Abstract
Glioblastoma multiforme (GBM) is the most aggressive of all brain tumors, with a median survival of less than 1.5 years. Recently, epigenetic alterations were found to play key roles in both glioma genesis and clinical outcome, demonstrating the need to integrate genetic and epigenetic data in predictive models. To enhance current models through discovery of novel predictive biomarkers, we employed a genome-wide, agnostic strategy to specifically capture both methylation-directed changes in gene expression and alternative associations of DNA methylation with disease survival in glioma. Human GBM-associated DNA methylation, gene expression, IDH1 mutation status, and survival data were obtained from The Cancer Genome Atlas. DNA methylation loci and expression probes were paired by gene, and their subsequent association with survival was determined by applying an accelerated failure time model to previously published alternative and expression-based association equations. Significant associations were seen in 27 unique methylation/expression pairs with expression-based, alternative, and combinatorial associations observed (10, 13, and 4 pairs, respectively). The majority of the predictive DNA methylation loci were located within CpG islands, and all but three of the locus pairs were negatively correlated with survival. This finding suggests that for most loci, methylation/expression pairs are inversely related, consistent with methylation-associated gene regulatory action. Our results indicate that changes in DNA methylation are associated with altered survival outcome through both coordinated changes in gene expression and alternative mechanisms. Furthermore, our approach offers an alternative method of biomarker discovery using a priori gene pairing and precise targeting to identify novel sites for locus-specific therapeutic intervention.
Introduction
Glioblastoma multiforme (GBM) is the most aggressive of all brain tumors and accounts for approximately 70% of all malignant gliomas.Citation1 Despite current treatments, patients with GBM have a median survival of only 12–15 mo.Citation1 This disease is thought to result from the outgrowth of clonal populations that harbor a combination of complex somatic gene alterations.Citation1 Genetic alterations include dysregulation of many angiogenic and proliferative pathways, including amplification of EGFR and overexpression of VEGF.Citation1 In addition, dysregulation of many members of the PI(3)K /Akt/RAS signaling pathway have also been implicated in the disease.Citation1 In 2006, Phillips et al. used these genetic alterations, as well as copy number variations (CNV), to distinguish subclasses of GBM with prognostic implications.Citation2 These analyses were further supported by several studies that assessed known, prevalent mutations in GBMs (EGFR, PTEN, IDH1, TP53, and NF1), copy number alterations, and expression changes in an integrative approach in order to more precisely define GBM subtypes important for survival prediction. These data and approaches strongly support the hypothesis that GBMs harbor a complex combination of somatic alterations that determine their phenotype.Citation3,Citation4
Recently, Frattini et al. (2013) used a novel statistical approach to identify drivers of gliomagenesis through integration of somatic mutations and CNV.Citation5 They classified three types of GBM: (1) GBM having deletions at sites containing mutations, (2) GBM having amplifications at sites containing mutations, and (3) GBM with recurrent mutations and no alteration in CNV.Citation5 They also identified fusion products involving the EGFR-SEPT14 loci. Their integrative analysis further added to the genetic understanding of GBM pathogenesis and marked specific targets for possible therapeutic intervention.Citation5
Epigenetics (particularly DNA methylation) plays an important role in gliomagenesis and glioma survival. Gene promoter DNA methylation has long been associated with gene silencing. Research has now identified a role for methylation in selecting alternate transcripts and gene promoters, giving rise to somatic events that can affect disease survival.Citation6-Citation10 Our group and others have reported an association between isocitrate dehydrogenase 1 and 2 (IDH1/2) mutations and a hypermethylator phenotype in gliomas that is associated with early age of onset and increased patient survival, specifically in lower-grade gliomas and secondary GBM.Citation6,Citation11 Our data, which made use of The Cancer Genome Atlas (TCGA) population (a population independent of our original data set), also demonstrated an association between TP53 and G-CIMP, a lack of association between EGFR and G-CIMP, and an overall increase in methylation genome-wide.Citation6
DNA methylation does not act solely through the mediation of gene expression (the mechanism that we designate as an expression-based association). DNA methylation has also been found to associate with chromosomal instability, the induction of splice variants, alterations in enhancer regions, changes in microRNA binding regions and expression control regions, and mutations. These somatic changes (which we designate as an alternative association) could also greatly influence survival but are much less well studied.Citation6-Citation10
These reports have highlighted the crosstalk between various types of carcinogenic somatic alterations and the need for a better understanding of the complex nature of somatic gene inactivation patterns involving genetic and epigenetic alterations that impact both the genesis and survival rates of glioma. Although there has been a call for integrative biomarkers that can sharpen predictive tools, most research has focused solely on the integration of genetic alterations (e.g., mutations) and their association with survival.Citation5,Citation12,Citation13 Here, we have made use of TCGA data sets to test our bioinformatics-based approach for identifying novel biomarkers of phenotypically-important relationships between DNA methylation, gene expression, and survival in GBM.
Results
DNA methylation and gene expression are significantly associated in GBM samples
After removal of all IDH1 mutant samples and replicates to prevent survival bias, the final phase 1 and phase 2 data sets contained n = 73 and n = 168 samples, respectively. Patient demographic data for all 241 GBM samples are presented in . Expression and methylation loci were paired by gene symbol for all 241 samples, resulting in a total of 66 202 unique methylation and expression pairs, which were used for the following analysis. In order to ensure functionality of methylation loci in the following analysis, an initial screen was conducted to determine the association of methylation and expression within each gene. To identify the methylation loci that regulate gene expression level, a linear model, as specified in Equation 2 (see Materials and Methods), was performed using the combined phase 1 and phase 2 data sets (n = 241). Pairs were designated as significant if they had a q-value < 0.05. Out of all 66 202 corresponding loci for both expression and methylation, 9821 were found to be significantly associated with each other (84.3% negatively correlated, 15.7% positively correlated). Samples were then separated back into the original phase 1 (n = 73) and phase 2 (n = 168) sets for survival analysis.
Table 1. Patient demographic and tumor* characteristics
DNA methylation and gene expression pairs are significantly associated with patient survival in GBM samples
To determine which DNA methylation and gene expression pairs are not only significantly associated with each other, but also significantly associated with survival, a Cox proportional hazards model was run on phase 1, phase 2, and pooled data sets. We used the Cox model to investigate the effect of gene expression, DNA methylation, and their interaction term on survival, adjusting for age, gender, and study phase (phase 1 vs. phase 2). “Study” was included as a model variable as a precautionary measure due to the inherent difference in how the presence of IDH1 mutation was determined for each of the two data sets. Tumors with a G-CIMP phenotype or IDH mutation were removed from this analysis due to their association with increased survival in GBM patients. Analysis of the phase 1 data set (n = 73) yielded 878 pairs (from the original 9821) that were significantly associated with survival (P < 0.05). Those 878 pairs were re-run using the phase 2 data set (n = 168) using the same model, which revealed 100 pairs with P < 0.05. Finally, we assessed the effects of the 100 pairs on overall survival using the pooled data set (n = 241) (Supplemental Material, Table S1). Pairs that significantly correlated with survival were chosen based on the q-value (BH) of the pooled model (cutoff: q < 0.10). Thirty-six unique methylation/expression pairs from 29 genes were significantly associated with survival. Of these 36 unique pairs, CpG locus cg23134520 was found to contain a SNP (rs6032566) and was removed from further analysis. This yielded 35 unique methylation/expression pairs from 28 different genes, which were used for the final mediation analysis ().
Table 2. Final 35 DNA methylation/gene expression pairs that are significantly associated with survival
Association of methylated loci with survival can be decomposed into (1) those whose action is mediated through expression and (2) those whose association with survival is not mediated in this fashion
We first estimated the association of DNA methylation with survival mediated through its presumptive effect on gene expression (expression-based association) and then assessed the association not directly mediated through gene expression (alternative association). The expression-based and alternative associations of paired loci with survival were estimated for the top 35 unique methylation/expression pairs (chosen from the linear model and Cox proportional hazards model) by using an accelerated failure time (AFT) model (see Supplemental Material, Table S2). This analysis yielded 10 unique methylation/expression pairs in which expression-mediated methylation was associated with survival outcome (or significant expression-based associations) (), 13 methylation/expression pairs where methylation did not work through expression of the same gene to affect survival (significant alternative association) (), and 4 methylation/expression pairs where methylation exerted its effect on survival outcome directly and through gene expression modulation (both significant alternative and expression-based associations) (). Of the 27 significant methylation and expression pairs, 22 DNA methylation loci were located within a CpG island. In general, pairs within the same gene had similar effects on survival (). In addition, all but three of the locus pairs (associated with CACNB1, RFXANK, and RAB21) had negative correlations, suggesting that the majority of the methylation/expression pairs were inversely related (see Supplemental Material, Fig. S1). Exon locations of methylation loci from significant pairs can be seen in the supplementary material (Fig. S2).
Figure 1. Significant expression-based and alternative associations of DNA methylation on gene expression and survival. The 35 unique DNA methylation/gene expression pairs were subjected to an Accelerated Failure Time (AFT) survival model and applied to alternative and expression-based equations (2–5 in methods). This yielded a total of 27 significant methylation/expression pairs, 10 had significant expression-based associations (A), 13 had significant alternative associations (B), and 4 had both significant expression-based and alternative associations (C). Grey lines indicate alternative associations, black lines indicate expression-based associations, gray circles indicate that the methylation locus for that gene pair was found in a CpG island, and black circles indicate that the methylation locus for that gene pair was not found in a CpG island. The y-axis indicates the change in survival time per 5% increase in methylation; therefore, effects that fall above the line are associated with an increase in survival, and effects that fall below the line are associated with a decrease in survival.
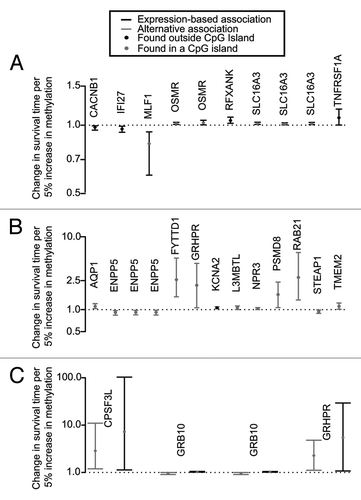
Discussion
The association of alterations in DNA methylation and gene expression in GBM with disease survival has been a major focus of recent studies, as it is apparent that outcome is not solely driven by somatic mutation. These previous studies generally identified loci whose methylation was inversely correlated with expression and examined the impact of those loci on patient outcome. Our study uniquely focused on methylation and attempted to classify the effects of methylation on survival into those mediated by expression and those not mediated by expression, thereby expanding the potential biomarker pool.
In 2013, Wang et al. used an integrative Bayesian analysis (iBAG) approach to analyze the association of DNA methylation with changes in gene expression and subsequently evaluated the association of changes in gene expression on GBM survival.Citation14 This linear approach resulted in the identification of several genes significantly associated with gene expression modulated by methylation. Consistent with these data, several genes that we showed were significantly modulated by DNA methylation, including OSMR, STEAP1, and GRB10, were also reported by Wang et al.Citation14 However, methylation not only exerts its effects on survival through expression of its associated gene, but can also operate through a variety of other mechanisms, including chromosomal fragility/instability, splicing variants, enhancer regions, and dysregulation of microRNA.Citation6-Citation10 Etcheverry et al. (2010) investigated the impact of DNA methylation on gene expression and outcome in GBM.Citation15 Their analysis focused on the relationship between DNA methylation and gene expression and the association of methylation with survival. They identified 421 CpG sites that were significantly inversely correlated between methylation and expression, 291 of which matched what we found to be correlated in our analysis. They also identified 13 genes that appeared to have consistently differential methylation and expression (between GBM and control brain) but were negatively correlated, suggesting that the regulation of these genes may be epigenetically modulated.Citation15 However, Wang et al. did not consider the joint effect of methylation and expression on outcome. In addition, IDH1 mutant-associated samples were removed from our study to ensure that the final results would not reflect a bias toward the IDH1 hypermethylator phenotype due to its association with increased survival.Citation6
Our final model focuses not only on how methylation acts through expression to affect survival but also assesses how methylation can associate with survival directly or as a proxy for alternative mechanisms (). The final 27 significant methylation/expression pairs contain genes associated with invasion, angiogenesis, and metabolism, and many have been previously linked to brain/glioma (). Of the 20 genes that contained the significant pairs, none appear to be associated with common amplifications or deletions found in GBM.Citation16 Ten pairs (from seven genes) had a significant expression-based association with survival, suggesting that DNA methylation in these genes affects survival outcome via expression of the associated gene. Interestingly, two genes contained multiple significant methylation/expression pairs. One of these genes, oncostatin M receptor (OSMR), contained two significant pairs, both with the same gene expression probe, but paired with different DNA methylation loci. The DNA methylation loci for these pairs fall in a CpG island within 550 bp of the transcription start site of the OSMR gene, and the pairs had a negative correlation of methylation and expression, suggesting that methylation of these loci could inhibit gene expression. The locus pairs (cg03138091_A_24_P388860 and cg26475085_A_24_P388860) were associated with a significant expression-based association for each CpG. It is known that OSMR β associates with Interleukin 31 Receptor α (IL31RA) to form the Interleukin 31 receptor (IL31) complex which activates the signal transducer and activator of transcription 3 (STAT3).Citation17 Priester et al. (2013) recently demonstrated that silencing of STAT3 inhibits glioma single cell infiltration and tumor growth, suggesting that STAT3 plays an important role in the invasiveness of gliomas.Citation18 If OSMR is silenced via DNA methylation of its promoter, this could lead to a decrease in OSMR gene expression and its association with IL31RA, inhibiting the subsequent activation of STAT3. Without activated STAT3, GBM growth and infiltration could be attenuated, potentially causing increased survival. This proposed mechanism supports the expression-based association of OSMR methylation on survival found in the present study.
Figure 2. Model for mediation analysis. First a linear model adjusted for study was used to determine significantly correlated methylation/expression pairs. Next, a Cox proportional hazards model was used to find significant association between survival and expression, methylation, and their interaction term (adjusting for age, gender, and study phase). An Accelerated failure time model (AFT) was used to estimate the association between survival and expression, methylation, and their interaction term (adjusting for age, gender, and study phase), and a mediation analysis was performed to estimate the alternative and expression-based associations on glioma survival.
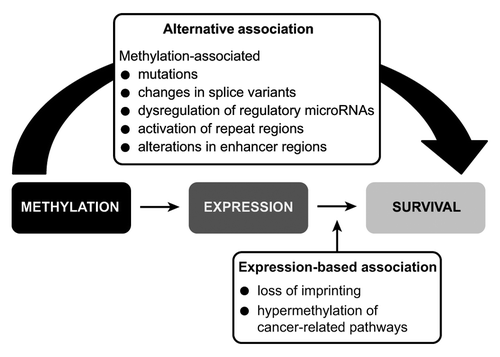
Table 3. Functions of significant genes and potential mechanisms in glioma
In addition to the 10 pairs with significant expression-based associations, there were also 14 methylation/expression pairs (in 12 genes) with significant alternative associations. In these genes, DNA methylation is associated with survival either directly or through mechanisms other than direct changes in gene expression. For instance, aquaporin 1 (AQP1) contained one methylation/expression pair, which is located in a CpG island within 300 bp of the transcription start site of the AQP1 gene. The pair showed a negative correlation, suggesting that methylation of this locus could inhibit gene expression. The major function of aquaporins (AQPs) is transportation of water across cell membranes, the disruption of which has been shown to disturb the blood-brain barrier and lead to cerebral edema.Citation19-Citation21 AQP1 and AQP4 are most abundantly expressed in the nervous system; the expression of both has been observed in GBM and found to correlate with malignancy, specifically cytotoxic cerebral edema, angiogenesis, and migration/invasion.Citation19,Citation22,Citation23 Recently, it has been shown that both AQP1 and AQP4 are direct targets of several microRNAs including microRNA 320a (miR-320a); furthermore, increased miR-320a is associated with a reduction in AQP1/4 expression.Citation24 Therefore, a possible mechanistic explanation for the alternative association we observe involves methylation of the microRNA target region on AQP1, inhibiting the binding of regulatory mircroRNAs such as miR-320a and ultimately allowing transcription of AQP1.
Interestingly, four methylation/expression pairs (three genes) had both significant alternative and expression-based associations. Of interest is the gene growth factor receptor-bound protein 10 (GRB10), which contained two significant pairs, both with the same DNA methylation locus but paired with different gene expression probes. The DNA methylation locus for these pairs falls in a CpG island of the GRB10 gene, and the pairs showed a negative correlation. The locus pairs (cg24302095_A_24_P235266 and cg24302095_A_24_P235268) have significant alternative associations that suggest a decrease in survival may be observed with a 5% increase in methylation; however, the pairs also have significant expression-based associations. GRB10 is an imprinted gene that is differentially expressed from two promoters. In the brain, it is paternally expressed.Citation25 GRB10 interacts with receptor tyrosine kinases and signaling molecules, most commonly insulin receptors and insulin-like growth factor receptors.Citation25,Citation26 In addition, monoallelic expression appears to be limited to fetal brain, skeletal muscle, and, most recently, placenta.Citation25,Citation26 Not only is expression of GRB10 tissue-specific, but it is also isoform specific.Citation25 Currently, 13 different splice variants of GRB10 have been identified, with all but one being expressed in the brain.Citation26 Overexpression of some isoforms has been shown to suppress growth.Citation25 Yu et al. (2011) found decreased expression of GRB10 in many human tumor types, including gliomas, compared with corresponding normal tissue.Citation27 These tumor samples demonstrated a negative correlation between GRB10 and PTEN expression. Furthermore, in a murine cell line, stabilization of Grb10 due to mTORC1-mediated phosphorylation resulted in inhibition of PI3K and ERK-MAPK pathways, suggesting a role for Grb10 as a tumor suppressor.Citation27 Conversely, Nord et al. (2009), using a 32K bacterial artificial chromosome array, found human GRB10 to be a putative novel oncogene in glioblastoma.Citation28 Mechanistic differences may be attributed to inherent imprinting differences in GRB10 between mice and humans. Nonetheless, DNA methylation of this CpG locus has the potential to cause alternative splice sites and may be responsible for the different isoforms of GRB10. Therefore, it is plausible that both the alternative and expression-based associations of this gene have a significant effect on survival. Further potential mechanisms for genes containing significant pairs can be found in .
There were several limitations to our work. First, we relied upon publically available data, which did not have complete IDH1 mutation data or survival data. We used a previously validated approach Citation6,Citation37 to control for this, but this remains a limitation. To address the issue of missing survival data, we used an accelerated failure time model to predict the survival time of censored values. In order to ensure functionality of methylation loci in our analysis, an initial screen was conducted, and only methylation and expression pairs that were significantly correlated within the same gene were used. It should be noted that promoter methylation of MGMT, which has been found in approximately 35–45% of GBM,Citation29,Citation30 was significantly correlated with MGMT gene expression (data not shown), but was not observed in our final list of significant pairs. This may be attributable to the relatively large number of subjects required to detect an association between treatment and methylation at this locus. Furthermore, MGMT expression has been found to be very low (no more than 15 000 molecules per cell)Citation31 and low sensitivity expression arrays have difficulty detecting lowly expressed genes. It has been demonstrated that even in cases where MGMT promoter methylation has been associated with survival outcome, it may not be simultaneously associated with MGMT expression.Citation32 Other mechanisms, such as polycomb repression, may suppress MGMT expression without associated DNA methylation.Citation33 Therefore, it is not surprising that our study did not detect a significant correlation between MGMT methylation and expression. Furthermore, literature has demonstrated several cases where MGMT methylation has not been associated with survival in temozolomide-treated GBM patients.Citation34,Citation35,Citation36
Additionally, there was one pediatric patient out of the 241 samples (age 10) that was not removed from the study prior to analysis. Finally, our approach focused on methylation that regulates expression of the same gene, as mentioned above, but would miss methylation loci that do not regulate gene expression and are associated with survival through alternative mechanisms. When establishing significant loci with no gene expression associations, difficulties such as distinguishing null findings arising due to severe multiple comparisons from those with true biology will be an issue.
Overall, our findings are consistent with the accepted concept that DNA methylation can associate with survival outcome via alterations in gene expression (e.g., OSMR). Our findings also suggest that methylation can associate with survival outcome through mechanisms other than dysregulation of gene transcription, including disruption of microRNA function, as is suggested in the case of AQP1. Additionally, some methylation/expression pairs have both significant alternative and expression-based associations, suggesting that different tumors are using discrete mechanisms and yielding different survival outcomes, as described for the proposed alternative and expression-based associations of GRB10. Importantly, our data suggest that this approach might be profitably applied to cancers other than GBM. Our method also brings to light pathways for future study into potential mechanisms in the pathogenesis of glioma. Though additional validation is needed, our work supports the concept that DNA methylation can function both through gene expression and alternative mechanisms to modulate survival outcomes among glioblastoma patients.
Materials and Methods
External data sets
Methylation, expression, and mutation data for glioblastoma multiforme (GBM) were downloaded from The Cancer Genome Atlas (TCGA) for two different sample sets. Level 1 HumanMethylation27 (Illumina) DNA methylation data and level 2 AgilentG4502A_07_1 and 2 gene expression data were downloaded for all available GBM batches. GBM batches 1, 2, 3, and 10 were used as the phase 1 set and GBM batches 16, 20, 26, 38, and 62 were used as the phase 2 data set. Patient samples lacking covariate data were removed; samples were further restricted to patients diagnosed with glioblastoma who were alive 30 d after their date of diagnosis. Data sets were not combined in further analyses due to the fact that phase 2 data did not have definitive IDH mutation status. Since IDH mutations are associated with survival, we were hesitant to combine the two data sets as mis-identification of IDH mutations could grossly affect findings.
Recursively partitioned mixture model to determine IDH1 mutation status
Patient survival, DNA methylation, gene expression, and IDH1 mutation data (phase 1 set only), was obtained for primary glioblastoma multiforme (GBM) samples. It has been widely acknowledged that IDH1 mutants are almost exclusively associated with a hypermethylater (G-CIMP) phenotype, and this phenotype is associated with increased survival in glioma.Citation10,Citation11 Therefore, we wanted to remove IDH mutant samples from our study so results would not be biased due to increased survival associated with this mutation. Since IDH mutation data was not available for the phase 2 sample set, we employed a recursively partitioned mixture model (RPMM) as described by Houseman et al.Citation37 and used in Christensen and Smith et al.Citation6 The RPMM successfully divided the phase 1 set into seven classes (see Supplemental Material, Fig. S2), and the samples in the top two most highly methylated classes, along with the samples having IDH mutations in the phase 1 set, were removed (TCGA.14.1458, TCGA.16.1460, TCGA.19.1788, TCGA.14.1456, TCGA.28.1756, TCGA.14.4157, TCGA.32.4208).
Methylation data
Methylation β values were extracted from raw idat files using GenomeStudio software (Illumina), which calculates β values using M/(M + U + 100), where M is the methylated signal, U is the unmethylated signal, and 100 is an arbitrary offset. Replicates that did not correlate were removed (TCGA.06.0137, TCGA.06.0145). For methylation loci, all loci that contained a detection P > 0.05 for any sample were removed from further analysis. Since approximately 25% of the survival data are censored, censored survival times were estimated using an accelerated failure time (AFT) model based on the Equation 1 below.
log(T) = b0 + b1Age + b2Gender + b3Study + b4(Age + Study) + b5(Study + Gender) + με
where T follows a Weibull distributionCitation38 (μ is a scale parameter, and ε follows an extreme value distribution). Next, methylation values were normalized for bead chip to control for potential batch effect using the ComBat methodCitation39 with adjustment for age, gender, survival, censored data, and survival-censored interaction.
Expression data
TCGA expression and methylation subject identification numbers were matched; all non-matching samples were removed from the data sets. Replicates in expression samples were either averaged or chosen based on the closest mean and standard deviation to the methylation distribution across all samples. The final data sets consist of a phase 1 data set (n = 73) and a phase 2 data set (n = 168) that contain complete data on overall survival, DNA methylation, and gene expression, with samples considered G-CIMP removed.
Final methylation/expression locus pairs
Methylation and expression loci were merged based on gene of origin. Annotation files for both platforms (HumanMethylation27 and AgilentG4502A_07_1 and 2) were downloaded from TCGA and matched by gene symbol, (using the manufacturer’s annotation) yielding 66 202 methylation/expression pairs. It should be noted that there are usually several methylation loci and/or expression probes found within each gene, so while each pair is unique upon merging, an individual methylation or expression locus may be repeated among several pairs.
Statistical analysis
To choose statistically significant methylation and expression pairs, expression was regressed on methylation in the pooled (n = 241) data set. The associated p-values were adjusted for false discovery rate (FDR) using the Benjamini-Hochberg (BH) procedure.Citation40 All methylation/expression pairs that had a q-value < 0.05 were identified as being significantly associated with each other (n = 9821 pairs).
To further siphon out statistically significant pairings, pairs were then assessed using a Cox proportional hazards model for the effect of expression, methylation, and their interaction on survival, controlling for age, gender, and study phase (phase 1 and phase 2, when applicable). A three degree-of-freedom (DF) Chi-square test was performed to test for significance of expression, methylation, and their cross-product interaction. The three-DF models were repeated for both phase 1 (n = 73) and phase 2 data sets (n = 168) separately and the pooled data set (n = 241). In order to reduce false positives, final statistically significant pairs were selected for having P < 0.05 in both phase 1 and phase 2 data sets and q-values of <0.1 in the pooled data set.
The associations of methylation and expression on survival were determined by a mediation analysis adopted from VanderWeeleCitation38 using the following equations for the expression-based and alternative associations of methylation on survival:
Equation 2. E[E|M, c] = β0 + β1M + β2c
Equation 3. log(T) = θ0 + θ1M + θ2E + θ3EM + θ4c + νε
Equation 4. △M→E→T = (θ2β1 + θ3β1m)(m − m*)
Equation 5. △M→T = (θ1 + θ3[β0 + β1m* + β2c + θ2σ2])(m − m*) + 0.5θ32σ2(m2 − m*2),
where T is survival time, E is expression, M is methylation, c is study, σ2 is the variance of the error term in Equation 2, ε is a random error in Equation 3 following the extreme value distribution, and ν is a scale parameter. For our purposes, m* is median methylation and (m-m*) is the change in methylation we are interested in observing. For example, we would set m-m* to 0.05 if we wanted to look at the change in survival for a 5% increase in methylation. Equation 2 represents the linear model for the association between expression and methylation, and Equation 3 represents the accelerated failure time model with interaction between methylation and expression. β0-β2 are the regression parameters for the linear model, and θ0-θ4 are the regression parameters for the accelerated failure time model. We used a stepwise mediation analysis that considers the relationships between methylation and expression (Eqn. 2) and their joint effect on survival (Eqn. 3). In our case, an alternative association is the effect that methylation alone (or as a proxy for alternative mechanisms) has on survival, and expression-based association is the effect of methylation on survival mediated through gene expression. Equation 4 represents the expression-based association, and Equation 5 represents the alternative association of methylation on survival,Citation38 both of which can be estimated by fitting the models in Equations 2 and 3. We used bootstrap to find the variances and confidence intervals of the expression-based and alternative associations.
To determine directionality of the association of methylation on expression, we looked at the coefficient in the linear model regressing expression on methylation (Eqn. 2). A negative coefficient suggests that methylation and expression are inversely related (i.e., increased methylation is associated with decreased expression and vise versa). A positive correlation demonstrates that methylation and expression are directly related (i.e., increased methylation is associated with increased expression).
Abbreviations: | ||
GBM | = | glioblastoma multiforme |
CNV | = | copy number variation |
G-CIMP | = | Glioma CpG Island Methylator Phenotype |
AFT | = | accelerated failure time |
iBag | = | integrative Bayesian analysis |
BH | = | Benjamini-Hochberg |
FDR | = | false discovery rate |
DF | = | degrees-of-freedom |
Additional material
Download Zip (744 KB)Disclosure of Potential Conflicts of Interest
No potential conflicts of interest were disclosed.
Acknowledgments
The authors would like to thank all individuals involved in the TCGA, particularly the patients who donated samples for use in this research.
Funding
This work was funded by grants from the National Cancer Institute (CA100679, K.T.K.); and the National Institutes of Health (CA126831, J.K.W).
References
- Wen PY, Kesari S. Malignant gliomas in adults. N Engl J Med 2008; 359:492 - 507; http://dx.doi.org/10.1056/NEJMra0708126; PMID: 18669428
- Phillips HS, Kharbanda S, Chen R, Forrest WF, Soriano RH, Wu TD, Misra A, Nigro JM, Colman H, Soroceanu L, et al. Molecular subclasses of high-grade glioma predict prognosis, delineate a pattern of disease progression, and resemble stages in neurogenesis. Cancer Cell 2006; 9:157 - 73; http://dx.doi.org/10.1016/j.ccr.2006.02.019; PMID: 16530701
- Cancer Genome Atlas Research Network. Comprehensive genomic characterization defines human glioblastoma genes and core pathways. Nature 2008; 455:1061 - 8; http://dx.doi.org/10.1038/nature07385; PMID: 18772890
- Verhaak RG, Hoadley KA, Purdom E, Wang V, Qi Y, Wilkerson MD, Miller CR, Ding L, Golub T, Mesirov JP, et al, Cancer Genome Atlas Research Network. Integrated genomic analysis identifies clinically relevant subtypes of glioblastoma characterized by abnormalities in PDGFRA, IDH1, EGFR, and NF1. Cancer Cell 2010; 17:98 - 110; http://dx.doi.org/10.1016/j.ccr.2009.12.020; PMID: 20129251
- Frattini V, Trifonov V, Chan JM, Castano A, Lia M, Abate F, Keir ST, Ji AX, Zoppoli P, Niola F, et al. The integrated landscape of driver genomic alterations in glioblastoma. Nat Genet 2013; 45:1141 - 9; http://dx.doi.org/10.1038/ng.2734; PMID: 23917401
- Christensen BC, Smith AA, Zheng S, Koestler DC, Houseman EA, Marsit CJ, Wiemels JL, Nelson HH, Karagas MR, Wrensch MR, et al. DNA methylation, isocitrate dehydrogenase mutation, and survival in glioma. J Natl Cancer Inst 2011; 103:143 - 53; http://dx.doi.org/10.1093/jnci/djq497; PMID: 21163902
- Ehrlich M. DNA hypomethylation in cancer cells. Epigenomics 2009; 1:239 - 59; http://dx.doi.org/10.2217/epi.09.33; PMID: 20495664
- Lopez-Serra P, Esteller M. DNA methylation-associated silencing of tumor-suppressor microRNAs in cancer. Oncogene 2012; 31:1609 - 22; http://dx.doi.org/10.1038/onc.2011.354; PMID: 21860412
- Wan J, Oliver VF, Zhu H, Zack DJ, Qian J, Merbs SL. Integrative analysis of tissue-specific methylation and alternative splicing identifies conserved transcription factor binding motifs. Nucleic Acids Res 2013; 41:8503 - 14; http://dx.doi.org/10.1093/nar/gkt652; PMID: 23887936
- Icli B, Wara AK, Moslehi J, Sun X, Plovie E, Cahill M, Marchini JF, Schissler A, Padera RF, Shi J, et al. MicroRNA-26a regulates pathological and physiological angiogenesis by targeting BMP/SMAD1 signaling. Circ Res 2013; 113:1231 - 41; http://dx.doi.org/10.1161/CIRCRESAHA.113.301780; PMID: 24047927
- Noushmehr H, Weisenberger DJ, Diefes K, Phillips HS, Pujara K, Berman BP, Pan F, Pelloski CE, Sulman EP, Bhat KP, et al, Cancer Genome Atlas Research Network. Identification of a CpG island methylator phenotype that defines a distinct subgroup of glioma. Cancer Cell 2010; 17:510 - 22; http://dx.doi.org/10.1016/j.ccr.2010.03.017; PMID: 20399149
- Rivenbark AG, Coleman WB. Dissecting the molecular mechanisms of cancer through bioinformatics-based experimental approaches. J Cell Biochem 2007; 101:1074 - 86; http://dx.doi.org/10.1002/jcb.21283; PMID: 17372928
- Ferté C, Trister AD, Huang E, Bot BM, Guinney J, Commo F, Sieberts S, André F, Besse B, Soria JC, et al. Impact of bioinformatic procedures in the development and translation of high-throughput molecular classifiers in oncology. Clin Cancer Res 2013; 19:4315 - 25; http://dx.doi.org/10.1158/1078-0432.CCR-12-3937; PMID: 23780890
- Wang W, Baladandayuthapani V, Morris JS, Broom BM, Manyam G, Do KA. iBAG: integrative Bayesian analysis of high-dimensional multiplatform genomics data. Bioinformatics 2013; 29:149 - 59; http://dx.doi.org/10.1093/bioinformatics/bts655; PMID: 23142963
- Etcheverry A, Aubry M, de Tayrac M, Vauleon E, Boniface R, Guenot F, Saikali S, Hamlat A, Riffaud L, Menei P, et al. DNA methylation in glioblastoma: impact on gene expression and clinical outcome. BMC Genomics 2010; 11:701; http://dx.doi.org/10.1186/1471-2164-11-701; PMID: 21156036
- Rao SK, Edwards J, Joshi AD, Siu IM, Riggins GJ. A survey of glioblastoma genomic amplifications and deletions. J Neurooncol 2010; 96:169 - 79; http://dx.doi.org/10.1007/s11060-009-9959-4; PMID: 19609742
- Chattopadhyay S, Tracy E, Liang P, Robledo O, Rose-John S, Baumann H. Interleukin-31 and oncostatin-M mediate distinct signaling reactions and response patterns in lung epithelial cells. J Biol Chem 2007; 282:3014 - 26; http://dx.doi.org/10.1074/jbc.M609655200; PMID: 17148439
- Priester M, Copanaki E, Vafaizadeh V, Hensel S, Bernreuther C, Glatzel M, Seifert V, Groner B, Kögel D, Weissenberger J. STAT3 silencing inhibits glioma single cell infiltration and tumor growth. Neuro Oncol 2013; 15:840 - 52; http://dx.doi.org/10.1093/neuonc/not025; PMID: 23486688
- Papadopoulos MC, Verkman AS. Aquaporin water channels in the nervous system. Nat Rev Neurosci 2013; 14:265 - 77; http://dx.doi.org/10.1038/nrn3468; PMID: 23481483
- Bonomini F, Rezzani R. Aquaporin and blood brain barrier. Curr Neuropharmacol 2010; 8:92 - 6; http://dx.doi.org/10.2174/157015910791233132; PMID: 21119879
- Wolburg H, Noell S, Fallier-Becker P, Mack AF, Wolburg-Buchholz K. The disturbed blood-brain barrier in human glioblastoma. Mol Aspects Med 2012; 33:579 - 89; http://dx.doi.org/10.1016/j.mam.2012.02.003; PMID: 22387049
- El Hindy N, Bankfalvi A, Herring A, Adamzik M, Lambertz N, Zhu Y, Siffert W, Sure U, Sandalcioglu IE. Correlation of aquaporin-1 water channel protein expression with tumor angiogenesis in human astrocytoma. Anticancer Res 2013; 33:609 - 13; PMID: 23393355
- Saadoun S, Papadopoulos MC, Hara-Chikuma M, Verkman AS. Impairment of angiogenesis and cell migration by targeted aquaporin-1 gene disruption. Nature 2005; 434:786 - 92; http://dx.doi.org/10.1038/nature03460; PMID: 15815633
- Sepramaniam S, Armugam A, Lim KY, Karolina DS, Swaminathan P, Tan JR, Jeyaseelan K. MicroRNA 320a functions as a novel endogenous modulator of aquaporins 1 and 4 as well as a potential therapeutic target in cerebral ischemia. J Biol Chem 2010; 285:29223 - 30; http://dx.doi.org/10.1074/jbc.M110.144576; PMID: 20628061
- Blagitko N, Mergenthaler S, Schulz U, Wollmann HA, Craigen W, Eggermann T, Ropers HH, Kalscheuer VM. Human GRB10 is imprinted and expressed from the paternal and maternal allele in a highly tissue- and isoform-specific fashion. Hum Mol Genet 2000; 9:1587 - 95; http://dx.doi.org/10.1093/hmg/9.11.1587; PMID: 10861285
- Monk D, Arnaud P, Frost J, Hills FA, Stanier P, Feil R, Moore GE. Reciprocal imprinting of human GRB10 in placental trophoblast and brain: evolutionary conservation of reversed allelic expression. Hum Mol Genet 2009; 18:3066 - 74; http://dx.doi.org/10.1093/hmg/ddp248; PMID: 19487367
- Yu Y, Yoon SO, Poulogiannis G, Yang Q, Ma XM, Villén J, Kubica N, Hoffman GR, Cantley LC, Gygi SP, et al. Phosphoproteomic analysis identifies Grb10 as an mTORC1 substrate that negatively regulates insulin signaling. Science 2011; 332:1322 - 6; http://dx.doi.org/10.1126/science.1199484; PMID: 21659605
- Nord H, Hartmann C, Andersson R, Menzel U, Pfeifer S, Piotrowski A, Bogdan A, Kloc W, Sandgren J, Olofsson T, et al. Characterization of novel and complex genomic aberrations in glioblastoma using a 32K BAC array. Neuro Oncol 2009; 11:803 - 18; http://dx.doi.org/10.1215/15228517-2009-013; PMID: 19304958
- Hegi ME, Diserens AC, Gorlia T, Hamou MF, de Tribolet N, Weller M, Kros JM, Hainfellner JA, Mason W, Mariani L, et al. MGMT gene silencing and benefit from temozolomide in glioblastoma. N Engl J Med 2005; 352:997 - 1003; http://dx.doi.org/10.1056/NEJMoa043331; PMID: 15758010
- Weller M, Felsberg J, Hartmann C, Berger H, Steinbach JP, Schramm J, Westphal M, Schackert G, Simon M, Tonn JC, et al. Molecular predictors of progression-free and overall survival in patients with newly diagnosed glioblastoma: a prospective translational study of the German Glioma Network. J Clin Oncol 2009; 27:5743 - 50; http://dx.doi.org/10.1200/JCO.2009.23.0805; PMID: 19805672
- Silber JR, Bobola MS, Ghatan S, Blank A, Kolstoe DD, Berger MS. O6-methylguanine-DNA methyltransferase activity in adult gliomas: relation to patient and tumor characteristics. Cancer Res 1998; 58:1068 - 73; PMID: 9500473
- Melguizo C, Prados J, González B, Ortiz R, Concha A, Alvarez PJ, Madeddu R, Perazzoli G, Oliver JA, López R, et al. MGMT promoter methylation status and MGMT and CD133 immunohistochemical expression as prognostic markers in glioblastoma patients treated with temozolomide plus radiotherapy. J Transl Med 2012; 10:250; http://dx.doi.org/10.1186/1479-5876-10-250; PMID: 23245659
- Zheng S, Houseman EA, Morrison Z, Wrensch MR, Patoka JS, Ramos C, Haas-Kogan DA, McBride S, Marsit CJ, Christensen BC, et al. DNA hypermethylation profiles associated with glioma subtypes and EZH2 and IGFBP2 mRNA expression. Neuro Oncol 2011; 13:280 - 9; http://dx.doi.org/10.1093/neuonc/noq190; PMID: 21339190
- Costa BM, Caeiro C, Guimarães I, Martinho O, Jaraquemada T, Augusto I, Castro L, Osório L, Linhares P, Honavar M, et al. Prognostic value of MGMT promoter methylation in glioblastoma patients treated with temozolomide-based chemoradiation: a Portuguese multicentre study. Oncol Rep 2010; 23:1655 - 62; PMID: 20428822
- Oberstadt MC, Bien-Möller S, Weitmann K, Herzog S, Hentschel K, Rimmbach C, Vogelgesang S, Balz E, Fink M, Michael H, et al. Epigenetic modulation of the drug resistance genes MGMT, ABCB1 and ABCG2 in glioblastoma multiforme. BMC Cancer 2013; 13:617; http://dx.doi.org/10.1186/1471-2407-13-617; PMID: 24380367
- Cecener G, Tunca B, Egeli U, Bekar A, Tezcan G, Erturk E, Bayram N, Tolunay S. The promoter hypermethylation status of GATA6, MGMT, and FHIT in glioblastoma. Cell Mol Neurobiol 2012; 32:237 - 44; http://dx.doi.org/10.1007/s10571-011-9753-7; PMID: 21928112
- Houseman EA, Christensen BC, Yeh RF, Marsit CJ, Karagas MR, Wrensch M, Nelson HH, Wiemels J, Zheng S, Wiencke JK, et al. Model-based clustering of DNA methylation array data: a recursive-partitioning algorithm for high-dimensional data arising as a mixture of beta distributions. BMC Bioinformatics 2008; 9:365; http://dx.doi.org/10.1186/1471-2105-9-365; PMID: 18782434
- VanderWeele TJ. Causal mediation analysis with survival data. Epidemiology 2011; 22:582 - 5; http://dx.doi.org/10.1097/EDE.0b013e31821db37e; PMID: 21642779
- Johnson WE, Li C, Rabinovic A. Adjusting batch effects in microarray expression data using empirical Bayes methods. Biostatistics 2007; 8:118 - 27; http://dx.doi.org/10.1093/biostatistics/kxj037; PMID: 16632515
- Benjamini Y, Hochberg Y. Controlling the false discovery rate: a practical and powerful approach to multiple testing. J R Stat Soc, B 1995; 57:289 - 300
- Ruan B, Pong K, Jow F, Bowlby M, Crozier RA, Liu D, Liang S, Chen Y, Mercado ML, Feng X, et al. Binding of rapamycin analogs to calcium channels and FKBP52 contributes to their neuroprotective activities. Proc Natl Acad Sci U S A 2008; 105:33 - 8; http://dx.doi.org/10.1073/pnas.0710424105; PMID: 18162540
- Hanissian SH, Teng B, Akbar U, Janjetovic Z, Zhou Q, Duntsch C, Robertson JH. Regulation of myeloid leukemia factor-1 interacting protein (MLF1IP) expression in glioblastoma. Brain Res 2005; 1047:56 - 64; http://dx.doi.org/10.1016/j.brainres.2005.04.017; PMID: 15893739
- Zagzag D, Salnikow K, Chiriboga L, Yee H, Lan L, Ali MA, Garcia R, Demaria S, Newcomb EW. Downregulation of major histocompatibility complex antigens in invading glioma cells: stealth invasion of the brain. Lab Invest 2005; 85:328 - 41; http://dx.doi.org/10.1038/labinvest.3700233; PMID: 15716863
- Halestrap AP. The SLC16 gene family - structure, role and regulation in health and disease. Mol Aspects Med 2013; 34:337 - 49; http://dx.doi.org/10.1016/j.mam.2012.05.003; PMID: 23506875
- Miranda-Gonçalves V, Honavar M, Pinheiro C, Martinho O, Pires MM, Pinheiro C, Cordeiro M, Bebiano G, Costa P, Palmeirim I, et al. Monocarboxylate transporters (MCTs) in gliomas: expression and exploitation as therapeutic targets. Neuro Oncol 2013; 15:172 - 88; http://dx.doi.org/10.1093/neuonc/nos298; PMID: 23258846
- Colen CB, Shen Y, Ghoddoussi F, Yu P, Francis TB, Koch BJ, Monterey MD, Galloway MP, Sloan AE, Mathupala SP. Metabolic targeting of lactate efflux by malignant glioma inhibits invasiveness and induces necrosis: an in vivo study. Neoplasia 2011; 13:620 - 32; PMID: 21750656
- Atkinson GP, Nozell SE, Benveniste ET. NF-kappaB and STAT3 signaling in glioma: targets for future therapies. Expert Rev Neurother 2010; 10:575 - 86; http://dx.doi.org/10.1586/ern.10.21; PMID: 20367209
- Smith SJ, Tilly H, Ward JH, Macarthur DC, Lowe J, Coyle B, Grundy RG. CD105 (Endoglin) exerts prognostic effects via its role in the microvascular niche of paediatric high grade glioma. Acta Neuropathol 2012; 124:99 - 110; http://dx.doi.org/10.1007/s00401-012-0952-1; PMID: 22311740
- Akhtar S, McIntosh P, Bryan-Sisneros A, Barratt L, Robertson B, Dolly JO. A functional spliced-variant of beta 2 subunit of Kv1 channels in C6 glioma cells and reactive astrocytes from rat lesioned cerebellum. Biochemistry 1999; 38:16984 - 92; http://dx.doi.org/10.1021/bi992114x; PMID: 10606534
- Yang X, Zhang Y, Li S, Liu C, Jin Z, Wang Y, Ren F, Chang Z. Rab21 attenuates EGF-mediated MAPK signaling through enhancing EGFR internalization and degradation. Biochem Biophys Res Commun 2012; 421:651 - 7; http://dx.doi.org/10.1016/j.bbrc.2012.04.049; PMID: 22525675
- Totong R, Schell T, Lescroart F, Ryckebüsch L, Lin YF, Zygmunt T, Herwig L, Krudewig A, Gershoony D, Belting HG, et al. The novel transmembrane protein Tmem2 is essential for coordination of myocardial and endocardial morphogenesis. Development 2011; 138:4199 - 205; http://dx.doi.org/10.1242/dev.064261; PMID: 21896630
- Smith KA, Lagendijk AK, Courtney AD, Chen H, Paterson S, Hogan BM, Wicking C, Bakkers J. Transmembrane protein 2 (Tmem2) is required to regionally restrict atrioventricular canal boundary and endocardial cushion development. Development 2011; 138:4193 - 8; http://dx.doi.org/10.1242/dev.065375; PMID: 21896629
- Halestrap AP, Meredith D. The SLC16 gene family-from monocarboxylate transporters (MCTs) to aromatic amino acid transporters and beyond. Pflugers Arch 2004; 447:619 - 28; http://dx.doi.org/10.1007/s00424-003-1067-2; PMID: 12739169