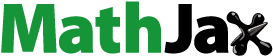
Abstract
Many human diseases are multifactorial, involving multiple genetic and environmental factors impacting on one or more biological pathways. Much of the environmental effect is believed to be mediated through epigenetic changes. Although many genome-wide genetic and epigenetic association studies have been conducted for different diseases and traits, it is still far from clear to what extent the genomic loci and biological pathways identified in the genetic and epigenetic studies are shared. There is also a lack of statistical tools to assess these important aspects of disease mechanisms. In the present study, we describe a protocol for the integrated analysis of genome-wide genetic and epigenetic data based on permutation of a sum statistic for the combined effects in a locus or pathway. The method was then applied to published type 1 diabetes (T1D) genome-wide- and epigenome-wide-association studies data to identify genomic loci and biological pathways that are associated with T1D genetically and epigenetically. Through combined analysis, novel loci and pathways were also identified, which could add to our understanding of disease mechanisms of T1D as well as complex diseases in general.
Introduction
Complex human diseases involve both genetic and environmental factors. Much progress has been made in revealing genetic loci associated with complex diseases in recent years, particularly through genome-wide association studies (GWAS). In comparison, the molecular mechanisms behind potential environmental effects for a complex disease are less clear. It has been suggested that environmental risk factors may impact on the disease risk of an individual through epigenetic modifications of DNA and histones.Citation1,Citation2 Although a large number of epigenome-wide association studies (EWAS) have to date been performed to identify such DNA methylation modifications in the genome, the results were only confirmed by independent replications in two cases.Citation3,Citation4 In the first case, Rakyan et al.Citation3 identified disease-associated methylation signals from a genome-wide DNA methylation scan of purified CD14+ monocytes from 15 T1D-discordant monozygotic twin pairs. Some of the association signals were not only replicated but also demonstrated to be present before disease diagnosis, thus indicating an etiological role of the epigenetic variations.Citation3 Liu et al.Citation4 identified two clusters within the major histocompatibility complex (MHC) region to be associated with risk of RA from genome-wide DNA methylation variations of a total of 354 cases and 337 controls, with both clusters showing evidence of association at replication.
One interesting observation from both studies is that some of the confirmed disease-associated epigenetic loci were also genetically associated with the disease, e.g., the MHC region for RA and T1D.Citation3,Citation4 In other words, both DNA sequence variations and DNA methylation variations in certain regions in the genome were found to be important for the etiology of the diseases. This observation leads to a more general question of to what extent environmental factors, through epigenetic changes, affect the same genomic regions and biological pathways that have been identified via genetic association studies. To answer such questions, an integrated approach needs to be developed to assess individual regions and biological pathways using both genetic and epigenetic data. In this investigation, we described a simple protocol based on detecting the combined effects of multiple genetic or epigenetic markers (in a region or pathway) for this purpose. We used the Rakyan et al. EWAS data as well as the corresponding T1D GWAS data generated by the Wellcome Trust Case Control Consortium (WTCCC)Citation5 to test the applicability of the method. We found shared genetic and epigenetic loci and biological pathways as well as unique epigenetic pathways for T1D and, through combined analysis of GWAS and EWAS data, we identified potential novel T1D risk loci.
Results
Shared genetic and epigenetic loci associated with T1D
First, combined epigenetic association analysis was performed to examine whether the known genetic loci associated with T1D were also associated with the disease as a group. For the 32 such loci with DNA methylation data in our data set (), no significant association was observed, suggesting that the majority of the genetic loci are not the targets of disease-associated DNA methylation modifications.
Table 1. Association of known T1D genetic loci with DNA methylation variation
Individual genetic loci were, however, identified, whose DNA methylation states were found to be associated with the disease. These included the HLA region (P = 2.6 × 10−4), in consistency with the findings of the original studies.Citation3 The association of GAD2 with T1D in the Raykan et al. EWAS data was also confirmed by the combined effect analysis (P = 0.0014), but it was not found to be significantly associated with T1D in the WTCCC GWAS data (P = 0.50). Through assessment of combined effects, additional genetic loci were identified to be associated with T1D epigenetically, with PGM1 (Phosphoglucomutase 1) as the most interesting candidate, whose encoded protein is one of the PGM isozymes, which catalyze the transfer of phosphate between the 1 and 6 positions of glucose, and is therefore an important component in the breakdown and synthesis of glucose. In the EWAS data, the methylation changes in the PGM1 locus were found to be strongly associated with T1D (P = 6.5 × 10−4). Two other loci, CD226 and ORMDL3 were also found to be associated with T1D with P = 0.015 and 0.042, respectively. As the Rakyan et al. study only had four additional twin pairs for validation, they were not used as an independent sample set to replicate the results because of insufficient statistical power. Instead, these four twin pairs were combined with the initial 15 twin pairs to form an augmented set of EWAS data, and separate combined effect tests were performed (). In the augmented data, CD226 and ORMDL3 were no longer significantly associated with T1D (). The strength of association for PGM1 was also reduced although it remained to be associated with the disease (P = 0.03). AFF3 (AF4/FMR2 Family, member 3) locus, however, was found to be a potential epigenetic candidate in the augmented EWAS data (P = 0.05) whereas it was not significant in the discovery samples alone (P = 0.063).
Combined analysis of EWAS and GWAS data identified novel candidate T1D risk loci
Just as it is interesting to examine whether a region where sequence variation is known to be associated with a disease is also a region where DNA methylation variation is important, the reverse is equally interesting. For the T1D EWAS data, out of the 15 593 loci containing methylation data, there were 25 and 623 loci found to be associated with T1D with P ≤ 0.001 and P ≤ 0.05, respectively. Only 317 out of the 623 loci remained significant (P ≤ 0.05) in the augmented EWAS data (the 15 initial twin pairs from the discovery stage plus the 4 additional twin pairs from the validation stage), with again 25 loci reaching P ≤ 0.001. First, we conducted a group analysis of these 25 and 317 loci in the WTCCC GWAS data, which contain SNP genotype information for 16 of the 25, and 204 of the 317 loci. No significant association with T1D was observed with either group. It is, however, possible that individual loci may be identified that are associated with the disease both genetically and epigenetically. For this purpose, we used the WTCCC GWAS data as the screening data and T1D GWAS data generated by T1DGCCitation6 as an independent data set to validate the findings.
The list of genome-wide SNP trend test P values from both the WTCCC and T1DGC studies were subject to a gene based test using the VEGAS program.Citation7 For both data sets, results were obtained for 258 of the 317 loci associated with the disease in the Rakyan et al. EWAS data. Of these, 30 and 20 had P ≤ 0.05 in the WTCCC and T1DGC data, respectively. As shown in , there were a total of 8 loci having locus-wise P ≤ 0.05 in the EWAS data and the two GWAS data sets, including HLA-DQB1 and PGM1. HMGN4 (high mobility group nucleosomal binding domain 4) and FAM109A (family with sequence similarity 109, member A) were found highly associated with T1D in the WTCCC and T1DGC GWAS samples (). The observed associations with the disease, however, may have been due to association with neighboring loci—HMGN4 is very close to the MHC region and FAM109A is next to the SH2B3 locus, both known T1D risk loci. The observation that they were found to be associated with T1D epigenetically was nevertheless interesting, although how they are involved in disease etiology remains unclear. The protein encoded by HMGN4 is thought to reduce the compactness of the chromatin fiber in nucleosomes, thereby enhancing transcription from chromatin templates,Citation8,Citation9 while FAM109A encodes a protein that localizes to the endosome and plays a role in endocytic trafficking.Citation10,Citation11
Table 2. Loci associated with T1D epigenetically with evidence of genetic association
There were four other loci, ARIH1 (ariadne homolog, ubiquitin-conjugating enzyme E2 binding protein, 1) on chromosome 15, EIF1 (eukaryotic translation Initiation factor 1) on chromosome 17, SLC1A5 (solute carrier family 1 [neutral amino acid transporter], member 5) and CA11 (carbonic anhydrase XI) on chromosome 19, were found to be modestly associated with T1D genetically and epigenetically (). EIF1 encodes a known protein translation initiation factor, whereas the protein encoded by ARIH1 is thought to maybe also play a role in protein translation.Citation12 SLC1A5 protein has a broad substrate specificity and acts as a cell surface receptor for feline endogenous virus RD114, baboon M7 endogenous virus and type D simian retroviruses.Citation13 Polymorphisms in SLC1A5 have been reported to be associated with diseases, such as Hartnup disorder and schizophrenia.Citation14,Citation15
Shared genetic and epigenetic pathways in T1D
In GWAS studies, it is now understood that there are many genetic variants in the genome that are associated with a disease but, due to their small effect sizes, they have not been identified individually. Their combined effects, however, may be detected through biological pathway based analysis of the GWAS data.Citation16,Citation17 This may also hold true for EWAS data, especially if the sample size is very small, as in the present study. To test this possibility and identify potential epigenetically important pathways, a total of 211 biological pathways obtained from the KEGG databaseCitation18 were assessed for their combined effects on T1D using the present GWAS and EWAS data.
There were no biological pathways identified to be associated with T1D with P ≤ 0.05, but four at a relaxed threshold of P ≤ 0.1, i.e., “Pentose phosphate pathway” (P = 0.063), “Lysine biosynthesis” (P = 0.064), “ECM-receptor interaction” (P = 0.076) and “Type I diabetes mellitus” (P = 0.091). Among the four pathways, “Type I diabetes mellitus” seemed to be the most interesting, as it might be identified as a T1D-associated pathway both genetically and epigenetically. In the biological pathway analysis using WTCCC GWAS data, the “Type I diabetes mellitus” pathway is indeed highly significantly associated with the disease, with p value equal to zero (). A closer examination of the EWAS results, however, indicated that the weak epigenetic association of this pathway in the EWAS data was driven primarily by the HLA loci and the GAD2 locus, as the association was no longer observed after they were excluded. Furthermore, in the augmented EWAS data (with the four additional twin pairs from the validation stage included), the strength of association of this pathway was weakened, rather than enhanced, to P = 0.21 (). The main exception in the pathway was the HLA-DQB1 locus, whose association was further strengthened in the augmented EWAS data as described above. The results, thus, did not provide evidence for this biological pathway as a whole to have both genetic and epigenetic variations that are important for disease etiology.
Figure 1. Biological pathway analyses of the GWAS (WTCCC) and EWAS data. Significance of the 211 KEGG pathways was assessed using 10 000 permutation of the individual level data based on the combined effects of all the SNPs (GWAS) or CpG sites (EWAS) located within individual pathways. (A) Pathways identified in the discovery EWAS data of the 15 twin pairs; (B) pathways identified in the augmented EWAS data; (C) pathways identified in the WTCCC GWAS data. For P values equal or close to zero, an alternative value of 1 × 10−10 [-log(p) = 10] was used for representing purposes.
![Figure 1. Biological pathway analyses of the GWAS (WTCCC) and EWAS data. Significance of the 211 KEGG pathways was assessed using 10 000 permutation of the individual level data based on the combined effects of all the SNPs (GWAS) or CpG sites (EWAS) located within individual pathways. (A) Pathways identified in the discovery EWAS data of the 15 twin pairs; (B) pathways identified in the augmented EWAS data; (C) pathways identified in the WTCCC GWAS data. For P values equal or close to zero, an alternative value of 1 × 10−10 [-log(p) = 10] was used for representing purposes.](/cms/asset/c258cb1c-0e3c-40be-95b9-f288b2eff9e3/kepi_a_10926407_f0001.gif)
Out of the four pathways identified in the EWAS data of the 15 twin pairs, only the “ECM-receptor interaction” pathway remained weakly associated with T1D in the augmented EWAS data (P ≤ 0.1). More interestingly, its strength of association was enhanced from P = 0.076 to P = 0.053 (). This pathway was, however, not significantly associated with T1D in the WTCCC GWAS data (P = 0.32) (). According to the KEGG database, the extracellular matrix (ECM) consists of a complex mixture of structural and functional macromolecules, which are important in environmental information processing, signaling molecules and interactions. If confirmed by independent EWAS data, this may represent a novel pathway that interacts actively with particular disease-associated environmental factors through epigenetic mechanisms.
Discussion
The genetic variants in the genome of an individual are regarded as comprising the background susceptibility for a complex disease, while exposure to various environmental factors along the individual’s life course provides triggers for the development of such a disease.Citation1,Citation2 Epigenetic regulation, such as imprinting control mechanisms, is known to be very important for human development and disease implications.Citation19 As supported by increasing evidence, much of the environmental exposure is believed to be impacted on an individual through his/her epigenome.Citation20 Unraveling the interplay between genetic, epigenetic and environmental factors for complex diseases and traits becomes increasingly important for understanding their causal mechanisms.Citation1,Citation2,Citation21
There are many different types of environmental factors, including social, economic and life style, as well as physical factors, such as air and water quality, and many of them are known to be associated with various complex traits and diseases. For example, smoking is known to be associated with a wide spectrum of complex diseases, such as cancersCitation22 and autoimmune diseases.Citation23 Life style and social economic conditions are also known to be associated with a wide spectrum of diseases and health problems, such as obesity.Citation24 These different types of environmental factors, however, are often confounding each other. It is therefore very likely that they may impact on both the same and different biological pathways of an individual. For a single complex disease, multifactorial environmental determinants are often the rule rather than exception. For example, both nutrition and viruses are long regarded as risk factors for T1D.Citation25 Heterogeneity is therefore expected among T1D patients in their epigenetic profile if their environmental exposure profile is different. This leads to the question of whether the often complex mixture of environmental risk factors, as manifested in epigenetic profile, impact on the same or different genes and biological pathways where the components of the background genetic susceptibility is located.
In this study, we described and adapted a simple statistical and computational protocol, under which analysis of genome-wide genetic and epigenetic data for complex diseases or traits can be integrated and combined. In the combined analysis of T1D GWAS and EWAS, candidate loci and biological pathways have been identified where DNA sequence variation and methylation variation may be both associated with the disease. “Type I diabetes mellitus” pathway is composed of important loci for the disease development, such as the HLA locus, it is therefore not surprising that it is one of the most important targets for genetic susceptibility, and some of its components may be also subject to environmental triggers and epigenetic changes. The observation that the “ECM-receptor interaction” pathway is weakly associated with the disease epigenetically but not genetically is much less expected. On the other hand, the epigenetic association of “ECM-receptor interaction” pathway with T1D may not be a total surprise, as it contains multiple signaling molecules that are involved in environmental information processing. If this pathway’s epigenetic association with the disease is confirmed in independent studies, it will provide new knowledge about disease mechanism, as it would suggest that specific epigenetic and environment-sensing loci (e.g., GAD2) and pathways are present.
The integrated analysis of genome-wide genetic and epigenetic analysis is potentially a powerful approach to identify not only important genetic and epigenetic loci and biological pathways, but also novel loci for a disease. Among loci that are associated both genetically and epigenetically, HLA-DQB1 and PGM1 (and perhaps also AFF3) are known disease risk loci, whereas loci such as SLC1A3 are potential novel disease associated loci. As more GWAS and EWAS data are generated for disease association studies, increasing number of novel risk loci may be identified in this way. For loci in regions of strong linkage disequilibrium (LD), such as the HMGN4 and FAM109A locus (close to the MHC region and SH2B3 gene, respectively), their genetic association with the diseases can be confounded by their LD with their neighboring known disease-associated loci. On the other hand, the combined analysis of genetic and epigenetic data (and potentially other “omics” data) may be a useful approach in dissecting the causal variants or narrow down their locations in such strong LD regions, such as the MHC and SH2B3 region, where causal variants are otherwise very difficult to pin down.
One limitation of the EWAS data used in the study is its small sample size and relative low coverage of DNA methylation sites across the genome. This obviously had important implications on the robustness of the findings as well as the observed scale and extent of the genetic-epigenetic correlations in disease-associated loci and biological pathways. Compared with GWAS, EWAS is still in its early stage with many study-design and data analysis issues to be addressed.Citation20,Citation26 But it is a fast developing field, and with the availability of increasing number of well-designed EWAS data sets, we believe that tools such as described in the present study will become more useful in revealing causal mechanisms of common complex diseases and traits.
Materials and Methods
Genome-wide association and methylation data for T1D
We use the GWAS data from phase I of the Wellcome Trust Case Control Consortium (WTCCC) study:Citation5 the case samples for type 1 diabetes (T1D), and control samples from the 1958 Birth Cohort study (58C) and British National Blood Service (NBS) all genotyped with Affymetrix Genechip Human Mapping 500K SNP array. Samples and SNPs with low genotyping quality were removed as described by the WTCCC. The final sample sets contained 1963 T1D patients, as well as a total of 2938 controls (1480 58C and 1458 NBS samples). Individual level data were downloaded from the European Genome-phenome Archive (EGA) database (https://www.ebi.ac.uk/ega/).
Independent genome-wide association data were generated by the Type 1 Diabetes Genetic Consortium (T1DGC),Citation6 which included 4041 T1D cases and 2604 controls from the British 1958 birth cohort, genotyped using Illumina HumanHap 550. For this data set, only summary analysis, such as trend-test P values across the genome were downloaded for gene-based validation purposes from the dbGaP database (http://www.ncbi.nlm.nih.gov/gap).
The genome-wide DNA methylation data for T1D were generated and published by Rakyan et al.Citation3 A total of 15 discordant monozygotic twin pairs were included, and genome-wide DNA methylation assay was performed using the Illumina Infinium HumanMethylation27 BeadChip. After normalization and data cleaning, a total 22 645 probes were available with β-values (range 0–1) produced as approximate representations of the absolute methylation percentage of specific CpG sites within the sample population. We used these β-values, provided by the authors, in the present study.
For validation purposes, Rakyan et al. included in their study 4 additional T1D-discordant monozygotic twin pairs assayed with the same genome-wide methylation array.Citation3 In this study, these 4 twin pairs were added to the original 15 twin pairs to form an augmented EWAS data set with analysis performed to examine whether any association of loci or pathways with the disease remained or improved.
All the EWAS and GWAS data sets and relevant analyses as described below were presented as a flowchart in .
Figure 2. Flowchart of EWAS and GWAS data sets and analysis procedures. Individual level genome-wide methylation data of a total of 19 monozygotic twin (MZ) pairs, discordant in T1D disease status, were available for the combined effect analysis either at locus-level or across individual biological pathways. Individual level genotype from the WTCCC-T1D GWAS (1963 T1D cases vs. 2938 controls) were also subject to similar analysis. For the T1DGC GWAS study (4041 T1D cases vs. 2604 controls), only genome-wide summary results were available. The list of genome-wide trend test P values of individual SNPs from both the T1DGC and WTCCC studies were used to generate locus level effect using the VEGAS program for cross-validation.
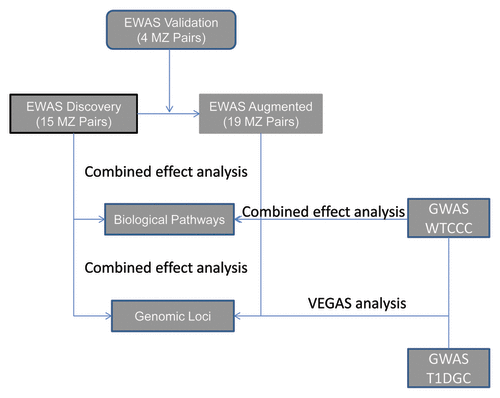
Bioinformatics analysis
For both GWAS and EWAS data, SNPs and probes were mapped to individual gene regions based on the annotation information downloaded from Ensembl (http://www.ensembl.org), and the probe annotation information provided by Illumina. For the biological pathway analysis, the relevant information was obtained from the KEGG (Kyoto Encyclopedia of Genes and Genomes) pathway database (http://www.genome.jp/kegg/pathway.html).Citation18 SNPs and Genes on the GWAS and EWAS data were then assigned to individual pathways accordingly.
A shared protocol to assess combined effects for GWAS and EWAS data
For both GWAS and EWAS data, modeling the correlation structure between SNPs (GWAS) and CpG methylation sites (EWAS) in any region needs to be taken into account when the combined effects of a group of loci or biological pathways are assessed. This can be addressed efficiently through permutational procedures, wherein the data set’s correlation structure is maintained while the sample status (e.g., case/control label) is permuted. A summary statistic, comparable across different types of data (e.g., GWAS vs. EWAS) is then subjected to permutational assessment using simulation. A well-known summary statistic measuring association is the sum of chi-square statistics, which can either be directly obtained (from a chi-square test) or transformed from other statistics such as a z-score, or other statistics related to non-parametric tests.
Once such test statistic is defined for a data set, permutation of the sample status can be performed to derive the permutated combined effect statistic’s values; these form an approximation to the null distribution of no association, and can then be fitted to standard statistical distributions, such as normal, skewed normal, or members of the general class of GAMLSS (generalized additive models for location, scale, and shape) distributions. Appropriate significance tests can then be conducted based on the null distribution, and the procedure can be applied to test associations among groups of genes or biological pathways, as further illustrated below.
Biological pathway analysis of the GWAS data
Our method of detecting the combined effects of all SNP main effects in a biological pathway is an adaptation of the method reported by Eleftherohorinou et al.Citation17 Briefly, for each individual SNP, a chi-square value from the trend test was generated using Plink.Citation27 Permutations were performed to assess the significance of the sum of such chi-square statistic values (Sst) for all the SNPs in a pathway, and the resulting null distribution of the summary statistic was approximated using the Sinh-Arcsinh (SHASH) probability distribution.Citation28 This model has four parameters, governing location (μ), scale (σ) and shape (ν,τ) and offers sufficient flexibility to fit a large number of empirical distributions; it can be easily fitted with the gamlss R package (http://www.gamlss.com).Citation28,Citation29 We generated 10 000 permutations of the sample case/control labels, and estimated the four parameters µ, σ, ν, τ in a SHASH model by maximizing the likelihood P(Sstk |µ,σ,ν,τ), k[1,10 000], where each Sstk was calculated as the sum statistic under a random permutation of the case control labels and k was the index of the permutation. The p-value of the sum statistic was then obtained as P =
, where Sst was the observed sum statistic of the real data and P(x|μ,σ,ν,τ) was the fitted SHASH distribution. This approach is an adaptation of the method described by Eleftherohorinou et al.,Citation17 but with the 3-parameter skew-normal distribution replaced by the more general 4-parameter SHASH model, which improves the fit to the permutational distribution ().
Figure 3. Fitting permuted pathway-wide combined epigenetic effects. The augmented T1D EWAS data were permuted 10 000 times, and for each permutation a combined effect value was obtained by summing over the squared z-score statistic values computed from individual methylation probes in an individual pathway. The data were then fitted using the skew-normal (solid line) distribution and the SHASH distribution (dashed line). For the example data shown, the AIC (Akaike’s information criterion) values were found to be 80 989 for the skewed normal and 80 961 for the SHASH models, respectively, indicating that an improved penalized fit was provided by the latter.
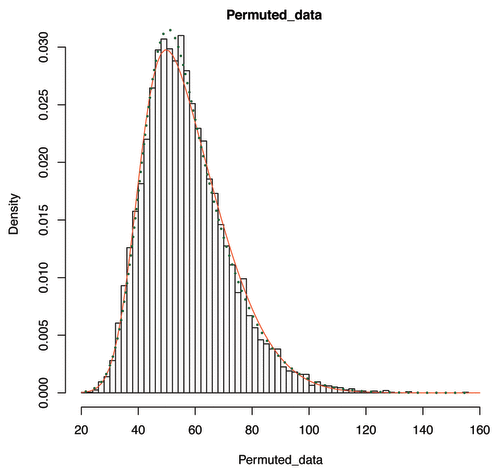
Biological pathway analysis of the EWAS data
Our method to assess the combined effects of DNA methylation variations in a biological pathway is similar with the method described above, except that the statistic to be summed over is not the chi-square statistic from a trend test of SNP genotypes, but is obtained by squaring a z-statistic computed from the EWAS data. For the present T1D data, as there were 15 monozygotic twins, a Wilcoxon signed-rank statistic W30 was computed and standardized as , where
and Nr is the non-zero pairs in the samples.Citation30
Gene group analysis of the GWAS and EWAS data
In a similar way as assessing the combined effects of all SNPs in an individual pathway, as described above, the combined effects of multiple SNPs or CpG sites within a single locus or within a group of loci can also be tested. For the present study, this strategy is particularly useful to examine the overlap between genetic and epigenetic variations for an underlying disease by assessing the combined effects of all DNA methylation variations within the group of genetic loci known to be associated with the same disease, i.e., T1D. The lists of such loci were obtained from the most recent literature publication ().Citation6
Acknowledgments
The University College London (UCL) Institute of Child Health receives a proportion of funding from the Department of Health's National Institute for Health Research Biomedical Research Centres funding scheme. Part of this work was undertaken at the Centre for Paediatric Epidemiology and Biostatistics, (UCL, London) which benefits from funding support from the MRC in its capacity as the MRC Centre of Epidemiology for Child Health. The Medical Research Council Centre of Epidemiology for Child Health is supported by funds from the UK Medical Research Council (grant G0400546). The authors also wish to thank the Wellcome Trust Case Control Consortium (WTCCC) and the Database of Genotypes and Phenotypes (dbGaP) for providing relevant genotyping data and summary GWAS analysis results in the study. The authors acknowledge the use of the UCL Legion High Performance Computer Facility (Legion@UCL), and associated support service, in the completion of this work.
Disclosure of Potential Conflicts of Interest
No potential conflicts of interest were disclosed.
References
- Jirtle RL, Skinner MK. Environmental epigenomics and disease susceptibility. Nat Rev Genet 2007; 8:253 - 62; http://dx.doi.org/10.1038/nrg2045; PMID: 17363974
- Petronis A. Epigenetics as a unifying principle in the aetiology of complex traits and diseases. Nature 2010; 465:721 - 7; http://dx.doi.org/10.1038/nature09230; PMID: 20535201
- Rakyan VK, Beyan H, Down TA, Hawa MI, Maslau S, Aden D, Daunay A, Busato F, Mein CA, Manfras B, et al. Identification of type 1 diabetes-associated DNA methylation variable positions that precede disease diagnosis. PLoS Genet 2011; 7:e1002300; http://dx.doi.org/10.1371/journal.pgen.1002300; PMID: 21980303
- Liu Y, Aryee MJ, Padyukov L, Fallin MD, Hesselberg E, Runarsson A, Reinius L, Acevedo N, Taub M, Ronninger M, et al. Epigenome-wide association data implicate DNA methylation as an intermediary of genetic risk in rheumatoid arthritis. Nat Biotechnol 2013; 31:142 - 7; http://dx.doi.org/10.1038/nbt.2487; PMID: 23334450
- Burton PR, Clayton DG, Cardon LR, Craddock N, Deloukas P, Duncanson A, et al, Wellcome Trust Case Control Consortium. Genome-wide association study of 14,000 cases of seven common diseases and 3,000 shared controls. Nature 2007; 447:661 - 78; http://dx.doi.org/10.1038/nature05911; PMID: 17554300
- Barrett JC, Clayton DG, Concannon P, Akolkar B, Cooper JD, Erlich HA, Julier C, Morahan G, Nerup J, Nierras C, et al, Type 1 Diabetes Genetics Consortium. Genome-wide association study and meta-analysis find that over 40 loci affect risk of type 1 diabetes. Nat Genet 2009; 41:703 - 7; http://dx.doi.org/10.1038/ng.381; PMID: 19430480
- Liu JZ, McRae AF, Nyholt DR, Medland SE, Wray NR, Brown KM, Hayward NK, Montgomery GW, Visscher PM, Martin NG, et al, AMFS Investigators. A versatile gene-based test for genome-wide association studies. Am J Hum Genet 2010; 87:139 - 45; http://dx.doi.org/10.1016/j.ajhg.2010.06.009; PMID: 20598278
- Ravasi T, Suzuki H, Cannistraci CV, Katayama S, Bajic VB, Tan K, Akalin A, Schmeier S, Kanamori-Katayama M, Bertin N, et al. An atlas of combinatorial transcriptional regulation in mouse and man. Cell 2010; 140:744 - 52; http://dx.doi.org/10.1016/j.cell.2010.01.044; PMID: 20211142
- Birger Y, Ito Y, West KL, Landsman D, Bustin M. HMGN4, a newly discovered nucleosome-binding protein encoded by an intronless gene. DNA Cell Biol 2001; 20:257 - 64; http://dx.doi.org/10.1089/104454901750232454; PMID: 11410162
- Vinayagam A, Stelzl U, Foulle R, Plassmann S, Zenkner M, Timm J, Assmus HE, Andrade-Navarro MA, Wanker EE. A directed protein interaction network for investigating intracellular signal transduction. Sci Signal 2011; 4:rs8; http://dx.doi.org/10.1126/scisignal.2001699; PMID: 21900206
- Swan LE, Tomasini L, Pirruccello M, Lunardi J, De Camilli P. Two closely related endocytic proteins that share a common OCRL-binding motif with APPL1. Proc Natl Acad Sci U S A 2010; 107:3511 - 6; http://dx.doi.org/10.1073/pnas.0914658107; PMID: 20133602
- Ardley HC, Tan NG, Rose SA, Markham AF, Robinson PA. Features of the parkin/ariadne-like ubiquitin ligase, HHARI, that regulate its interaction with the ubiquitin-conjugating enzyme, Ubch7. J Biol Chem 2001; 276:19640 - 7; http://dx.doi.org/10.1074/jbc.M011028200; PMID: 11278816
- Rasko JE, Battini JL, Gottschalk RJ, Mazo I, Miller AD. The RD114/simian type D retrovirus receptor is a neutral amino acid transporter. Proc Natl Acad Sci U S A 1999; 96:2129 - 34; http://dx.doi.org/10.1073/pnas.96.5.2129; PMID: 10051606
- Deng X, Sagata N, Takeuchi N, Tanaka M, Ninomiya H, Iwata N, Ozaki N, Shibata H, Fukumaki Y. Association study of polymorphisms in the neutral amino acid transporter genes SLC1A4, SLC1A5 and the glycine transporter genes SLC6A5, SLC6A9 with schizophrenia. BMC Psychiatry 2008; 8:58; http://dx.doi.org/10.1186/1471-244X-8-58; PMID: 18638388
- Potter SJ, Lu A, Wilcken B, Green K, Rasko JE. Hartnup disorder: polymorphisms identified in the neutral amino acid transporter SLC1A5. J Inherit Metab Dis 2002; 25:437 - 48; http://dx.doi.org/10.1023/A:1021286714582; PMID: 12555937
- Wang K, Li MY, Bucan M. Pathway-based approaches for analysis of genomewide association studies. Am J Hum Genet 2007; 81:1278 - 83; http://dx.doi.org/10.1086/522374; PMID: 17966091
- Eleftherohorinou H, Wright V, Hoggart C, Hartikainen A-L, Jarvelin M-R, Balding D, Coin L, Levin M. Pathway analysis of GWAS provides new insights into genetic susceptibility to 3 inflammatory diseases. PLoS One 2009; 4:e8068; http://dx.doi.org/10.1371/journal.pone.0008068; PMID: 19956648
- Kanehisa M, Goto S, Kawashima S, Okuno Y, Hattori M. The KEGG resource for deciphering the genome. Nucleic Acids Res 2004; 32:Database issue D277 - 80; http://dx.doi.org/10.1093/nar/gkh063; PMID: 14681412
- Schulz R, Proudhon C, Bestor TH, Woodfine K, Lin CS, Lin SP, Prissette M, Oakey RJ, Bourc’his D. The parental non-equivalence of imprinting control regions during mammalian development and evolution. PLoS Genet 2010; 6:e1001214; http://dx.doi.org/10.1371/journal.pgen.1001214; PMID: 21124941
- Rakyan VK, Down TA, Balding DJ, Beck S. Epigenome-wide association studies for common human diseases. Nat Rev Genet 2011; 12:529 - 41; http://dx.doi.org/10.1038/nrg3000; PMID: 21747404
- Ptak C, Petronis A. Epigenetics and complex disease: from etiology to new therapeutics. Annu Rev Pharmacol Toxicol 2008; 48:257 - 76; http://dx.doi.org/10.1146/annurev.pharmtox.48.113006.094731; PMID: 17883328
- Herbst RS, Heymach JV, Lippman SM. Lung cancer. N Engl J Med 2008; 359:1367 - 80; http://dx.doi.org/10.1056/NEJMra0802714; PMID: 18815398
- Costenbader KH, Karlson EW. Cigarette smoking and autoimmune disease: what can we learn from epidemiology?. Lupus 2006; 15:737 - 45; http://dx.doi.org/10.1177/0961203306069344; PMID: 17153844
- Reilly JJ, Armstrong J, Dorosty AR, Emmett PM, Ness A, Rogers I, Steer C, Sherriff A, Avon Longitudinal Study of Parents and Children Study Team. Early life risk factors for obesity in childhood: cohort study. BMJ 2005; 330:1357 - 9; http://dx.doi.org/10.1136/bmj.38470.670903.E0; PMID: 15908441
- Hewagama A, Richardson B. The genetics and epigenetics of autoimmune diseases. J Autoimmun 2009; 33:3 - 11; http://dx.doi.org/10.1016/j.jaut.2009.03.007; PMID: 19349147
- Down TA, Rakyan VK, Turner DJ, Flicek P, Li H, Kulesha E, Gräf S, Johnson N, Herrero J, Tomazou EM, et al. A Bayesian deconvolution strategy for immunoprecipitation-based DNA methylome analysis. Nat Biotechnol 2008; 26:779 - 85; http://dx.doi.org/10.1038/nbt1414; PMID: 18612301
- Purcell S, Neale B, Todd-Brown K, Thomas L, Ferreira MAR, Bender D, Maller J, Sklar P, de Bakker PI, Daly MJ, et al. PLINK: a tool set for whole-genome association and population-based linkage analyses. Am J Hum Genet 2007; 81:559 - 75; http://dx.doi.org/10.1086/519795; PMID: 17701901
- Jones MC, Pewsey A. Sinh-arcsinh distributions. Biometrika 2009; 96:761 - 80; http://dx.doi.org/10.1093/biomet/asp053
- Rigby RA, Stasinopoulos DM. Generalized additive models for location, scale and shape. J Roy Stat Soc C-App. 2005; 54:507 - 44; http://dx.doi.org/10.1111/j.1467-9876.2005.00510.x
- Wilcoxon F. Individual Comparisons by Ranking Methods. Biom Bull 1945; 1:80 - 3; http://dx.doi.org/10.2307/3001968