Abstract
Stem cells have been found in most tissues/organs. These somatic stem cells produce replacements for lost and damaged cells, and it is not completely understood how this regenerative capacity becomes diminished during aging. To study the possible involvement of epigenetic changes in somatic stem cell aging, we used murine hematopoiesis as a model system. Hematopoietic stem cells (HSCs) were enriched for via Hoechst exclusion activity (SP-HSC) from young, medium-aged and old mice and subjected to comprehensive, global methylome (MeDIP-seq) analysis. With age, we observed a global loss of DNA methylation of approximately 5%, but an increase in methylation at some CpG islands. Just over 100 significant (FDR < 0.2) aging-specific differentially methylated regions (aDMRs) were identified, which are surprisingly few considering the profound age-based changes that occur in HSC biology. Interestingly, the polycomb repressive complex -2 (PCRC2) target genes Kiss1r, Nav2 and Hsf4 were hypermethylated with age. The promoter for the Sdpr gene was determined to be progressively hypomethylated with age. This occurred concurrently with an increase in gene expression with age. To explore this relationship further, we cultured isolated SP-HSC in the presence of 5-aza-deoxycytdine and demonstrated a negative correlation between Sdpr promoter methylation and gene expression. We report that DNA methylation patterns are well preserved during hematopoietic stem cell aging, confirm that PCRC2 targets are increasingly methylated with age, and suggest that SDPR expression changes with age in HSCs may be regulated via age-based alterations in DNA methylation.
Introduction
Stem cells have been found in most tissues/organs. These somatic stem cells produce replacements for lost and damaged cells, and it is not completely understood how this regenerative capacity becomes diminished during aging. In addition, diseases of the immune system especially myeloid cancers, inflammatory diseases and immune deficiencies, are major burdens of human aging. As a result, there is remarkable interest to delineate age-based alterations in primitive cells of the hematopoietic system. To study the possible involvement of epigenetic changes in aging of the human hematopoietic system, we used murine hematopoiesis as a model system.
Hematopoietic stem cells (HSCs) are responsible for the maintenance of immune and hematopoietic cells throughout life.Citation1 Several studies have reported a decline in HSC functionality and a differentiation bias toward myelopoiesis with age.Citation2-Citation6 This occurs concurrently with in an increase in the absolute number and relative frequency of HSCs.Citation4,Citation5
Primitive HSCs possess high ABC/G2 pump activity and can be identified via Hoechst dye exclusion assays.Citation7 HSCs that are identified by their Hoechst exclusion activity are known as side population (SP) cells. We have previously reported that the SP subset of murine HSCs accumulates with age.Citation4 In particular, cells with the highest Hoechst exclusion activity (termed “lower SP cells” [LSP]) increase with age when compared with other subsets of murine stem and progenitor cells.Citation4,Citation5 As such, analysis of lower SP cells from aged mice and comparison to lower SP from their non-aged counterparts provides an opportunity to examine the nature of aging murine HSCs that have been identified by a physiological property (ABCG2 activity) that is conserved across multiple species, including humans.Citation8
Functional and gene expression changes in aging HSCs have been previously reported.Citation5,Citation9 However, the exact mechanism governing these changes remains to be elucidated. Epigenetic mechanisms have been implicated in regulation of fundamental stem cell functions, some of which are altered during aging of HSCs. Indeed, dysregulated expression of epigenetic modifiers has been reported with age.Citation9 DNA methylation (DNAm) involves the addition of a methyl group to the carbon-5 position of cytosine bases, this occurs predominantly in a CG context which is enriched at CpG islands (CGIs), but also at other features in the genome.Citation10DNAm in particular may be important in HSC regulation as mice lacking enzymes that catalyze DNAm at CG dinucleotides, DNA methyltransferases Dnmt1, and Dnmt3a, have defects in HSC self-renewal and differentiation.Citation11-Citation13
We have previously described an optimized version of methylated DNA immunoprecipitation-based sequencing (MeDIP-seq)Citation14-Citation20 with low starting concentrations, termed Nano-MeDIP-seq.Citation21 Nano-MeDIP-seq is a method that allows the unbiased analysis of genome wide DNA methylation from as little as 50ng DNA.
DNA methylation patterns have been previously reported for young HSCs, and more recently DNA methylation patterns analyzed via bisulphite sequencing have been reported for aging HSCs.Citation22-Citation24 Here, we utilized Nano-MeDIP-seq for the analysis of the SP-HSC methylome and report global DNA methylation analysis of a cell population that is highly enriched for HSCs throughout murine aging.
Results
Isolation of Lower Side Population cells
We isolated lower side population cells (LSPs) via flow cytometry from adult female C57b/6 mice at different time points: Young (8 – 12 weeks, referred to as Young), middle-aged (~12 mo, referred to as Mid), and old (22 - 24 mo, referred to as Old). shows the sample collection logic for this study. We enriched for HSCs by selecting for LSPs, which are bone marrow cells defined by the lowest Hoechst staining as determined by their position on the nucleated bone marrow cells (NBMCs) Hoechst profile plot (). We confirmed, by antibody staining for canonical stem cell markers that are reportedly conserved during the murine lifespan (Lin-, c-Kit+, Sca-1+ (KLS); and CD150+) that LSP cells isolated in this study, (referred to from this point on as SP-HSCs) were enriched for HSCs ().Citation3 Further confirmation of the immature phenotype of sorted cells was achieved via re-analysis of previously isolated cells for c-Kit+, Sca-1+, CD150+, and CD48- (, murine cells analyzed in a carrier human cell line [KG1a]).
Figure 1. Isolation of LSP cells. (A) Schematic of study design. SP-HSC were isolated from C57bl/6 mice at three time points from pools of 30 – 45 mice and 3 - 10 mice for each replicate of Young and Mid/Old samples respectively. DNA and RNA was concurrently isolated from FACs purified SP-HSC. 150 - 300 ng of DNA was used for methylome analysis by MeDIP-seq and 50 – 100 ng RNA was used for transcriptome analysis by RNA-seq. Three biological replicates were performed for each step as indicated by (x3). (B) Side population (SP) analysis of Hoechst stained bone marrow cells by FACs. Cells were first gated to select for live cells and Hoechst staining (left), to select for lower SP (LSP) cells that show uniformity in their scatter profile (middle and right). (C) Confirmation that LSP cells from young, mid, and old mice are enriched for LT-HSCs, by their positivity for CD117 (c-Kit), Sca-1, and CD150, as well as negativity for lineage antigens. (D) Confirmation of the immature phenotype of isolated LSP cells via staining of sorted cells for CD48, Sca-1, CD117, and CD150.
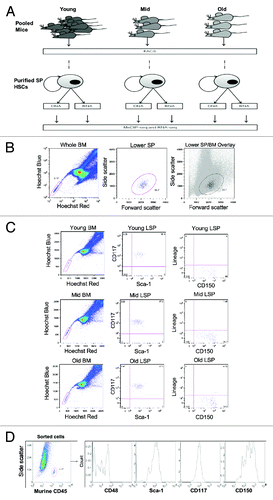
Generation of the SP-HSC methylomes and data quality assessment
We obtained DNA for three separate pools at the aforementioned three time points and generated the corresponding SP-HSC methylomes in triplicates for each age group, using Nano-MeDIP-seq (). This resulted in an average of 5.25 Gb raw paired-end reads per samples (SD ± 1.15 Gb) of which approximately 90% were successfully paired and aligned to the mouse genome (NCBIM37). After filtering (materials and methods), we obtained an average of 1.92 Gb (SD ± 0.3 Gb) high quality (q ≥ 10), uniquely mapping paired-end reads. Approximately 60% of all CpGs in the mouse genome were covered at least 1 fold (Fig. S1), and all MeDIP samples showed a clear CpG enrichment when compared with input control samples (Average MeDIP enrichment score = 2.73; SD ± 0.12; n = 9, Average input enrichment score of 1.11; SD ± 0.03; n = 2). We found a good correlation for all SP-HSC MeDIP samples (average Pearson’s correlation of 0.84, SD ± 0.09). One of the biological replicates for the “Old” time point had a lower correlation of approximately 0.7 and was excluded from global analyses to maintain stringency.
Analysis of the aging SP-HSC methylomes
To investigate DNAm changes in SP-HSC with age, we performed comparative analyses (materials and methods) on normalized methylome data from the Young, Mid, and Old SP-HSC. We detected a global loss of DNAm with age () which we quantified to an approximately 5% decrease (p < 0.001, Kolmogorov-Smirnov test) in the average methylation level aggregated over all covered CpG sites in the Old compared with Young samples (). We did, however, observe an age-related gain of DNAm at some CGIs in Old samples ().
Figure 2. HSC Methylome. (A) Circos plot of SP-HSC methylomes. Outermost circle shows an ideogram of the mouse genome subdivided by chromosomes. The three blue circles show the Old, Mid, and Young SP-HSC methylomes, respectively. The color code indicates the level of global methylation (dark blue, high, and light blue low). The innermost circle depicts CpG density (dark orange, high, and light orange low). (B) Box plots of global and (C) CGI, DNA methylation levels for Young, Mid, and Old SP-HSC. DNA methylation levels are expressed as MEDIPS scores.
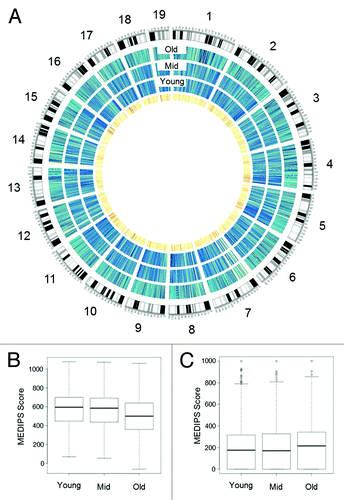
The MeDUSA pipelineCitation20 was used to determine age-specific differentially methylated regions (aDMRs) between the different time points. 53.8% of the mappable mouse genome had a minimum of 10 reads, which allowed us to confidently detect the presence of aDMRs between our Young, Mid, and Old methylomes. A total of 111 significant (FDR < 0.2) aDMRs were found between Old and Young samples, of which 71 were hypermethylated and 40 hypomethylated in the Old samples. These aDMRs appeared to be randomly distributed across all mouse chromosomes (), with the exception of chromosome 14 and 16 (where no DMRs were detected) and chromosome 5, where we found a hotspot significantly (p-value < 0.001) enriched for significantly (FDR < 0.01) hypermethylated aDMRs. This age-related methylation hotspot corresponds to the location of at least 4 known members of the Speer gene family, which includes 14 genes that encode putative glutamate-rich proteins of unknown function.
Figure 3. Differentially methylated genes. (A) Location of significant (FDR < 0.2) age-specific differentially methylated regions (aDMRs). aDMRs were mapped to corresponding genomic coordinates on mouse chromosomes and are represented on an Ensemble-generated ideogram (Ensemble, mm9 genome build NCBIM37). Hyper aDMRs are shown in blue and hypo aDMRs in red. (B) Methylation heatmap of all aDMRs. Yellow indicates hypomethylation and blue indicates hypermethylation.
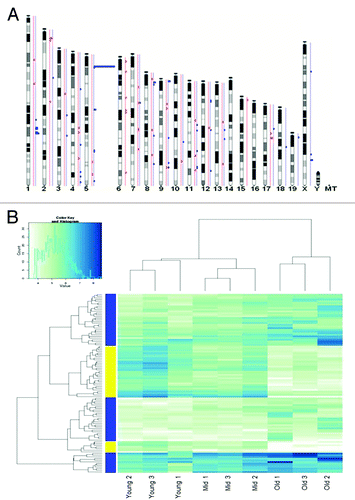
Using a previously determined cut-off of 100 Kb,Citation25 we were able to associate 97% of the identified aDMRs with genes, henceforth referred to as differentially methylated genes (DMGs) (Table S1), and observed a progressive change in methylation with age for many aDMGs. shows a DNAm heatmap for all DMRs, displaying progressive and directional DNAm change at several regions in the Young, Mid, and Old samples.
Using annotation from Ensembl (Ensembl 62), aDMRs were categorized according to their genomic location. Hypermethylated aDMRs were significantly enriched in CGIs (Fishers Exact p-value = 2.33E-09), CGI shores (p-value = 4.30E-09), and exons (p-value = 6.18E-11), and hypo aDMRs were significantly enriched in exons (p-value = 2.14E-06), CGI shores (p-value = 8.39E-05), introns (p-value = 3.6E-03), and intergenic regions (p-value = 0.028). Further analysis using the Ensembl regulatory build (Ensembl 67) revealed that CTCF binding sites were most enriched in the hyper aDMRs and c-MYB sites were most enriched in hypo aDMRs.
Polycomb repressive complex target genes are differentially methylated with age
It has recently been reported that targets of the polycomb repressive complex -2 (PCRC2) members EED and SUZ12 are differentially methylated with age.Citation22Specifically, these PCRC2 targets seem to gain methylation with age. We compared our aDMGs with the data recently published by Beerman et al. and found three PCRC2 target genes that are hypermethylated with age in both studies: Kiss1r, Nav2, and Hsf4 (p < 0.0025, Fisher’s exact test).
Examination of aDMRs with histone data from the ENCODE project revealed that Kiss1r is hypermethylated with age in the 3′ CGI, an area that has been associated with enrichment of the histone marks H3K4me1, H3K4me3, H3K9ac, and H3K27me3. Hsf4 hypermethylation is located in the shore region of its promoter-associated CGI. Interestingly, the region of the Nav2 gene that is hypermethylated with age is intronic, contains multiple regulatory regions (including H3K4me1, H3K4me3, and H3K27ac) and may be a potential enhancer region. In addition to Nav2 hypermethylation, we found an exonic region associated with H3K4me1 that was hypomethylated with age.
Comparison between our data and published data on Suz12 targets in mice ES cellsCitation26 revealed 2 additional potential targets of the PCRC2, Amotl2, and Cyfip2, which, in our study, were hyper and hypomethylated respectively. In addition, Trim37 is a PCRC1 target and was hypermethylated with age in our study. One other gene classified as hypo-aDMG in our study (NPAS3) had a functional equivalent that was reported as a PCRC2 target that was hypermethylated with age by Beerman et al. (NPAS2).
Induced SDPR promoter demethylation is associated with increased gene expression
SDPR has been previously implicated in HSC aging via reports of age-based increases in gene expression.Citation5,Citation9 Here we report that the sdpr gene has progressive loss of DNAm with age at the promoter region. () This was confirmed by bisulphite pyro-sequencing of independent Young, Mid, and Old samples (). As previously reported, this age-based loss of promoter methylation occurs concurrently with a progressive gain of gene expression in SP cells (). To further explore this relationship between age-based promoter hypomethylation and increased gene expression, we demethylated highly purified primary SP-HSC by 5-Aza'deoxyCytidine (5-Aza'dC) treatment during a 4 d ex vivo culture process. The culture conditions have been previously described to promote slight expansion (cell division) of HSCs with minimal differentiation.Citation27 This treatment protocol resulted in an upregulation of Sdpr gene expression (, p-value = 0.028 for 2 µM treatment). Hypomethylation of the Sdpr promoter following 5-Aza'dC treatment was confirmed by bisulfite pyro-sequencing of 2 biological replicates (, p-value < 0.005). These results are consistent with an inverse relationship between Sdpr promoter methylation and gene expression.
Figure 4.Sdpr analysis. (A) Screenshot of MeDIP-seq profiles for Sdpr gene in UCSC genome browser (UCSC mm9). Tracks are color-coded blue, green, and orange for Young, Mid, and Old samples, respectively. Three biological replicates are shown for each age group. Peak height represents DNA methylation. The two aDMRs identified in the Sdpr gene are boxed in red and black. The red box depicts the aDMR selected for validation (B–E). This region aligns with other members of the human CAVIN gene family. ‘Reg. Feature’ denotes the location of the Ensembl promoter associated regulatory feature ENSMUSR00000405109. The promoter associated H3K9ac, H3K36me3, H3K4me3, and RNAP II are localized to this region. (B) Validation of Sdpr promoter hypomethylation (aDMR boxed in red [A]) using bisulfite pyro-sequencing. Data was obtained from SP-HSC pools for three biological replicates for Young samples and four biological replicates each for Mid and Old samples. A methylation heatmap for the individual CpGs assayed, and for each replicate is shown. This data are also displayed as a chart (D). (C) RT-PCR data to confirm published reports of increased Sdpr gene expression with age (n = 3 for each, mean ± SD). (E) Functional analysis of same Sdpr aDMR by ex-vivo culturing of SP-HSC in the presence of Aza-deoxycytidine and expression analysis using qRT-PCR. Data was obtained from independent experiments for a total of four biological replicates. Expression values shown are relative to untreated control. (E) Validation of same Sdpr aDMR for promoter hypomethylation following ex-vivo culturing in the presence of Aza-deoxycytidine Data are shown for two biological replicates. 2–3 mice were pooled per replicate and average value for two treatment replicates is shown for each biological replicate (replicate 1 and 2 – blue and red bars respectively). FC is fold change. Error bars depict standard error of the mean (S.E.M) in all cases (*p < 0.05; **p < 0.005; ***p < 0.0005).
![Figure 4.Sdpr analysis. (A) Screenshot of MeDIP-seq profiles for Sdpr gene in UCSC genome browser (UCSC mm9). Tracks are color-coded blue, green, and orange for Young, Mid, and Old samples, respectively. Three biological replicates are shown for each age group. Peak height represents DNA methylation. The two aDMRs identified in the Sdpr gene are boxed in red and black. The red box depicts the aDMR selected for validation (B–E). This region aligns with other members of the human CAVIN gene family. ‘Reg. Feature’ denotes the location of the Ensembl promoter associated regulatory feature ENSMUSR00000405109. The promoter associated H3K9ac, H3K36me3, H3K4me3, and RNAP II are localized to this region. (B) Validation of Sdpr promoter hypomethylation (aDMR boxed in red [A]) using bisulfite pyro-sequencing. Data was obtained from SP-HSC pools for three biological replicates for Young samples and four biological replicates each for Mid and Old samples. A methylation heatmap for the individual CpGs assayed, and for each replicate is shown. This data are also displayed as a chart (D). (C) RT-PCR data to confirm published reports of increased Sdpr gene expression with age (n = 3 for each, mean ± SD). (E) Functional analysis of same Sdpr aDMR by ex-vivo culturing of SP-HSC in the presence of Aza-deoxycytidine and expression analysis using qRT-PCR. Data was obtained from independent experiments for a total of four biological replicates. Expression values shown are relative to untreated control. (E) Validation of same Sdpr aDMR for promoter hypomethylation following ex-vivo culturing in the presence of Aza-deoxycytidine Data are shown for two biological replicates. 2–3 mice were pooled per replicate and average value for two treatment replicates is shown for each biological replicate (replicate 1 and 2 – blue and red bars respectively). FC is fold change. Error bars depict standard error of the mean (S.E.M) in all cases (*p < 0.05; **p < 0.005; ***p < 0.0005).](/cms/asset/cbea91c9-6353-4709-82f4-f100aad7d63d/kepi_a_10926017_f0004.gif)
Discussion
We conducted methylome analysis of primitive, primary mouse SP-HSCs from (8–12 weeks), Mid (~12 mo), and Old (22–24 mo) C57bl/6 mice. This population is highly enriched for HSCsCitation7,Citation28 and contains the subset of primitive cells that seems to accumulate with age.Citation4 The LSP subset overlaps with other subsets previously demonstrated as enriched for HSCs throughout the murine lifespan.Citation3-Citation5,Citation12
Consistent with recently published data,Citation22 we report that the overall DNA methylation pattern is highly conserved during HSC aging. This is remarkable, especially when one considers the profound changes in HSC biology that occur during murine aging, such as lineage skewing and accumulation of primitive HSC subsets. Despite this overall maintenance of DNA methylation patterns, we report that over 100 genes are differentially methylated with age, the majority of which were defined as hypermethylation.
We also note that when analyzed globally, there is a significant (5%) loss of global DNA methylation during murine HSC aging. This is consistent with studies into human aging of other cell types, but non-HSCs were analyzed.Citation29-Citation32 Our global loss of DNA methylation is however, inconsistent with the HSC global hypermethylation recently reported by Beerman et al.Citation22 The most likely reason for this is the use of different technologies. We used MeDIP-seq, which provides approximately 60% genome coverage, including CGIs (where we identified global gain of methylation) and many non-CGI features (where we identified global loss of methylation). Beerman et al utilized reduced-representation bisulphite sequencing (RRBS), which only provides 5–10% genome coverage, predominantly of CGIs (where they also identified global gain of methylation) and thus were blind to the much larger loss of methylation at non-CGI features. Where we have overlapping coverage, our data confirm their finding that genes that are targets of the PCRC2 members EED and SUZ12 are differentially methylated with age. In addition, we report age-related differential methylation of 2 other targets of the PCRC2 that were not reported by Beerman et al.Citation22
It may be that this age-based methylation of PCRC2 targets occurs as a result of reduced PCRC2 activity with age. Indeed, the PCRC2 complex has been implicated in the control of fundamental HSC cellular functions that may be related to an age-based decline. For instance, HSCs undergo INK4a/ARF-mediated senescence when the PCRC2 member Ezh2 is downregulated.Citation33
We chose to examine a possible relationship between sdpr gene promoter methylation and gene expression as we observed promoter hypomethylation with age, and an age-related upregulation of Sdpr in HSCs has previously been reported.Citation5,Citation9 Three days of 5-Aza'dC treatment on highly purified LSPs resulted in an increased gene expression that occurred concurrently with a reduction in promoter methylation. These results, as well as the findings that Sdpr was upregulated in Dnmt1-Knockout (KO) mice HSCsCitation13 and in Dnmt3-KO mice HSCs,Citation12 is consistent with an inverse relationship between DNAm and Sdpr gene expression and suggests that SDPR gene expression may be directly or indirectly controlled by promoter methylation. One caveat is that since 5-Aza'dC is a global demethylation agent, the effect on SDPR expression may be due to demethylation of other genes. However, this proof of principle experiment provides a tool for further analysis of the relationship between DNAm and gene expression in rare subsets of cells enriched for HSCs.
While age-related upregulation of Sdpr in HSCs has previously been observed, we have demonstrated that it is linked to loss of promoter methylation. Sdpr, which is also known as Cavin-2, was originally identified as a gene whose expression was increased in serum-starved cells and may be associated with cellular senescence.Citation34 One explanation for the age-based decline in the hematopoietic system that is coupled with an apparent increase in primitive HSCs is an accumulation of damaged or dysfunctional cells that do not contribute to hematopoiesis. It may be that increased Sdpr expression in aged HSCs indicates an inactive, senescence-like state.
In addition, an independent role in caveolae formation and cell signaling has been reported.Citation35Caveolae are thought to be invaginated lipid rafts that provide a common platform for various signaling molecules, leading to enhanced cell signaling and potent signal transduction. Overexpression of Sdpr in cultured cells leads to caveolae elongationCitation35 and perhaps results in an increased surface area for interacting cell signaling molecules. Increased expression in aged HSCs may cause an altered sensitivity to survival or growth signals.
In conclusion, we report that DNA methylation patterns are well preserved during hematopoietic stem cell aging, confirm that PCRC2 targets are differentially methylated with age, and suggest that SDPR gene expression changes with age in HSCs may be regulated via age-based alterations in promoter DNA methylation.
Materials and Methods
Mice and hematopoietic stem cell preparation
Wild-type Young (8–12 weeks), Mid (~12 mo), and Old (22–24 mo) C57bl/6 mice were sacrificed using an approved method. Mice were originally from the Jackson immune-research laboratories (Bar Harbour, ME). All of our animals were maintained in a specific pathogen-free animal facility. Whole bone marrow cells were isolated by gentle flushing into 1x HBSS, 10mM Hepes solution (Sigma Aldrich) using a 27G syringe. Erythrocytes were lysed with 3 volumes of Ammonium Chloride (Stem Cell Technologies) and debris removed by filtering cell suspension through a 40 µM nylon cell strainer. SP staining was performed as previously described.Citation7 Briefly, total nucleated bone marrow cells (NBMCs) were resuspended in DMEM staining media at 1 × 106 cells per ml and incubated with 5 µg per ml Hoechst 33342 DNA binding dye (Sigma Aldrich) for 90 min at 37°C with mixing at 30 min intervals. Hoechst stained cells were resuspended in DMEM, 2% FCS, and 10 mM HEPES solution at approximately 1 × 107 cells/ml. Cells were maintained at 4°C until analysis/sorting and during antibody labeling. Lower SP cells were sorted directly into 1.5 ml eppendorf tubes containing either 1x PBS, or RLT cell lysis buffer (Qiagen) for single RNA extraction or Stemspan Serum-Free Expansion Media (SFEM, Stem Cell Technologies) for ex-vivo culture. Approximately 5 × 106 Hoechst stained NBMCs were analyzed for HSC immunophenotype by SP;KLS;CD150,CD48. Antibody labeling was performed as previously describedCitation4 using the following antibodies: CD150, phycoerythrin (PE, Biolegend); Sca-1, PE/Cyanine7 (PE/Cy7, Biolegend); CD 117 (c-Kit),allophycocyanine/Cy7 (APC/Cy7, eBioscience); and Biotinylated Lineage antibody cocktail (anti-CD5, anti-CD11b, anti-B220, anti–Gr-1, and anti–Ter-119), subsequently labeled with Percp-conjugated streptavidin (SA-Percp, BD Biosciences). An amount of 2 µg/ml Propidium iodide (PI, Sigma Aldrich) was added to resuspension media to facilitate the exclusion of dead cells during cell sorting on the Moflo cell sorter (Beckman Coulter) and NBMCs analysis on the LSRII (BD Biosciences). PI was substituted for 1:1000 Dapi (Sigma Aldrich) for post sort analysis on the Cyan ADP (Beckman Coulter).
Ex vivo culture and aza-cytidine treatment of primary SP-HSCs
Lower SP cells were purity sorted into SFEM and cultured as previously describedCitation27 but with minor adjustments to cytokine concentrations. Cells were incubated at 37°C and 5% CO2, in a round bottom 96 well plate (corning) at 1000–2000 cells per 200 µl SFEM plus 2 µg Heparin and cytokines (3 ng recombinant mouse SCF, 6 ng recombinant mouse TPO, 6 ng recombinant mouse IGF-II, 3 ng recombinant human FGF-I, and 30 ng recombinant mouse ANGPTL3). For 5-Aza'dC induced hypomethylation, ex vivo cultured cells were treated with two doses of 0–2 µM 5-Aza'dC. The first dose was applied approximately 18 h after culture was initiated. Cells were maintained for a further 30 h after which 50% of media was replaced with fresh 100 µl SFEM plus 1 µg Heparin and 33.3% of the original cytokine concentrations listed above. 50% of initial 5-Aza’dC dose was added to cells 18 h after media replacement and ex vivo culture was continued for another 30 h. LSP cells were cultured ex vivo for a total of 4 d.
DNA isolation and methylation analysis
An amount of 150–300 ng of DNA was isolated from 7–8 × 104 LSP cells in RLT lysis buffer. Thirty to forty Young mice and 3-10 Mid, or Old mice were pooled per sample. DNA and RNA were simultaneously extracted from pooled LSPs using the AllPrep DNA/RNA Kit from Qiagen according to manufacturer’s instructions. Methylation analysis of extracted DNA was performed by Nano-MeDIP-seq as previously described.Citation21MeDIP libraries of 190–200 bp was prepared for three biological replicates each for 2, 12, and 24 mo old mice and subjected to 36 bp paired end (PE) sequencing on either the Illumina GA IIx (“Old 1,” “Old 2”, and “Young 1”) or the Illumina Hi-seq platform for all other samples. Pyro-sequencing was used for validation. Briefly, 1 × 104 cells per sample were bisulfite converted using EpiTect Plus LyseAll Bisulfite Kit and bisulfite conversion efficiency was found to be at least 99% for all samples as determined by qPCR. Control reactions on unmethylated and in vitro methylated samples were also conducted to confirm a linear detection of methylation levels by pyro-sequencing. Pyro-sequencing and PCR amplification of candidate regions were conducted using the PyroMark assay design software and PyroMark PCR kit respectively. Pyro-sequencing was performed using the PyroMark Gold Q96 reagents and the PyroMark Q96 MD Pyro-sequencer. All materials used for Pyro-sequencing were from Qiagen, except for primers, which were obtained from Sigma. All methods were performed according to manufacturer’s instructions.
RNA purification and transcript analysis
Differential expression of candidate genes was analyzed by quantitative reverse transcriptase- PCR (qRT-PCR). Here, 0.5–1 × 10−4 cells per sample were converted to cDNA using the SuperScript® III CellsDirectcDNA Synthesis Kit (Invitrogen). qRT-PCR of individual transcripts was then performed using Taq-Man gene expression assays (Applied Biosystems). All steps were completed according to manufacturer’s instructions. Gene expression was normalized to β-Actin housekeeping gene and fold change (FC) was calculated by the ΔΔCt method.
MeDIP-seq data analysis
The generated MeDIP-seq data were analyzed using our computational pipeline MeDUSACitation20 v1.0.0 and the MEDIPSCitation20,Citation36 v1.0.0 R Bioconductor package. MeDUSA constitutes several discrete stages of analysis. Briefly, BWA v0.5.8Citation37 was used to align the paired end sequence data to the reference mouse genome build (NCBIM37) using default settings. Filtering was performed to remove reads failing to map as a proper pair and those pairs in which neither read scored an alignment score of ≥ 10. In cases of non-unique reads, all but one pair was removed. Quality control was performed using the tool FastQC v0.9.4 (http://www.bioinformatics.bbsrc.ac.uk/projects/fastqc/) and MEDIPS. Additionally between replicate genome-wide correlations were calculated using QCSeqs from the Useq package.Citation38 Correlations were calculated using a window size of 500 bp, increasing in 250 bp increments. A minimum number of 5 reads in a window was required prior to inclusion in the correlation. DMRs were called using the Bioconductor package DESeq.Citation39DESeq was run with the estimateDispersions sharing mode set as ‘fit-only’. Only regions containing a minimum of 10 reads summed from the cohorts being compared were included for DMR analysis (termed our bump-list).
In order to identify regions of the genome enriched for DMRs, permutation analysis was performed. Our DMRs were placed in 100 kb windows across the genome and the most enriched location was identified and the relevant DMR count stored. 111 regions were randomly selected from the bump-list and placed in 100 kb windows. The windows were assessed to determine how often a region was found greater than our observed data. This was performed 1000 times in order to calculate an empirical p-value for our region of interest.
Genome-wide methylation scores were generated using the MEDIPS package. The paired reads were not extended and were placed in bins of 50 bp across the genome. The CpG coupling vector was generated with fragment lengths of 700 bp and using the “count” function. Circos v0.55Citation40 was used to generate a circular genome plot displaying these methylation scores. Feature annotation was obtained from Ensembl 62 and from the Ensembl Regulatory Build 67. CpG island shores were defined as extending 2000 bp upstream and downstream of an annotated CpG island. DMRs and bumps were placed in their relevant features using custom perl scripts and bedtoolsCitation41 v2.10.2. We used the Fishers Exact test to identify feature types enriched for DMRs.
Accession Numbers
The generated MeDIP-seq data was deposited into the GEO database under accession number GSE41658 .
Abbreviations: | ||
HSC | = | hematopoietic stem cell |
SP | = | side population |
LSP | = | lower side population |
SSCs | = | somatic stem cells |
NBMCs | = | nucleated bone marrow cells |
DNAm | = | DNA methylation |
CGI | = | CpG island |
MeDIP | = | methylated DNA immunoprecipitation |
MeDIP-seq | = | methylated DNA immunoprecipitation based sequencing |
DMR | = | differentially methylated region |
DMG | = | differentially methylated gene |
aDMR | = | age differentially methylated region |
qRT-PCR | = | quantitative reverse transcriptase polymerase chain reaction |
SFEM | = | Serum-Free Expansion Media |
Aza or 5-Aza’dC | = | 5-Aza’deoxyCytidine |
FDR | = | false discovery rate |
S.D | = | standard deviation |
FC | = | fold change |
PCRC2 | = | polycomb repressive complex -2 |
Additional material
Download Zip (224.7 KB)Acknowledgments
OT was supported by a PhD studentship from the UK Medical Research Council. The experimental part was supported by EU-FP7 project IDEAL-HEALTH-2010–259679. DP was supported by The Royal Society (RG2009/R1), EU-FP7 project IDEAL (259679), and the BBSRC (56102). Research in the Beck laboratory was supported by: Wellcome Trust (084071), Royal Society Wolfson Research Merit Award (WM100023) and EU-FP7 projects IDEAL (259679), EPIGENESYS (257082), and BLUEPRINT (282510). We thank all the staff of UCL Biological Service Unit, UCL Genomics as well as Dr Ayad Eddaoudi of the ICH FACS Laboratory for technical assistance.
Disclosure of Potential Conflicts of Interest
No potential conflicts of interest were disclosed.
Author Contributions
OT, DJP, and SB conceived the study. OT performed the experiments. GAW and WE did the bioinformatics and statistical analysis. OT, GAW, WE, and, TM analyzed data. DB, DJP, and SB contributed materials. OT, TA, and DJP performed FACS analysis. OT, SB, and DJP wrote the paper with contributions from all co-authors.
Supplemental Materials
Supplemental materials may be found here: www.landesbioscience.com/journals/epigenetics/article/26017
References
- Spangrude GJ, Heimfeld S, Weissman IL. Purification and characterization of mouse hematopoietic stem cells. Science 1988; 241:58 - 62; http://dx.doi.org/10.1126/science.2898810; PMID: 2898810
- Kim M, Moon HB, Spangrude GJ. Major age-related changes of mouse hematopoietic stem/progenitor cells. Ann N Y AcadSci 2003; 996:195 - 208; http://dx.doi.org/10.1111/j.1749-6632.2003.tb03247.x; PMID: 12799297
- Morrison SJ, Wandycz AM, Akashi K, Globerson A, Weissman IL. The aging of hematopoietic stem cells. Nat Med 1996; 2:1011 - 6; http://dx.doi.org/10.1038/nm0996-1011; PMID: 8782459
- Pearce DJ, Anjos-Afonso F, Ridler CM, Eddaoudi A, Bonnet D. Age-dependent increase in side population distribution within hematopoiesis: implications for our understanding of the mechanism of aging. Stem Cells 2007; 25:828 - 35; http://dx.doi.org/10.1634/stemcells.2006-0405; PMID: 17158238
- Rossi DJ, Bryder D, Zahn JM, Ahlenius H, Sonu R, Wagers AJ, Weissman IL. Cell intrinsic alterations underlie hematopoietic stem cell aging. ProcNatlAcadSci U S A 2005; 102:9194 - 9; http://dx.doi.org/10.1073/pnas.0503280102; PMID: 15967997
- Sudo K, Ema H, Morita Y, Nakauchi H. Age-associated characteristics of murine hematopoietic stem cells. J Exp Med 2000; 192:1273 - 80; http://dx.doi.org/10.1084/jem.192.9.1273; PMID: 11067876
- Goodell MA, Brose K, Paradis G, Conner AS, Mulligan RC. Isolation and functional properties of murine hematopoietic stem cells that are replicating in vivo. J Exp Med 1996; 183:1797 - 806; http://dx.doi.org/10.1084/jem.183.4.1797; PMID: 8666936
- Goodell MA, Rosenzweig M, Kim H, Marks DF, DeMaria M, Paradis G, Grupp SA, Sieff CA, Mulligan RC, Johnson RP. Dye efflux studies suggest that hematopoietic stem cells expressing low or undetectable levels of CD34 antigen exist in multiple species. Nat Med 1997; 3:1337 - 45; http://dx.doi.org/10.1038/nm1297-1337; PMID: 9396603
- Chambers SM, Shaw CA, Gatza C, Fisk CJ, Donehower LA, Goodell MA. Aging hematopoietic stem cells decline in function and exhibit epigenetic dysregulation. PLoSBiol 2007; 5:e201; http://dx.doi.org/10.1371/journal.pbio.0050201; PMID: 17676974
- Suzuki MM, Bird A. DNA methylation landscapes: provocative insights from epigenomics. Nat Rev Genet 2008; 9:465 - 76; http://dx.doi.org/10.1038/nrg2341; PMID: 18463664
- Bröske AM, Vockentanz L, Kharazi S, Huska MR, Mancini E, Scheller M, Kuhl C, Enns A, Prinz M, Jaenisch R, et al. DNA methylation protects hematopoietic stem cell multipotency from myeloerythroid restriction. Nat Genet 2009; 41:1207 - 15; http://dx.doi.org/10.1038/ng.463; PMID: 19801979
- Challen GA, Sun D, Jeong M, Luo M, Jelinek J, Berg JS, Bock C, Vasanthakumar A, Gu H, Xi Y, et al. Dnmt3a is essential for hematopoietic stem cell differentiation. Nat Genet 2011; 44:23 - 31; http://dx.doi.org/10.1038/ng.1009; PMID: 22138693
- Trowbridge JJ, Snow JW, Kim J, Orkin SH. DNA methyltransferase 1 is essential for and uniquely regulates hematopoietic stem and progenitor cells. Cell Stem Cell 2009; 5:442 - 9; http://dx.doi.org/10.1016/j.stem.2009.08.016; PMID: 19796624
- Beck S. Taking the measure of the methylome. Nat Biotechnol 2010; 28:1026 - 8; http://dx.doi.org/10.1038/nbt1010-1026; PMID: 20944589
- Bock C, Tomazou EM, Brinkman AB, Müller F, Simmer F, Gu H, Jäger N, Gnirke A, Stunnenberg HG, Meissner A. Quantitative comparison of genome-wide DNA methylation mapping technologies. Nat Biotechnol 2010; 28:1106 - 14; http://dx.doi.org/10.1038/nbt.1681; PMID: 20852634
- Butcher LM, Beck S. AutoMeDIP-seq: a high-throughput, whole genome, DNA methylation assay. Methods 2010; 52:223 - 31; http://dx.doi.org/10.1016/j.ymeth.2010.04.003; PMID: 20385236
- Down TA, Rakyan VK, Turner DJ, Flicek P, Li H, Kulesha E, Gräf S, Johnson N, Herrero J, Tomazou EM, et al. A Bayesian deconvolution strategy for immunoprecipitation-based DNA methylome analysis. Nat Biotechnol 2008; 26:779 - 85; http://dx.doi.org/10.1038/nbt1414; PMID: 18612301
- Harris RA, Wang T, Coarfa C, Nagarajan RP, Hong C, Downey SL, Johnson BE, Fouse SD, Delaney A, Zhao Y, et al. Comparison of sequencing-based methods to profile DNA methylation and identification of monoallelic epigenetic modifications. Nat Biotechnol 2010; 28:1097 - 105; http://dx.doi.org/10.1038/nbt.1682; PMID: 20852635
- Weber M, Davies JJ, Wittig D, Oakeley EJ, Haase M, Lam WL, Schübeler D. Chromosome-wide and promoter-specific analyses identify sites of differential DNA methylation in normal and transformed human cells. Nat Genet 2005; 37:853 - 62; http://dx.doi.org/10.1038/ng1598; PMID: 16007088
- Wilson GA, Dhami P, Feber A, Cortázar D, Suzuki Y, Schulz R, Schär P, Beck S. Resources for methylome analysis suitable for gene knockout studies of potential epigenome modifiers. Gigascience 2012; 1:3; http://dx.doi.org/10.1186/2047-217X-1-3; PMID: 23587164
- Taiwo O, Wilson GA, Morris T, Seisenberger S, Reik W, Pearce D, Beck S, Butcher LM. Methylome analysis using MeDIP-seq with low DNA concentrations. Nat Protoc 2012; 7:617 - 36; http://dx.doi.org/10.1038/nprot.2012.012; PMID: 22402632
- Beerman I, Bock C, Garrison BS, Smith ZD, Gu H, Meissner A, Rossi DJ. Proliferation-dependent alterations of the DNA methylation landscape underlie hematopoietic stem cell aging. Cell Stem Cell 2013; 12:413 - 25; http://dx.doi.org/10.1016/j.stem.2013.01.017; PMID: 23415915
- Hogart A, Lichtenberg J, Ajay SS, Anderson S, Margulies EH, Bodine DM, NIH Intramural Sequencing Center. Genome-wide DNA methylation profiles in hematopoietic stem and progenitor cells reveal overrepresentation of ETS transcription factor binding sites. Genome Res 2012; 22:1407 - 18; http://dx.doi.org/10.1101/gr.132878.111; PMID: 22684279
- Ji H, Ehrlich LI, Seita J, Murakami P, Doi A, Lindau P, Lee H, Aryee MJ, Irizarry RA, Kim K, et al. Comprehensive methylome map of lineage commitment from haematopoietic progenitors. Nature 2010; 467:338 - 42; http://dx.doi.org/10.1038/nature09367; PMID: 20720541
- Feber A, Wilson GA, Zhang L, Presneau N, Idowu B, Down TA, Rakyan VK, Noon LA, Lloyd AC, Stupka E, et al. Comparative methylome analysis of benign and malignant peripheral nerve sheath tumors. Genome Res 2011; 21:515 - 24; http://dx.doi.org/10.1101/gr.109678.110; PMID: 21324880
- Pasini D, Bracken AP, Hansen JB, Capillo M, Helin K. The polycomb group protein Suz12 is required for embryonic stem cell differentiation. Mol Cell Biol 2007; 27:3769 - 79; http://dx.doi.org/10.1128/MCB.01432-06; PMID: 17339329
- Zhang CC, Kaba M, Ge G, Xie K, Tong W, Hug C, Lodish HF. Angiopoietin-like proteins stimulate ex vivo expansion of hematopoietic stem cells. Nat Med 2006; 12:240 - 5; http://dx.doi.org/10.1038/nm1342; PMID: 16429146
- Pearce DJ, Ridler CM, Simpson C, Bonnet D. Multiparameter analysis of murine bone marrow side population cells. Blood 2004; 103:2541 - 6; http://dx.doi.org/10.1182/blood-2003-09-3281; PMID: 14644998
- Fraga MF, Ballestar E, Paz MF, Ropero S, Setien F, Ballestar ML, Heine-Suñer D, Cigudosa JC, Urioste M, Benitez J, et al. Epigenetic differences arise during the lifetime of monozygotic twins. ProcNatlAcadSci U S A 2005; 102:10604 - 9; http://dx.doi.org/10.1073/pnas.0500398102; PMID: 16009939
- Gentilini D, Mari D, Castaldi D, Remondini D, Ogliari G, Ostan R, Bucci L, Sirchia SM, Tabano S, Cavagnini F, et al. Role of epigenetics in human aging and longevity: genome-wide DNA methylation profile in centenarians and centenarians’ offspring. Age (Dordr) 2012; Forthcoming http://dx.doi.org/10.1007/s11357-012-9463-1; PMID: 22923132
- Gonzalo S. Epigenetic alterations in aging. J ApplPhysiol 2010; 109:586 - 97; http://dx.doi.org/10.1152/japplphysiol.00238.2010; PMID: 20448029
- Heyn H, Li N, Ferreira HJ, Moran S, Pisano DG, Gomez A, Diez J, Sanchez-Mut JV, Setien F, Carmona FJ, et al. Distinct DNA methylomes of newborns and centenarians. ProcNatlAcadSci U S A 2012; 109:10522 - 7; http://dx.doi.org/10.1073/pnas.1120658109; PMID: 22689993
- Agherbi H, Gaussmann-Wenger A, Verthuy C, Chasson L, Serrano M, Djabali M. Polycomb mediated epigenetic silencing and replication timing at the INK4a/ARF locus during senescence. PLoS One 2009; 4:e5622; http://dx.doi.org/10.1371/journal.pone.0005622; PMID: 19462008
- Gustincich S, Schneider C. Serum deprivation response gene is induced by serum starvation but not by contact inhibition. Cell Growth Differ 1993; 4:753 - 60; PMID: 8241023
- Hansen CG, Bright NA, Howard G, Nichols BJ. SDPR induces membrane curvature and functions in the formation of caveolae. Nat Cell Biol 2009; 11:807 - 14; http://dx.doi.org/10.1038/ncb1887; PMID: 19525939
- Chavez L, Jozefczuk J, Grimm C, Dietrich J, Timmermann B, Lehrach H, Herwig R, Adjaye J. Computational analysis of genome-wide DNA methylation during the differentiation of human embryonic stem cells along the endodermal lineage. Genome Res 2010; 20:1441 - 50; http://dx.doi.org/10.1101/gr.110114.110; PMID: 20802089
- Li H, Durbin R. Fast and accurate short read alignment with Burrows-Wheeler transform. Bioinformatics 2009; 25:1754 - 60; http://dx.doi.org/10.1093/bioinformatics/btp324; PMID: 19451168
- Nix DA, Courdy SJ, Boucher KM. Empirical methods for controlling false positives and estimating confidence in ChIP-Seq peaks. BMC Bioinformatics 2008; 9:523; http://dx.doi.org/10.1186/1471-2105-9-523; PMID: 19061503
- Anders S, Huber W. Differential expression analysis for sequence count data. Genome Biol 2010; 11:R106; http://dx.doi.org/10.1186/gb-2010-11-10-r106; PMID: 20979621
- Krzywinski M, Schein J, Birol I, Connors J, Gascoyne R, Horsman D, Jones SJ, Marra MA. Circos: an information aesthetic for comparative genomics. Genome Res 2009; 19:1639 - 45; http://dx.doi.org/10.1101/gr.092759.109; PMID: 19541911
- Quinlan AR, Hall IM. BEDTools: a flexible suite of utilities for comparing genomic features. Bioinformatics 2010; 26:841 - 2; http://dx.doi.org/10.1093/bioinformatics/btq033; PMID: 20110278