Abstract
Food allergy is mediated by a combination of genetic and environmental risk factors, potentially mediated by epigenetic mechanisms. CD4+ T-cells are key drivers of the allergic response, and may therefore harbor epigenetic variation in association with the disease phenotype. Here we retrospectively examined genome-wide DNA methylation profiles (~450 000 CpGs) from CD4+ T-cells on a birth cohort of 12 children with IgE-mediated food allergy diagnosed at 12-months, and 12 non-allergic controls. DNA samples were available at two time points, birth and 12-months. Case:control comparisons of CD4+ methylation profiles identified 179 differentially methylated probes (DMP) at 12-months and 136 DMP at birth (FDR-adjusted P value < 0.05, delta β > 0.1). Approximately 30% of DMPs were coincident with previously annotated SNPs. A total of 96 allergy-associated non-SNP DMPs were present at birth when individuals were initially disease-free, potentially implicating these loci in the causal pathway. Pathway analysis of differentially methylated genes identified several MAP kinase signaling molecules. Mass spectrometry was used to validate 15 CpG sites at 3 candidate genes. Combined analysis of differential methylation with gene expression profiles revealed gene expression differences at some but not all allergy associated differentially methylated genes. Thus, dysregulation of DNA methylation at MAPK signaling-associated genes during early CD4+ T-cell development may contribute to suboptimal T-lymphocyte responses in early childhood associated with the development of food allergy.
Introduction
IgE-mediated food allergies appear to be increasingCitation1 at a rate more rapid than changes to genome sequence would allow in isolation.Citation2 Such conditions are thought to arise through complex, but still poorly understood, gene-environment interactions during critical periods of immune development. Epigenetic variation is postulated to be an important mechanism through which these interactions are mediated. Epigenetic processes regulate gene expression during immune development, and evidence suggests disruption in these processes can modify disease risk in a manner analogous to single nucleotide polymorphisms (SNPs). Importantly, epigenetic processes can be altered by environmental and developmental cues with increasing evidence suggesting that altered epigenetic profiles may be heritable across generations.Citation3
DNA methylation is a widely studied epigenetic modification, tightly regulated during hematopoietic development.Citation4,Citation5 Studies show that environmental exposures, such as dietary factorsCitation6,Citation7 and air pollution,Citation8 as well as stochastic factors can alter DNA methylation profiles in blood. Thus, variation in the DNA methylation profile during immune development could stably alter the host immune response at the individual level, or may underscore much of the variation in the clinical manifestations of allergic diseases at the population level.Citation9 While this has yet to be fully explored, a role for disruption of DNA methylation profiles in association with allergic disease has been reported in patients with asthma,Citation10,Citation11 rhinitis,Citation12 and atopic dermatitis.Citation13
We have previously demonstrated that dysregulated neonatal CD4+ T-lymphocyte responses are a feature of pediatric food allergy.Citation14 Using gene expression profiling, we compared the transcriptome of CD4+ cells from 30 infants diagnosed with food allergy at 12 mo and 30 non-allergic controls in a retrospective birth cohort, following short-term polyclonal activation of T-cells. We demonstrated that the allergy-associated suboptimal T-cell activation response in newborns involves altered expression of several genes signaling via the TNF- NF-κB pathway. In this follow-up study, we have performed an epigenome-wide association study (EWAS) on purified CD4+ T cells from a subset of these individuals comprising 12 that developed IgE food allergy at 12 mo and 12 age-matched non-allergic controls. All subjects had longitudinally collected DNA samples at birth and 12 mo. Data generated provide preliminary evidence of longitudinally stable (metastable) differences in DNA methylation profiles in pediatric IgE food allergy, a proportion of which is detectable at birth prior to disease onset.
Results
DNA methylation profiling was performed on purified genomic DNA from CD4+ T-cells isolated ex vivo from patients with IgE-mediated food allergy. We began with an exploration of normalized genome-wide DNA methylation profiles using hierarchical clustering and multidimensional scaling (MDS) plots to visualize sample relationships. Unsupervised clustering of genome-wide profiles separated samples according to age, which was the primary source of variation within the data set (). These age-related variations in the CD4+ T-cell epigenome have previously been reported and were not the focus of the current analysis.Citation5 A more thorough examination of other sources of variation suggested consistent differences between phenotypes, which appear driven by only a minor proportion of probes on the array (5022 probes by ANOVA, P < 0.01, ). We next sought to undertake a differential methylation analysis between cases and controls in 12-mo DNA samples, obtained during active disease. Given that CD4+ T-cells are not entirely homogenous, and as it is likely that differences in the CD4+ T-cell subpopulations are associated with food allergy, we employed a surrogate variable analysisCitation15 to overcome potential confounding in association with cellular heterogeneity. Surrogate variables were incorporated as covariates into the linear model to adjust for their effects. Comparing samples collected at 12 mo for food allergy cases and controls identified 179 differentially methylated probes (DMPs) (Adj. P value < 0.05 and delta β > 0.1). The same analysis using birth samples identified 153 DMP (Adj. P value < 0.05 and delta β > 0.1). A total of 136 DMP were common to both analyses suggesting that variation at these sites between groups was stable across both time points (; Table S1). This was confirmed by formal inference testing of the entire data set, adjusting for the effects of age, in which our criteria for differential methylation (as described in the methods) were met for these CpG. Of the metastable DMP, 44/136 (~32%) were within 10 base pairs of a reported SNP and, therefore, may be influenced by SNPs. We divided the metastable DMP into SNP-associated and non SNP-associated and compared these by MDS analysis. It was clear by examining sample clustering patterns that intra-sample variation was much higher for SNP-associated probes indicative of sequence variant effects (). In contrast, between-phenotype variation was much higher in magnitude for non-SNP DMP suggesting these are less likely to be affected by SNPs. Removal of the SNP-associated probes reduced the list of temporally stable disease associated DMPs to 92. The observed difference in methylation between cases and controls for these 92 loci was bidirectional, with an effect size of 10–20% absolute difference in methylation (depending on the CpG), which was consistent at both ages (). In total, 55/92 were gene-associated whereas 43 were intragenic. Gene-associated probes span 49 unique genes, with 6/49 genes (12%) represented by 2 or more DMPs (). We used the DAVID bioinformatics tool to run a pathways enrichment test on the differentially methylated genes, which suggested the KEGG pathway “MAPK signaling pathway” was enriched (unadjusted P = 0.042, ). We selected several candidate loci for further validation by Sequenom EpiTyper analysis. Assays were designed to target specific CpG sites within DMPs identified by array analysis, plus surrounding CpG sites. EpiTyper analysis confirmed both the magnitude and direction of differential methylation for selected candidates at both ages ().
Figure 1. A subset of DNA methylation profiles of CD4+ cells distinguishes food allergy cases from controls. (A) Unsupervised hierarchical clustering of whole genome methylation data derived from ex vivo isolated CD4+ cells of food allergy cases (n = 12, labeled black) and non-allergic controls (n = 12, labeled gray) (left panel). Sample annotation is indicated by shaded boxes below the dendrogram. MDS analysis of the same methylation data fails to discriminate samples based on disease-status (right panel). (B) Unsupervised clustering of the same DNA methylation data based on phenotype-associated probes identified by ANOVA distinguishes disease-phenotypes (left panel). MDS analysis based on the filtered data successfully discriminates food allergy cases and controls. ALLERGY.1 = allergic 12 min, ALLERGY.0 = allergic birth.
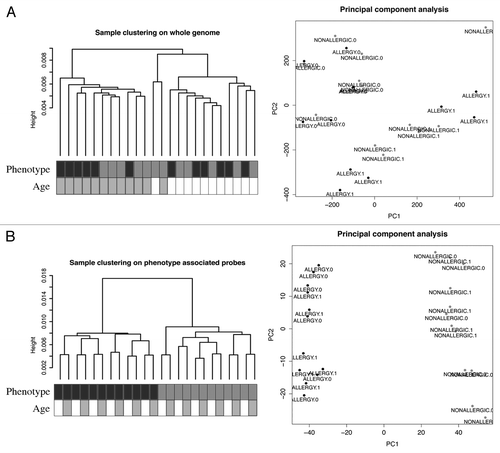
Figure 2. EWAS analysis test identifies differentially methylated CpG (A) Volcano plot depiction of the case vs. control test for differential methylation. DMP were identified by a combination of significance and effect size. The plot shows the log fold genome-wide methylation measurements (x-axis) by the –log P value (FDR-adjusted). Significant data points lie above the gray line. Those with an absolute delta Beta effect size of >10% are shown in black and were identified as candidates. (B) Venn diagram of DMPs detected at each age.
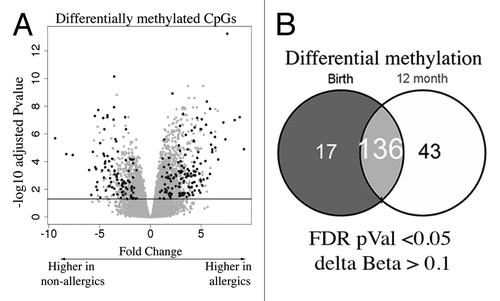
Figure 3. Principal component analysis (PCA) and heatmap visualization of metastable DMPs (A) PCA of SNP-associated (top panel) vs. non SNP-associated metastable DMP. The between-sample variance relative to the between-class variance is higher for SNP-associated probes. Pooled samples are labeled alphabetically. Cases are shown in red, controls in blue. (B) Cluster heatmap analysis of 92 non-SNP metastable DMP. Rows represent probes and columns represent samples. Cells are colored according to level of methylation (Blue = low methylation, yellow = high methylation, scale denotes row standard deviations). Sample annotation is indicated below the dendrogram.
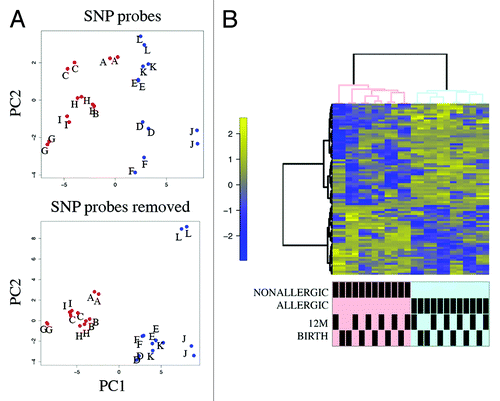
Table 1. Food allergy associated differentially methylated genes
Table 2. Pathways enrichment analysis of food allergy differentially methylated genes
Figure 4. DNA methylation levels measured by Sequenom EpiTyper validate BeadArray methylation data. Target validation for 3 different genes, (A) CACNA1B a calcium signaling molecule (B) KCNN3 a calcium activated channel molecule (C) NAPRT1, an oxidative stress gene. Bars show average percent methylation with standard deviation. Statistical analysis performed by Man-Whitney U test corrected for multiple testing (q-value 1%).
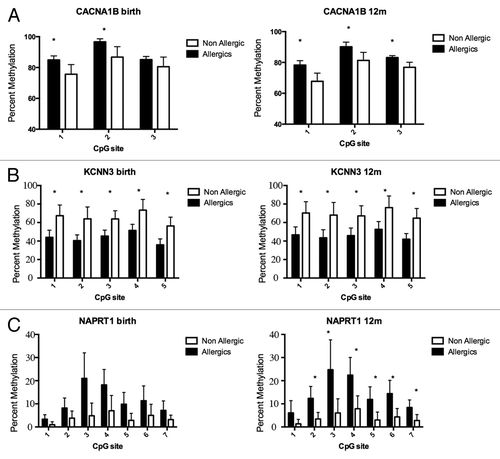
In contrast to the temporally stable allergy-associated DMPs, a number of DMPs were unique to the neonatal samples (17/153, data not shown). Analysis of associated genes did not reveal any noteworthy molecules with a previously described role in allergy, and there were no enriched pathways or gene ontologies in this list. Similarly, 42 out of 179 DMP were uniquely identified in 12-mo samples only. These were associated with 32 genes including a differentially methylated region (DMR) of four consecutive probes hypomethylated within the HLA-DQB1 gene (). These DMPs were in previously annotated SNPs are therefore likely to be affected by underlying haplotypes. Curiously, however, these probes did not appear differentially methylated at birth as well as 12 mo as would be anticipated by a genetically driven difference between the comparator groups. Pathway analysis of 12-mo specific DMP-associated genes revealed enrichment for “intestinal immune network for IgA production” (P = 0.04). The genes in this pathway identified in our data set include HLA-DQB1, CD80, and TNFRSF17.
Figure 5. Differential methylation at HLA-DQB1 and scatterplot of relationship between methylation and gene expression. (A) The figure shows group average methylation data mapped to genomic region for HLADQB1 gene. The top panel shows 12-mo methylation measurements from allergics (blue) and non-allergics (red) plotted against genomic location. The dashed lines indicate the differentially methylated region and the gene transcript is show below. The location of a CpG island is indicated in red and the –log P values for each data point are shown in the bottom panel. (B) Scatterplot of log fold change (FC) DNA methylation (case v control) and gene expression (case vs. control).
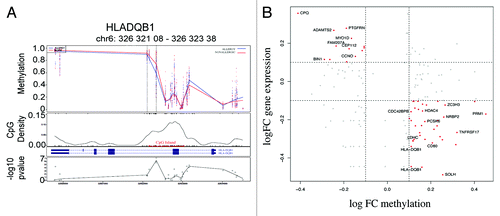
The potential functional effects of gene-associated DMPs were examining through the analysis of previously generated gene expression data for these samples. We examined the relationship between the group average cases vs. controls log fold change (FC) values (FC methylation vs. FC gene expression). A clear relationship between methylation and gene expression was evident for a subset of genes, but not for all, in accordance with previous studies. By dividing CpGs into promoters and gene bodies, and comparing their relationship with gene expression across these classes suggested CpGs in promoters are more likely to correlate with gene expression rather than CpGs in the gene body (Fig. S1), suggesting a complex relationship between methylation and gene expression.
Discussion
There is a growing evidence for a role for epigenetic disruption in allergic disease, but the extent to which these effects are causally related to disease is unclear. In this study, we explored the hypothesis that altered DNA methylation profiles may contribute to the pathogenesis of food allergy. To the best of our knowledge this is the first EWAS study conducted in pediatric food allergy.
Subjects in this study were part of a larger cohort of children with IgE-mediated food allergy, previously used to identify functional differences in the TNF-mediate NF-kB gene response in association with allergic phenotype.Citation10,Citation14 As part of this previous analysis, CD4+ T-cells were activated using anti-CD3 and driven through polyclonal expansion for 24 h after which time gene expression profiles were measured. In the current study, we analyzed available DNA samples from individuals at baseline (unstimulated) to examine whether pre-existing differences in DNA methylation might underscore suboptimal CD4+ T-cell gene expression. Our data identified temporally stable changes in DNA methylation at genes involved in MAP kinase signaling. Importantly, such changes were present in CD4+ T-cells at birth, prior to the development food allergy.
The MAPK pathway is a pro-inflammatory pathway that previous studies have shown is a central regulator of NF-kB transcriptional activation.Citation16 In CD4+ T-cells, the MAPK pathway is induced on activation of the TCR and regulates cytokine gene transcription.Citation17 Alternatively, TLR4 expressing CD4+ T-cells signal via the MAPK pathway to induce tonic inhibitory signals in mice.Citation18 Therefore, we speculate that epigenetic disruption of the MAPK pathway may produce suboptimal signaling leading to the dysregulated CD4+ T-cell responses previously observed in this cohort. Further work is needed to address this specific hypothesis, particularly since these pathways are also regulated post-transcriptionally.
This study design utilized matched DNA samples collected longitudinally, which addresses causation, a central challenge in EWAS study designs.Citation19 We have focused on a purified population of cells from which preexisting functional evidence suggests are relevant to the pathogenesis of disease. Despite this, CD4+ T-cells are heterogeneous and, therefore, we utilized a surrogate variable analysis to capture unwanted heterogeneity and included these effects as covariates in the linear modeling. This is becoming increasingly recognized as an effective and necessary step in such analyses.Citation20 The pooled design adopted here precludes examination of data at the individual level and, therefore, the data reflect group averages. Analysis of gene expression data supports a likely functional role for the identified differential methylation; however, consistent with previous reports, the relationship between gene expression and DNA methylation is complex, with only a subset of identified differential methylation with corresponding difference in associated gene expression.
Our findings are novel and should be considered preliminary and hypothesis generating, given the small sample size. The effects reported here necessitate more detailed follow-up studies in other cohorts. This opens up exciting new avenues of investigation in the causes and mechanisms of food allergy.
Methods
Description of the cohort used for DNA methylation profiling
Subjects were retrospectively selected from a prospective birth cohort recruited through Princess Margaret Hospital with institutional ethics approval. Atopic mothers (as determined by positive skin prick test to a standard panel of allergens) were recruited in the last trimester of pregnancy from community antenatal clinics. Well-defined clinical data was collected on the cohort determined by prospective follow up assessment from birth to 2.5 y. Childhood subjects with IgE-mediated food allergy and non-allergic age-matched controls used for methylation profiling were a subset of a larger group of 60 individuals with previously collected genome-wide gene expression data generated as part of a food allergy array profiling study.Citation14 A pediatric allergist determined allergic status based on clinical outcomes at the 12-mo physical assessment, case history and allergy testing. The food allergic group consisted of children who developed IgE food allergy mostly to hen’s egg (n = 11/12), cow’s milk (n = 1/12), or peanut (n = 2/12). Food allergy was defined by clear immediate symptoms (1–2 h) on exposure to egg, milk, or peanut (including anaphylaxis, angioedema, or urticaria) and confirmed IgE-mediated sensitivity by virtue of a positive skin prick test (>3 mm wheal size) at 12 mo of age. Age-matched non-allergic children showed no evidence of either allergic disease or sensitization (by skin prick test) to any of the allergens tested at any postnatal visits (1, 2.5-y or 5-y). Biospecimens used for this study were retrospectively selected based on 12-mo diagnosis of food allergy and availability of matched birth samples. All included individuals were born to atopic mothers as determined by skin prick testing. Exclusion criteria for this study were maternal smoking, pregnancy complications including gestational diabetes or preeclampsia, or evidence of congenital defects in the newborn. The phenotypes and clinical characteristics of these individuals used in this study are provided in Table S2.
EWAS study design
This was a hypothesis-driven exploratory study in which tissue-specificity and likely role for epigenetic mechanisms was justified based on previous functional evidence. Samples were obtained from CD4+ T-cells isolated by magnetic bead separation as described elsewhere.Citation14 Longitudinal samples were available at birth and 12 mo for all individuals. Methylation data were generated on the Illumina Infinium HumanMethylation450 BeadArray platform with samples randomized across arrays. All samples were processed as a single batch.
Preparation of DNA samples for methylation profiling
DNA quantity was a limiting factor in this experiment since DNA samples were available only for individuals with sufficient cells remaining after prioritization of cells for gene expression analysis published in the original study. In total 24 individuals had available longitudinal DNA samples. Analysis of clinical data did not suggest these individuals differ from the wider cohort with the exception of gender (Table S1). To obtain sufficient quantity of DNA, equal quantities of DNA from 2 donors were pooled prior to bisulphite conversion. A total of six pools of allergics (n = 2 individuals per pool) and six pools of non-allergics (n = 2 per pool) were created. Pool construction was matched at both ages so as to maintain a longitudinal design. Males and females were mixed as sex matching was not possible, and confounding from sex-specific effects was therefore averaged out at the pool level. Quality assessment was performed on DNA pools by spectrophotometry and all pools were concentration normalized to 1ug prior to bisulphite conversion. Pooling has been shown to provide accurate estimates of group averages in DNA methylation data.Citation21 Bisulphite conversion was performed using Methyl Xceed kit from Human Genetic Signatures and tested for completion by an in-house bisulphite-specific PCR assay.
DNA methylation data acquisition and QC
Bisulphite treated DNA samples were shipped to Service XS for methylation array profiling. Raw iDAT files were processed using the minfi packageCitation22 from the bioconductor projectCitation23 in the R statistical environment. The minfi package was used for QC analysis by inspecting control probes on the array none of which were found to be outlying. We also examined the distribution of methylated and un-methlyated signals across the experiment to look for outliers. Raw data was preprocessed using the SWAN normalization method in minfi to normalize Type I and Type II probe bias.Citation24 Probes with a detection P value call > 0.01 in 1 or more samples were removed. Probes on the X and Y-chromosomes were removed to eliminate gender bias. Probes previously demonstrated to potentially cross-hybridize non-specifically in the genome were removed.Citation25 Potential SNP-associated probes were flagged for post-hoc analysis. The log2 ratio for methylated probe intensity to unmethylated probe intensity, the M value, was subsequently derived and used for statistical inference. Beta values were derived from signal intensities as defined by the ratio of methylated to unmethylated probes given by B = M/(U/M*100) and were used to compliment M-value as a measure of effect size. Hierarchical clustering of samples with average linkage used to define clusters together with multidimensional scaling for exploratory analysis of genome-wide DNA methylation profiles. This data has been deposited with the Gene Expression Omnibus and is available under the accession number GSE34639.
Statistical inference
Statistical inference of microarray data was performed in R using the limma package available in bioconductor.Citation26 To identify differentially methylated probes (DMP) we fitted an empirical Bayes linear model to the M-values with age and phenotype as the main dependent variables. This was a repeated measures factorial design. The correlation structure between arrays from the same individuals was estimated using the “corfit” function in limmaCitation26 and was incorporated into the model. A surrogate variable analysisCitation15 was performed to capture any unwanted heterogeneity due to cell composition and these were incorporated into the model. Array weights were used to estimate the model fit for each array and these weights were similarly incorporated in the model. A moderated t testCitation26 was used to test for between group differences. P values were adjusted for multiple testing using the False Discovery Rate method (FDR)Citation27 to control the false discovery rate at 5%. Our criteria for identifying candidate allergy-associated DMP included both adjusted P value significance and effect size. Threshold for minimum effect size was delta Beta value of 0.1 (10% methylation), which is widely considered to be a conservative cutoff for biological rather than technical variation. DMP were defined as FDR-adjusted P value < 0.05 and delta Beta > 0.1. Pathways analysis was conducted using the DAVID bioinformatics softwareCitation28 using the human genome as the background for enrichment test.
Affymetrix human gene 1.0ST data acquisition and processing
Gene expression data have been published elsewhere.Citation14 In brief, pools of total RNA were hybridized to Affymetrix Human Gene 1.0ST arrays. After rigorous quality assessment with all samples passing QC the expression data were preprocessed with the PLIER algorithm (gcbg background subtraction, quantile normalization, iterPLIER summarization). A log2 data transformation was applied in the R environment (http://cran.r-project.org/).
Validation of array targets
Target validation was performed using the Sequenom EpiTYPER (Sequenom, San Diego, USA) Sequenom assays were designed using the Sequenom EpiDesigner software (http://www.epidesigner.com/). Twenty ng of bisulphite DNA was amplified by PCR for EpiTyper analysis. Amplification conditions were as follows: 95 °C for 10 min, 5 cycles of 95 °C for 10 s, 56 °C for 1 min 30 s, and 72 °C for 1 min 30 s and 35 cycles of 95 °C for 10 s, 60 °C for 1 min 30 s, and 72 °C for 1 min 30 s, followed by 72 °C for 7 min. Primer sequences are provided in the supplement. All samples were run in triplicate. The complete amplicon sequences are provided in Table S3.
Abbreviations: | ||
DMP | = | differentially methylated probe |
DMR | = | differentially methylated region |
SNP | = | single nucleotide polymorphism |
Treg | = | regulatory T cell |
SPT | = | Skin prick test |
HM450 | = | Illumina Infinium HumanMethylation450 BeadChip array |
Additional material
Download Zip (301.6 KB)Disclosure of Potential Conflicts of Interest
No potential conflicts of interest were disclosed.
Funding
This work was funded by the National Health and Medical Research Council of Australia (APP10002381), the CASS foundation (SM/11/3647/RMR:DSA), NHMRC Centre of Food and Allergy Research Excellence (#1041420).
References
- Osborne NJ, Koplin JJ, Martin PE, Gurrin LC, Lowe AJ, Matheson MC, Ponsonby A-L, Wake M, Tang MLK, Dharmage SC, et al, HealthNuts Investigators. Prevalence of challenge-proven IgE-mediated food allergy using population-based sampling and predetermined challenge criteria in infants. J Allergy Clin Immunol 2011; 127:668 - , e1-2; http://dx.doi.org/10.1016/j.jaci.2011.01.039; PMID: 21377036
- Prescott S, Allen KJ. Food allergy: riding the second wave of the allergy epidemic. Pediatr Allergy Immunol 2011; 22:155 - 60; http://dx.doi.org/10.1111/j.1399-3038.2011.01145.x; PMID: 21332796
- Morgan DK, Whitelaw E. The case for transgenerational epigenetic inheritance in humans. Mamm Genome 2008; 19:394 - 7; http://dx.doi.org/10.1007/s00335-008-9124-y; PMID: 18663528
- Trowbridge JJ, Snow JW, Kim J, Orkin SH. DNA methyltransferase 1 is essential for and uniquely regulates hematopoietic stem and progenitor cells. Cell Stem Cell 2009; 5:442 - 9; http://dx.doi.org/10.1016/j.stem.2009.08.016; PMID: 19796624
- Martino D, Maksimovic J, Joo J-H, Prescott SL, Saffery R. Genome-scale profiling reveals a subset of genes regulated by DNA methylation that program somatic T-cell phenotypes in humans. Genes Immun 2012; 13:388 - 98; http://dx.doi.org/10.1038/gene.2012.7; PMID: 22495533
- Hollingsworth JW, Maruoka S, Boon K, Garantziotis S, Li Z, Tomfohr J, Bailey N, Potts EN, Whitehead G, Brass DM, et al. In utero supplementation with methyl donors enhances allergic airway disease in mice. J Clin Invest 2008; 118:3462 - 9; PMID: 18802477
- Chang H, Zhang T, Zhang Z, Bao R, Fu C, Wang Z, Bao Y, Li Y, Wu L, Zheng X, et al. Tissue-specific distribution of aberrant DNA methylation associated with maternal low-folate status in human neural tube defects. J Nutr Biochem 2011; 22:1172 - 7; http://dx.doi.org/10.1016/j.jnutbio.2010.10.003; PMID: 21333513
- Perera F, Tang W-Y, Herbstman J, Tang D, Levin L, Miller R, Ho S-M. Relation of DNA methylation of 5′-CpG island of ACSL3 to transplacental exposure to airborne polycyclic aromatic hydrocarbons and childhood asthma. PLoS One 2009; 4:e4488; http://dx.doi.org/10.1371/journal.pone.0004488; PMID: 19221603
- Martino DJ, Prescott SL. Progress in understanding the epigenetic basis for immune development, immune function, and the rising incidence of allergic disease. Curr Allergy Asthma Rep 2013; 13:85 - 92; http://dx.doi.org/10.1007/s11882-012-0312-1; PMID: 23054626
- Nadeau K, McDonald-Hyman C, Noth EM, Pratt B, Hammond SK, Balmes J, Tager I. Ambient air pollution impairs regulatory T-cell function in asthma. J Allergy Clin Immunol 2010; 126:845 - , e10; http://dx.doi.org/10.1016/j.jaci.2010.08.008; PMID: 20920773
- Isidoro-García M, Dávila-González I, Pascual de Pedro M, Sanz-Lozano C, Lorente-Toledano F. Interactions between genes and the environment. Epigenetics in allergy. Allergol Immunopathol (Madr) 2007; 35:254 - 8; http://dx.doi.org/10.1157/13112992; PMID: 18047817
- Nestor CE, Barrenäs F, Wang H, Lentini A, Zhang H, Bruhn S, Jörnsten R, Langston MA, Rogers G, Gustafsson M, et al. DNA methylation changes separate allergic patients from healthy controls and may reflect altered CD4+ T-cell population structure. PLoS Genet 2014; 10:e1004059; http://dx.doi.org/10.1371/journal.pgen.1004059; PMID: 24391521
- Liang Y, Wang P, Zhao M, Liang G, Yin H, Zhang G, Wen H, Lu Q. Demethylation of the FCER1G promoter leads to FcεRI overexpression on monocytes of patients with atopic dermatitis. Allergy 2012; 67:424 - 30; http://dx.doi.org/10.1111/j.1398-9995.2011.02760.x; PMID: 22150093
- Martino DJ, Bosco A, McKenna KL, Hollams E, Mok D, Holt PG, Prescott SL. T-cell activation genes differentially expressed at birth in CD4+ T-cells from children who develop IgE food allergy. Allergy 2012; 67:191 - 200; http://dx.doi.org/10.1111/j.1398-9995.2011.02737.x; PMID: 22077487
- Leek JT, Storey JD. Capturing heterogeneity in gene expression studies by surrogate variable analysis. PLoS Genet 2007; 3:1724 - 35; http://dx.doi.org/10.1371/journal.pgen.0030161; PMID: 17907809
- Olson CM, Hedrick MN, Izadi H, Bates TC, Olivera ER, Anguita J. p38 mitogen-activated protein kinase controls NF-kappaB transcriptional activation and tumor necrosis factor alpha production through RelA phosphorylation mediated by mitogen- and stress-activated protein kinase 1 in response to Borrelia burgdorferi antigens. Infect Immun 2007; 75:270 - 7; http://dx.doi.org/10.1128/IAI.01412-06; PMID: 17074860
- Dodeller F, Schulze-Koops H. The p38 mitogen-activated protein kinase signaling cascade in CD4 T cells. Arthritis Res Ther 2006; 8:205; http://dx.doi.org/10.1186/ar1905; PMID: 16542479
- González-Navajas JM, Fine S, Law J, Datta SK, Nguyen KP, Yu M, Corr M, Katakura K, Eckman L, Lee J, et al. TLR4 signaling in effector CD4+ T cells regulates TCR activation and experimental colitis in mice. J Clin Invest 2010; 120:570 - 81; http://dx.doi.org/10.1172/JCI40055; PMID: 20051628
- Michels KB, Binder AM, Dedeurwaerder S, Epstein CB, Greally JM, Gut I, Houseman EA, Izzi B, Kelsey KT, Meissner A, et al. Recommendations for the design and analysis of epigenome-wide association studies. Nat Methods 2013; 10:949 - 55; http://dx.doi.org/10.1038/nmeth.2632; PMID: 24076989
- Jaffe AE, Irizarry RA. Accounting for cellular heterogeneity is critical in epigenome-wide association studies. Genome Biol 2014; 15:R31; http://dx.doi.org/10.1186/gb-2014-15-2-r31; PMID: 24495553
- Docherty SJ, Davis OSP, Haworth CMA, Plomin R, Mill J. Bisulfite-based epityping on pooled genomic DNA provides an accurate estimate of average group DNA methylation. Epigenet Chromatin 2009; 2:3
- Aryee MJ, Jaffe AE, Corrada-Bravo H, Ladd-Acosta C, Feinberg AP, Hansen KD, Irizarry RA. Minfi: a flexible and comprehensive Bioconductor package for the analysis of Infinium DNA methylation microarrays. Bioinformatics 2014; Forthcoming http://dx.doi.org/10.1093/bioinformatics/btu049; PMID: 24478339
- Gentleman RC, Carey VJ, Bates DM, Bolstad B, Dettling M, Dudoit S, Ellis B, Gautier L, Ge Y, Gentry J, et al. Bioconductor: open software development for computational biology and bioinformatics. Genome Biol 2004; 5:R80; http://dx.doi.org/10.1186/gb-2004-5-10-r80; PMID: 15461798
- Maksimovic J, Gordon L, Oshlack A. SWAN: Subset-quantile within array normalization for illumina infinium HumanMethylation450 BeadChips. Genome Biol 2012; 13:R44; http://dx.doi.org/10.1186/gb-2012-13-6-r44; PMID: 22703947
- Chen Y-A, Lemire M, Choufani S, Butcher DT, Grafodatskaya D, Zanke BW, Gallinger S, Hudson TJ, Weksberg R. Discovery of cross-reactive probes and polymorphic CpGs in the Illumina Infinium HumanMethylation450 microarray. Epigenetics 2013; 8:203 - 9; http://dx.doi.org/10.4161/epi.23470; PMID: 23314698
- Smyth GK. Linear models and empirical bayes methods for assessing differential expression in microarray experiments. Stat Appl Genet Mol Biol 2004; 3:e3; http://dx.doi.org/10.2202/1544-6115.1027; PMID: 16646809
- Storey JD, Tibshirani R. Statistical significance for genomewide studies. Proc Natl Acad Sci U S A 2003; 100:9440 - 5; http://dx.doi.org/10.1073/pnas.1530509100; PMID: 12883005
- Huang W, Sherman BT, Lempicki RA. Systematic and integrative analysis of large gene lists using DAVID bioinformatics resources. Nat Protoc 2009; 4:44 - 57; http://dx.doi.org/10.1038/nprot.2008.211; PMID: 19131956