Abstract
Offspring exposed to gestational diabetes mellitus (GDM) have an increased risk for chronic diseases, and one promising mechanism for fetal metabolic programming is epigenetics. Therefore, we postulated that GDM exposure impacts the offspring’s methylome and used an epigenomic approach to explore this hypothesis. Placenta and cord blood samples were obtained from 44 newborns, including 30 exposed to GDM. Women were recruited at first trimester of pregnancy and followed until delivery. GDM was assessed after a 75-g oral glucose tolerance test at 24–28 weeks of pregnancy. DNA methylation was measured at > 485,000 CpG sites (Infinium HumanMethylation450 BeadChips). Ingenuity Pathway Analysis was conducted to identify metabolic pathways epigenetically affected by GDM. Our results showed that 3,271 and 3,758 genes in placenta and cord blood, respectively, were potentially differentially methylated between samples exposed or not to GDM (p-values down to 1 × 10−06; none reached the genome-wide significance levels), with more than 25% (n = 1,029) being common to both tissues. Mean DNA methylation differences between groups were 5.7 ± 3.2% and 3.4 ± 1.9% for placenta and cord blood, respectively. These genes were likely involved in the metabolic diseases pathway (up to 115 genes (11%), p-values for pathways = 1.9 × 10−13 < p < 4.0 × 10−03; including diabetes mellitus p = 4.3 × 10−11). Among the differentially methylated genes, 326 in placenta and 117 in cord blood were also associated with newborn weight. Our results therefore suggest that GDM has epigenetic effects on genes preferentially involved in the metabolic diseases pathway, with consequences on fetal growth and development, and provide supportive evidence that DNA methylation is involved in fetal metabolic programming.
Introduction
In response to the increasing prevalence of obesityCitation1 and excess gestational weight gain,Citation2 women are more likely to develop pregnancy complications such as gestational diabetes mellitus (GDM).Citation3,Citation4 As a consequence, GDM prevalence is increasing along with increasing rates of obesity.Citation5 The prevalence of GDM ranges from 1% to 20%, depending on the population studied and the diagnostic criteria used.Citation6 Epidemiological studies have shown that GDM is associated with several detrimental health consequences among offspring, such as an increased risk of macrosomia or large-for-gestational-age at birthCitation7 and/or developing overweight/obesity later in life,Citation8-Citation10 insulin resistance/type 2 diabetesCitation11,Citation12 and other cardiovascular disease risk factors.Citation13 These studies reporting a relationship between the exposition to an altered in utero condition (here GDM) and the risk of developing metabolic disorders support the fetal programming (Barker’s) and the Developmental Origins of Health and Diseases (DOHaD) hypotheses.Citation14,Citation15 A better understanding of the mechanisms through which GDM exposure translates into the development of these diseases among offspring is urgently needed in order to develop effective prenatal preventive strategies and break the vicious circle that perpetuates obesity and type 2 diabetes across generations.
Accumulating evidence suggests that maternal hyperglycemia and/or resulting fetal hyperinsulinism epigenetically program a predisposition for offspring to develop obesity and type 2 diabetes later in life.Citation16 Epigenetic marks can be subjected to reprogramming in response to both stochastic and environmental stimuli such as the in utero environment.Citation17 In addition, a large number of epigenetic marks are relatively stable over time,Citation18 suggesting that those acquired early in life may have a long-lasting impact on future health. In this respect, we previously showed that maternal hyperglycemia is associated with placental DNA methylation adaptations at the LEPCitation19 and ADIPOQCitation20 genes. Similarly, a recent study reported that DNA methylation levels at the maternally imprinted MEST gene were significantly lower in placenta and cord blood tissues exposed to GDM than in those not exposed to GDM.Citation21 Furthermore, morbidly obese adults had a decreased blood MEST methylation compared with normal-weight adults.Citation21 Taken together, these findings suggest that maternal glucose metabolism dysregulation affects DNA methylation at genes involved in energy and glucose metabolism (LEP and ADIPOQ), and fetal and placental growth, somatic differentiation and neurobiological and behavioral functions (MEST), which may contribute to the development of obesity.
Accordingly, we hypothesized that DNA methylation levels at a number of additional genes were also affected by an exposure to GDM. As proposed by the fetal programming and DOHaD hypotheses, these genes will likely be involved in metabolic pathways related to obesity and type 2 diabetes development. Previous studies have relied on a candidate gene approach to identify genes epigenetically affected by GDM exposure. In the current study, a genome-wide approach was used for the first time to examine DNA methylation profile differences in placenta and cord blood samples exposed or not to GDM. The primary objective was to identify additional genes and their biological pathways showing epigenetic dysregulation in response to GDM. The secondary objective was to examine whether the differentially methylated genes identified were correlated with birth weight, which is an index of fetal growth and development.
Results
Maternal and newborn characteristics of the 44 mother-newborn pairs are shown in . On average, women were slightly overweight at first trimester of pregnancy but were all metabolically healthy. No difference between groups in glucose and lipid profile at first trimester of pregnancy was observed. As expected, the GDM group had higher 2 h post-oral glucose tolerance test (OGTT) glucose levels and, on average, gained less weight during pregnancy than the non-GDM group.
Table 1. Maternal and newborn characteristics
DNA methylation analyses
The statistical analyses were restricted to autosomal epialleles, which represent 231,818 and 117,321 loci in placenta and cord blood respectively (). Placenta samples showed significantly more DNA methylation variability than cord blood samples (χ2 = 58,663, p < 0.0001). The best p-values obtained were 1.61 × 10−06 and 2.0 × 10−05 for placenta and cord blood analyses, respectively (Table S1). Using an intended relaxed criterion in accordance with the study design and the applied converging analytical strategy (p < 0.05), 8,657 loci in the placenta and 7,855 in cord blood were potentially differentially methylated between the GDM and non-GDM groups. These loci were distributed over 3,271 and 3,757 genes, respectively (). The mean DNA methylation difference between the groups was 5.7 ± 3.2% for placenta genes, with 95% of them showing a mean difference ≥ 2% and 75% a mean difference ≥ 3%. Cord blood genes had a mean DNA methylation difference of 3.4 ± 1.9%, with 93% of them showing a mean difference ≥ 2% and 53% a mean difference ≥ 3%. The best hits (best p-values) in the placenta and cord blood were respectively DPP6 (dipeptidyl-peptidase 6) (Δβ-value = 13.0%, p = 1.0 × 10−06) and STC2 (stanniocalcin 2) (Δβ-value = 7.4%, p = 1.0 × 10−05). However, VIPR1 (vasoactive intestinal peptide receptor 1) in placenta (Δβ-value = 5.6%, p = 7.4 ×10–05) and TRIB1 (tribbles homolog 1) in cord blood (Δβ-value = 5.3%, p = 2.0 × 10−05) were identified as the most promising obesity and type 2 diabetes candidate genes among the top 5 differentially methylated genes (Table S1). When comparing placenta and cord blood results, we found that more than 25% (n = 1,029) of the differentially methylated genes (at p < 0.05) affected by GDM were common to both tissues ().
Common disease and disorder pathways affected by GDM
The top genes (p < 0.01) with potential DNA methylation differences between placenta and cord blood samples exposed or not to GDM were selected to conduct pathway analyses. A mean DNA methylation difference of 7.3 ± 3.8% was observed for placenta genes, with 99.5% of them showing a mean difference ≥ 2% and 90% a mean difference ≥ 3%. Cord blood genes had a mean DNA methylation difference of 4.1 ± 2.1%, with 99.5% of them showing a mean difference ≥ 2% and 79% a mean difference ≥ 3%. The three top pathways emerging from Ingenuity pathway analyses (IPA) of the placenta were: (1) cardiovascular diseases (91 genes, p-values for pathway = 3.3 × 10−07 < p < 4.4 × 10−03), (2) metabolic diseases (88 genes, 9.8 × 10−06 < p < 4.37 × 10−03 and (3) psychological disorders (72 genes, 2.5x10−05 < p < 4.2x10−03) ( and ; Table S2). The top pathways identified in the cord blood were: (1) gastro-intestinal diseases (94 genes, 1.1 × 10−07 < p < 4.1 × 10−03), (2) metabolic diseases (70 genes, 1.1 × 10−07 < p < 4.1 × 10−03) and (3) endocrine system disorders (64 genes, 1.1 × 10−07 < p < 4.6 × 10−03) ( and ; Table S2). Finally, when we restricted the analysis to the differentially methylated genes common to both tissues, IPA identified immunological diseases (134 genes, 1.4 × 10−13 < p < 4.4 × 10−03) in addition to the previously identified pathways of metabolic diseases (115 genes, 1.9 × 10−13 < p < 4.00 × 10−03) and endocrine system disorders (104 genes, 1.9 × 10−13 < p < 4.00 × 10−03) ( and ; Table S2).
Figure 2. Ingenuity pathway analysis: top-ranked common disease and disorder pathways that were epigenetically affected by gestational diabetes mellitus. Top-ranked common disease and disorder pathways to which belonged differentially methylated genes in placenta (p < 0.01, n = 781), cord blood (p < 0.01, n = 758) and both tissues (p < 0.05, n = 1029). #, number of genes involved in each pathway.
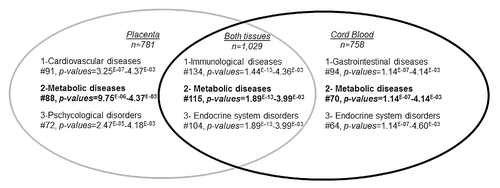
Figure 3. Ingenuity pathway analysis: top-ranked diseases and disorders associated to each of the common disease and disorder pathways epigenetically affected by gestational diabetes mellitus. Light gray bars, Ingenuity Pathway Analysis (IPA) results obtained with differentially methylated genes in placenta (p < 0.01, n = 781); black bars, IPA results obtained with differentially methylated genes in cord blood (p < 0.01, n = 758); gray bars, IPA results obtained with differentially methylated genes that were common to both tissues (p < 0.05, n = 1029). n, number of genes involved in each disease/disorder.
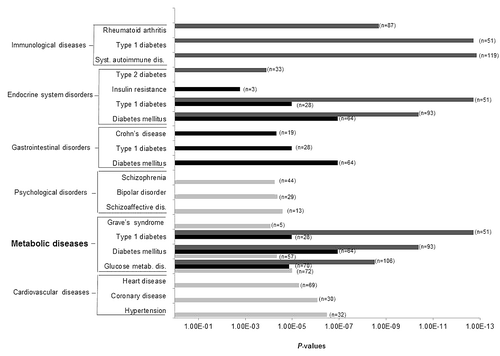
Correlations between DNA methylation levels and newborn weight
Among the first set of potentially differentially methylated genes identified (at p < 0.05), 10% in the placenta (326 out of 3271) and 3% in cord blood (117 out of 3757) were also correlated with newborn weight (p < 0.05; r > 0.297 for placenta; r > 0.312 for cord blood) (). The mean DNA methylation difference was of 6.5 ± 3.3% for these placenta genes and 3.6 ± 2.7% for cord blood genes.
Discussion
To the best of our knowledge, this is the first study reporting the impact of GDM exposure on offspring DNA methylation levels across the genome using the 450K platform. Our primary goal was to better understand the impact of GDM at the epigenome-wide level by identifying genes and their pathways epigenetically affected by GDM. Our secondary goal was to examine whether the differentially methylated genes identified were correlated with birth weight. The most important finding of this study is that GDM epigenetically affects genes that are predominantly involved in metabolic diseases pathway (including glucose-related disorders), in line with the hypothesis that GDM exposure increases the risk of metabolic disorders through epigenetic modifications. Furthermore, several of the potentially differentially methylated genes identified were also correlated with newborn weight. Importantly, these results were obtained in the context of mild maternal hyperglycemia. Indeed, although women included in this study were diagnosed with GDM based on the World Health Organization (WHO) criteria,Citation22 only two fulfilled the Canadian Diabetes Association more severe criteria for GDM diagnosis.Citation4 Our results therefore suggest that even a slight deterioration in the in utero environment, reflected here by a modest hyperglycemia, may induce epigenetic adaptations that have the potential to influence the long-term risk for obesity and type 2 diabetes in the offspring. Our findings emphasize the need to develop better preconception and early pregnancy health care programs aiming at preventing maternal hyperglycemia and eventually the associated epigenetic modifications that may determine the future health of the child.
Interestingly, we found that more than 25% of the differentially methylated genes were common to both placenta and cord blood. Whether these epigenetic marks reflect biological phenomena occurring in other tissues remains to be determined. At this time, it is still unclear in what proportion the DNA methylation profile correlates between different cell types. However, experimental work with rats suggests that methylation changes induced by the maternal diet can be similar in cord blood and liver.Citation23 Recently, data on humans showed that DNA methylation appears to be independent of tissue of origin for some genomic regions, especially those containing imprinted genes or metastable epialleles,Citation24,Citation25 whereas other regions have a clear tissue-specific dependence.Citation25,Citation26 These results therefore suggest that DNA methylation marks affected by in utero challenges may correlate, at least partially, between different cell types. Importantly, these epigenetic marks acquired early in life may have a long-lasting impact on future health, as reported by a recent study showing that some epigenetic marks in buccal cells were stable from birth to 1 y of age.Citation25 Furthermore, studies have shown that cord blood methylation levels were associated with obesity-related phenotypes in childhoodCitation27,Citation28; these results suggest that epigenetic marks might reflect biological phenomena occurring in other tissues involved in growth and energy metabolism, such as the adipose tissue or the hypothalamus.
Common diseases and disorders pathway analyses were performed to determine the overall biological relevance of the identified differentially methylated genes in placenta or cord blood. More than 75% of the genes selected to conduct these analyses had a mean DNA methylation difference ≥ 3%, which is expected to be of biological significance. Indeed, a difference in methylation levels of 3.4% at the H19 DMR gene at birth predicted overweight/obesity in children at one y of age.Citation29 Pathway analyses revealed that those genes were related to cardiovascular, gastrointestinal and metabolic diseases, to mention only a few. The metabolic diseases pathway (including glucose metabolism-related disorders) was the most promising as it was among the two top-ranked pathways in both placenta and cord blood, as well as among the differentially methylated genes common to both tissues.
As suggested by the pathway analysis results, a number of the identified epivariations affect glucose metabolism candidate genes. Among them, VIPR1 in placenta and TRIB1 in cord blood are very promising candidate genes. VIPR1 encodes a receptor for vasoactive intestinal peptides (VIP). VIP stimulate insulin secretion in a glucose-dependent manner as well as glucagon secretion.Citation30 Vipr1 knockout mice have been generated; they show growth retardation, increased metabolic rate, increased insulin sensitivity and a reduced body fat mass, suggesting that VIPR1 plays a role in energy metabolism, lipid metabolism and regulation of body weight.Citation30 VIPR1 is also expressed in cells of the immune system, and it has been shown to play a role in controlling inflammation,Citation31 a central feature of obesity and type 2 diabetes. Animal studies reported that the administration of a VIPR1 agonist ameliorated streptozotocin-induced type 1 diabetes (by increasing the insulin-secreting activity of islets) and protected mice against oxidative stress and inflammation associated with diabetes.Citation32 Tribbles-1 (TRIB1) is a novel gene that appears to be particularly important for the regulation of the lipid/energy homeostasis. Several genome-wide association studies have reported an association between TRIB1 SNPs and plasma lipid concentrations (triglycerides, HDL-c and cholesterol).Citation33-Citation36 In mice, TRIB1 gene deficiency has been associated with an impaired cytokine expression in white adipocytes and resistance to high-fat diet-induced obesity.Citation37
We also examined whether the differentially methylated genes identified in our study were correlated with newborn weight. We found a correlation between DNA methylation levels and newborn weight for only 10% and 3% of the genes significantly affected by GDM exposure in placenta and cord blood respectively. These genes showed a mean DNA methylation difference of 6.5 ± 3.3% (placenta genes) and 3.6 ± 2.7% (cord blood genes), which is again expected to be of biological significance.Citation29 Among the differentially methylated genes that were the most significantly correlated with newborn weight (p < 0.01), Msh homeobox 1(MSX1) was the only one which was previously found to be associated with birth weight or fetal growthCitation38 (Table S3). It is worth noting that GDM-induced DNA methylation changes will very likely affect the infants’ risk of developing obesity and type 2 diabetes later in life without affecting their birth weight. Supporting this hypothesis, two recent studies found strong associations between cord blood DNA methylation levels and obesity-related phenotypes measured at age 9 y, whereas cord blood DNA methylation levels at the same genes were not associated with newborn weight.Citation27,Citation28 These findings suggest that some of the epipolymorphisms observed may have a modest effect on fetal growth, but may have a more pronounced effect on the phenotype during postnatal and childhood development and lifelong development of metabolic disorders.
We found that a number of genes were potentially epigenetically affected by GDM exposure in the placenta and cord blood. However, we cannot exclude the possibility that other factors may have influenced the observed changes in the DNA methylation pattern. Women in the GDM group gained less weight than those in the non-GDM group throughout pregnancy, reflecting the clinical management by a dietary approach. It is therefore likely that some of the associations observed between DNA methylation levels and GDM were influenced by gestational weight gain (GWG). This possibility was verified but, given the small sample size and the exclusion of five women in the GDM group for whom GWG was missing, these analyses were performed only on the most significant loci (p < 0.001, Table S1). The results suggest that GWG contributed only modestly to these associations as only 16% and 10% of the associations in the placenta and cord blood respectively were lost after adjusting for GWG. Our results may also have been influenced by the treatment the GDM mothers received (diet vs. diet+insulin). However, due to the small sample size, we were unable to test this hypothesis. Nevertheless, no DNA methylation difference between the diet (n = 88) and diet+insulin (n = 98) treatment groups was found in the El Hajj et al. study.Citation21 This also suggests that GDM treatments have only a marginal effect on the DNA methylation profile, although it does not exclude that other loci might be affected. In the current study, we also report that some differentially methylated genes between placenta and cord blood exposed or not to GDM were correlated with newborn weight (after adjustment for newborn gestational age and sex). Other factors are known to be associated with newborn weight, such as maternal BMICitation39 and GWG.Citation40 However, the correlations remained largely unchanged after further adjustments for these factors.
Our study has a number of strengths. Among them, the longitudinal follow-up of the women is critical. It allows first to correctly identify women who developed GDM, avoiding misclassification of those who may have undiagnosed diabetes before pregnancy; second, to more precisely match groups; and third, to take into account the metabolic changes throughout pregnancy (potential confounding factors). Furthermore, this study was conducted in a homogeneous population from European descent, which is a strength in genetic and epigenetic studies, although the results might not be applicable to other populations and should therefore be validated. Also, because of the needed extensive clinical follow-up of women throughout pregnancy and the cost of such an epigenome-wide study, it has not been possible to increase the number of samples analyzed. The resulting limited statistical power precluded making any strong conclusions at the individual gene level. It should also be noted that there are limitations associated with the use of pathway analysis software, such as IPA. These tools have been designed for general extraction of information from biological texts. However, this “text-mining” approach is limited by the robustness of its algorithm, with the possibility of generating inaccurate information. Lastly, it is difficult to exclude the possibility that minor differences in cell counts may exist between placenta and cord blood samples exposed or not to GDM. Unfortunately, cell counts were not available in the current study. However, Talens et al.Citation41 previously reported that the majority of inter-individual variation in DNA methylation is not affected (or only marginally) by cellular composition. Consequently, and because many loci were identified in both placenta and cord blood, it is unlikely that differences in cell composition had large effects on the current results. Despite these limitations, the study design and proposed analytical strategy as well as the converging results between placenta and cord blood tissues all contribute to increase our confidence in the results. Furthermore, this study remains the larger ever performed on this topic, and recruitment is still ongoing. This will allow us eventually to confirm and validate these results, as well as investigate their functional impact on gene expression. Indeed, neither gene expression nor evidence of chromatin structure changes was investigated in the current study. These research angles will be pursued in follow-up studies.
In summary, this is the first epigenome-wide study investigating the effects of GDM on the newborn epigenome and whether fetal growth and development might be affected by these epigenetic adaptations. Our results suggest that GDM has epigenetic effects at genes that are preferentially involved in metabolic diseases, including diabetes, probably through both β-cell function and energy/adiposity regulation. In addition, we showed that some of the GDM-exposed dysregulated genes are also involved in fetal growth and development, which may explain, at least partially, the well-known association between GDM and macrosomia. Our data therefore provide supportive evidence that DNA methylation is involved in fetal metabolic programming.
Materials and Methods
Women with a singleton pregnancy were recruited in the Saguenay area from a founder population of French-Canadian origin (self-reported). They were all followed from 1st trimester of pregnancy to delivery, with three visits (12–14 weeks, 24–28 weeks and 36–37 weeks of gestation) at the research center. Women were excluded from the study if they were ≥ 40 y old, had pre-gestational diabetes or other disorders known to affect glucose metabolism (polycystic ovarian syndrome, uncontrolled thyroid disorders and renal insufficiency), or a positive history of alcohol and/or drug abuse during the current pregnancy. The Chicoutimi Hospital Ethics Committee approved the project. All women provided written informed consent before their inclusion in the study, in accordance with the Declaration of Helsinki.
At each visit, anthropometric variables were measured using standardized procedures,Citation42 and blood samples were collected after a 12 h fast. Blood glucose, insulin and lipid measurements were made on fresh serum samples at the Chicoutimi Hospital Clinical Laboratory. Plasma glucose, cholesterol and triglyceride levels were evaluated using a Beckman analyzer (model CX7; Beckman), and insulin measurements were performed using a radioimmunoassay method (Advia Centaur; Simens). Glucose tolerance was assessed using a 75-g OGTT performed at 24 - 28 weeks of gestation. Women with a 2 h post-OGTT glucose level ≥ 7.8 mmol/L were classified as having GDM based on the 1999 WHO criteriaCitation22 and were treated with diet only or with diet and insulin to achieve glycemic control based on clinical guidelines. Clinical information about the newborn was collected at birth from medical files and included gestational age, weight, length and sex of the newborn.
This study included 44 women. Thirty women classified as having GDMCitation22 (16 treated with diet only and 14 with diet and insulin) were available in our cohort and were therefore selected. Among the remaining cohort, 14 NGT women were randomly selected to avoid differences between groups in glucose and lipid profile at first trimester of pregnancy.
Placenta and cord blood sampling
Placenta and cord blood samples were taken within minutes of delivery (on average < 15 min) and kept in RNALater (Qiagen) at -80 °C until nucleic acid extraction. Placenta biopsies were collected from the fetal side, consisting of the intervillous tissues and chorionic villi. Placenta samples were available for 44 subjects and cord blood samples for 40 of the same newborns. There was no significant difference between maternal and newborn characteristics for cord blood and placenta BeadChips analyses, although 4 samples were missing in the former data set.
DNA methylation analyses
DNA was purified from placenta biopsies using the All Prep DNA/RNA/Protein Mini Kit (Qiagen) and from cord-blood samples using the Gentra Puregen Cell Kit (Qiagen). The methylation levels of > 485,000 individual cytosines across the genome were measured using the Infinium HumanMethylation450 BeadChips (Illumina, Inc.). This microarray uses multiplex genotyping assays on bisulfite treated genomic DNA. Briefly, for each CpG site to be interrogated, a probe specific to each “allele” (methylated vs. unmethylated cytosines) is designed. Then, a single-base extension of the probes incorporates a labeled ddNTP. The level of methylation for each locus is determined by calculating the ratio of the fluorescent signals from the methylated (cytosine) vs. unmethylated (uracil) sites. Each probe signal is then used to compute a β value (β), which is a quantitative measure of DNA methylation ranging from 0 (no cytosine methylation) to 1 (complete cytosine methylation). Quality controls were conducted according to the manufacturer’s recommendations. The quality control steps included: DNP and Biotin staining, bisulfite conversion (Fig. S1), extension, hybridization, target removal, negative and non-polymorphic controls. The whole-genome DNA methylation analyses were performed at the McGill University and Genome Quebec Innovation Center.
Differential DNA methylation analyses were performed using the R software (version 2.12) and bioconductor packages (version 2.10). To identify differentially methylated CpG sites between samples exposed or not to GDM, the difference in mean methylation levels between the two groups was analyzed using an unpaired Wilcoxon test. Although the HumanMethylation450 BeadChip array accuracy has already been confirmed,Citation43 16 CpG dinucleotides in placenta and 3 in cord blood samples were validated using bisulfite DNA pyrosequencing. These CpGs were selected for validation in the context of this and ongoing follow-up studies. DNA methylation levels obtained with both methods were highly correlated (r ≥ 0.88; p < 0.001, Table S4), supporting an appropriate overall efficiency, sensitivity, and specificity without remarkable deviations from the optimal performance.
BeadChips and statistical analysis: the applied strategy
It was anticipated that the sample size might be at limit to achieve the requested statistical power for such a genome-wide analysis (false discovery rate [FDR] or Bonferroni) if aiming to identify individual loci. Accordingly, post-hoc power analysis revealed a 31% power (with an α = 0.05, two-sided). We therefore decided to focus our attention on pathway analyses to reduce the chance of false positives. The following analytical strategy based on converging results obtained from placenta and cord blood analyses (same newborns) was planned to circumvent this potential limitation. The supportive idea is that converging results will be more likely to be true positives and will thus increase the confidence in the results.
First, placenta and cord blood tissues from the same newborn were analyzed, thus increasing the effective sample size that was at first limited. Second, the converging analytical strategy was also applied to a step-by-step statistical approach. We first excluded the following loci from the statistical analyses: (1) loci on sex chromosomes (X and Y; highly complex due to differences between males and females and X-inactivation by DNA methylation in women); (2) loci with a β-value (% DNA methylation) < 0.10 and > 0.90, considered unmethylated and fully methylated respectively in either the GDM or non-GDM group; and (3) loci with low variability (standard deviation (SD) < 3%) in either the GDM or non-GDM group. Therefore, the number of statistical tests was reduced by limiting the analysis to autosomal epialleles (alleles on non-sex chromosomes that are variably methylated, despite similar DNA sequences, because of epigenetic modifications established during early development that are thought to be particularly vulnerable to environmental influences). An unpaired Wilcoxon test was then used to identify loci showing DNA methylation differences between placenta and cord blood samples exposed or not to GDM. The significance level was set to p < 0.05 (intended relaxed criteria in accordance with the study design and the applied converging analytical strategy) to identify a first set of differentially methylated genes. This first set was used to evaluate the potential overall effects GDM may have had on the newborn epigenetic profile and the extent to which common genes were differentially methylated between placenta and cord blood. Further analyses were performed to test whether DNA methylation levels of these genes were correlated with the newborn birth weight. Indeed, birth weight is a phenotype considered to integrate the impact of the fetal environment, is strongly influenced by maternal glycemia, and has been associated with a risk of adult chronic diseases at both end of the spectrum. Pearson’s correlation coefficients were calculated. Correlation analyses were adjusted for newborn gestational age and sex.
Pathway analysis helps to add structure to the very large amount of data generated by microarrays. This type of analysis allows determining whether differentially methylated genes belong to predefined networks more than by chance alone. Gene ontology enrichment was performed using the Ingenuity Pathway Analysis (IPA) software (Ingenuity System). IPA compares a provided list of genes (differentially methylated genes in this case) to a reference list of genes included in various biological pathways. It provides a p-value based on a hypergeometric test identifying over-represented gene ontology categories.
Three consecutive IPA were performed. The first two analyses included placenta or cord blood genes showing DNA methylation differences between the GDM and non-GDM groups at p < 0.01. A more stringent gene selection criterion was used (p < 0.01 instead of p < 0.05) to prevent over-representing genes that were modestly differentially methylated. The third analysis included differentially methylated genes that were common to both tissues. A less stringent p-value (p < 0.05) was used to select common genes because a selective criterion (i.e., shared epigenetic signature across the two tissues) had already been applied before this analysis. To identify the most promising GDM-affected common disease and disorder pathways, we concentrated on the three top-ranked pathways as well as the three top-ranked diseases and disorders associated to each pathway.
Unpaired Student t-tests were used to assess the statistical differences in maternal and newborn characteristics between the GDM and non-GDM groups. Variables not normally distributed (first trimester fasting cholesterol and LDL-c levels, and second trimester 2 h post-OGTT glucose levels) were log-transformed. Significance level was set at p < 0.05 (two-sided). Statistical analyses were performed using the SAS software (version 9.1.3).
Abbreviations: | ||
BMI | = | body mass index |
DPP6 | = | dipeptidyl-peptidase 6 |
GDM | = | gestational diabetes mellitus |
GWG | = | gestational weight gain |
IPA | = | Ingenuity Pathway Analysis |
NGT | = | normal glucose tolerance |
OGTT | = | oral glucose tolerance test |
STC2 | = | stanniocalcin 2 |
TRIB1 | = | tribbles homolog 1 |
VIPR1 | = | vasoactive intestinal peptide receptor 1 |
WHO | = | World Health Organization |
Additional material
Download Zip (353.5 KB)Acknowledgments
This project was supported by ECOGENE-21, the Canadian Institutes of Health Research (CIHR team in community genetics [grant #CTP-82941]), the CIHR and Fonds de la Recherche du Québec, Santé (FRQ-S). SMR is recipient of a postdoctoral fellowship from the Canadian Diabetes Association. AAH is a recipient of a scholarship from Diabète Québec, the Faculty of Medicine and Health Sciences, Université de Sherbrooke and the FRQ-S. LB and MFH are junior research scholars from the FRQ-S. MFH is also supported by a Canadian Diabetes Association clinical scientist award. LB, MFH, PP and JPB are members of the FRQ-S-funded Centre de recherche clinique Étienne-Le Bel (affiliated to Centre Hospitalier de l’Université de Sherbrooke).
We warmly acknowledge the contribution of Sébastien Claveau (MSc), ECOGENE-21 Laboratory; Nadia Mior, ECOGENE-21 Laboratory; Jeannine Landry (RN), ECOGENE-21 Clinical Research Center; and Chantale Aubut (RN), ECOGENE-21 Clinical Research Center for their dedicated work in this study. We also express our gratitude to Céline Bélanger, Chicoutimi Hospital, for her thoughtful revision of the manuscript.
Disclosure of Potential Conflicts of Interest
No potential conflicts of interest were disclosed.
Authors Contributions
SMR and AAH performed the data analysis and interpretation and wrote the manuscript. GV performed the data analysis and revised the manuscript. JSP, JPB, DG revised the manuscript. MFH contributed to discussions and revised the manuscript. PP and DB participated in the conception of the study design and revised the manuscript. LB conceived the study design, participated in the data interpretation, contributed to discussions and revised the manuscript.
Supplemental Materials
Supplemental materials may be found here: http://www.landesbioscience.com/journals/epigenetics/article/25578/
References
- Chu SY, Kim SY, Bish CL. Prepregnancy obesity prevalence in the United States, 2004-2005. Matern Child Health J 2009; 13:614 - 20; http://dx.doi.org/10.1007/s10995-008-0388-3; PMID: 18618231
- Chu SY, Callaghan WM, Bish CL, D'Angelo D. Gestational weight gain by body mass index among US women delivering live births, 2004-2005: fueling future obesity. Am J Obstet Gynecol 2009; 200:271
- Gibson KS, Waters TP, Catalano PM. Maternal weight gain in women who develop gestational diabetes mellitus. Obstet Gynecol 2012; 119:560 - 5; http://dx.doi.org/10.1097/AOG.0b013e31824758e0; PMID: 22353954
- CDA. Canadian Diabetes Association: 2008 Clinical Practice Guidelines for the Prevention and Management of Diabetes in Canada. Canadian Journal of Diabetes 2008; 32:S1 - 201
- Finkelstein EA, Khavjou OA, Thompson H, Trogdon JG, Pan L, Sherry B, et al. Obesity and severe obesity forecasts through 2030. Am J Prev Med 2012; 42:563 - 70; http://dx.doi.org/10.1016/j.amepre.2011.10.026; PMID: 22608371
- ADA. Diagnosis and Classification of Diabetes Mellitus. Diabetes Care 2011; 34:S62 - 9; http://dx.doi.org/10.2337/dc11-S062; PMID: 21193628
- Wendland EM, Torloni MR, Falavigna M, Trujillo J, Dode MA, Campos MA, et al. Gestational diabetes and pregnancy outcomes--a systematic review of the World Health Organization (WHO) and the International Association of Diabetes in Pregnancy Study Groups (IADPSG) diagnostic criteria. BMC Pregnancy Childbirth 2012; 12:23; http://dx.doi.org/10.1186/1471-2393-12-23; PMID: 22462760
- Lawlor DA, Lichtenstein P, Långström N. Association of maternal diabetes mellitus in pregnancy with offspring adiposity into early adulthood: sibling study in a prospective cohort of 280,866 men from 248,293 families. Circulation 2011; 123:258 - 65; http://dx.doi.org/10.1161/CIRCULATIONAHA.110.980169; PMID: 21220735
- Crume TL, Ogden L, West NA, Vehik KS, Scherzinger A, Daniels S, et al. Association of exposure to diabetes in utero with adiposity and fat distribution in a multiethnic population of youth: the Exploring Perinatal Outcomes among Children (EPOCH) Study. Diabetologia 2011; 54:87 - 92; http://dx.doi.org/10.1007/s00125-010-1925-3; PMID: 20953862
- Silverman BL, Rizzo TA, Cho NH, Metzger BE, The Northwestern University Diabetes in Pregnancy Center. Long-term effects of the intrauterine environment. Diabetes Care 1998; 21:Suppl 2 B142 - 9; PMID: 9704242
- Bunt JC, Tataranni PA, Salbe AD. Intrauterine exposure to diabetes is a determinant of hemoglobin A(1)c and systolic blood pressure in pima Indian children. J Clin Endocrinol Metab 2005; 90:3225 - 9; http://dx.doi.org/10.1210/jc.2005-0007; PMID: 15797952
- Dabelea D, Mayer-Davis EJ, Lamichhane AP, D’Agostino RB Jr., Liese AD, Vehik KS, et al. Association of intrauterine exposure to maternal diabetes and obesity with type 2 diabetes in youth: the SEARCH Case-Control Study. Diabetes Care 2008; 31:1422 - 6; http://dx.doi.org/10.2337/dc07-2417; PMID: 18375420
- West NA, Crume TL, Maligie MA, Dabelea D. Cardiovascular risk factors in children exposed to maternal diabetes in utero. Diabetologia 2011; 54:504 - 7; http://dx.doi.org/10.1007/s00125-010-2008-1; PMID: 21153896
- Hales CN, Barker DJ. Type 2 (non-insulin-dependent) diabetes mellitus: the thrifty phenotype hypothesis. Diabetologia 1992; 35:595 - 601; http://dx.doi.org/10.1007/BF00400248; PMID: 1644236
- Gluckman PD, Cutfield W, Hofman P, Hanson MA. The fetal, neonatal, and infant environments-the long-term consequences for disease risk. Early Hum Dev 2005; 81:51 - 9; http://dx.doi.org/10.1016/j.earlhumdev.2004.10.003; PMID: 15707715
- Plagemann A. Maternal diabetes and perinatal programming. Early Hum Dev 2011; 87:743 - 7; http://dx.doi.org/10.1016/j.earlhumdev.2011.08.018; PMID: 21945359
- Jaenisch R, Bird A. Epigenetic regulation of gene expression: how the genome integrates intrinsic and environmental signals. Nat Genet 2003; 33:Suppl 245 - 54; http://dx.doi.org/10.1038/ng1089; PMID: 12610534
- Wong CC, Caspi A, Williams B, Craig IW, Houts R, Ambler A, et al. A longitudinal study of epigenetic variation in twins. Epigenetics 2010; 5:516 - 26; http://dx.doi.org/10.4161/epi.5.6.12226; PMID: 20505345
- Bouchard L, Thibault S, Guay SP, Santure M, Monpetit A, St-Pierre J, et al. Leptin gene epigenetic adaptation to impaired glucose metabolism during pregnancy. Diabetes Care 2010; 33:2436 - 41; http://dx.doi.org/10.2337/dc10-1024; PMID: 20724651
- Bouchard L, Hivert MF, Guay SP, St-Pierre J, Perron P, Brisson D. Placental adiponectin gene DNA methylation levels are associated with mothers’ blood glucose concentration. Diabetes 2012; 61:1272 - 80; http://dx.doi.org/10.2337/db11-1160; PMID: 22396200
- El Hajj N, Pliushch G, Schneider E, Dittrich M, Müller T, Korenkov M, et al. Metabolic programming of MEST DNA methylation by intrauterine exposure to gestational diabetes mellitus. Diabetes 2013; 62:1320 - 8; http://dx.doi.org/10.2337/db12-0289; PMID: 23209187
- WHO. . Definition, Diagnosis and Classification of Diabetes Mellitus and Its Complications: Report of a WHO Consultation. Part 1: Diagnosis and Classification of Diabetes Mellitus. 1999.
- Burdge GC, Hanson MA, Slater-Jefferies JL, Lillycrop KA. Epigenetic regulation of transcription: a mechanism for inducing variations in phenotype (fetal programming) by differences in nutrition during early life?. Br J Nutr 2007; 97:1036 - 46; http://dx.doi.org/10.1017/S0007114507682920; PMID: 17381976
- Waterland RA, Kellermayer R, Laritsky E, Rayco-Solon P, Harris RA, Travisano M, et al. Season of conception in rural gambia affects DNA methylation at putative human metastable epialleles. PLoS Genet 2010; 6:e1001252; http://dx.doi.org/10.1371/journal.pgen.1001252; PMID: 21203497
- Murphy SK, Huang Z, Hoyo C. Differentially methylated regions of imprinted genes in prenatal, perinatal and postnatal human tissues. PLoS One 2012; 7:e40924; http://dx.doi.org/10.1371/journal.pone.0040924; PMID: 22808284
- Ollikainen M, Smith KR, Joo EJ, Ng HK, Andronikos R, Novakovic B, et al. DNA methylation analysis of multiple tissues from newborn twins reveals both genetic and intrauterine components to variation in the human neonatal epigenome. Hum Mol Genet 2010; 19:4176 - 88; http://dx.doi.org/10.1093/hmg/ddq336; PMID: 20699328
- Relton CL, Groom A, St Pourcain B, Sayers AE, Swan DC, Embleton ND, et al. DNA methylation patterns in cord blood DNA and body size in childhood. PLoS One 2012; 7:e31821; http://dx.doi.org/10.1371/journal.pone.0031821; PMID: 22431966
- Godfrey KM, Sheppard A, Gluckman PD, Lillycrop KA, Burdge GC, McLean C, et al. Epigenetic gene promoter methylation at birth is associated with child’s later adiposity. Diabetes 2011; 60:1528 - 34; http://dx.doi.org/10.2337/db10-0979; PMID: 21471513
- Perkins E, Murphy SK, Murtha AP, Schildkraut J, Jirtle RL, Demark-Wahnefried W, et al. Insulin-like growth factor 2/H19 methylation at birth and risk of overweight and obesity in children. J Pediatr 2012; 161:31 - 9; http://dx.doi.org/10.1016/j.jpeds.2012.01.015; PMID: 22341586
- Moody TW, Ito T, Osefo N, Jensen RT. VIP and PACAP: recent insights into their functions/roles in physiology and disease from molecular and genetic studies. Curr Opin Endocrinol Diabetes Obes 2011; 18:61 - 7; http://dx.doi.org/10.1097/MED.0b013e328342568a; PMID: 21157320
- Delgado M, Robledo G, Rueda B, Varela N, O’Valle F, Hernandez-Cortes P, et al. Genetic association of vasoactive intestinal peptide receptor with rheumatoid arthritis: altered expression and signal in immune cells. Arthritis Rheum 2008; 58:1010 - 9; http://dx.doi.org/10.1002/art.23482; PMID: 18383379
- Yu R, Zhang H, Huang L, Liu X, Chen J. Anti-hyperglycemic, antioxidant and anti-inflammatory effects of VIP and a VPAC1 agonist on streptozotocin-induced diabetic mice. Peptides 2011; 32:216 - 22; http://dx.doi.org/10.1016/j.peptides.2010.11.017; PMID: 21129425
- Edmondson AC, Braund PS, Stylianou IM, Khera AV, Nelson CP, Wolfe ML, et al. Dense genotyping of candidate gene loci identifies variants associated with high-density lipoprotein cholesterol. Circ Cardiovasc Genet 2011; 4:145 - 55; http://dx.doi.org/10.1161/CIRCGENETICS.110.957563; PMID: 21303902
- Kathiresan S, Melander O, Guiducci C, Surti A, Burtt NP, Rieder MJ, et al. Six new loci associated with blood low-density lipoprotein cholesterol, high-density lipoprotein cholesterol or triglycerides in humans. Nat Genet 2008; 40:189 - 97; http://dx.doi.org/10.1038/ng.75; PMID: 18193044
- Kraja AT, Vaidya D, Pankow JS, Goodarzi MO, Assimes TL, Kullo IJ, et al. A bivariate genome-wide approach to metabolic syndrome: STAMPEED consortium. Diabetes 2011; 60:1329 - 39; http://dx.doi.org/10.2337/db10-1011; PMID: 21386085
- Varbo A, Benn M, Tybjærg-Hansen A, Grande P, Nordestgaard BG. TRIB1 and GCKR polymorphisms, lipid levels, and risk of ischemic heart disease in the general population. Arterioscler Thromb Vasc Biol 2011; 31:451 - 7; http://dx.doi.org/10.1161/ATVBAHA.110.216333; PMID: 21071687
- Ostertag A, Jones A, Rose AJ, Liebert M, Kleinsorg S, Reimann A, et al. Control of adipose tissue inflammation through TRB1. Diabetes 2010; 59:1991 - 2000; http://dx.doi.org/10.2337/db09-1537; PMID: 20522600
- Turan N, Ghalwash MF, Katari S, Coutifaris C, Obradovic Z, Sapienza C. DNA methylation differences at growth related genes correlate with birth weight: a molecular signature linked to developmental origins of adult disease?. BMC Med Genomics 2012; 5:10; http://dx.doi.org/10.1186/1755-8794-5-10; PMID: 22498030
- Yu Z, Han S, Zhu J, Sun X, Ji C, Guo X. Pre-pregnancy body mass index in relation to infant birth weight and offspring overweight/obesity: a systematic review and meta-analysis. PLoS One 2013; 8:e61627; http://dx.doi.org/10.1371/journal.pone.0061627; PMID: 23613888
- Ludwig DS, Currie J. The association between pregnancy weight gain and birthweight: a within-family comparison. Lancet 2010; 376:984 - 90; http://dx.doi.org/10.1016/S0140-6736(10)60751-9; PMID: 20691469
- Talens RP, Boomsma DI, Tobi EW, Kremer D, Jukema JW, Willemsen G, et al. Variation, patterns, and temporal stability of DNA methylation: considerations for epigenetic epidemiology. FASEB J 2010; 24:3135 - 44; http://dx.doi.org/10.1096/fj.09-150490; PMID: 20385621
- Lohman TG, Roche AF, Martorell R, eds. Anthropometric standardization reference manual. Champaign, IL, Human Kinetics Books, 1988.
- Bibikova M, Barnes B, Tsan C, Ho V, Klotzle B, Le JM, et al. High density DNA methylation array with single CpG site resolution. Genomics 2011; 98:288 - 95; http://dx.doi.org/10.1016/j.ygeno.2011.07.007; PMID: 21839163