eradication of these cells, therefore cancer cells need to escape this immune response (immune escape). This is accomplished by both genetic mutations and epigenetic modifications resulting in the loss of MHC class I and II molecules in some cancer cells (immuno-editing); these cells then get selected for further proliferation.Citation40 Indeed, we show in this study that in human primary PCa tissue samples MHC-related gene expression is deregulated. This is in line with earlier reports: for instance Sharpe et al. concluded that expression of MHC class I and II determinants (such as B2M) was downregulated in tissue samples from PCa patients.Citation41 While B2M expression is downregulated, HLA-A expression does not differ significantly in our results. Tamura et al. concluded that expression of HLA-A is downregulated when PCa cells progress from hormone therapy sensitive to hormone resistant PCa.Citation42 Downregulation of HLA-A may not have occurred in our patients’ samples yet, as most cancers are in an early stage when a radical prostatectomy is performed.
Immunotherapy has become a common approach to treat cancer. It can be used to target specific pathways, such as the use of trastuzumab to target HER2 positive breast cancer cells,Citation43 or to modulate the immune system against cancer cells.The role of immunotherapy in PCa treatment is less well established. Although it is generally accepted that the immune system plays a role in PCa, the exact mechanism by which the immune system is involved in PCa initiation, progression and treatment remains unclear.Citation41,Citation44 Sipuleucel-T has been approved by the US FDA as a cellular immunotherapy that improves overall survival in asymptomatic or minimally symptomatic metastatic castrate-resistant PCa patients, and other immunotherapies such as ipilimumab are in advanced stages of clinical development.Citation45,Citation46 However, some have doubted its efficacy.Citation47,Citation48 Therefore, immunotherapy is not yet widely used in PCa treatment.
As VPA and vorinostat upregulate FGS involved in the immune system in DU-145 and PC3 cells, treatment by HDACis may prompt a natural or induced immune response against tumor cells by the immune system or immunotherapy, respectively. Studies in other cancer types have also found that HDACis enhance the expression of immunologically important molecules and induce immune responses, strengthening our findings.Citation49 As there currently is no tissue available from patients after treatment with HDACis, it is not possible to assess whether such immune responses occur in PCa patients. However, our results indicate that HDACis may revert the acquired B2M deficiency found in cancer cells resistant to immunotherapy.Citation50 Considering the increasing importance of immunotherapy in PCa, it is imperative that future studies test the hypothesis that pretreatment with HDACis may sensitize PCa cells for immunotherapy. The clinical response to sipuleucel-T, ipilimumab and/or other immunotherapies may be increased through reversal of (epi)genetic modifications that lead to immune escape of the cancer cell.
Results from our study further indicate that posttranslational changes may not be solely responsible for the regulatory effects of HDACis during mitosis, but translational downregulation of genes involved in mitotic regulation may also play a role in creating mitotic defects. Treatment with high doses of SAHA seems to result in progression through mitosis in the absence of a mitotic spindle in HDACi-sensitive cells. This may lead to aneuploidy, a G0/G1 phase arrest and eventually to cell death.Citation51 Similar results have been reported by Noh et al.,Citation52 in which treatment of HeLa cells with Trichostatin A resulted in a transcription-dependent defective mitosis with loss of checkpoint control. This effect may be increased when treating PCa cells with agents that deregulate the spindle assembly checkpoint. Combination therapy of HDACis with one such spindle checkpoint deregulator, aurora kinase inhibitors, has recently been tested in lymphoma cells in vitro and in vivo, with success.Citation53 Both results from this study and our results encourage testing combinations of HDACis with aurora kinase inhibitors or other agents that deregulate the spindle checkpoint, such as polo-like kinase 1 (Plk1-)inhibitors, in PCa and other solid tumors. Preliminary results of preclinical studies by our group involving combination therapies with HDACis (VPA/vorinostat) and an aurora kinase inhibitor (AMG 900) or Plk1-inhibitors (BI 2536/volasertib [BI 6727]) in PCa indicate that these combinations result in increased anti-tumor activity with acceptable toxicities.Citation54,Citation55
Our AFA results identified other FGS differentially expressed upon HDACi-treatment that may be of clinical importance too, such as upregulation of the androgen receptor signaling pathway. Increased sensitivity to androgen therapy after VPA treatment has been reported with other PCa cell lines.Citation56 Our data suggest that DU-145 and PC3 cells may have increased sensitivity to androgen therapy after HDACi-treatment as well, potentially by upregulation of androgen receptor expression.Citation8 Further studies need to be performed to assess increased androgen sensitivity after HDACi-treatment in PCa.
In summary, in this study we successfully applied AFA. This created an expansive and complete library of biological processes modulated by HDACis in PCa cell lines, and is therefore a valuable resource for researchers involved in research with HDACis. We further explored two pathways that were differentially expressed upon HDACi-treatment that may be of interest for future combination therapy. Novel studies combining HDACis with inhibitors of identified pathways are granted to assess clinical benefit for PCa patients. Furthermore, this study indicates that AFA can be used as an unbiased rational approach to identify novel combination strategies against cancer. Performing such analyses generates a comprehensive list of FGS differentially expressed upon cancer cell treatment.
Materials and Methods
Ethics statement
Anonymized human PCa specimens were obtained from the University Medical Center Utrecht (UMCU), after it was ensured that enough material remained to serve the patient’s and family’s needs, and in accordance to the Dutch code of conduct for the use of leftover body material.Citation57 All investigations involving human samples were performed in strict adherence to the Declaration of Helsinki.
Cell lines and chemicals
PCa cells were obtained from the American Type Culture Collection (ATCC) and maintained as described by our group previously.Citation15 Stock solutions of 10 mM vorinostat (AtonPharma/Merck) dissolved in DMSO were used for all experiments; vorinostat was further diluted in complete RPMI-1640 media (Invitrogen). VPA (Sigma-Aldrich) was stored as a salt, and freshly dissolved in complete RPMI-1640 media (RPMI-1640 media supplemented with 10% fetal bovine serum [FBS]) before each experiment. Colcemid (Sigma-Aldrich) was acquired as a 10 µg/ml solution in Hank’s balanced salt solution (HBSS) and stored at 4°C.
Microarray experiment
Microarray data were obtained from time course experiments in which PC3 and DU-145 cells were treated with VPA or vorinostat for 48 h, and are available from the National Center for Biotechnology Information (NCBI) Gene Expression Omnibus (GEO) database (series GSE34452). Expression data were processed using the R-Bioconductor library limma.Citation58-Citation63 Differential gene expression data were acquired using a generalized linear model approach (“multiple-loop, double-cube” design) as described by Kortenhorst et al.Citation15 A detailed explanation of all procedures and methods used for microarray data pre-processing, and differential gene expression analysis and detection has been described in detail previously.Citation15,Citation64 Briefly, in this previous study we applied a complex design based on a “multiple-loop, double-cube” cDNA microarray experiment to enhance our understanding of the molecular underpinnings of HDACi resistance in PCa cells. To this end, we analyzed gene expression data from a total of 22 dual-color microarray hybridizations comparing DU-145 and PC3 cells treated with vorinostat and VPA after an incubation of 48 and 96 h. Data were normalized using within-array “loess” normalization, and an ANOVA coupled with empirical Bayes standard errors shrinkage was used to identify differentially expressed genes between treated cells and controls. HDAC inhibition resulted in differential expression of 2.8% to 10% of genes (adjusted P-value < 0.001) across all conditions. Between 51% to 72% of such genes were upregulated, while 28% to 49% were downregulated. Larger differential gene expression was observed in DU-145 cells compared with PC3 cells, with VPA treatment compared with vorinostat, and with longer drug exposures.
Other public domain data were obtained from GEO and the Connectivity Map, publicly available from the NCBI GEO database, along with MIAME (minimum information about a microarray experiment) compliant information. Details regarding the source of gene expression data sets used, the platforms and annotation packages employed, the pre-processing procedures adopted for each data set, as well as the statistical details of the analysis performed, are described extensively in the Supplemental Material and at http://luigimarchionni.org/HDACIs.html.
Analysis of Functional Annotation (AFA)
We applied Analysis of Functional Annotation (AFA), a gene set analysis approach, on differential gene expression data obtained from distinct comparisons and across different studies to identify biological processes and signaling pathways modulated by HDACis in PCa cells.Citation10,Citation11,Citation18,Citation19,Citation65 Overall, this methodology extends gene set analysis procedures, such as gene set enrichment analysis (GSEA) or parametric analysis of gene set enrichment (PAGE), by investigating biological processes enrichment over multiple experimental conditions as briefly summarized below.Citation12-Citation14
FGS, recapitulating distinct and complementary biological concepts such as cellular signaling pathways, PPI networks, downstream transcriptional responses, gene expression regulatory networks orchestrated by transcription factors and microRNA targets, were retrieved in the form of gene lists from various publicly available databases (see Table S1 and http://luigimarchionni.org/HDACIs.html). These collections included the Reactome, the HPRD, GO, KEGG, the MSigDB and NCBI Entrez Gene databases.Citation14,Citation21-Citation29
A one-sided Wilcoxon rank sum test was separately applied across all investigated comparisons to test whether any given FGS was differentially expressed, upregulated or downregulated, using the absolute and signed t-statistics to order genes (for details on the linear model analysis see the Supplemental Material and Kortenhorst et al.Citation15). The enrichment analysis was performed on all non-redundant genes present on the microarray, according to the NCBI Entrez Gene database annotation.Citation66 Filtering of redundant microarray features (i.e., probes mapping to the same NCBI Entrez Gene identifier) was achieved by retaining only the probes with the largest absolute t-statistics for further analysis. Correction for multiple hypothesis testing was obtained separately for each FGS collection by applying the Benjamini and Hochberg method.Citation67 Differentially expressed FGS were visualized using heatmaps; an adjusted p-value < 0.05 was considered significant.
To validate the results from the AFA on our microarray data, we further performed differential gene expression analysis and AFA on publicly available data sets of HDACi-treated PCa cells. Three data sets were available (GSE8645, GSE31620, and Connectivity Map). In one data set (GSE8645), LNCaP cells had been treated with either 7.5 µM CG-1521 or 5 µM Trichostatin A (both HDACis) for 24 h, after which a microarray was performed.Citation68 In data set GSE31620, LNCaP cells were treated for 36 h with Trichostatin A and/or the DNA-methylating agent 5-Azacytidine after which microRNA microarrays were performed.Citation16 In the Connectivity Map PC3 cells had been treated with various HDACis at various dosages for 6 h.Citation17 A detailed description of these data sets is available in the Supplemental Material and http://luigimarchionni.org/HDACIs.html.
Flow cytometry
DU-145 and PC3 cells were synchronized in S-phase by a double thymidine block (). Cells were plated in 100 mm dishes; at 30–50% confluency, cells were incubated in DMEM-10/thymidine media (DMEM [Invitrogen] supplemented with 10% FBS and 2 mM thymidine [Sigma-Aldrich]) for 12h. Subsequently cells were washed twice in PBS and incubated in DMEM-10 media. After 16h, the media was replaced with DMEM-10/thymidine and cells were incubated for 12–14h. Cells were released from S-phase by removing the thymidine-containing media; three hours later DMEM-10 media containing an HDACi and/or colcemid was added. Cells were harvested immediately after the S-phase release, at the start of treatment, and 3, 6, 9, 11, 14 or 17h after treatment initiation.
Cells were prepared for flow cytometry analysis using the method of Vindelov.Citation69 Flow cytometry was performed on a BD-LSR II flow cytometer (BD Biosciences); data were interpreted using CellQuest (BD Biosciences).
Tissue microarrays (TMAs) and immunohistochemistry (IHC)
For TMAs, human PCa samples were obtained from archives of the Pathology Department of the UMCU. Samples from 71 patients who had undergone radical prostatectomy were collected; information concerning survival and PSA recurrence of these patients was collected. Tumors had been formalin-fixed and paraffin-embedded after resection.
From paraffin blocks four 1 mm cores were taken per specimen. These cores were transferred to a recipient composite paraffin block using a Beecher MT1 manual arrayer (Beecher Instruments). Four micron slides were prepared from each TMA. Spots that contained necrotic/dead tissue or fibrin instead of cancer cells were excluded.
IHC was performed with 4 µm paraffin sections on the BOND-MAX (Leica) using the Bond Polymer Refine Detection™ (Leica) kit. With the exception of B2M, antigen retrieval was performed in citrate buffer (pH 6.0) for 20 min at 100 °C. Sections were then incubated with anti-HLA-A antibody HCA2 (HCE kweek, UMCU) 1:200, anti-HLA-B antibody HC10 (HCE kweek) 1:400, anti-B2M (Dako) 1:600, anti-HLA-DR-α (Dako) 1:60 or anti-HLA-DR-β (Dako) 1:800, consecutively followed by incubation with Bond Polymer Refine Detection kit items (Leica) and incubation with DAB solution (Bond Polymer Refine Detection, Leica). Slides were counterstained with hematoxylin (Bond Polymer Refine Detection, Leica), and blindly examined and scored for protein expression by two investigators (MSQK, PJD). Appropriate negative and positive controls were used.
Abbreviations: | ||
AFA | = | analysis of functional annotation |
ANOVA | = | analysis of variance |
ATCC | = | American type culture collection |
B2M | = | β-2 microglobulin |
CAT | = | correspondence at the top |
cDNA | = | complementary deoxyribonucleic acid |
CREB | = | cAMP response element binding protein |
CTCL | = | cutaneous T-cell lymphoma |
DAB | = | 3,3′-Diaminobenzidine |
DMEM | = | Dulbecco’s modified eagle medium |
DMSO | = | dimethyl sulfoxide |
DNA | = | deoxyribonucleic acid |
EGFR1 | = | epidermal growth factor receptor 1 |
FBS | = | fetal bovine serum |
FDR | = | false discovery rate |
FGS | = | functional gene set |
GEO | = | gene expression omnibus |
GO | = | Gene Ontology |
GSEA | = | Gene Set enrichment analysis |
HBSS | = | Hank’s balanced salt solution |
HDAC | = | histone deacetylase |
HDACi | = | histone deacetylase inhibitor |
HER2 | = | human epidermal growth factor receptor 2 |
HLA | = | human leukocyte antigen |
HPRD | = | human protein reference database |
IHC | = | immunohistochemistry |
ISRE | = | interferon-stimulated response element |
KEGG | = | Kyoto Encyclopedia of Genes and Genomes |
LILRB | = | Leukocyte immunoglobulin-like receptor B |
MHC | = | major histocompatibility complex |
MIAME | = | minimum information about a microarray experiment |
MSigDB | = | molecular signatures database |
mTOR | = | mammalian target of rapamycin |
NCBI | = | National Center for Biotechnology Information |
PCa | = | prostate cancer |
Plk1 | = | polo-like kinase 1 |
PPI | = | protein-protein interaction |
PSA | = | prostate-specific antigen |
RPMI | = | Roswell Park Memorial Institute medium |
SAHA | = | suberoylanilide hydroxamic acid |
TMA | = | tissue microarray |
UMCU | = | University Medical Center Utrecht |
US FDA | = | United States Food and Drug Administration |
VPA | = | valproic acid |
Additional material
Download Zip (11.2 MB)Acknowledgments
The authors would like to thank Marianna Zahurak for her contribution to the statistical analysis for this project and René Medema for his support and discussion. This study was supported by the Prostate Cancer Foundation, the Flight Attendant Medical Research Institute (SK), NIH (National Institute of Health) - NCI (National Cancer Institute) SPORE (Specialized Programs of Research Excellence) in Prostate Cancer P50CA058236 (MAC), by NIH-NCI 5P30CA006973 (MAC, LM, SK), and Aegon International Research Fellowship in Oncology (MAC, SK). MSQK was also partially supported by a fellowship from the Catharina van Tussebroek Fund. The funders had no role in the study design, data collection and analysis, decision to publish, or preparation of the manuscript.
Disclosure of Potential Conflicts of Interest
No potential conflicts of interest were disclosed.
Supplemental Materials
Supplemental materials may be found here: http://www.landesbioscience.com/journals/epigenetics/article/25574
References
- Villar-Garea A, Esteller M. Histone deacetylase inhibitors: understanding a new wave of anticancer agents. Int J Cancer 2004; 112:171 - 8; http://dx.doi.org/10.1002/ijc.20372; PMID: 15352027
- Prince HM, Bishton MJ, Harrison SJ. Clinical studies of histone deacetylase inhibitors. Clin Cancer Res 2009; 15:3958 - 69; http://dx.doi.org/10.1158/1078-0432.CCR-08-2785; PMID: 19509172
- Munster P, Marchion D, Bicaku E, Lacevic M, Kim J, Centeno B, et al. Clinical and biological effects of valproic acid as a histone deacetylase inhibitor on tumor and surrogate tissues: phase I/II trial of valproic acid and epirubicin/FEC. Clin Cancer Res 2009; 15:2488 - 96; http://dx.doi.org/10.1158/1078-0432.CCR-08-1930; PMID: 19318486
- Candelaria M, Gallardo-Rincón D, Arce C, Cetina L, Aguilar-Ponce JL, Arrieta O, et al. A phase II study of epigenetic therapy with hydralazine and magnesium valproate to overcome chemotherapy resistance in refractory solid tumors. Ann Oncol 2007; 18:1529 - 38; http://dx.doi.org/10.1093/annonc/mdm204; PMID: 17761710
- Arce C, Pérez-Plasencia C, González-Fierro A, de la Cruz-Hernández E, Revilla-Vázquez A, Chávez-Blanco A, et al. A proof-of-principle study of epigenetic therapy added to neoadjuvant doxorubicin cyclophosphamide for locally advanced breast cancer. PLoS One 2006; 1:e98; http://dx.doi.org/10.1371/journal.pone.0000098; PMID: 17183730
- Mahalingam D, Medina EC, Esquivel JA 2nd, Espitia CM, Smith S, Oberheu K, et al. Vorinostat enhances the activity of temsirolimus in renal cell carcinoma through suppression of survivin levels. Clin Cancer Res 2010; 16:141 - 53; http://dx.doi.org/10.1158/1078-0432.CCR-09-1385; PMID: 20028765
- Chen X, Wong JY, Wong P, Radany EH. Low-dose valproic acid enhances radiosensitivity of prostate cancer through acetylated p53-dependent modulation of mitochondrial membrane potential and apoptosis. Mol Cancer Res 2011; 9:448 - 61; http://dx.doi.org/10.1158/1541-7786.MCR-10-0471; PMID: 21303901
- Alimirah F, Chen J, Basrawala Z, Xin H, Choubey D. DU-145 and PC-3 human prostate cancer cell lines express androgen receptor: implications for the androgen receptor functions and regulation. FEBS Lett 2006; 580:2294 - 300; http://dx.doi.org/10.1016/j.febslet.2006.03.041; PMID: 16580667
- Seo SK, Jin HO, Woo SH, Kim YS, An S, Lee JH, et al. Histone deacetylase inhibitors sensitize human non-small cell lung cancer cells to ionizing radiation through acetyl p53-mediated c-myc down-regulation. J Thorac Oncol 2011; 6:1313 - 9; http://dx.doi.org/10.1097/JTO.0b013e318220caff; PMID: 21642861
- Schaeffer EM, Marchionni L, Huang Z, Simons B, Blackman A, Yu W, et al. Androgen-induced programs for prostate epithelial growth and invasion arise in embryogenesis and are reactivated in cancer. Oncogene 2008; 27:7180 - 91; http://dx.doi.org/10.1038/onc.2008.327; PMID: 18794802
- Daniel VC, Marchionni L, Hierman JS, Rhodes JT, Devereux WL, Rudin CM, et al. A primary xenograft model of small-cell lung cancer reveals irreversible changes in gene expression imposed by culture in vitro. Cancer Res 2009; 69:3364 - 73; http://dx.doi.org/10.1158/0008-5472.CAN-08-4210; PMID: 19351829
- Kim SY, Volsky DJ. PAGE: parametric analysis of gene set enrichment. BMC Bioinformatics 2005; 6:144; http://dx.doi.org/10.1186/1471-2105-6-144; PMID: 15941488
- Mootha VK, Lepage P, Miller K, Bunkenborg J, Reich M, Hjerrild M, et al. Identification of a gene causing human cytochrome c oxidase deficiency by integrative genomics. Proc Natl Acad Sci U S A 2003; 100:605 - 10; http://dx.doi.org/10.1073/pnas.242716699; PMID: 12529507
- Subramanian A, Tamayo P, Mootha VK, Mukherjee S, Ebert BL, Gillette MA, et al. Gene set enrichment analysis: a knowledge-based approach for interpreting genome-wide expression profiles. Proc Natl Acad Sci U S A 2005; 102:15545 - 50; http://dx.doi.org/10.1073/pnas.0506580102; PMID: 16199517
- Kortenhorst MS, Zahurak M, Shabbeer S, Kachhap S, Galloway N, Parmigiani G, et al. A multiple-loop, double-cube microarray design applied to prostate cancer cell lines with variable sensitivity to histone deacetylase inhibitors. Clin Cancer Res 2008; 14:6886 - 94; http://dx.doi.org/10.1158/1078-0432.CCR-08-0119; PMID: 18980983
- Hudson RS, Yi M, Esposito D, Watkins SK, Hurwitz AA, Yfantis HG, et al. MicroRNA-1 is a candidate tumor suppressor and prognostic marker in human prostate cancer. Nucleic Acids Res 2012; 40:3689 - 703; http://dx.doi.org/10.1093/nar/gkr1222; PMID: 22210864
- Lamb J. The Connectivity Map: a new tool for biomedical research. Nat Rev Cancer 2007; 7:54 - 60; http://dx.doi.org/10.1038/nrc2044; PMID: 17186018
- Ross AE, Marchionni L, Vuica-Ross M, Cheadle C, Fan J, Berman DM, et al. Gene expression pathways of high grade localized prostate cancer. Prostate 2011; http://dx.doi.org/10.1002/pros.21373; PMID: 21360566
- Ross AE, Marchionni L, Phillips TM, Miller RM, Hurley PJ, Simons BW, et al. Molecular effects of genistein on male urethral development. J Urol 2011; 185:1894 - 8; http://dx.doi.org/10.1016/j.juro.2010.12.095; PMID: 21421236
- He X, Marchionni L, Hansel DE, Yu W, Sood A, Yang J, et al. Differentiation of a highly tumorigenic basal cell compartment in urothelial carcinoma. Stem Cells 2009; 27:1487 - 95; http://dx.doi.org/10.1002/stem.92; PMID: 19544456
- Croft D, O’Kelly G, Wu G, Haw R, Gillespie M, Matthews L, et al. Reactome: a database of reactions, pathways and biological processes. Nucleic Acids Res 2011; 39:Database issue D691 - 7; http://dx.doi.org/10.1093/nar/gkq1018; PMID: 21067998
- Goel R, Harsha HC, Pandey A, Prasad TS. Human Protein Reference Database and Human Proteinpedia as resources for phosphoproteome analysis. Mol Biosyst 2012; 8:453 - 63; http://dx.doi.org/10.1039/c1mb05340j; PMID: 22159132
- Ashburner M, Ball CA, Blake JA, Botstein D, Butler H, Cherry JM, et al, The Gene Ontology Consortium. Gene ontology: tool for the unification of biology. Nat Genet 2000; 25:25 - 9; http://dx.doi.org/10.1038/75556; PMID: 10802651
- Harris MA, Clark J, Ireland A, Lomax J, Ashburner M, Foulger R, et al, Gene Ontology Consortium. The Gene Ontology (GO) database and informatics resource. Nucleic Acids Res 2004; 32:Database issue D258 - 61; http://dx.doi.org/10.1093/nar/gkh036; PMID: 14681407
- Kanehisa M, Goto S. KEGG: kyoto encyclopedia of genes and genomes. Nucleic Acids Res 2000; 28:27 - 30; http://dx.doi.org/10.1093/nar/28.1.27; PMID: 10592173
- Kanehisa M, Goto S, Hattori M, Aoki-Kinoshita KF, Itoh M, Kawashima S, et al. From genomics to chemical genomics: new developments in KEGG. Nucleic Acids Res 2006; 34:Database issue D354 - 7; http://dx.doi.org/10.1093/nar/gkj102; PMID: 16381885
- Kanehisa M, Araki M, Goto S, Hattori M, Hirakawa M, Itoh M, et al. KEGG for linking genomes to life and the environment. Nucleic Acids Res 2008; 36:Database issue D480 - 4; http://dx.doi.org/10.1093/nar/gkm882; PMID: 18077471
- Mootha VK, Lindgren CM, Eriksson KF, Subramanian A, Sihag S, Lehar J, et al. PGC-1alpha-responsive genes involved in oxidative phosphorylation are coordinately downregulated in human diabetes. Nat Genet 2003; 34:267 - 73; http://dx.doi.org/10.1038/ng1180; PMID: 12808457
- Wheeler DL, Barrett T, Benson DA, Bryant SH, Canese K, Chetvernin V, et al. Database resources of the National Center for Biotechnology Information. Nucleic Acids Res 2008; 36:Database issue D13 - 21; http://dx.doi.org/10.1093/nar/gkm1000; PMID: 18045790
- Cerami EG, Gross BE, Demir E, Rodchenkov I, Babur O, Anwar N, et al. Pathway Commons, a web resource for biological pathway data. Nucleic Acids Res 2011; 39:Database issue D685 - 90; http://dx.doi.org/10.1093/nar/gkq1039; PMID: 21071392
- Clauset A, Newman ME, Moore C. Finding community structure in very large networks. Phys Rev E Stat Nonlin Soft Matter Phys 2004; 70:066111; http://dx.doi.org/10.1103/PhysRevE.70.066111; PMID: 15697438
- van den Elsen PJ, Holling TM, Kuipers HF, van der Stoep N. Transcriptional regulation of antigen presentation. Curr Opin Immunol 2004; 16:67 - 75; http://dx.doi.org/10.1016/j.coi.2003.11.015; PMID: 14734112
- Gobin SJ, Peijnenburg A, Keijsers V, van den Elsen PJ. Site alpha is crucial for two routes of IFN gamma-induced MHC class I transactivation: the ISRE-mediated route and a novel pathway involving CIITA. Immunity 1997; 6:601 - 11; http://dx.doi.org/10.1016/S1074-7613(00)80348-9; PMID: 9175838
- Irizarry RA, Warren D, Spencer F, Kim IF, Biswal S, Frank BC, et al. Multiple-laboratory comparison of microarray platforms. Nat Methods 2005; 2:345 - 50; http://dx.doi.org/10.1038/nmeth756; PMID: 15846361
- Bolanos-Garcia VM. Assessment of the mitotic spindle assembly checkpoint (SAC) as the target of anticancer therapies. Curr Cancer Drug Targets 2009; 9:131 - 41; http://dx.doi.org/10.2174/156800909787580980; PMID: 19275754
- Philip PA, Benedetti J, Corless CL, Wong R, O’Reilly EM, Flynn PJ, et al. Phase III study comparing gemcitabine plus cetuximab versus gemcitabine in patients with advanced pancreatic adenocarcinoma: Southwest Oncology Group-directed intergroup trial S0205. J Clin Oncol 2010; 28:3605 - 10; http://dx.doi.org/10.1200/JCO.2009.25.7550; PMID: 20606093
- Herbst RS, Ansari R, Bustin F, Flynn P, Hart L, Otterson GA, et al. Efficacy of bevacizumab plus erlotinib versus erlotinib alone in advanced non-small-cell lung cancer after failure of standard first-line chemotherapy (BeTa): a double-blind, placebo-controlled, phase 3 trial. Lancet 2011; 377:1846 - 54; http://dx.doi.org/10.1016/S0140-6736(11)60545-X; PMID: 21621716
- Rosenthal SA, Bae K, Pienta KJ, Sobczak ML, Asbell SO, Rajan R, et al, Radiation Therapy Oncology Group Trial 9902. Phase III multi-institutional trial of adjuvant chemotherapy with paclitaxel, estramustine, and oral etoposide combined with long-term androgen suppression therapy and radiotherapy versus long-term androgen suppression plus radiotherapy alone for high-risk prostate cancer: preliminary toxicity analysis of RTOG 99-02. Int J Radiat Oncol Biol Phys 2009; 73:672 - 8; http://dx.doi.org/10.1016/j.ijrobp.2008.05.020; PMID: 18990504
- Hughes TR, Marton MJ, Jones AR, Roberts CJ, Stoughton R, Armour CD, et al. Functional discovery via a compendium of expression profiles. Cell 2000; 102:109 - 26; http://dx.doi.org/10.1016/S0092-8674(00)00015-5; PMID: 10929718
- Tomasi TB, Magner WJ, Khan AN. Epigenetic regulation of immune escape genes in cancer. Cancer Immunol Immunother 2006; 55:1159 - 84; http://dx.doi.org/10.1007/s00262-006-0164-4; PMID: 16680460
- Sharpe JC, Abel PD, Gilbertson JA, Brawn P, Foster CS. Modulated expression of human leucocyte antigen class I and class II determinants in hyperplastic and malignant human prostatic epithelium. Br J Urol 1994; 74:609 - 16; http://dx.doi.org/10.1111/j.1464-410X.1994.tb09193.x; PMID: 7530126
- Tamura K, Furihata M, Tsunoda T, Ashida S, Takata R, Obara W, et al. Molecular features of hormone-refractory prostate cancer cells by genome-wide gene expression profiles. Cancer Res 2007; 67:5117 - 25; http://dx.doi.org/10.1158/0008-5472.CAN-06-4040; PMID: 17545589
- Slamon DJ, Leyland-Jones B, Shak S, Fuchs H, Paton V, Bajamonde A, et al. Use of chemotherapy plus a monoclonal antibody against HER2 for metastatic breast cancer that overexpresses HER2. N Engl J Med 2001; 344:783 - 92; http://dx.doi.org/10.1056/NEJM200103153441101; PMID: 11248153
- Josson S, Nomura T, Lin JT, Huang WC, Wu D, Zhau HE, et al. β2-microglobulin induces epithelial to mesenchymal transition and confers cancer lethality and bone metastasis in human cancer cells. Cancer Res 2011; 71:2600 - 10; http://dx.doi.org/10.1158/0008-5472.CAN-10-3382; PMID: 21427356
- Small EJ, Schellhammer PF, Higano CS, Redfern CH, Nemunaitis JJ, Valone FH, et al. Placebo-controlled phase III trial of immunologic therapy with sipuleucel-T (APC8015) in patients with metastatic, asymptomatic hormone refractory prostate cancer. J Clin Oncol 2006; 24:3089 - 94; http://dx.doi.org/10.1200/JCO.2005.04.5252; PMID: 16809734
- van den Eertwegh AJ, Versluis J, van den Berg HP, Santegoets SJ, van Moorselaar RJ, van der Sluis TM, et al. Combined immunotherapy with granulocyte-macrophage colony-stimulating factor-transduced allogeneic prostate cancer cells and ipilimumab in patients with metastatic castration-resistant prostate cancer: a phase 1 dose-escalation trial. Lancet Oncol 2012; 13:509 - 17; http://dx.doi.org/10.1016/S1470-2045(12)70007-4; PMID: 22326922
- Kantoff PW, Higano CS, Shore ND, Berger ER, Small EJ, Penson DF, et al, IMPACT Study Investigators. Sipuleucel-T immunotherapy for castration-resistant prostate cancer. N Engl J Med 2010; 363:411 - 22; http://dx.doi.org/10.1056/NEJMoa1001294; PMID: 20818862
- Huber ML, Haynes L, Parker C, Iversen P. Interdisciplinary critique of sipuleucel-T as immunotherapy in castration-resistant prostate cancer. J Natl Cancer Inst 2012; 104:273 - 9; http://dx.doi.org/10.1093/jnci/djr514; PMID: 22232132
- Khan AN, Tomasi TB. Histone deacetylase regulation of immune gene expression in tumor cells. Immunol Res 2008; 40:164 - 78; http://dx.doi.org/10.1007/s12026-007-0085-0; PMID: 18213528
- Bernal M, Ruiz-Cabello F, Concha A, Paschen A, Garrido F. Implication of the β2-microglobulin gene in the generation of tumor escape phenotypes. Cancer Immunol Immunother 2012; 61:1359 - 71; http://dx.doi.org/10.1007/s00262-012-1321-6; PMID: 22833104
- Ma X, Ezzeldin HH, Diasio RB. Histone deacetylase inhibitors: current status and overview of recent clinical trials. Drugs 2009; 69:1911 - 34; http://dx.doi.org/10.2165/11315680-000000000-00000; PMID: 19747008
- Noh EJ, Lim DS, Jeong G, Lee JS. An HDAC inhibitor, trichostatin A, induces a delay at G2/M transition, slippage of spindle checkpoint, and cell death in a transcription-dependent manner. Biochem Biophys Res Commun 2009; 378:326 - 31; http://dx.doi.org/10.1016/j.bbrc.2008.11.057; PMID: 19038231
- Kretzner L, Scuto A, Dino PM, Kowolik CM, Wu J, Ventura P, et al. Combining histone deacetylase inhibitor vorinostat with aurora kinase inhibitors enhances lymphoma cell killing with repression of c-Myc, hTERT, and microRNA levels. Cancer Res 2011; 71:3912 - 20; http://dx.doi.org/10.1158/0008-5472.CAN-10-2259; PMID: 21502403
- Wissing MD, Mendonca J, Kortenhorst MSQ, Kaelber NS, Gonzalez M, Kim E, et al. Targeting prostate cancer through a combination of Polo-like kinase inhibitors and histone deacetylase inhibitors [abstract]. In: Proceedings of the 101st Annual Meeting of the American Association for Cancer Research; 2010 Apr 17-21; Washington, DC. Philadelphia (PA): AACR; 2010. Abstract nr 5414.
- Paller CJ, Wissing MD, Kim E, Kortenhorst MSQ, Gerber S, Rosen M, et al. Preclinical profile of AMG 900 in combination with HDACIs in prostate cancer [abstract]. In: Proceedings of the 103rd Annual Meeting of the American Association for Cancer Research; 2012 Mar 31-Apr 4; Chicago, IL. Philadelphia (PA): AACR; 2012. Abstract nr 2049.
- Chou YW, Chaturvedi NK, Ouyang S, Lin FF, Kaushik D, Wang J, et al. Histone deacetylase inhibitor valproic acid suppresses the growth and increases the androgen responsiveness of prostate cancer cells. Cancer Lett 2011; 311:177 - 86; http://dx.doi.org/10.1016/j.canlet.2011.07.015; PMID: 21862211
- van Diest PJ. No consent should be needed for using leftover body material for scientific purposes. For. BMJ 2002; 325:648 - 51; http://dx.doi.org/10.1136/bmj.325.7365.648; PMID: 12242180
- Ihaka R, Gentleman RR. A language for data analysis and graphics. J Comput Graph Statist 1996; 5:299 - 314
- Gentleman RC, Carey VJ, Bates DM, Bolstad B, Dettling M, Dudoit S, et al. Bioconductor: open software development for computational biology and bioinformatics. Genome Biol 2004; 5:R80; http://dx.doi.org/10.1186/gb-2004-5-10-r80; PMID: 15461798
- Smyth GK. Linear models and empirical bayes methods for assessing differential expression in microarray experiments. Stat Appl Genet Mol Biol 2004; 3:e3; http://dx.doi.org/10.2202/1544-6115.1027; PMID: 16646809
- Smyth GK, Michaud J, Scott HS. Use of within-array replicate spots for assessing differential expression in microarray experiments. Bioinformatics 2005; 21:2067 - 75; http://dx.doi.org/10.1093/bioinformatics/bti270; PMID: 15657102
- Smyth GK, Speed T. Normalization of cDNA microarray data. Methods 2003; 31:265 - 73; http://dx.doi.org/10.1016/S1046-2023(03)00155-5; PMID: 14597310
- Smyth GK, Yang YH, Speed T. Statistical issues in cDNA microarray data analysis. Methods Mol Biol 2003; 224:111 - 36; PMID: 12710670
- Smyth GK. Limma: linear models for microarray data. In: Gentleman R, Carey RV, Dudoit S, Irizarry R, Huber W, editors. Bioinformatics and Computational Biology Solutions using R and Bioconductor. Springer; 2005. 397-420.
- Benassi B, Flavin R, Marchionni L, Zanata S, Pan Y, Chowdhury D, et al. MYC is activated by USP2a-mediated modulation of microRNAs in prostate cancer. [Published OnlineFirst January 5, 2012] Cancer Discov 2012; 2:236 - 47; http://dx.doi.org/10.1158/2159-8290.CD-11-0219; PMID: 22585994
- Sayers EW, Barrett T, Benson DA, Bolton E, Bryant SH, Canese K, et al. Database resources of the National Center for Biotechnology Information. Nucleic Acids Res 2012; 40:Database issue D13 - 25; http://dx.doi.org/10.1093/nar/gkr1184; PMID: 22140104
- Benjamini Y, Hochberg Y. Controlling the False Discovery Rate: a Practical and Powerful Approach to Multiple Testing. J R Stat Soc, B 1995; 57:289 - 300
- Roy S, Jeffrey R, Tenniswood M. Array-based analysis of the effects of trichostatin A and CG-1521 on cell cycle and cell death in LNCaP prostate cancer cells. Mol Cancer Ther 2008; 7:1931 - 9; http://dx.doi.org/10.1158/1535-7163.MCT-07-2353; PMID: 18645003
- Vindeløv LL, Christensen IJ, Nissen NI. A detergent-trypsin method for the preparation of nuclei for flow cytometric DNA analysis. Cytometry 1983; 3:323 - 7; http://dx.doi.org/10.1002/cyto.990030503; PMID: 6188586