Abstract
Illumina’s Infinium HumanMethylation450 BeadChip arrays were used to examine genome-wide DNA methylation profiles in 22 sample pairs from colorectal cancer (CRC) and adjacent tissues and 19 colon tissue samples from cancer-free donors. We show that the methylation profiles of tumors and healthy tissue samples can be clearly distinguished from one another and that the main source of methylation variability is associated with disease status. We used different statistical approaches to evaluate the methylation data. In general, at the CpG-site level, we found that common CRC-specific methylation patterns consist of at least 15,667 CpG sites that were significantly different from either adjacent healthy tissue or tissue from cancer-free subjects. Of these sites, 10,342 were hypermethylated in CRC, and 5,325 were hypomethylated. Hypermethylated sites were common in the maximum number of sample pairs and were mostly located in CpG islands, where they were significantly enriched for differentially methylated regions known to be cancer-specific. In contrast, hypomethylated sites were mostly located in CpG shores and were generally sample-specific. Despite the considerable variability in methylation data, we selected a panel of 14 highly robust candidates showing methylation marks in genes SND1, ADHFE1, OPLAH, TLX2, C1orf70, ZFP64, NR5A2, and COL4A. This set was successfully cross-validated using methylation data from 209 CRC samples and 38 healthy tissue samples from The Cancer Genome Atlas consortium (AUC = 0.981 [95% CI: 0.9677–0.9939], sensitivity = 100% and specificity = 82%). In summary, this study reports a large number of loci with novel differential methylation statuses, some of which may serve as candidate markers for diagnostic purposes.
Introduction
Colorectal cancer (CRC) remains a major cancer concern worldwide. In Russia, its incidence and mortality have significantly increased over the past decade. CRC poses the second highest morbidity due to cancer in Russian men, 10.9%, following lung cancer; in Russian women, the CRC mortality is 12.3%, third after breast and skin cancer.Citation1 Although numerous studies have revealed a series of molecular alterations associated with and involved in CRC pathogenesis, the current knowledge remains insufficient for early diagnosis and adequate risk assessment. The last decade, however, has seen advances in new genome-scale technologies that have been used to characterize the full spectrum of molecular heterogeneity in many types of cancer cells.Citation2-Citation4 At present, it is generally assumed that CRC arises as a consequence of an accumulation of genetic and epigenetic alterations, which transforms colonic epithelial cells into adenocarcinoma cells.Citation5 The epigenetic changes associated with CRC, especially aberrant CpG island methylation in the promoter regions of tumor suppressor genes, have become an area of great interest in the field of cancer research. In general, up to 10% of CpG islands in cancer epigenomes may be aberrantly methylated, which can lead to the silencing of thousands of gene promoters in the average cancer.Citation6,Citation7 Moreover, recent studies have shown that CRC-associated aberrant methylation is not exclusively limited to CpG islands but may be extended to “CpG island shores” or areas that are less dense in CpG dinucleotides within 2 kb upstream of a CpG island.Citation8 The methylation of CpG island shores may also be associated with the transcriptional inactivation and expression of splice variants.
Recent technological advances now offer the ability to explore the processes underlying tumorigenesis at the genome level while performing a large-scale search for new candidate biomarkers for cancer diagnosis. One of the most widely used commercial platforms for methylation profiling at the genome level and at single CpG resolution is the Infinium Methylation Assay from Illumina, Inc. The recently launched Infinium HumanMethylation450 BeadChip presents a significant improvement in CpG site density detection (482,421 CpG and 3,091 non-CpG sites) and, at the gene level, covers 99% of RefSeq genes with multiple sites in annotated promoters (1,500 bp or 200 bp upstream of the transcription start site), 5′-UTRs, first exons, gene body and 3′-UTRs.Citation9
To our knowledge, no published study has focused on epigenetic diversity in CRC using this version of the high-density methylation array. In the current study, CpG-level methylation statuses of tumor tissue and matched healthy tissue from CRC patients as well as normal tissue from cancer-free donors were obtained using Infinium HumanMethylation450 BeadChips. This enabled us to characterize differentially methylated regions involved in colorectal cancer pathogenesis and identify novel DNA methylation markers that have not previously been associated with aberrant methylation in CRC.
Results
Clinical and pathological characteristics of CRC patients
The clinical and pathological characteristics are described in . We analyzed cancer tissue samples (C) and matched healthy mucosa tissue samples (N1) from 22 patients diagnosed with colorectal adenocarcinomas at the State Research Center of Coloproctology (SRCC), Moscow, Russia. The inclusion criteria were as follows: no cancer other than CRC, no indications of CRC heredity and no radio- or chemo-therapy before surgical resection. In addition to patient samples, healthy colonic mucosa samples (N2) were obtained from 19 neoplasia-free subjects. These controls underwent screening colonoscopy but presented no colonic abnormalities and possessed no history of colonic neoplasia, IBD, or chemotherapy for any malignancies. We used those cancer-free samples to evaluate the possible presence of field cancerization in affected patients. After the purpose and nature of all of the procedures were fully explained, written consent was obtained from all patients. The study protocol was approved by the institutional review board at the Research Institute of Physical Chemical Medicine in Moscow, Russia.
Table 1. Patient characteristics
Normalization of methylation data
The genome-wide CpG methylation profiles of 22 pairs of CRC tissue and adjacent normal mucosa and 19 normal mucosa samples from cancer-free patients were generated using the HumanMethylation450 BeadChip. The estimated methylation status per sample totaled 485,577 loci, covering 21,231 genes. Methylation at each locus was measured using β-values that were generated using the Illumina GenomeStudio software based on the intensity of the methylated and unmethylated probes. Before further calculations, a built-in Detection Score filter was used, leaving only values with significantly higher mean signal intensities from multiple probes for a given CpG locus than those of the negative control in the same set of chip data (at the level of p < 0.05). The average number of loci detected (p < 0.05) for the CRC samples, adjacent healthy mucosa samples and healthy mucosa samples from cancer-free patients were 484,552, 484,035 and 484,647, respectively. These results suggested uniform amplification and hybridization conditions for all samples.
Infinum 1 (InfI) and Infinum 2 (InfII) represent two types of chemistry incorporated on the HumanMethylation450 BeadChip. Because each type utilizes different mechanisms for detection, some bias in β-value distributions has been shown in previous studies on the development of the new chip.Citation10,Citation11 We also noticed that InfI and InfII show different dynamic behaviors in the assay and that some data processing is required to make the two data sets comparable. The data normalization methods implemented in the current version of GenomeStudio software (v 2011.1) cannot remove the bias caused by the different types of chemistry. Therefore, to adjust β-values, we used a peak-based correction approach that was developed by Dedeurwaerder et al. and implemented in Illumina Methylation Analyzer (IMA), a recently published R package.Citation12
The density plots of the β-value distributions for the InfI and InfII probes from the same sample before and after peak correction are shown in Figure S1. A typical distribution of β-values has a bimodal shape in which one peak corresponds to low or unmethylated probes with a β-value close to 0, while the second peak corresponds to highly or fully methylated probes with a β-value close to 1.
Before correction, the peaks associated with the InfII probes were found to have a decreased quantitative dynamic range over less extreme values. After peak correction, the density plots associated with InfI and InfII were found to be more similar. During the subsequent calculations of differentially methylated regions, the corrected β-values for the InfI and InfII probes had a random, unbiased distribution in the compared groups. In contrast, using uncorrected data led to the enrichment of the InfI probes, which had a greater dynamic range (data not shown).
Analysis of variability in the methylation data
We tested different approaches to estimate the most significant source of variability in the methylation data from the clinical samples: multivariate ANOVA, principal component analysis (PCA) and pairwise Spearman's correlation. Of these, we used a multivariate ANOVA test because we expected that more than two dependent variables could be associated with the differences in methylation levels, including tissue type (cancer vs. normal), person-to-person variation, patient sex and chip-to-chip variation (batch effect).
Because sex chromosomes are highly methylated, we performed two variations of the test. The first test was based on the full set of data and the second test was based on data from which the β-values of the sex chromosomes were excluded. shows that “tissue type” and “sex” were the most significant sources of variation before the sex chromosome methylation data were filtered. The results from the multivariate ANOVA test based on autosomal β-values clearly show that tissue type is the main source of variability in the methylation data (Mean F Ratio 8.7), while the mean F ratio of the variability of other sources is still quite low.
Figure 1. Variation in methylation data. (A) Bar chart of methylation in the analyzed samples estimated using multivariate ANOVA. Blue bars show the results for all analyzed CpG sites included in the array; violet bars indicate results only for autosomal CpGs. The F-ratio for each factor (source) represents the F-statistics for that factor/F-statistics for error (noise). After removing sex chromosomal markers, the main source of variability in the methylation data are associated with tissue type (tumor vs normal). (B) The first two principle components identified in PCA of DNA methylation profiles distinguished tumor samples from healthy colon samples in the autosomal data set. Red dots indicate tumors (C); blue triangles indicate normal colon samples from patients with CRC (N1); green squares indicate normal colon samples from healthy donors (N2). Both N1 and N2 form dense clusters, while the methylation profiles in CRC samples are more variable. (C) Heatmap of Spearman's correlations and hierarchical clustering between all possible sample pairs based on all autosomal CpG sites. Normal samples are highly correlated in contrast to low correlation between N1 and CRC samples.
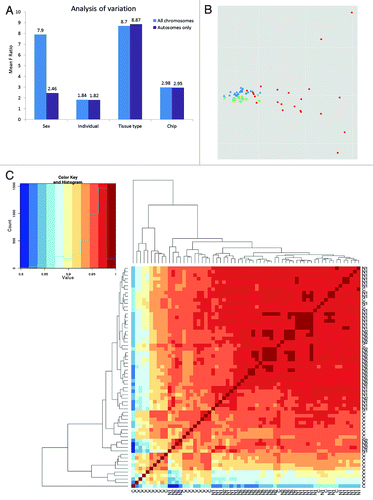
Considering this finding, we excluded all sex chromosome markers from the subsequent analysis of differential methylation in CRC and control samples. In summary, after the initial data normalization, peak correction and filtering of sex chromosomes β-values, the number of analyzed CpG sites in our data set remained 444,888 for each type of clinical sample.
The subsequent principal component analysis executed on 444,888 CpGs per data set suggested a clustering of samples by pathological status (tissue type) (). The plot of the first two components can explain approximately 46% of the variation observed. As follows from , samples from cancerous (N1) and non-cancerous (N2) normal tissue form dense clusters in accordance with methylation status; this process is in contrast to the more spatially distributed values for the pathological tissue samples (C).
To detect cluster similarities based on the different methylation statuses in the samples, we performed a pairwise Spearman's correlation between all possible pairs in the clinical samples. The calculated values of the correlation coefficient (0.8 to 1) directly corresponded to the similarity of the compared samples (Fig. S2). A colored heatmap visualization of Spearman's correlation data and the sample clustering data are presented in . Similar to the PCA-based plot, these data show a distinct cluster of highly correlated healthy tissue samples and more variability by methylation groups related to cancer tissue samples. Finally, we used a quantile-quantile (Q-Q) plot of –log10 (p-values) to visualize the association between methylation and disease status at each of the 444,888 CpG sites analyzed (Fig. S3). The observed quantiles are consistently higher than their expected values under the null hypothesis of no disease association, providing evidence of the site-specific disease association of a large number of CpG sites.
Differential methylation in colorectal carcinoma tissue compared with cancerous and non-cancerous colon tissue
We compared the genome-wide differential methylation status of 22 CRC tissue samples (C), 22 adjacent cancer-free colonic tissue samples (N1) and 19 samples of normal colonic tissue from cancer-free patients (N2). Two different sets of control samples were used to discriminate genes potentially involved in field cancerization, including genes already carrying hypermethylation events that are linked to an increased risk of carcinogenic progression.Citation13-Citation16 The differentially methylated regions (DMRs) were analyzed using the IMA-R package, as described in the methods section. To be conservative, we report only differentially methylated regions with absolute delta β-values of at least 0.2 at FDR 0.05.
We initially utilized the site-level version of the test based on all 444,888 CpG sites. By comparing CRC to adjacent normal tissue (C vs. N1), we found a total of 23,793 differentially methylated (DM) CpGs. A comparison between CRC and normal tissue from cancer-free subjects (C vs. N2) resulted in 23,688 CpG sites. In both comparisons, 17,821 CpG sites were confirmed to be differentially methylated. These sites were inspected for possible technical artifacts related to the cross-hybridization of probes with repetitive DNA regions and biases due to SNP-containing probes; 194 probes were found to map to multiple locations (at least 2 mismatches), 23 probes were located in genome repeats, and 1,936 probes containing single nucleotide polymorphisms (SNP) were excluded from further analysis. After data filtration 15,667 DM CpG sites remained, of which 10,342 CpG were hypermethylated and 5,325 were hypomethylated (;Table S1).
Figure 2. Genomic distribution of differentially methylated regions. (A) DMRs identified by site-level (CpGs) and region-level (gene- and CpG island-based categories) variants based on an analysis of differential methylation status. (B) Venn diagram of the intersection between DMRs identified using different methods. (C) Stacked bar charts showing the distribution of the hypermethylated and hypomethylated CpG sites over six gene categories: TSS1500, TSS200, 5′UTR, 1st exon, gene body, 3′UTR and intergenic regions. For categorization, the CpG counts were normalized by the number of CpGs in the same category represented on the 450K array. The percentage of normalized CpG counts is indicated in the bars. (D) Stacked bar charts showing the distribution of the hypermethylated and hypomethylated CpG sites over CpG islands, CpG shores, CpG shelves and Open Sea regions. For categorization, the CpG counts were normalized by the number of CpGs in the same category represented on the 450K array. The percentage of normalized CpG counts is indicated in the bars.
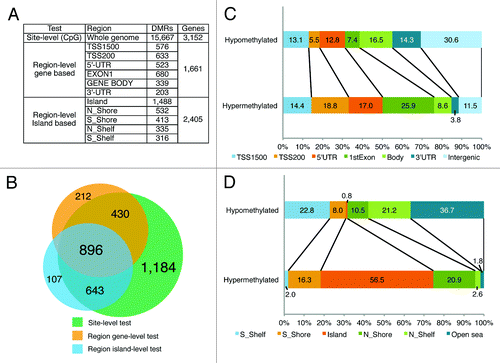
Next, we implemented two variations of a region-level differential test in IMA-R. In contrast to the site-level test, the comparisons are based on the average methylation values (methylation index) calculated for the incorporated number of CpGs in extended regions. Six gene-based regions were used to calculate the methylation index (TSS1500, TSS200, 5′-UTR, first exon, gene body, and 3′-UTR), and five CpG island-based regions were used (CpG island, south and north shores [regions flanking island], and south and north shelves [regions flanking shores]). The given region definition is based on the original Illumina methylation annotation for the Human Methylation450 BeadChip.Citation9
A gene-based variant of the region-level test resulted in the identification of 1,661 significant differentially methylated genes, which were common in both (C vs. N1) and (C vs. N2) comparisons. This number represents the sum of all unique gene names that resulted from six different, separately analyzed, gene categories: 5′-UTR (523 genes), TSS1500 (576 genes), TSS200 (633 genes), first exon (680 genes), gene body (339 genes) and 3′-UTR (203 genes) (;Table S2).
A CpG island-based variant of the region-level test was conducted in the same manner, resulting in 1,488 DMRs located in CpG islands, 532 and 413 DMRs located inside the north and south shores, respectively, and 335 and 316 DMRs distributed in the north and south shelves, respectively (Table S3). In total, this test resulted in 2,405 unique DMRs distributed among the five CpG-related regions ().
All primary data on DMRs calculated using the site-level, gene-based region-level and CpG island-based region-level tests are presented in the supplementary files.
We compared the DMR data obtained using different methods by mapping the positions identified in the site-level and CpG island tests and identifying the genes located in the islands, shores, and shelves. shows the numbers of differentially methylated genes found using identical criteria (absolute delta β = 0.2 at FDR 0.05) in the site-level, gene-based and CpG island-based tests.
There were at least 896 common genes identified using all compared methods (central intersection in the Venn diagram, (). The identification of this set of genes using various methods suggests that the genes are actually differentially methylated in CRC tissue compared with the N1 and N2 samples.
Finally, to establish a ranked list of DMRs within the results, we sorted our data according to the magnitude of differences in DNA methylation status among sample groups (β differences) and the statistical significance (adjusted P values). A volcano plot graphically represents the distribution of significant CpG sites from the site-level test sorted by B-differences and P-values (Fig. S4).
lists the 20 top-ranking markers identified by site- and region-level variants in the IMA test. The table contains 36 unique genes, five of which (ADHFE1, C1orf70, CHST2, MSC, and ZNF625) were identified using all three methods. These 36 genes were significantly hypermethylated in CRC, and 28 of them were previously reported to have altered DNA methylation patterns in CRC.Citation8,Citation17-Citation20 Eight of the top ranked genes (SND1, OPLAH, C1orf70, MIR124–3, C9orf50, ZFP64, DPY19L2,and ZNF829) have no published data on their methylation status in cancer development.
Table 2. List of the 20 top-ranking methylation markers identified by different methods
A direct comparison of these methods may not be accurate due to several limitations that are related to the averaging of the methylation data and gene mapping. Such an approach may incorrectly identify CpG sites that are outside the gene regions or CpG sites corresponding to different regions of different genes. Additionally, it is not always possible to correctly identify gene categories in the island-level IMA-R test. Moreover, multiple probes for the same gene can be both hyper- and hypomethylated in different gene regions. For example, in our CRC data set, the 34 probes located in the 5′UTR, TSS200 and TSS1500 regions of EYA4 were significantly hypermethylated, while 2 probes that hybridized to the gene body region showed hypomethylated statuses. Of the 3,152 total DM genes identified using the site-level test, 444 show both types of methylation differences.
The IMA-R approach to average the methylation values for the CpG sites located in the extended gene regions can also lead to either bias or data loss. In contrast to the site-level test, the region-level IMA-R test failed to identify some well-known methylation sites in CRC genes (i.e., SEPT9, SMAD3, TCF7L, and IGF2).Citation17,Citation21-Citation23 In subsequent steps, we thus focused on the CpG site-level methylation data analysis (15,667 CpGs) and considered the above-mentioned limitations of data representation at the gene level.
Genomic distribution and functional annotations of differentially methylated CpG sites
Of the 15,667 DM CpGs identified in the site-level test, 10,962 (70%) were mapped to 3,152 genes. The genomic and gene-related regions of the significantly hyper- or hypomethylated CpG sites are distributed differently. For comparison, we normalized CpG counts by the number of all CpGs in the same gene category represented on the 450K array. shows that the largest portion of hypermethylated CpGs (25.9%) were located in the 1st exon of the genes and subsequently decreased in other categories (18.8%, TSS200; 17.0%, 5′UTR; 14.4%, TSS1500; 11.5%, Intergenic regions; 8.6%, Gene body and 3.8% in 3′UTR). In contrast, almost a third (30.6%) of the significantly hypomethylated CpG sites were not associated with known genes, while the rest were mainly located in gene body (16.5%) and 3′UTR (14.3%) regions and, to a lesser extent, in other gene categories. The distribution analysis of significantly differentially methylated CpG sites in genomic regions showed that 56.5% of the hypermethylated CpG sites are in CpG islands and that fewer are in the north (20.9%) and south (16.3%) CpG shores or the north (2.6%) and south (2%) CpG shelves (). In contrast, significantly hypomethylated CpG sites are more common in the open sea (36.7%), north (33.5%) and south (36%) CpG shelves than in other genomic regions.
Table S4 lists additional biological characteristics and classifications of differentially methylated CpG sites that we identified in accordance with the array manufacturer's annotated features. A substantial portion of these CpG sites are located within annotated regulatory elements and significantly enriched for informatics-predicted enhancers (4,604 CpGs), DNase I hypersensitive sites (4,083 CpGs), and unclassified cell type specific regulatory elements (2,276 CpGs). More than 29% (4,614/15,667) of the differentially methylated CpG sites that we found coincided with annotated DMRs. Nevertheless, the distributions of hypermethylated and hypomethylated CpG sites in these categories were asymmetrical. Hypermethylated CpG sites constitute 24.2% of all array-annotated DMRs, whereas hypomethylated sites constitute only 0.8%. The prevalence of hypermethylated to hypomethylated CpGs was also observed in categories of DNase I Hypersensitive sites (3,502 to 581 CpGs) and unclassified cell type specific regulatory elements (1,971 to 305 CpGs). The ratio was different only in the predicted enhancers category wherein the number of hyper- and hypo-methylated CpG sites was approximately equal (2,241 to 2,363), but enrichment was significant only for hypomethylated CpG sites (p = 3.30E-15). Considerable enrichment of the hypomethylated CpGs for predicted enhancers may suggest possible transcriptional activities of the corresponding genes. In addition to the biological characteristics, Table S4 shows the unbiased distribution of DM CpGs identified by InfI and InfII probes (4,715/10,952 [43.05%]) when compared with the ratio of the total InfI and InfII probes represented on the array (135,501/350,076 [38.7%]), indicating effective data normalization.
In the next step, we analyzed the possible over-representation of some Gene Ontology (GO), Panther Pathway and KEGG Pathway database categories among the identified hypermethylated genes. The analysis was performed using the web-based tools GREAT v 2.0. and Gene Set Analysis Toolkit V2.Citation24,Citation25 Using the basal+extension associations rule, we mapped hypermethylated CpG sites to the nearest genes on the GREAT web server using the following parameters: constitutive 1.0 kb upstream, 1.0 kb downstream and up to 1.0 kb max extension. An analysis of the 1,229 hypermethylated genes revealed the significant enrichment of multiple modules in various GO terms (Table S5). For the list of analyzed genes, if we used “molecular function” for categorization, then we observed that the most enriched groups include DNA binding, transcription factor activity and large module of “channel activities” functional categories. Categorization by “biological process” showed significant enrichment for developmental and cell differentiation activities, and the “cellular component” category enrichment test indicated that proteins encoded by analyzed genes mainly associate with plasma membranes. These data are consistent with the findings from recently published CRC methylation studies. Significant enrichment of hypermethylated genes for DNA binding and transcription factor activity categories has been shown in studies that used the previous version of the Illumina HumanMethylation array (27K).Citation17,Citation18 The similar findings as well as enrichment for channel activity GO categories have been described in a recent CRC genome-wide methylation sequencing study by Simmer et al.Citation26 At the same time, there are rather modest relationships between results of GO enrichment analysis for DNA methylation and published gene expression data in CRC. For “biological process,” two general GO categories (multicellular organismal process and anatomical structure developmental) were consistently overrepresented in our gene list compared with data from a recent systematic enrichment analysis of gene expression in CRC development.Citation27
Pathway-based analyses showed a significant enrichment for genes involved in the Wnt and Cadherin signaling pathways and for genes that play a role in neuroactive ligand-receptor interaction, the calcium signaling pathway and cell adhesion processes. Among them, the Wnt and cadherin-signaling pathways attracted more attention. In addition to mutations in Wnt pathway components, the silencing of Wnt antagonists by DNA hypermethylation was confirmed for CRC and other cancers (human medulloblastoma and pancreatic adenocarcinoma).Citation28-Citation30 One of the key components of the WNT signaling pathway, β-catenin, also functions as a component of the cadherin complex. The cadherin gene family encodes proteins that control cell-cell adhesion and influence cell migration, and they are also known to be involved in colorectal carcinogenesis.Citation31
In summary, the network analysis of DM CpG sites shows a significant enrichment for GO and pathway categories altered in CRC and other cancers, providing evidence that methylation changes in these sites are biologically meaningful. In turn, the substantial predominance of hypermethylated CpG sites in the promoter regions and 1st exon categories, as well as the enrichment of hypermethylated CpG sites in CpG islands, suggest the potential prevalence of gene inactivation mechanisms in CRC development. Most hypomethylated CpG sites are located in open sea and shelf regions, implying a potential role for CpG methylation in genomic instability.
Methylation features in normal tissue from CRC patients and healthy donors
Initially, we used two variants of normal tissue, CRC-related tissue (N1) and healthy donor tissue (N2), to evaluate possible differences in gene methylation related to field cancerization. Previously, marked differences in gene methylation levels between people with and without colon neoplasms have been shown for a number of genes,Citation13-Citation16 although our data analysis did not reveal much difference. Principal component analysis (PCA) executed on the whole data set showed a clustering of samples by pathological status but little substantial variances between N1 and N2 tissues (). The results of the PCA analysis on normal only samples (N1 and N2) showed significant, but small, differences in their methylation status (Fig. S5). Nevertheless, no differentially methylated regions were found to be significantly associated with methylation differences using the IMA-R test (abs. delta β-value > 0.2 at FDR 0.05). Given that most of the published field effect genes are hypermethylated in CRC, less methylated in CRC-related normal tissue and least methylated in the cancer-free control, we decided to more thoroughly review the pattern in the methylation values of previously identified DM CpG sites in the three data sets. We used a filter to sort the significantly hypermethylated sites with a sequential decrease in methylation in the C→N1→N2 direction and at least a 0.1 β difference between each group (∆β ≥ 10C−N1, ∆β ≥ 10N1−N2). As a result, we generated a list of 284 CpG sites located in the 5′UTR, TSS200, TSS1500, and 1st exon regions of 171 genes (Table S6).
Of these 171 genes, at least four, NEFM, SFP1, WIF1, and ESR1, have been published previously as “field effect” genes. NEFM encodes a neurofilament medium polypeptide that was shown to have increased methylation levels in individuals with both past and present H. Pylori infection in a recent study on gastric cancer.Citation32SFRP1 is one gene that is commonly methylated and silenced in CRC. For SFRP1, Belshaw et al.Citation13 showed age-dependent methylation in normal colon mucosa and found differences in gene methylation levels between people with and without CRC. In the same study, significant epigenetic modifications in apparently normal mucosa were also shown for the genes WIF1 and ESR1. Most of the genes identified (102, Table S6) are known to be frequently methylated in CRC, although their role in the field cancerization remains unclear.
Molecular heterogeneity of pathological samples
shows a PCA score plot for the methylation statuses of all analyzed CpG sites; these data show that the pathological samples are more variable than either N1 or N2. To evaluate the extent of variability among the pathological samples, we calculated the methylation differences in each of the 22 cancer and adjacent normal tissue pairs with absolute values of β-differences greater than 0.2 (see Methods). In contrast to the site-level IMA-R test, the number of DM CpG sites identified in each sample pair varied greatly, with values ranging from 2,058 to 100,731. A direct comparison of these tests showed that only 13 to 44 percent of the 15,667 core CpG sites overlapped with the sites found in the paired test. Moreover, hypomethylated CpG sites in 18 of the 22 pairs appeared to be more common than hypermethylated CpGs in the CRC tissue samples (Table S7). Nevertheless, in the combined set of all unique DM CpG sites that we identified in sample pairs, hypermethylation slightly predominated over hypomethylation. Despite the great variability of methylation at the individual level, some DM CpG sites occurred more frequently in the sample pairs: at least 11 DM CpG sites are common to all 22 pairs, while many more are shared between fewer pairs (Table S8).
In a recently published study that assessed the recurrent status of DMR, “support” (a variable defined as the number of tumors in which a region was differentially methylated compared with the matched normal tissues) was calculated using a paired test.Citation26 In our study, the support values of the DM CpG sites are integers between 0 and 22, as 22 paired samples were analyzed. To set up the threshold value for support, we approximated the experimental distribution of the support value using a binomial distribution with parameters n = 22, p = 0.045 (see Methods). After Bonferroni correction, we established a minimal support value of 10 as being statistically significant. Therefore, the DM CpG sites identified using our paired test were defined as statistically significant if they were common to at least 10 paired samples. In total, 14,499 hypermethylated and 15,539 hypomethylated CpG sites that were common in sample pairs and had support values of 10–22 were selected (Table S9). There is a clear tendency of the proportion of hypermethylated sites to increase in CRC with increasing support values compared with normal tissue, whereas large fractions of CpGs with lower support values can be either hyper- or hypomethylated (Table S8 and Fig. S6). The same direct relationship was noted by Simmer et al.,Citation26 who suggested that hypermethylated CpG sites with high support may have a common functional role in the tumors. The more heterogeneous and sample-specific CpG sites are likely to be sporadic.
Several studies on the variability of methylation in CRC showed that a subset of the cancers named CpG island methylator phenotype (CIMP) are associated with high degrees of aberrant methylation at a specific set of genomic loci.Citation33,Citation34 CIMP cancers seem to have distinct epidemiology, histology, and molecular features and can significantly contribute to the molecular heterogeneity of CRC.Citation35 We analyzed our tumor samples for CIMP status using a five-locus marker panel (RUNX3, SOCS1, NEUROG1, IGF2, and CACNA1G) proposed by Weisenberger et al.Citation34 Tumor samples demonstrating hypermethylation (β-value > 0.8) in the TSS200 region in at least 3 of the 5 loci were classified as CIMP-positive. Only 2 of the 22 CRC samples (93p2 and 88p2) met this criterion, consistent with published findings demonstrating a low frequency (3–12%) of CIMP variants in the distal colon and rectal cancers.Citation36,Citation37 Both 93p2 and 88p2 had a large number of DM CpG sites (100,731 and 80,961, respectively). At the same time, they were negative for somatic mutations in KRAS and BRAF genes that are frequently found in CIMP-positive CRC samples.Citation38
Finally, we assessed the possible relation between DNA methylation in CRC and available data for somatic mutations and clinicopathological characteristics. The combined data on the molecular heterogeneity of the pathological samples and available clinical traits are listed in Table S10. PCA and hierarchical clustering based on the DNA methylation profiles were performed to categorize the CRC samples into different subgroups. Stable clusters were obtained for CRC samples with a CIMP-positive phenotype, but they were not obtained for other traits (Fig. S7). Although a weak trend in clustering according to the PCA plot based on histological grades was observed, there were no clear correlations with other clinical (e.g., tumor stage, sex, age) or molecular (mutations) data (Fig. S8). No regions were found to be significantly associated with the suggested histological grade differences (low vs. moderately differentiated) at the gene level.
Selection of diagnostic markers
Hypermethylation of CpG islands is a promising biomarker that shows high potential for translation into non-invasive CRC detection approaches.Citation39 Some methylation markers are already being used in clinical practice. Among them, stoolbased methylated vimentin (VIM) and bloodbased methylated septin (SEPT9) are considered to be non-invasive, clinically validated markers for the early detection of CRC.Citation40,Citation41 Despite the considerable variability in methylation associated with CRC, we decided to screen our data for potential markers that discriminate well between CRC and healthy tissue. We applied filtering criteria to our list of the 15,667 DM CpG sites found in the site-level group differences test to select candidate CpG sites with large and replicable differences in methylation levels. We selected CpG sites according to the following criteria: (1) sites that were hypermethylated in cancer samples and the β-difference between tumors and adjacent tissues (N1) were greater than 0.4; (2) sites showing no significant methylation differences between N1 and N2; (3) sites with Information Gain = 1 in which no methylation level overlapped between CRCs and healthy tissue samples (i.e., the minimal β-values of the hypermethylated sites in CRC were greater than the maximum β-values for the same sites in the normal tissue, and vice versa); (4) sites with a mean methylation level in healthy tissue less than 0.25; and (5) sites with support values greater than 10.
After filtering, we selected a list of 14 CpG sites that matched these criteria: cg19283840, cg01588438, cg18065361, cg16306898, cg08090772, cg15487867, cg06319475, cg09383816, cg09296001, cg26256223, cg07990546, cg25480336, cg16993043, and cg27546237. These mapped to 8 known genes: ADHFE1, C1orf70, SND1, OPLAH, TLX2, ZFP64, NR5A2, and COL4A. Box plots showing the distribution of β-values of selected CpG sites are shown in Figure S9A. The methylation values of the selected CpG sites were used to develop a diagnostic model based on classifying CRC and healthy tissue. An Information Gain estimation, creation and validation model were performed in R software, as described in the Methods.
Next, we analyzed selected CpG sites and evaluated our model on a publicly available external methylation data set of 209 colon adenocarcinoma and 38 normal colon samples from The Cancer Genome Atlas (TCGA, http://tcga.cancer.gov/). The methylation status of these samples was determined using the same version of the 450K methylation array. CRC-associated hypermethylation without overlapping methylation values in the corresponding N1 samples was observed at all 14 selected CpG sites (Fig. S9B). The specificity and sensitivity of the methylation levels were evaluated using receiver-operator curve (ROC) analysis. The methylation levels at all CpG sites significantly distinguished the CRCs from the controls (p < 0.05; ). The highest discriminative accuracy was shown by cg09296001, located in SND1 (AUROC = 1, CI 1–1); other candidate markers also achieved particularly high diagnostic accuracy (AUROC > 0.8, p < 2.2×10−16; ; Fig. S10). We also compared the diagnostic accuracy of individual CpG markers and the combined multi-locus methylation panel based on a multiple logistic regression of all 14 selected CpG sites. However, the use of the multi-locus methylation panel did not improve the discrimination of CRCs from healthy colon tissue relative to the best-performing single locus markers (AUROC = 0.981; 95% CI: 0.9677–0.9939; 100% sensitivity and 82% specificity). Finally, we tested the clusterization of all pathological and normal samples using the PCA of the reduced set of CpG sites, consisting of only 14 selected markers (Fig. S11). The PCA analysis results showed a clear separation of pathological and normal samples into two independent clusters, which also confirms the discriminating ability of selected CpG sites.
Table 3. The most informative CpG sites selected as potential biomarkers
Discussion
Since the first epigenetic alteration in CRC was described nearly three decades ago by A.P. Feinberg and B. Vogelstein, colorectal cancer remains one of the most studied models in the field of DNA methylation research.Citation42 In fact, due to its large methylation variability, CRC is a suitable first choice for benchmarking numerous experimental methods developed for genome-wide DNA methylation mapping. Although CRC methylation studies use a variety of genome-scale sequencing approaches, the application of methylation array technologies was limited, until recently, by relatively small genome coverage. To evaluate the diversity of methylation in CRC at the genome-scale level, we used a new version of the lllumina HumanMethylation450 array to analyze DNA methylation profiles in 22 sample pairs of CRC tumors and adjacent tissues and in 19 colon tissue samples obtained from healthy donors. These two variants of healthy samples allowed us to eliminate possible inter-individual variation and test various statistical approaches for analyzing methylation data.
First, we showed that irrespective of the data processing methods used, the methylation profiles of tumors and healthy tissue samples could be clearly distinguished from one another. Although a growing knowledge base exists regarding tissue- and cancer-specific DNA methylation, we still have little information concerning person-specific DNA methylation and its possible impact on correctly assessing the pathological status of the patient. Several recent reports that focused on inter-individual variability did not provide a sufficiently unambiguous answer on this issue, mainly due to the inclusion of healthy subjects in the studies.Citation43-Citation46 In addition, inter-individual variability in cancer-specific DNA methylation experiments could be an artifact related to the field cancerization effect. Our results showed that DNA methylation patterns were largely conserved across normal colon tissues from CRC and healthy subjects; although we have identified a number of genes potentially involved in the field effect, their total impact on methylation in normal tissue is rather low. The involvement of several of these genes in field cancerization has been previously shown, although the involvement of other such genes is the subject of further research. Even minor methylation variability should be considered for robustness and accuracy in the course of cancer biomarker optimization; during this process, it is important to select CpG sites that demonstrate small amounts of inter-individual variation but strong variation between the cancer and control groups.
Because no universally accepted methodology exists for analyzing Infinium methylation arrays, we used different statistical approaches to evaluate the methylation data. The IMA-R software included several tests based on a general linear model and could be used to infer methylation changes associated with a continuous covariate.Citation12 Alternatively, the paired test was used to identify CpG sites that were differentially methylated between CRC tumors and adjacent healthy colon tissue. There is considerable overlap between the results obtained using either method. The paired test is better at assessing the diversity of methylation at the individual “cancer-normal” samples level, while the IMA-R test is better suited to comparing the average methylation values between clinical groups and can efficiently identify common, group-specific methylation patterns. The redundancy of the paired test can be overcome though the use of “support,” an additional variable calculated based on the number of sample pairs with common methylated CpG sites, as proposed by Simmer et al.Citation26 An extra data-filtering step with a support value of 10 after the paired test gives results comparable to those obtained using a site-level IMA test.
In general, at the CpG site level, we found that common CRC-specific methylation patterns consisting of at least 15,667 CpG sites were significantly different from those of the healthy tissue samples. Most of the common CpG sites in the CRC tumor tissues were hypermethylated (10,342, 66%) rather than hypomethylated (5,325, 34%), which is in agreement with the proportions observed in previous CRC genome-scale methylation studies.Citation4,Citation8,Citation17-Citation19,Citation26,Citation47 Many of the differentially methylated CpG sites in our data set (34.8%) are located in the CpG islands or, if displaying their positions in corresponding genes, in the 1st exon (21.0%) and 5′UTR (15.9%) regions. CpG sites in the CpG islands, the 5′UTR and the 1st exon regions were more likely to be hypermethylated than sites outside the CpG islands (). These data are consistent with the findings from recently published array-based CRC methylation studies,Citation26,Citation47 although initially we expected to see a substantial portion of the methylation variations in CpG shores, as was shown by Irizarry et al.Citation8 It is interesting, however, that the CpG sites common to the maximum number of “cancer-normal” pairs identified using a stringent paired test (support > 10) were similar in that they were mainly hypermethylated. Furthermore, hypermethylated CpG sites are better enriched for known DMRs annotated on the array. Gene Ontology and pathway analysis showed a significant enrichment of the genes containing hypermethylated CpG sites that were related to transcription factor activities and to developmental and cell differentiation processes and that were involved in the Wnt and Cadherin signaling pathways. These facts suggest that DNA hypermethylation is a common feature of CRC and that at least some of the top ranking high-confidence hypermethylated CpG sites most likely have a pathogenic role in the formation of cancer (i.e., are driving epimutations). In contrast, the hypomethylated CpG sites preferentially localize in open seas and show great diversity between samples, and their representation among the set of CpG sites common to CRC is small, which suggests that they are merely a consequence of pathological processes. The absence of matching expression analysis in our study did not allow us to further clarify these assumptions, although the analysis of published data shows a rather low connection between aberrant DNA methylation and gene expression in CRC.Citation47,Citation48
The use of a new, high-content methylation array allowed us to identify a considerably larger number of differentially methylated regions in CRC than was previously possible. Some of the most significant and robust of these sites could be considered candidates for methylation-based CRC diagnostics. In summary, all CpG sites with significant differential methylation after filtering for technical artifacts (related to probing for cross-hybridization in repetitive DNA regions and SNP-containing probes) mapped to 3,152 genes. Of these genes, 60% (1,910) were previously reported to have altered DNA methylation patterns in CRC, and recently published data from the Cancer Genome Atlas project identified an additional 654 genes that are associated with CRC tumor aggression.Citation4 A large fraction of the differentially methylated genes that we identified (1,242) are not known to be CRC-related. Even considering known issues related to statistical significance and data representation at the gene name level, quite a large number of genes that were not previously associated with CRC were identified. These genes include several top ranking, high-confidence genes with large differences in β-values: SND1, OPLAH, C1orf70 (TMEM240), C9orf50, MIR124–3, ZFP64, ZNF829, and DPY19L2, whose functional role in CRC is the subject of further research. Three of these, SND1, OPLAH, and ZFP64, are included in the prototype of the diagnostic panel. All 14 CpG sites included in this group were additionally verified using different statistical approaches, confirming that their identification was not coincidental. Simultaneously, their cross-validation against an external methylation data set from TCGA shows the reliability and reproducibility of the methylation differences identified in the array and confirms the high discriminative potential of the selected markers.
There is little information on the possible role of the selected markers in CRC pathogenesis. The highest discriminative accuracy was shown by the cg09296001 CpG site located in SND1. SND1 encodes the staphylococcal nuclease domain-containing protein, which is a multifunctional protein that modulates transcription, mRNA (mRNA)-splicing, RNA interference (RNAi) function, and mRNA stability.Citation49 The upregulation of SND1 has been detected in numerous human tumors, including breast cancer, prostate cancer, hepatocellular carcinoma and colon cancer.Citation50,Citation51 The high impact of SND1 co-expression with cytoplasmic metadherin (MTDH) as a poor prognostic predictor in colorectal tumor was shown in a recent study by Wang et al.Citation52 However, the possible epigenetic mechanisms by which SND1 expression is regulated remain unknown. Our results showed that most of the differentially methylated CpG sites in SND1, including selected marker cg09296001, were located in the gene body. In contrast, methylation in the promoter region was low, as in CRC and in normal samples, and does not contradict with gene overexpression.
Five CpG sites in our selected panel were found that are located in the TSS200 region of ADHFE1, a gene that encodes iron-containing alcohol dehydrogenase, an enzyme responsible for the oxidation of 4-hydroxybutyrate in mammals.Citation53 The hypermethylation of the ADHFE1 promoter in colorectal cancer has recently been demonstrated in studies using the HumanMethylation 27 array.Citation18,Citation19 An inverse correlation between methylation and gene expression was observed for ADHFE1 in the work of B. Oster et al.,Citation19 while hypermethylation in cancer samples was confirmed by pyrosequencing in the study of Kim et al.Citation18 Despite these studies, the functional role of ADHFE1 in CRC pathogenesis remains unclear.
For the genes OPLAH, TMEM240, TLX2, NR5A2, COL4A1, and ZFP64, even less data regarding methylation and colorectal cancer are available. OPLAH encodes 5-oxoprolinase, an enzyme responsible for glutathione synthesis and degradation; OPLAH 3′region hypermethylation has been reported as example of a shared feature in some tumors.Citation54TMEM240 encodes the transmembrane protein 240 gene and was found to have seven differentially methylated CpG sites in our data set. Of these, cg15487867 has been recently described as a significant site for distinguishing hepatocellular carcinoma.Citation55TLX2 encodes a crucial factor for enteric nervous system development, and it has been shown that TLX2 loss-of-function may play a role in the tumorigenesis of gastrointestinal stromal tumors.Citation56 The hypermethylation of COL4A1 in CRC samples was reported by Kibriya et al., but the functional role of methylation remains unclear.Citation17ZFP64 (Zinc finger protein 64 homolog) was confirmed by proteomic analysis to be upregulated in liver metastases of colorectal carcinoma.Citation57NR5A2, also known as LRH-1, belongs to the nuclear receptor subfamily 5 and is associated with intestinal tumorigenesis in mouse models.Citation58 Finally, nucleotide polymorphisms near NR5A2 have been shown to be associated with pancreatic cancer in a genome-wide association study, suggesting a role for NR5A2 in tumorigenesis.Citation59 While the functional significance of methylation in selected genes has not been studied, most of these genes are associated with different types of cancer to some extent.
In terms of clinical importance, epigenetic alterations are considered to be promising markers for the early detection, diagnosis, prognosis and management of patients with cancer. With respect to the use of methylated CpGs as biomarkers specifically for CRC, the most advanced uses are as DNAbased non-invasive CRC screening assays. Hypermethylation of promoter regions in CRC occurs early in some genes, and these regions are promising candidates for early detection markers. These markers can be used with biopsy samples obtained during endoscopy. Tumor-specific DNA could be present in the bloodstream, and cancer cells and DNA could be present in the feces, both of which present opportunities for the development of non-invasive tests. The first commercially available non-invasive tests for CRC diagnosis that are based on the analysis of methylation in VIM and SEPT9 genes and are already being used.Citation40,Citation41
With respect to the our set of candidate markers, further validation studies of an independent cohort using alternative techniques of testing for selected markers in different sample types (such as stool- and plasma-based variants) are needed to evaluate the frequency and possible clinical value of hypermethylation in CRC. Despite these limitations, we were able to use these methylated sites to successfully distinguish CRC tissues from healthy tissues using the large external TCGA methylation data set with only minor misclassifications (AUROC > 0.88). This result suggests a strong diagnostic potential for the tested markers, and we hope that they are potentially applicable in improving early CRC diagnosis. Our use of the TCGA data set allowed us to enhance and compare our methylation marker discovery protocol to known CRC risk factors. Some well-known genes, including SEPT9, HLTF, and VIM, have been widely discussed in the context of CRC diagnostics but were not found to be good markers in our study. These genes showed rather modest, though significant, differences in methylation (Δβ 0.2–0.3), but were not included in the diagnostic set due to overlapping methylation values between CRCs and healthy tissue (information gain < 1).
Our study possesses some limitations due to the cohort size and insufficiency of some clinical characteristics. We found no significant associations between methylation and tumor stage, sex and age, except for a trend in the differences associated with the histological grade of tumors. Because most of the tumors used in our study were derived from a prospective collection, survival data are not available. We believe, however, that epigenetically related molecular heterogeneity in CRC may serve both as a source for early tumor marker selection and as a factor responsible for the substantial variability in treatment response among patients; it could thus be used as a tool for assessing tumor aggressiveness.
An additional feature of our study consists of analyzing rectal carcinoma tumor samples. Epidemiologically and therapeutically, colon and rectal tumors are considered to be different.Citation60 However, recently published data from the Cancer Genome Atlas project indicate that the overall patterns of changes in methylation, mRNA and miRNA are indistinguishable between colon and rectal carcinomas.Citation4 Thus, the methylation data obtained from the clinical samples studied in the present work may be adequate for use in comparative analyses in other CRC methylation studies. All methylation data discussed above have been deposited in the NCBI Gene Expression Omnibus and will be accessible through GEO Series accession number GSE42752 (http://www.ncbi.nlm.nih.gov/geo/query/acc.cgi?acc=GSE42752).
Most of the studies on CRC methylation published to date were performed using either the previous version of Illumina HumanMethylation array (27K) or enrichment-based DNA sequencing approaches. The 27K array (comprising 27,578 CpG sites) provides information on a small part of the entire genome and mainly covers promoter regions. Although enrichment-based DNA sequencing methods are very powerful tools, they have low statistical power in CpG-poor genomic regions and relatively low resolution. As the first 450K Human Methylation array validation study to focus on CRC was performed mainly on cell lines and several normal colon mucosa samples,Citation61 our work is (to our knowledge) one of the first 450K array studies aimed at understanding genome-wide methylation in CRC, healthy colon samples from the same patient and healthy colon samples from cancer-free patients.
In summary, the use of the new, cost-effective high-content 450K microarray has allowed us to successfully apply an unbiased approach to the discovery of novel methylation markers that are associated with colorectal cancer. We have also been able to adequately assess the methylation diversity in CRC at the genome-scale level. Robust data obtained from the current study may be valuable in improving our understanding of the role of aberrant methylation and other molecular mechanisms in CRC pathogenesis. We believe that collaborative efforts to investigate these molecular mechanisms will allow epigenetically based approaches to be commonly used to guide CRC prevention and treatment in the near future.
Methods
Sample collection
Fresh frozen cancer tissue samples (C) were obtained from surgically removed colonic specimens. Tumor samples were macrodissected to ensure the purity of the tumor. From the same patient, adjacent normal mucosa tissue samples (N1) were collected from resected, unaffected parts of the colon located approximately 5–10 cm away from the tumor site. All samples were collected from the operating room immediately after surgical resection (C, N1) or after colonoscopy (N2). Samples were fresh frozen and were shipped on ice for subsequent DNA extraction and methylation assays.
DNA sample preparation, bisulfite conversion and methylation level measurement
DNA was extracted from frozen tissue samples using the Promega Wizard® Genomic DNA Purification Kit, as described by the manufacturer. DNA quantification was performed using a Qubit® 2.0 Fluorometer (Invitrogen). The bisulfite conversion of DNA was conducted using the Zymo bisulfite gold kit. The Infinium Methylation 450K assay was performed according to Illumina’s standard protocol. Processed methylation chips were scanned using an iScan reader (Illumina). Paired samples (CRC and corresponding healthy tissues) were processed on the same chip, and all samples were processed at the same time to avoid chip-to-chip variation.
Data quality control and preprocessing
Infinium Methylation data were processed using the Methylation Module of the GenomeStudio software package (v. 2011.1). For quality control, methylation measures with a detection p-value > 0.05 and samples with a CpG coverage < 95% were removed. Ultimately all 63 samples passed the coverage criteria. The data were initially normalized using internal controls in the GenomeStudio software. The methylation levels of CpG sites were calculated as β-values (β = Intensity (methylated)/intensity (methylated + unmethylated). The data were further normalized using a peak correction algorithm embedded in the IMA-R package.Citation12 Finally, to avoid sex-specific methylation bias, CpG sites on the sex chromosomes were removed, leaving 444,888 autosomal CpG sites to be used in further analyses.
Differential methylation analysis
Multivariate ANOVA analysis was performed using Partek Genomic Suite software (v 6.6). The averaged methylation values were compared between clinical groups at the CpG site level using a general linear model test implemented in the IMA-R package. The following criteria were used: β-difference > 0.2 and a false discovery rate corrected p-value < 0.05. These same criteria were used to calculate the methylation difference among the region-level variants identified in the IMA-R test. The methylation index of gene- and CpG island-based regions was calculated in IMA-R, and region-specific β-values were median-averaged. To identify the methylation difference in paired samples, we calculated a β-values matrix in which pathological β-values were subtracted from normal β-values for each of the 22 “cancer-normal” sample pairs with absolute β-difference values greater than 0.2. “Support” was calculated as the number of tumors that had a statistically significant difference in methylation status with the matched healthy tissues and was determined using the binomial distribution (Fig. S12). After modeling the probability density according to binomial distribution (n = 22, p = 0.045) and applying a Bonferroni correction for multiple comparisons, we established a support value of 10 as being statistically significant.
Functional annotations of differentially methylated CpG sites
An enrichment analysis of the hypermethylated regions for the Gene Ontology (GO), Panther Pathway and KEGG Pathway databases was conducted using the GREAT web service.Citation24 Bed files for the analyzed regions were compiled based on the hg19 coordinates from the 450K array manifest file using the Basal+extension associations rule (constitutive 1.0 kb upstream, 1.0 kb downstream and up to 1.0 kb max extension). These files were used to map CpG sites to their nearest genes. Binomial statistics with FDR correction was used to find significantly enriched ontologies.
Diagnostic markers selection and model evaluation
The most significant and replicable CpG sites were selected from the results of the site-level differential methylation test to be candidate markers for CRC detection. These sites were consecutively selected from C vs. N1 according to the following criteria: (∆βC−N1≥ 0.4 ∆βN1−N2≤ 0.1; IG = 1; MeanN1 ≤ 0.25; Supp > 10), where C, N1 and N2 are the average methylation β-values for a given CpG site in tumors, matched healthy tissues and tissues samples from healthy donors, respectively; IG, Information Gain; Mean, mean methylation level; Supp, support value, calculated as described above. Logistic regression model training and validation of Cancer Genome Atlas data was performed with R statistics using the pROC package. Estimated ROC curves were compared using DeLong’s test.Citation62 Each model was validated on separate a data set from TCGA colon adenocarcinoma and normal colon methylation data (https://tcga-data.nci.nih.gov/tcgafiles/ftp_auth/distro_ftpusers/anonymous/tumor/coad/cgcc/jhu-usc.edu/humanmethylation450/methylation/).
Data access
The data generated for this work have been deposited in the NCBI Gene Expression Omnibus (GEO) (www.ncbi.nlm.nih.gov/geo) and are accessible through GEO Series accession number GSE42752.
Abbreviations: | ||
CRC | = | colorectal cancer |
DMR | = | differentially methylated region |
DM CpG | = | differentially methylated CpG site |
FDR | = | false discovery rate |
PCA | = | principal component analysis |
CIMP | = | CpG island methylator phenotype |
TSS | = | transcription start site |
Additional material
Download Zip (3.7 MB)Acknowledgments
We thank ES Kostryukova and EN Ilina for technical support.
Disclosure of Potential Conflicts of Interest
No potential conflicts of interest were disclosed.
Supplemental Materials
Supplemental materials may be found here: http://www.landesbioscience.com/journals/epigenetics/article/25577
References
- Avksentyeva M. Colorectal cancer in Russia. Eur J Health Econ 2010; 10:Suppl 1 S91 - 8; http://dx.doi.org/10.1007/s10198-009-0195-9; PMID: 20012132
- Cancer Genome Atlas Research Network. Comprehensive genomic characterization defines human glioblastoma genes and core pathways. Nature 2008; 455:1061 - 8; http://dx.doi.org/10.1038/nature07385; PMID: 18772890
- Cancer Genome Atlas Research Network. Integrated genomic analyses of ovarian carcinoma. Nature 2011; 474:609 - 15; http://dx.doi.org/10.1038/nature10166; PMID: 21720365
- Cancer Genome Atlas Network. Comprehensive molecular characterization of human colon and rectal cancer. Nature 2012; 487:330 - 7; http://dx.doi.org/10.1038/nature11252; PMID: 22810696
- Fearon ER. Molecular genetics of colorectal cancer. Annu Rev Pathol 2011; 6:479 - 507; http://dx.doi.org/10.1146/annurev-pathol-011110-130235; PMID: 21090969
- Pfeifer GP, Rauch TA. DNA methylation patterns in lung carcinomas. Semin Cancer Biol 2009; 19:181 - 7; http://dx.doi.org/10.1016/j.semcancer.2009.02.008; PMID: 19429482
- Costello JF, Frühwald MC, Smiraglia DJ, Rush LJ, Robertson GP, Gao X, et al. Aberrant CpG-island methylation has non-random and tumour-type-specific patterns. Nat Genet 2000; 24:132 - 8; http://dx.doi.org/10.1038/72785; PMID: 10655057
- Irizarry RA, Ladd-Acosta C, Wen B, Wu Z, Montano C, Onyango P, et al. The human colon cancer methylome shows similar hypo- and hypermethylation at conserved tissue-specific CpG island shores. Nat Genet 2009; 41:178 - 86; http://dx.doi.org/10.1038/ng.298; PMID: 19151715
- Bibikova M, Barnes B, Tsan C, Ho V, Klotzle B, Le JM, et al. High density DNA methylation array with single CpG site resolution. Genomics 2011; 98:288 - 95; http://dx.doi.org/10.1016/j.ygeno.2011.07.007; PMID: 21839163
- Dedeurwaerder S, Defrance M, Calonne E, Denis H, Sotiriou C, Fuks F. Evaluation of the Infinium Methylation 450K technology. Epigenomics 2011; 3:771 - 84; http://dx.doi.org/10.2217/epi.11.105; PMID: 22126295
- Touleimat N, Tost J. Complete pipeline for Infinium(®) Human Methylation 450K BeadChip data processing using subset quantile normalization for accurate DNA methylation estimation. Epigenomics 2012; 4:325 - 41; http://dx.doi.org/10.2217/epi.12.21; PMID: 22690668
- Wang D, Yan L, Hu Q, Sucheston LE, Higgins MJ, Ambrosone CB, et al. IMA: an R package for high-throughput analysis of Illumina’s 450K Infinium methylation data. Bioinformatics 2012; 28:729 - 30; http://dx.doi.org/10.1093/bioinformatics/bts013; PMID: 22253290
- Belshaw NJ, Elliott GO, Foxall RJ, Dainty JR, Pal N, Coupe A, et al. Profiling CpG island field methylation in both morphologically normal and neoplastic human colonic mucosa. Br J Cancer 2008; 99:136 - 42; http://dx.doi.org/10.1038/sj.bjc.6604432; PMID: 18542073
- Nosho K, Kawasaki T, Ohnishi M, Suemoto Y, Kirkner GJ, Zepf D, et al. PIK3CA mutation in colorectal cancer: relationship with genetic and epigenetic alterations. Neoplasia 2008; 10:534 - 41; PMID: 18516290
- Shen L, Kondo Y, Rosner GL, Xiao L, Hernandez NS, Vilaythong J, et al. MGMT promoter methylation and field defect in sporadic colorectal cancer. J Natl Cancer Inst 2005; 97:1330 - 8; http://dx.doi.org/10.1093/jnci/dji275; PMID: 16174854
- Svrcek M, Buhard O, Colas C, Coulet F, Dumont S, Massaoudi I, et al. Methylation tolerance due to an O6-methylguanine DNA methyltransferase (MGMT) field defect in the colonic mucosa: an initiating step in the development of mismatch repair-deficient colorectal cancers. Gut 2010; 59:1516 - 26; http://dx.doi.org/10.1136/gut.2009.194787; PMID: 20947886
- Kibriya MG, Raza M, Jasmine F, Roy S, Paul-Brutus R, Rahaman R, et al. A genome-wide DNA methylation study in colorectal carcinoma. BMC Med Genomics 2011; 4:50; http://dx.doi.org/10.1186/1755-8794-4-50; PMID: 21699707
- Kim YH, Lee HC, Kim SY, Yeom YI, Ryu KJ, Min BH, et al. Epigenomic analysis of aberrantly methylated genes in colorectal cancer identifies genes commonly affected by epigenetic alterations. Ann Surg Oncol 2011; 18:2338 - 47; http://dx.doi.org/10.1245/s10434-011-1573-y; PMID: 21298349
- Oster B, Thorsen K, Lamy P, Wojdacz TK, Hansen LL, Birkenkamp-Demtröder K, et al. Identification and validation of highly frequent CpG island hypermethylation in colorectal adenomas and carcinomas. Int J Cancer 2011; 129:2855 - 66; http://dx.doi.org/10.1002/ijc.25951; PMID: 21400501
- Ausch C, Kim YH, Tsuchiya KD, Dzieciatkowski S, Washington MK, Paraskeva C, et al. Comparative analysis of PCR-based biomarker assay methods for colorectal polyp detection from fecal DNA. Clin Chem 2009; 55:1559 - 63; http://dx.doi.org/10.1373/clinchem.2008.122937; PMID: 19541867
- Issa JP, Vertino PM, Boehm CD, Newsham IF, Baylin SB. Switch from monoallelic to biallelic human IGF2 promoter methylation during aging and carcinogenesis. Proc Natl Acad Sci U S A 1996; 93:11757 - 62; http://dx.doi.org/10.1073/pnas.93.21.11757; PMID: 8876210
- Jubb AM, Quirke P, Oates AJ. DNA methylation, a biomarker for colorectal cancer: implications for screening and pathological utility. Ann N Y Acad Sci 2003; 983:251 - 67; http://dx.doi.org/10.1111/j.1749-6632.2003.tb05980.x; PMID: 12724230
- Lofton-Day C, Model F, Devos T, Tetzner R, Distler J, Schuster M, et al. DNA methylation biomarkers for blood-based colorectal cancer screening. Clin Chem 2008; 54:414 - 23; http://dx.doi.org/10.1373/clinchem.2007.095992; PMID: 18089654
- McLean CY, Bristor D, Hiller M, Clarke SL, Schaar BT, Lowe CB, et al. GREAT improves functional interpretation of cis-regulatory regions. Nat Biotechnol 2010; 28:495 - 501; http://dx.doi.org/10.1038/nbt.1630; PMID: 20436461
- Dexter D, Prodduturi N, Zhang B. WebGestalt2: an updated and expanded version of the Web-based Gene Set Analysis Toolkit. BMC Bioinformatics 2010; 11:1 - 10; PMID: 20043860
- Simmer F, Brinkman AB, Assenov Y, Matarese F, Kaan A, Sabatino L, et al. Comparative genome-wide DNA methylation analysis of colorectal tumor and matched normal tissues. Epigenetics 2012; 7:1355 - 67; http://dx.doi.org/10.4161/epi.22562; PMID: 23079744
- Lascorz J, Hemminki K, Försti A. Systematic enrichment analysis of gene expression profiling studies identifies consensus pathways implicated in colorectal cancer development. J Carcinog 2011; 10:7; http://dx.doi.org/10.4103/1477-3163.78268; PMID: 21483658
- Kongkham PN, Northcott PA, Croul SE, Smith CA, Taylor MD, Rutka JT. The SFRP family of WNT inhibitors function as novel tumor suppressor genes epigenetically silenced in medulloblastoma. Oncogene 2010; 29:3017 - 24; http://dx.doi.org/10.1038/onc.2010.32; PMID: 20208569
- Vincent A, Omura N, Hong SM, Jaffe A, Eshleman J, Goggins M. Genome-wide analysis of promoter methylation associated with gene expression profile in pancreatic adenocarcinoma. Clin Cancer Res 2011; 17:4341 - 54; http://dx.doi.org/10.1158/1078-0432.CCR-10-3431; PMID: 21610144
- Suzuki H, Gabrielson E, Chen W, Anbazhagan R, van Engeland M, Weijenberg MP, et al. A genomic screen for genes upregulated by demethylation and histone deacetylase inhibition in human colorectal cancer. Nat Genet 2002; 31:141 - 9; http://dx.doi.org/10.1038/ng892; PMID: 11992124
- Wheeler JM, Kim HC, Efstathiou JA, Ilyas M, Mortensen NJ, Bodmer WF. Hypermethylation of the promoter region of the E-cadherin gene (CDH1) in sporadic and ulcerative colitis associated colorectal cancer. Gut 2001; 48:367 - 71; http://dx.doi.org/10.1136/gut.48.3.367; PMID: 11171827
- Nanjo S, Asada K, Yamashita S, Nakajima T, Nakazawa K, Maekita T, et al. Identification of gastric cancer risk markers that are informative in individuals with past H. pylori infection. Gastric Cancer 2012; 15:382 - 8; http://dx.doi.org/10.1007/s10120-011-0126-1; PMID: 22237657
- Toyota M, Ahuja N, Ohe-Toyota M, Herman JG, Baylin SB, Issa JP. CpG island methylator phenotype in colorectal cancer. Proc Natl Acad Sci U S A 1999; 96:8681 - 6; http://dx.doi.org/10.1073/pnas.96.15.8681; PMID: 10411935
- Weisenberger DJ, Siegmund KD, Campan M, Young J, Long TI, Faasse MA, et al. CpG island methylator phenotype underlies sporadic microsatellite instability and is tightly associated with BRAF mutation in colorectal cancer. Nat Genet 2006; 38:787 - 93; http://dx.doi.org/10.1038/ng1834; PMID: 16804544
- Curtin K, Slattery ML, Samowitz WS. CpG island methylation in colorectal cancer: past, present and future. Patholog Res Int 2011; 2011:902674; PMID: 21559209
- Slattery ML, Wolff RK, Curtin K, Fitzpatrick F, Herrick J, Potter JD, et al. Colon tumor mutations and epigenetic changes associated with genetic polymorphism: insight into disease pathways. Mutat Res 2009; 660:12 - 21; http://dx.doi.org/10.1016/j.mrfmmm.2008.10.001; PMID: 18992263
- Barault L, Charon-Barra C, Jooste V, de la Vega MF, Martin L, Roignot P, et al. Hypermethylator phenotype in sporadic colon cancer: study on a population-based series of 582 cases. Cancer Res 2008; 68:8541 - 6; http://dx.doi.org/10.1158/0008-5472.CAN-08-1171; PMID: 18922929
- Shen L, Toyota M, Kondo Y, Lin E, Zhang L, Guo Y, et al. Integrated genetic and epigenetic analysis identifies three different subclasses of colon cancer. Proc Natl Acad Sci U S A 2007; 104:18654 - 9; http://dx.doi.org/10.1073/pnas.0704652104; PMID: 18003927
- Mikeska T, Bock C, Do H, Dobrovic A. DNA methylation biomarkers in cancer: progress towards clinical implementation. Expert Rev Mol Diagn 2012; 12:473 - 87; http://dx.doi.org/10.1586/erm.12.45; PMID: 22702364
- Chen WD, Han ZJ, Skoletsky J, Olson J, Sah J, Myeroff L, et al. Detection in fecal DNA of colon cancer-specific methylation of the nonexpressed vimentin gene. J Natl Cancer Inst 2005; 97:1124 - 32; http://dx.doi.org/10.1093/jnci/dji204; PMID: 16077070
- Tóth K, Sipos F, Kalmár A, Patai AV, Wichmann B, Stoehr R, et al. Detection of methylated SEPT9 in plasma is a reliable screening method for both left- and right-sided colon cancers. PLoS One 2012; 7:e46000; http://dx.doi.org/10.1371/journal.pone.0046000; PMID: 23049919
- Feinberg AP, Vogelstein B. Hypomethylation distinguishes genes of some human cancers from their normal counterparts. Nature 1983; 301:89 - 92; http://dx.doi.org/10.1038/301089a0; PMID: 6185846
- Bock C, Walter J, Paulsen M, Lengauer T. Inter-individual variation of DNA methylation and its implications for large-scale epigenome mapping. Nucleic Acids Res 2008; 36:e55; http://dx.doi.org/10.1093/nar/gkn122; PMID: 18413340
- Byun HM, Siegmund KD, Pan F, Weisenberger DJ, Kanel G, Laird PW, et al. Epigenetic profiling of somatic tissues from human autopsy specimens identifies tissue- and individual-specific DNA methylation patterns. Hum Mol Genet 2009; 18:4808 - 17; http://dx.doi.org/10.1093/hmg/ddp445; PMID: 19776032
- Gervin K, Hammerø M, Akselsen HE, Moe R, Nygård H, Brandt I, et al. Extensive variation and low heritability of DNA methylation identified in a twin study. Genome Res 2011; 21:1813 - 21; http://dx.doi.org/10.1101/gr.119685.110; PMID: 21948560
- Krausz C, Sandoval J, Sayols S, Chianese C, Giachini C, Heyn H, et al. Novel insights into DNA methylation features in spermatozoa: stability and peculiarities. PLoS One 2012; 7:e44479; http://dx.doi.org/10.1371/journal.pone.0044479; PMID: 23071498
- Hinoue T, Weisenberger DJ, Lange CP, Shen H, Byun HM, Van Den Berg D, et al. Genome-scale analysis of aberrant DNA methylation in colorectal cancer. Genome Res 2012; 22:271 - 82; http://dx.doi.org/10.1101/gr.117523.110; PMID: 21659424
- Sproul D, Nestor C, Culley J, Dickson JH, Dixon JM, Harrison DJ, et al. Transcriptionally repressed genes become aberrantly methylated and distinguish tumors of different lineages in breast cancer. Proc Natl Acad Sci U S A 2011; 108:4364 - 9; http://dx.doi.org/10.1073/pnas.1013224108; PMID: 21368160
- Ying Z, Li J, Li M. Astrocyte elevated gene 1: biological functions and molecular mechanism in cancer and beyond. Cell Biosci 2011; 1:36; http://dx.doi.org/10.1186/2045-3701-1-36; PMID: 22060137
- Tsuchiya N, Nakagama H. MicroRNA, SND1, and alterations in translational regulation in colon carcinogenesis. Mutat Res 2010; 693:94 - 100; http://dx.doi.org/10.1016/j.mrfmmm.2010.09.001; PMID: 20883704
- Kuruma H, Kamata Y, Takahashi H, Igarashi K, Kimura T, Miki K, et al. Staphylococcal nuclease domain-containing protein 1 as a potential tissue marker for prostate cancer. Am J Pathol 2009; 174:2044 - 50; http://dx.doi.org/10.2353/ajpath.2009.080776; PMID: 19435788
- Wang N, Du X, Zang L, Song N, Yang T, Dong R, et al. Prognostic impact of Metadherin-SND1 interaction in colon cancer. Mol Biol Rep 2012; 39:10497 - 504; http://dx.doi.org/10.1007/s11033-012-1933-0; PMID: 23065261
- Kardon T, Noël G, Vertommen D, Schaftingen EV. Identification of the gene encoding hydroxyacid-oxoacid transhydrogenase, an enzyme that metabolizes 4-hydroxybutyrate. FEBS Lett 2006; 580:2347 - 50; http://dx.doi.org/10.1016/j.febslet.2006.02.082; PMID: 16616524
- Xu Y, Hu B, Choi AJ, Gopalan B, Lee BH, Kalady MF, et al. Unique DNA methylome profiles in CpG island methylator phenotype colon cancers. Genome Res 2012; 22:283 - 91; http://dx.doi.org/10.1101/gr.122788.111; PMID: 21990380
- Shen J, Wang S, Zhang YJ, Wu HC, Kibriya MG, Jasmine F, et al. Exploring genome-wide DNA methylation profiles altered in hepatocellular carcinoma using Infinium HumanMethylation 450 BeadChips. Epigenetics 2013; 8:34 - 43; http://dx.doi.org/10.4161/epi.23062; PMID: 23208076
- Kaifi JT, Wagner M, Schurr PG, Wachowiak R, Reichelt U, Yekebas EF, et al. Allelic loss of Hox11L1 gene locus predicts outcome of gastrointestinal stromal tumors. Oncol Rep 2006; 16:915 - 9; PMID: 16969514
- Li SY, An P, Cai HY, Bai X, Zhang YN, Yu B, et al. Proteomic analysis of differentially expressed proteins involving in liver metastasis of human colorectal carcinoma. Hepatobiliary Pancreat Dis Int 2010; 9:149 - 53; PMID: 20382585
- Schoonjans K, Dubuquoy L, Mebis J, Fayard E, Wendling O, Haby C, et al. Liver receptor homolog 1 contributes to intestinal tumor formation through effects on cell cycle and inflammation. Proc Natl Acad Sci U S A 2005; 102:2058 - 62; http://dx.doi.org/10.1073/pnas.0409756102; PMID: 15684064
- Petersen GM, Amundadottir L, Fuchs CS, Kraft P, Stolzenberg-Solomon RZ, Jacobs KB, et al. A genome-wide association study identifies pancreatic cancer susceptibility loci on chromosomes 13q22.1, 1q32.1 and 5p15.33. Nat Genet 2010; 42:224 - 8; http://dx.doi.org/10.1038/ng.522; PMID: 20101243
- Minsky BD. Unique considerations in the patient with rectal cancer. Semin Oncol 2011; 38:542 - 51; http://dx.doi.org/10.1053/j.seminoncol.2011.05.008; PMID: 21810513
- Sandoval J, Heyn H, Moran S, Serra-Musach J, Pujana MA, Bibikova M, et al. Validation of a DNA methylation microarray for 450,000 CpG sites in the human genome. Epigenetics 2011; 6:692 - 702; http://dx.doi.org/10.4161/epi.6.6.16196; PMID: 21593595
- DeLong ER, DeLong DM, Clarke-Pearson DL. Comparing the areas under two or more correlated receiver operating characteristic curves: a nonparametric approach. Biometrics 1988; 44:837 - 45; http://dx.doi.org/10.2307/2531595; PMID: 3203132