Abstract
Tumor suppressor genes (TSGs) are commonly inactivated by somatic mutation and/or promoter methylation; yet, recent high-throughput genomic studies have not identified key TSGs inactivated by both mechanisms. We pursued an integrated molecular analysis based on methylation binding domain sequencing (MBD-seq), 450K Methylation arrays, whole exome sequencing, and whole genome gene expression arrays in primary head and neck squamous cell carcinoma (HNSCC) tumors and matched uvulopalatopharyngoplasty tissue samples (UPPPs). We uncovered 186 downregulated genes harboring cancer specific promoter methylation including PAX1 and PAX5 and we identified 10 key tumor suppressor genes (GABRB3, HOXC12, PARP15, SLCO4C1, CDKN2A, PAX1, PIK3AP1, HOXC6, PLCB1, and ZIC4) inactivated by both promoter methylation and/or somatic mutation. Among the novel tumor suppressor genes discovered with dual mechanisms of inactivation, we found a high frequency of genomic and epigenomic alterations in the PAX gene family of transcription factors, which selectively impact canonical NOTCH and TP53 pathways to determine cell fate, cell survival, and genome maintenance. Our results highlight the importance of assessing TSGs at the genomic and epigenomic level to identify key pathways in HNSCC, deregulated by simultaneous promoter methylation and somatic mutations.
Introduction
Head and neck squamous cell carcinoma (HNSCC) is the sixth most common cancer worldwide with an approximate 50% five-year survival rate.Citation1 Two groups that independently studied the genetic origins of HNSCC reported inactivating mutations in NOTCH1.Citation1,Citation2 This was the first strong evidence of NOTCH1 mutations in solid tumors; analysis of the mutations suggested that NOTCH1 might act as a tumor suppressor gene (TSG) in HNSCC.Citation1,Citation2 Notwithstanding this important finding, and contrary to original expectations, these and other detailed analyses of HNSCC did not uncover a great number of recurrent somatic mutations in novel genes.Citation3,Citation4
The number of known mutations and specific mutational hotspots in HNSCC tumors only partially explains their biological complexity and limits the development of novel diagnostic markers and therapeutic agents. TP53 was again identified as the most commonly mutated gene in HNSCC and, while mutant TP53 has been associated with poor survival,Citation5 the most important biologic consequences of this alteration have been elusive. Moreover, it was also known that overall and disease-specific survival is higher in patients with HPV-associated HNSCC tumors,Citation6 and that this distinct molecular and pathologic subtype displays an average of 4 somatic mutations per tumor, while HPV-negative HNSCC tumors harbor 20.Citation1 HPV-associated HNSCCs have a distinctly different molecular landscape from HPV-negative HNSCCs. TP53 is rarely identified as mutated in HPV-positive HNSCC patients because the HPV E6 oncoprotein silences TP53, protecting the cells from apoptosis and senescence, while the HPV E7 oncoprotein deregulates the cell cycle.Citation7,Citation8 CDKN2A, a principal cyclin-dependent kinase inhibitor that decelerates the cell cycle, is lost in HPV-negative HNSCCCitation9 and amplified in HPV-positive HNSCC.Citation10 HNSCCs also exhibit many chromosomal abnormalities, including amplifications of the 11q13 region containing the cyclin D1 gene and the 7p11 region encoding EGFR, which lead to proto-oncogene activation.Citation1
Aberrant DNA methylation of CpGs in the proximity of predicted transcription start sites (TSS) often leads to alterations in gene function and pathway deregulation in human cancer. Epigenetic events linked to TSG inactivation through promoter methylation, are more frequent events than somatic mutations in cancer, and may be driving tumorigenic initiation and progression. Promoter methylation of CDK2NA, HOXA9, NID2, EDNRB, KIF1A, and DCC have previously been identified and characterized in HNSCC.Citation11-Citation13
To date, recent high-throughput methylation studiesCitation14,Citation15 have not focused on the relative contribution of TSGs inactivation by DNA methylation and somatic mutations in oncogenesis and may have severely underestimated the true frequency of inactivation of key TSGs and signaling pathways. We tested the hypothesis that TSG promoter methylation predominantly occurs in genes or pathways with well-known somatic mutations and/or deletions in most HNSCC tumors, including TP53, CDK2NA,Citation16 and, more recently, NOTCH1Citation1,Citation2 and FAT1,Citation17 as well as in recently described genes with low frequency mutations.Citation18
Results
Patient selection
One hundred and eight (108) head and neck squamous cell carcinoma (HNSCC) and 35 uvulopalatopharyngealplasty (UPPP) patients were consented for this study at Johns Hopkins Medical Institutions hospitals and MD Anderson Cancer Center. The study was approved by the Ethics Committee of each participating hospital, as well as by the Johns Hopkins Institutional Review Board. The 143 samples were divided into Discovery and Prevalence cohorts. The Discovery cohort consisted of 32 HNSCC and 16 UPPP samples. The Prevalence cohort consisted of 76 HNSCC and 19 UPPP samples. The 32 tumor samples selected for the Discovery cohort were accrued in Hopkins (n=17) and in MD Anderson (n=15). The 35 UPPP samples were accrued at Hopkins.
Johns Hopkins component
Fresh-frozen surgically resected HNSCC (n = 93) and UPPPP (n = 35) tissue samples were obtained from patients at Johns Hopkins Medical Institutions in Baltimore. HNSCC tissue was analyzed by frozen section histology to estimate neoplastic cellularity. In order to enrich the samples for neoplastic cells, normal tissue was removed from the samples using macro-dissection based on the frozen section histology. HPV tumor status was determined for oropharyngeal tumors per standard clinical care using in situ hybridization. Hybridization was performed using the HPV III Family16 probe set that captures HPV genotypes 16, 18, 33, 35, 45, 51, 52, 56, and 66. HPV16-positive controls included an HPV16-positive oropharyngeal cancer and the SiHa and CaSki cell lines. HPV tumor status was also determined by E6/E7 PCR primer amplification.
MD Anderson component
Fresh-frozen surgically resected tumor samples (n = 15) were obtained from consented patients treated for HNSCC at the University of Texas MD Anderson Cancer Center, under an Institutional Review Board approved protocol. Frozen tissue was embedded in optimal cutting temperature compound and cryosections from the top and middle of specimens were stained with hematoxylin and eosin prior to being evaluated by a pathologist for the presence of >60% tumor nuclei content or absence of tumor (i.e., normal). Samples that passed this criterion were sectioned all the way through and washed once in PBS prior to isolating genomic DNA using an ArchivePure DNA purification kit.
describes the clinical attributes of the combined 143 patient samples from the Discovery and Validation cohorts, as well as of the 289 samples from The Cancer Genome Atlas that were used to confirm the TP53mut/PAX5 met and the NOTCH1mut/PAX1met associations. The 32 HNSCC patient samples selected for Discovery in this epigenetic study were the same used by Agrawal et al. for the genetic study of HNSCCCitation1. This study design allowed us to analyze mutation, methylation, and expression data from the same HNSCC patients. We queried the methylome of these 32 HNSCC patients and frequency matched 16 UPPP normal controls using the Human Methylation 450K Beadchip (Illumina). However, the HNSCC transcriptome was queried with different platforms. In Hopkins the GeneST1.0 arrays (Affymetrix) were used to query the HNSCC and UPPP transcriptome and in MD Anderson they queried the HNSCC transcriptome with the Human Exon 1.0ST arrays (Affymetrix). The integrated methylation/mRNA expression analysis we are reporting was performed using transcriptome data from the 32 samples (16 HNSCC and 16 UPPP) accrued at Hopkins because we did not find a satisfactory method of combining GeneST1.0 and Human Exon 1.0ST array data. Nor did we have transcriptome and methylome data from normal patient samples collected at MD Anderson to compare with the results obtained with patients accrued at Hopkins.
Table 1. Summary of clinical attributes of samples in Discovery, Prevalence, and TCGA cohorts
Characterization of the HNSCC methylome using MBD-seq
In the first part of our study, we used a methylated DNA binding domain based sequencing (MBD-seq) approach similar, in principle, to what has been previously described,Citation19 but with significant modifications (). This analysis was performed on a subset of tumors from the same Discovery Cohort in which Agrawal et al. discovered and mapped mutations in HNSCC,Citation1 comprising ten patients and ten frequency matched normal controls (uvulopalatopharyngoplasty tissue samples [UPPP]). The ten tumor samples were obtained before chemotherapy or radiation treatment, ensuring that the changes we identified are truly reflective of tumor biology, and were micro-dissected to achieve a neoplastic cellularity of greater than 60%. Following MBD-seq, an average of 48.9 million 50 bp reads was obtained for each sample, with an average of 68% of these reads aligning to the hg19 genome, 67% of which were aligning uniquely (Data set 1).
Figure 1. Representation of the workflow of the study. The figure ascribes all the platforms and techniques used in the discovery and the two independent validation sets. The number of samples recruited its time are also depicted in brackets.
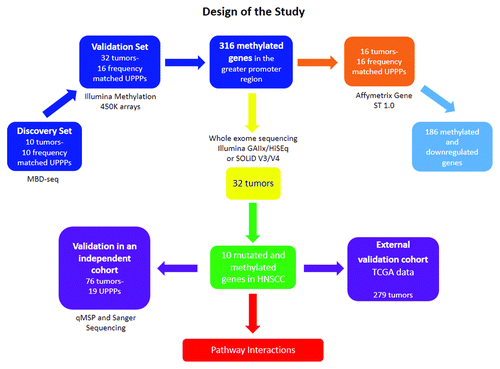
Our purpose was to study alterations in the promoter region of TSGs and for this study we focused on the “greater promoter,” a region that encompasses the well-studied proximal promoter that harbors CpG Islands (1500 bases up and downstream from the TSS) and the distal promoter (6000 bp upstream of the TSS), which includes recently identified CpG Island ShoresCitation20 and Shelves ().Citation21 (From this point on, wherever the term promoter is used it refers to the greater promoter region as defined here).
Figure 2. (A) Illustration defining the Greater Promoter region. Using a functional genomic distribution viewpoint we define five CpG genomic locations in relation to their distance to the Transcription Start Site: Proximal promoter, distal promoter, first exon, gene body and intergenic locations. From a CpG content and neighborhood context viewpoint we define four CpG genomic locations in relation to their distance to the nearest CpG Island: CpG Island, CpG Island Shore, CpG Island Shelf, Open Sea and Gene Body. The Greater promoter window is fixed in relation to the TSS. Therefore, the location of CpG Islands will influence the number of significant sequencing reads and 450K probes per gene that are included in our analysis; (B) Workflow for identification of differential methylation in the greater promoter of HNSCC, using next-generation MBD-sequencing and 450K methylation platforms. Schematic description of the analytic pipeline developed to unveil the HNSCC methylome. This pipeline enriches for mean genome-wide differences in CpG methylation as also for genome-wide differences in CpG methylation variability at each chromosomal location, for both, methylation sequencing and methylation 450K array data.
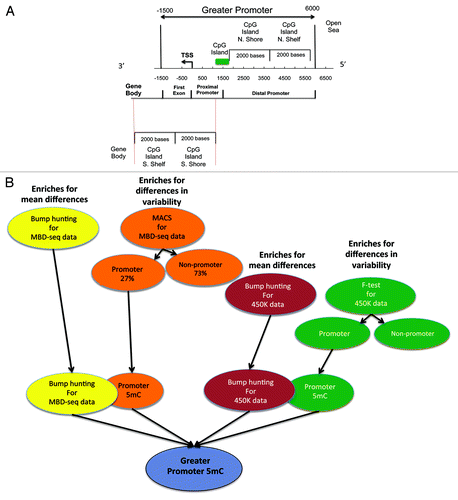
We used two independent and highly validated analytical approaches, model-based analysis of ChIP-seq (MACS)Citation22 and Bump hunting,Citation23 to identify methylation changes across the HNSCC genome. MACS identified 9648 alterations, 60% of which were gains in methylation events (). The vast majority of the methylome changes identified by MACS (73%) were observed outside of the promoter region. Within the promoter region, we observed mostly (89%) gain of methylation events. The majority of the methylation loss identified by MACS (93%) occurred outside the promoter region. These genome-wide methylation motifs were integrated with the differentially methylated regions (DMRs) identified by Bump hunting to obtain the first detailed next-gen analysis of the HNSCC promoter methylome (Data set 2; Tables S1 and S2).
Confirmation of methylated sites using 450K arrays and integration with expression arrays
To validate the MBD-seq results, we evaluated genome-wide differential methylation with the Infinium HumanMethylation450K Beadchip (450K array) in 41 cancer (including the 10 samples sequenced with MBD-seq) and 16 UPPP samples. The intersection of genome-wide methylation sequencing and methylation array screens uncovered 316 genes, which harbor promoter methylation in HNSCC (Table S3). To determine the extent of correlation between differential methylation and mRNA expression patterns, we performed mRNA expression microarray analysis (Affymetrix Gene ST 2.0) using 16 tumor and 16 UPPP samples from the samples included in the cohort analyzed with the 450K array platform. We found close to 60% concordance between concurrent greater promoter methylation and gene downregulation. 186 methylated genes were found to harbor methylation and downregulation; PAX1 and PAX5 exhibited the greatest expression loss (; Table S4A). Table S4B shows the relationship between methylation frequency of the significantly downregulated candidate genes with FC < –2 and the expression levels of these candidate genes for the 16 tumor samples queried with mRNA arrays in the Discovery cohort. We have also included Figure S1 to show the expression level box plots for these candidate genes in the 16 HNSCC and 16 UPPP samples queried in the Discovery cohort.
Figure 3. Integrative analysis of co-localized promoter methylation and somatic mutations with concurrent expression changes. (A) Methylated genes with fold change differences in expression greater than 2; (B) Genes with co-localized promoter methylation and somatic mutations in HNSCC. Methylation frequency is represented by the red color. Mutation frequency is represented by the blue color. The purple color represents the combined frequency of methylation and mutation events in the Discovery cohort.
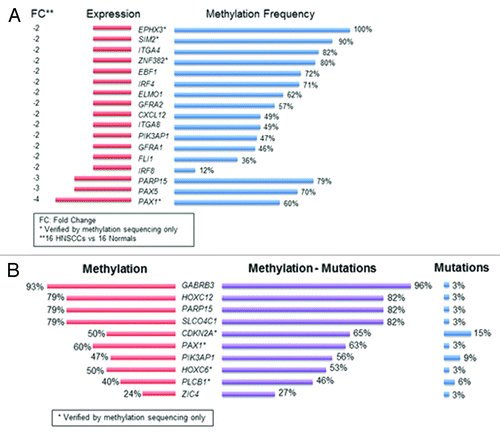
Integration of promoter methylation with somatic mutation profiles
We subsequently intersected the promoter methylome with the mutational landscape of HNSCCCitation1 and identified concurrent promoter methylation and somatic mutations in ten tumor suppressor genes: GABRB3, HOXC12, PARP15, SLCO4C1, CDKN2A, PAX1, PIK3AP1, HOXC6, PLCB1, and ZIC4 (). CDKN2A (p16), one of the most frequently altered tumor suppressor genes in human cancer by mutation, methylation, and/or deletion was confirmed on this list. The rest of the genes displayed a very low mutation frequency in HNSCC, together with frequent inactivation by promoter methylation.
Unbiased genome-wide and gene set enrichment analyses
Unbiased genome-wide analyses were performed to visualize and interpret the large amount of data produced by the sequencing and microarray experiments. Unsupervised hierarchical clustering of the differential methylation events in HNSCC revealed a genome-wide loss of methylation. More than half of the genes in cancer have lost methylation when compared with normal samples at the genome-wide level. This massive loss of methylation often suggests the acquisition of plasticity and de-differentiation associated with stem cellsCitation24 (Fig. S2A), together with specific gains in promoter methylation (Fig. S2B). To better understand the relationship between genome-wide DNA methylation loss and copy number alterations we examined the correlation between genome-wide DNA methylation frequency and copy number alterations. The Spearman correlation coefficient between methylation frequency and copy number loss was 0.02. The Spearman correlation coefficient between methylation frequency and copy number gain was –0.004. The lack of correlation between DNA methylation and genomic aberrations in genes for which both methylome and copy number alterations are available in the Discovery cohort can be seen in Table S5. The genome-wide DNA methylation and genomic aberrations in tumor samples for which both methylome and copy number alterations are available in the Discovery cohort can be seen in Figure S3.
Analysis of Functional Annotation (AFA)Citation25 was then used to integrate the HNSCC methylation, mutation, and expression landscapes and detect alterations in cellular signaling pathways, protein-protein interaction networks, and gene ontology (GO) in HNSCC. AFA revealed that pathways involved in development, differentiation, adhesion, proliferation, and biological/cellular/transcriptional regulation are impacted by concurrent promoter methylation, mutations, and differential gene expression in HNSCC (Figs. S4 and S5; Table S6). AFA also showed that pathways involved in immune system development, and cell differentiation, proliferation, growth and renewal are impacted by concurrent epigenetic and genetic alterations in HNSCC (Fig. S6).
Methylation-Mutations Interactions with Risk Factors in the Discovery Cohort
When attempting to search for any associations between the mutated and/or methylated genes and HNSCC risk factors (TP53 mutations, HPV status, and smoking history) in the Discovery Cohort, we observed interesting patterns for the PAX1 and PAX5 genes. PAX1 was methylated in all HPV negative tumors whereas zero methylation events of this gene were observed in HPV positive tumors. PAX1 was also methylated in most patients with a history of tobacco exposure (71%), while only 33% of patients without tobacco exposure history exhibited PAX1 methylation. Most HPV negative tumors (83%) showed PAX5 methylation compared with 25% of HPV positive tumors. On the contrary, tumors from patients with a history of tobacco exposure (57%) had similar frequency of PAX5 methylation to patients with no smoking history (67%). We also observed concurrent genomic and epigenomic associations with viral and tobacco exposures. Patients with TP53 mutations also had PAX1 promoter methylation, history of tobacco exposure, and were HPV negative. Most (83%) of the patients with TP53 mutations had evidence of PAX5 methylation (Fig. S7).
Validation of PAX1, ZIC4, PLCB1, and PAX5 promoter methylation with quantitative methylation specific PCR and TCGA data
We performed qMSP for 3 genes, PAX1, ZIC4, and PLCB1, in 76 tumor samples previously used by Agrawal in a HNSCC deep sequencing studyCitation1 and 19 UPPP samples (Table S7 provides qMSP primers and probes information). All 3 genes appeared on our top ten list of TSG inactivated by both mechanisms and we confirmed a high frequency of tumor specific methylation in the Validation cohort. PAX1 was methylated in 68% of the cancer cases, ZIC4 in 80% and PLCB1 in 52%. We then tested PAX 5 because it is in the PAX gene family and appeared on the list of the most frequently inactivated genes by promoter methylation and downregulation of expression. PAX5 was methylated in 77% of the HNSCC cases in the validation cohort, a number very similar to the 70% methylation frequency identified in the discovery set. We found that PAX1 (P < 0.0001), ZIC4 (P < 0.0001), PLCB1 (P < 0.001), and PAX5 (P < 0.0001) methylation distinguished tumor from UPPP samples (). Receiver Operator Characteristic (ROC) curve analysis revealed that PAX1 had 68% sensitivity, 90% specificity and a 0.72 AUC; ZIC4 had 73% sensitivity, 100% specificity, and a 0.87 AUC; PLCB1 had 55% sensitivity, 84% specificity, and a 0.70 AUC; and PAX5 had 80% sensitivity, 94% specificity, and a 0.86 AUC (). A gene panel combining promoter methylation results for these four genes had 96% sensitivity, 94% specificity, a 0.97 AUC, and a Positive Predictive Value of 98.5% (). A chi-square test of independence revealed an association between methylation in PLCB1 and tumor site, P < 0.01. Tumors of the oral cavity and oropharynx were the most frequently methylated.
Figure 4. qMSP results for PAX1, PAX5, ZIC4 and PLCB1. (A) Graphical expression of the logistic regression, Pr (HNSCC = 1) = logit−1 (b0 + b1 x methylation) in tissue from 76 participants with data overlain. The predictor methylation is the qMSP value for each case (1) and each control (0). Cutoff methylation values for PAX1, PAX5 ZIC4 and PLCB1 are shown by the vertical dotted line. Probability of HNSCC is shown in red; (B) Scatterplots of quantitative MSP analysis of candidate genes promoters in the Validation screen cohort, which consisted of 76 HNSCC tumor tissue samples and 19 normal tissue samples obtained from uvulopharyngopalatoplasty (UPPP) procedures performed in non-cancer patients. The relative level of methylated DNA for each gene in each sample was determined as a ratio of MSP for the amplified gene to ACTB and then multiplied by 1000 [(average value of duplicates of gene of interest / average value of duplicates of ACTB) x 1000] for, PAX1, PAX5, ZIC4, and PLCB1. Red line denotes cutoff value; (C) Sensitivity, Specificity and AUC results for qMSP analysis; (D) Receiver Operator Characteristics (ROC) curve for promoter methylation of PAX1, PAX5, ZIC4 and PLCB1 genes in the validation cohort. The figure shows that for this four gene panel the qMSP results have 96% sensitivity, 94% specificity, a 0.97 AUC and a Positive Predictive Value of 98.5%.
![Figure 4. qMSP results for PAX1, PAX5, ZIC4 and PLCB1. (A) Graphical expression of the logistic regression, Pr (HNSCC = 1) = logit−1 (b0 + b1 x methylation) in tissue from 76 participants with data overlain. The predictor methylation is the qMSP value for each case (1) and each control (0). Cutoff methylation values for PAX1, PAX5 ZIC4 and PLCB1 are shown by the vertical dotted line. Probability of HNSCC is shown in red; (B) Scatterplots of quantitative MSP analysis of candidate genes promoters in the Validation screen cohort, which consisted of 76 HNSCC tumor tissue samples and 19 normal tissue samples obtained from uvulopharyngopalatoplasty (UPPP) procedures performed in non-cancer patients. The relative level of methylated DNA for each gene in each sample was determined as a ratio of MSP for the amplified gene to ACTB and then multiplied by 1000 [(average value of duplicates of gene of interest / average value of duplicates of ACTB) x 1000] for, PAX1, PAX5, ZIC4, and PLCB1. Red line denotes cutoff value; (C) Sensitivity, Specificity and AUC results for qMSP analysis; (D) Receiver Operator Characteristics (ROC) curve for promoter methylation of PAX1, PAX5, ZIC4 and PLCB1 genes in the validation cohort. The figure shows that for this four gene panel the qMSP results have 96% sensitivity, 94% specificity, a 0.97 AUC and a Positive Predictive Value of 98.5%.](/cms/asset/2ba2f13e-aa90-40ce-99e6-7ee85b8ca91b/kepi_a_10929025_f0004.gif)
All samples harboring CDKN2A mutations had PAX1 methylation (P < 0.0001) as did most of TP53 mutated samples (P < 0.01). More than half of NOTCH1 (61.5%, P < 0.0001) mutated samples also exhibited PAX1 promoter methylation. All the samples with mutations in FBXW7 (P < 0.0001), and most of the samples with mutations in TP53 (79%, P < 0.0001), and NOTCH1 (92%, P < 0.0001) were methylated in the PAX5 promoter even after controlling for HPV-status and history of tobacco use (Table S8A).
We further corroborated our qMSP and somatic mutation results by analyzing The Cancer Genome Atlas (TCGA) publicly available data from 279 HNSCC patients (https://tcga-data.nci.nih.gov). PAX5 promoter methylation was associated with TP53 mutations (P = 0.02), while PAX1 promoter methylation was associated with NOTCH1 mutations (P < 0.0001), even after controlling for HPV-status and tobacco use. This evidence suggests a frequent occurrence of previously unreported interactions between PAX1 and PAX5 promoter methylation and exonic mutations in NOTCH1 and TP53 in HNSCC, respectively (Table S8B).
To provide additional evidence of expression downregulation of PAX1 and PAX5 in HNSCC we compared mRNA expression in HNSCC and UPPP samples. Differential transcript levels for PAX1 and PAX5 were confirmed by quantitative RT-PCR in some of the RNA samples used for microarray analysis and RNA samples from an independent set (Figs. 8A and B). Expression levels were studied in 13 HNSCC and 17 UPPP samples that were readily available. The relative expression levels showed consistency with the results obtained for PAX1 and PAX5 in the Discovery cohort with genome-wide methylation and mRNA expression platforms.
We also performed PAX5 knock-in and knock out studies in p53 wild type (p53wt) and p53 mutated (p53mut) HNSCC cell lines to assess the role of PAX5 as a tumor suppressor gene connected to the p53 pathway in HNSCC. We studied the functional consequences of PAX5 induction in 022(p53wt) and 22A(p53mut) HNSCC cell lines. Following transfection with (Myc-DDK-tagged)-Human paired box 5, 022 and 22A cells exhibited a dramatic decrease in cell proliferation and PAX5 expression levels were significantly increased, when measured 48h after transient transfection (Fig. S9).
Conversely, 22B(p53mut) HNSCC cells showed modest increase in cell proliferation compared with the control, when PAX5 was inhibited by siRNA following transfection with PAX5 siRNAs. Expression levels were significantly decreased, 48h after transient transfection (Fig. S10).
Proposed pathway interplay
After gene set enrichment analysis revealed that concurrent promoter methylation, mutations, and differential gene expression impacted cell differentiation, proliferation, growth and renewal pathways we performed a review study of the most important, potentially impacted cancer pathways in HNSCC. We found evidence of a strong interplay between somatic mutations in p53 and NOTCH1 and gene downregulation associated to PAX1 and PAX5 promoter methylation in HNSCC.
p53-PAX5
Our literature search revealed that p53-PAX5 interactions are implicated in apoptotic and/or proliferating signals. p53 is a downstream target for PAX proteins.Citation26,Citation27 The human p53 gene harbors a PAX binding site within its un-translated first exon that is conserved throughout evolution, which suggests the importance of this interaction. Frequent promoter methylation of PAX5 has been reported in ductal carcinoma in situ, invasive breast cancer, and neuroendocrine carcinomas.Citation28,Citation29 Furthermore, PAX5 has been reported to function as a tumor suppressor gene in hepatocellular carcinomaCitation30 and gastric cancer,Citation31 directly binding to the p53 promoter (Fig. S11). PAX5 also plays an important role in the commitment of lymphoid progenitors to the B lymphocyte lineage.Citation32 The mechanism through which PAX5 acts in B-cell differentiation is well established.Citation33-Citation35 Recent studies identified some of these interactions also in solid tumors, but little has been shown so far ().Citation36-Citation38 In our Discovery cohort we identified high frequency of PAX5 promoter methylation in HNSCC, coinciding with low expression levels, a finding that supports a TSG function.
Figure 5. Proposed genomic and epigenomic interactions in HNSCC: (A) Proposed partial pathway interplay of p53 and PAX5 in HNSCC. Downregulation of PAX5 leads to differentiation. When methylated, PAX5 an upstream target of p53, fails to activate the later which is also silenced due to mutations, and thus DNA repair, Apoptosis, and Growth Arrest pathways are inactive; (B) PAX1-NOTCH1 interplay through crosstalk of Hedgehog and Notch pathways in cell differentiation and proliferation signals. Notch1 induces p21 expression, either directly through the canonical pathway or indirectly through Hes1 and NFAT activation, leading in both cases to cell cycle arrest. Active Notch1 targets either the Hox family or Hes1. Hes1 is active and will block differentiation. The HOX family of transcription factors, downstream targets of Notch signaling, is frequently silenced, thus blocking the activation of PAX1 which is also downregulated in HNSCC and will not promote differentiation. PAX1 expression can also be induced by Shh through Gli2, which is active. Finally, proliferation is promoted through Gli2 interaction with CCND1 and CCND2.
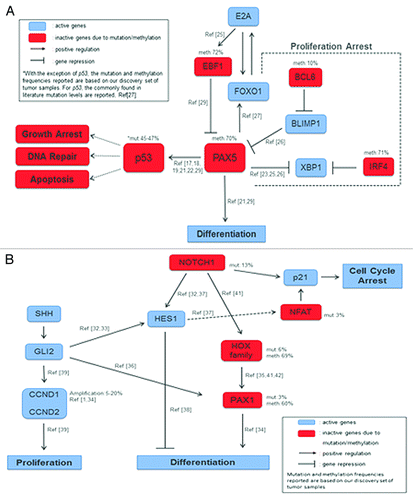
NOTCH1-PAX1
Agrawal et al. revealed inactivating mutations in NOTCH1 gene, depicting its importance in HNSCC, proposing also a tumor suppressor function in this particular type of tumors.Citation1 When NOTCH1 acts as a TSG it inhibits proliferation and promotes entry to differentiation.Citation39 Targeting the Notch proliferation pathway is really difficult. Preliminary data in cell lines showed that downregulation of NOTCH1 in NOTCH1 mutant cell lines leads to a modest effect of cell cycle acceleration and anti-NOTCH1 agents are only effective in NOTCH1 wild type cell lines.Citation40 In our study, PAX1 was found to be the most frequently methylated and downregulated gene. In addition to that, several HOX family genes, some of which are known to interact with NOTCH signaling, were prominent in our list of methylated genes in HNSCC (Fig. S12). Our evidence suggests an interaction between NOTCH1 and PAX1 through the HOX family of transcription factorsCitation39,Citation41-Citation43 and also with the Hedgehog pathway through Hes1, in which the well-established CCND1 amplification plays an important role.Citation44-Citation48 PAX1 plays a role in sclerotome differentiation and has been shown to interact with homeobox genes which play a prominent role in normal development and the control of cell proliferation.Citation49 Retinoid acid (RA) signaling acts via Hox gene pathways,Citation50,Citation51 some of which are able to regulate PAX1 through canonical NOTCH1 expression. These interactions are described in .
Discussion
We have conducted the first comprehensive integrated genomic and epigenomic analysis in HNSCC, focusing on identifying TSG genes that demonstrate concurrent promoter methylation with downregulation of expression and somatic mutations. Recent studies in HNSCC, published as we were finalizing this manuscript, focused on therapeutic pathways affected by somatic mutations and copy number alterations,Citation52-Citation54 but only described the clustering effects of DNA methylation at a global genome wide level.Citation52 We performed the first detailed genome wide analysis of the HNSCC methylome that studied expression alterations associated with differential methylation patterns in the greater promoter region, focusing on key TSGs that are also inactivated by somatic mutations. The main focus of this paper was to identify the number of tumor suppressor genes differentially methylated in the greater promoter region and mutated in HNSCC, and examine their combined impact in expression downregulation. We controlled for chromosomal deletions using CNV data previously generated in the Discovery cohort, to distinguish if expression alterations were related to methylation events or to deletions in specific regions of the chromosome.
Many genes with dense promoter methylation and downregulation of expression are candidate TSGs. However, genes inactivated by both somatic mutations and promoter methylation are likely to be key drivers of oncogenesis. Our analysis identified 10 downregulated genes inactivated by somatic mutation and promoter methylation. We selected PAX1, ZIC4, PLCB1, and PAX5 for further validation and we were indeed able to detect stark differences in DNA methylation levels between cancer and UPPP samples. ZIC4 and PLCB1 were the genes with the lowest methylation frequency in HNSCC, exhibiting also low mutation frequency, and yet, we confirmed ZIC4 and PLCB1 promoter methylation differences between normal and cancer samples in the Validation cohort.
PAX1 and PAX5 were highlighted as key genes in this study as they belong to the same family of transcription factors and were both found to be methylated and downregulated in HNSCC. PAX1 and PAX5 are genes involved in differentiation/proliferation signals and the gene set enrichment analysis performed clearly depicted these signaling pathways as deregulated in HNSCC in our discovery set of tumors.
We observed interesting relationships among the most commonly mutated and methylated genes in HNSCC: 61.5% of the NOTCH1 mutated samples also exhibited PAX1 methylation and 79% of the samples carrying TP53 mutations were also methylated in the PAX5 gene promoter. External validation in 279 primary HNSCC samples from the TCGA project verified our initial findings. Combined, this evidence suggests the frequent occurrence of previously unreported interactions between PAX1 and PAX5 promoter methylation and exonic mutations in NOTCH1 and TP53 in HNSCC.
The greater promoter PAX1 and PAX5 methylation levels reported in this manuscript were obtained with three different platforms that use diverse technology, chemistry, sample preparation and data analysis pipelines to obtain methylation values. We have combined these three platforms into a very robust methylation detection pipeline.
PAX1 and PAX5 methylation levels listed in the Discovery cohort are both, from MBD-seq and the 450K BeadChip assay. The 450K results were used to confirm the MBD-seq results in the subset of ten Discovery samples that were sequenced and, by proxy, validate the methylation results of the other 22 Discovery cohort samples that were only queried with the 450K arrays. The levels of PAX1 and PAX5 methylation levels listed for the Prevalence cohort were obtained with quantitative methylation specific PCR (qMSP). The levels of PAX1 and PAX5 listed for TCGA were obtained with the 450K BeadChip assay. The difference in PAX1 methylation between HPV positive and HPV negative tumors was significant in the Discovery cohort. The difference between the two in the Prevalence cohort was not significant (P = 0.225), perhaps due to the small sample size of HPV positive patients.
PAX genes, a family of nine transcription factors which act as cell lineage specific regulators of the tissues where they are normally expressed, are now also recognized as important factors in cancer progression. PAX genes, similarly to the NOTCH gene family, may play previously unrecognized fundamental roles in balancing proliferation and differentiation signals, two conceptually opposite cellular processes in canonical cancer research. The PAX family of genes may ultimately, following Waddington’s epigenetic landscape metaphor, be part of an epigenomic mediated switch between cancer initiation and cancer maintenance pathways, which stochastically drive cancer progression, immune system avoidance, acquisition of tumor resistance, and establishment of metastatic disease. Loss of ΝOTCH1 function due to mutation, or mutation/methylation-dependent silencing of downstream genes, such as PAX1 or the HOX family genes, is likely to abrogate normal cell differentiation.
Clinically, TP53 mutation has been shown time and again to be among the worst molecular alterations in patients with HNSCC.Citation36,Citation55 Patients that harbor p53 mutant tumors are more likely to relapse after complete resection and radiation therapy.Citation5 We confirmed a high frequency of PAX methylated tumors in 279 HNSCC tumor samples from the TCGA cohort and found that tumors which already harbor a p53 mutation, also harbor PAX5 promoter methylation.
Together, our results support the notion that differential promoter methylation and somatic mutations are the main cause of gene inactivation and pathway deregulation in HNSCC. We have unveiled hitherto unknown interactions between mutated and methylated genes that are associated with gene expression alterations in HNSCC. Characterization of the complete HNSCC methylome has contributed insights into the clustering of specific genetic and epigenetic events in the greater promoter region and highlights the importance of understanding the relative contribution of each to the overall frequency of TSG inactivation. We plan future in vitro studies focused on the functional consequences of the inactivation of these high frequency genes and will further explore the above proposed gene interactions. Understanding the complete contribution of genomic and epigenomic alterations to specific genes and pathways in cancer will reveal novel high frequency specific markers for better risk assessment (as we observed in the TCGA cohort above) and will highlight the true frequency of therapeutic pathways to better target the disease at the molecular level.
Materials and Methods
Participants
Patient selection
Head and Neck Squamous Cell Carcinoma (n = 91) and uvulopalatopharyngealplasty (UPPP) patients (n = 35) were consented for this study at the Johns Hopkins Medical Institutions hospitals and MD Anderson Cancer Center. The study was approved by the Ethics Committee of each participating hospital, as well as by the Johns Hopkins Institutional Review Board.
Johns Hopkins component
Fresh-frozen surgically resected tissue and matched blood were obtained from patients at Johns Hopkins Medical Institutions in Baltimore. Tissue was analyzed by frozen section histology to estimate neoplastic cellularity. In order to enrich the samples for neoplastic cells, normal tissue was removed from the samples using macro-dissection based on the frozen section histology. HPV tumor status was determined for oropharyngeal tumors per standard clinical care using in situ hybridization. Hybridization was performed using the HPV III Family16 probe set that captures HPV genotypes 16, 18, 33, 35, 45, 51, 52, 56, and 66. HPV16-positive controls included an HPV16-positive oropharyngeal cancer and the SiHa and CaSki cell lines. HPV tumor status was also determined by E6/E7 PCR primer amplification.
MD Anderson Component
Fresh-frozen surgically resected tumor and matched non-malignant adjacent tissue were obtained from consented patients treated for HNSCC at the University of Texas MD Anderson Cancer Center, under an Institutional Review Board approved protocol. Frozen tissue was embedded in optimal cutting temperature compound and cryosections from the top and middle of specimens were stained with hematoxylin and eosin prior to being evaluated by a pathologist for the presence of >60% tumor nuclei content or absence of tumor (i.e., normal). Samples that passed this criterion were sectioned all the way through and washed once in PBS prior to isolating genomic DNA using an ArchivePure DNA purification kit.
Methylated binding domain sequencing (MBD-seq)
Preparation of libraries
Tissue samples were digested with 1% SDS and 50 μg/mL proteinase K (Boehringer Mannheim) at 48 °C overnight, followed by phenol/chloroform extraction and ethanol precipitation of DNA. Two micrograms of DNA were sonicated to a modal size of ~150–250 bp, and end-repaired using the NEBNext SOLiD DNA library preparation kit end-repair module following the manufacturer's protocol (New England Biolabs). After column-purification (using the Qiagen PCR purification kit), SOLiD P1 and P2 adapters lacking 5′ phospate groups (Life Technologies) were ligated using the NEBNext adaptor ligation module and column-purified, and subjected to nick-translation by treating with Platinum Taq polymerase to remove the nick.
Affinity enrichment and capture of methylated DNA fragments
The resulting library was divided into two fractions, a total input fraction, and an enriched methylated fraction. The enriched methylated fraction was then subjected to affinity enrichment of methylated DNA fragments by using 6xHis-MBD2-MBD polypeptides immobilized on magnetic beads as described previously.Citation56,Citation57 The resulting enriched methylated fraction and the total input fraction were then subjected to library amplification using the NEBNext amplification module according to the manufacturer’s protocols, using 4–6 cycles for the total input, and 10–12 cycles for the enriched methylated fractions. Library fragments that were between 200–300 bp were size selected after agarose gel electrophoresis.
Massively parallel sequencing of MBD-seq libraries
The libraries were then subjected to emulsion PCR and bead enrichment following the SOLiD emulsion PCR protocol (Life Technologies). The resulting beads were then deposited on the SOLiD flow cell and subjected to massively parallel 50 bp single-read sequencing on a SOLiD v4.0 sequencer octet segment, with one octet segment for the total input and another one for the enriched methylated fraction. The details of the sequencing output for each sample from the sequencing run (number of tags, coverage, etc.) are provided in Table S9. Reads were aligned to hg19 using default settings in bioscope v1.3, with the exception of the bam output method, which was changed to alignment score.
Bioinformatics analysis of MBD-seq data
MACS analysis and identification of differential methylation
For the purposes of the analysis we divided the genome into two broad regions: the greater promoter, was defined as the region encompassing 6000 bases upstream and 1500 bases downstream from the transcription start site (TSS). From the functional genome distribution standpoint the greater promoter region, includes CpG sites in the proximal promoters, 1500 bases upstream from the described TSS, and 1500 bases downstream from the TSS, in the 5′ untranslated region and exon1. From the CpG content and neighborhood context the differentially methylated CpGs in HNSCC were located in CpG islands, CpG shores (regions 2000 bp upstream and downstream of but not inside CpG islands), CpG shelves (regions 2000 bp upstream and downstream of but not inside the shores), or as isolated CpGs in the area of the genome now defined as Open Sea. Methylated regions were identified as peaks of aligned sequencing tags in the enriched compared with total input fraction using MACS v1.4 software,Citation22,Citation58,Citation59 which allows identification of peaks after accounting for both global and local biases using the total input fraction. Peaks of methylation were identified for each sample separately.
To identify differentially methylated regions we first used stringent parameters to define presence and absence of methylation in EACH sample as follows: we used a low MACS P value cut-off (P < 10–6) to identify regions that are methylated, and another cut-off (MACS P > 10–2) to identify those regions that have very little evidence for methylation.
Next, for any given comparison of group A vs. group B (e.g., Group A = all tumors; Group B = all normals), we identified all regions that showed absence of methylation in all samples of Group A and presence of methylation in at least one sample from group B. All such overlapping regions across samples with peak calls in Group B were then merged, and the number of such samples and the lowest p-value of the peaks for these samples were recorded as the aggregate differentially methylated region. This analysis therefore yields regions in which all samples in Group A have absence of methylation peaks, and at least one sample in Group B has a methylation peak. The converse comparisons (i.e., absence of methylation in Group B, with at least one sample having a methylation peak in Group A) were also performed to obtain regions showing both gain of- and loss of-methylation.
Methylation bumphunting for identification of differentially methylated regions
Differential methylation was also identified using an independent approach called bumphunting that has been previously used to identify differential peaks in methylation data. Methylation bumphunting is a data analysis pipeline that effectively models measurement error, removes batch effects, detects regions of interest and attaches statistical uncertainty to regions identified as differentially methylated.Citation23 These methods are implemented in the bumphunter Bioconductor package and described in more detail in the section “Bioinformatics for 450K data.” Reported functionally relevant findings have been generally associated with genomic regions rather than single CpGs, either CpG islands,Citation60 CpG island shores,Citation20 genomic blocks,Citation61 or generic 2-kb regions.Citation62 Epigenomic bumps may have greater variability in size and shape than MBD-seq peaks.
Integration of MACS and bumphunting results
To identify the promoter regions that are differentially methylated in HNSCC when compared with normal oral mucosa, we intersected the list of methylated probes that discriminated between tumor and normal tissue identified with MACS with the list of methylated regions that discriminated between tumor and normal tissue identified with bumphunting. We used R (v3.00) to analyze the correlation of methylated promoter regions and HNSCC etiological factors.
Verification of MBD-seq results with HumanMethylation450K DNA BeadChip assay
450K array description and sample preparation
The 450K is a two-color array that detects cytosine methylation at 485, 512 methylation loci, mostly at CpGs, but also at a small number of cytosine residues outside of the CpG context, using bisulfite converted DNA. For each individual CpG two different signals are detected. One signal measures the amount of methylated DNA (Meth) and the other one measures the amount of unmethylated DNA (Unmeth). The Meth and Unmeth signals are measured with two different assays called “Type I” design or a “Type II” design. A β (β) value is generated by both Type I and Type II design probes to denote the methylation level of the CpG loci using the ratio of intensities between Meth and Unmeth (β value = methylation intensity / methylation + unmethylated intensity of the given CpG locus). Each methylation locus is interrogated by one of these designs. For a type I locus the Meth and Unmeth signals are measured by two paired probes, with a given locus using either the red or green signal from these probes. Type II loci are assayed using a single probe, with Meth and Unmeth signals derived from the green and red channels respectively. In addition to the methylation loci, the array contains a small number of control probes and 65 probes measuring common SNPs, intended for sample tracking. Type II probes use only one probe per methylation locus and hence allows more loci on the array, at a fixed array size. However, due to the chemistry used by the type II probe design, type II probes can only tolerate up to three CpGs within the 50bp probe. The type I design tolerates more CpGs within the 50bp probe, but assumes that all methylation loci in the probed sequence are in the same state.Citation63
Bisulfite modification of genomic DNA (2 μg) was performed with EpiTect Bisulfite Kit (QIAGEN) according to the manufacturer’s protocol. We hybridized bisulfite converted DNA from normal (UPPP) tissue (n = 16) and Head and Neck Squamous Cell Carcinoma (HNSCC) tissue (n = 31) samples to the 450K array.
Bioinformatics for 450K data
Bioinformatics strategies were used for background correction, normalization, and data analysis of differentially methylated genomic regions between tumor, and normal tissue. As with the analysis of methylation sequencing data we used two different analytic pipelines: a pipeline designed to capture the variability in methylated signals across the arrays using an F-testCitation11 and the bumphunting pipeline.Citation23 We used the minfi and bumphunter packages found in Bioconductor to perform background correction, normalization, and data analysis of differentially methylated genomic regions between tumor and normal tissue. The minfi package provides tools for analyzing Illumina’s Methylation arrays, with a special focus on the new 450k array for humans, and includes methods for preprocessing, quality assessment, and detection of differentially methylated regions from the kilobase to the megabase scale.Citation64 The bumphunter package is meant to work on data with several biological replicates, similar to the lmFit function in limma. While bumphunter is written using genomic data as an illustrative example, most of it is generalizable to other data types (with some one-dimensional location information).
F-test analytic pipeline
The selection of significantly methylated CpGs in the Illumina 450K Infinium assay data was performed in a stepwise manner. The 450.idat files were preprocessed and background corrected with the preprocessIllumina function in minfi to obtain β values. An F-test was performed across all 47 samples to identify CpGs with a significant difference in β values between normal and malignant tissue. Since the empirical P values were calculated genome-wide, adjustment for multiple testing was performed. Q-values were computed from the empirical P values using the Benjamin and Hochberg correction. Probes with q-values less than 0.05 were deemed statistically significant and were included in the CpG list. We then selected only those CpGs that showed a methylation difference of at least 0.25 between cancer and normal tissues and a β value of at least 0.3 in cancer, as previously described.Citation11 All bioinformatics analyses were performed using R version 3.0.
450K-bumphunting analytic pipeline
We performed pre-processing with the minfi package, which applies a version of subset quantile normalization to the Meth and Unmeth intensities separately. The distribution of type I and type II probes is forced to be the same by first quantile normalizing the type II probes across samples and then interpolating a reference distribution to which the type I probes are normalized. For the probes on the X and Y chromosomes the males and females are normalized separately. Sex is determined by the getSex function using copy number information. The stratified quantile normalization method is implemented by the preprocessQuantile function (the function does no background correction and removes zeros using the fix2 MethOutlier function). This algorithm relies on the assumptions necessary for quantile normalization and involves both within- and between- sample normalization.Citation64
Integration of F-test and 450K-bumphunting results
To identify the promoter regions that are differentially methylated in HNSCC when compared with normal oral mucosa, we intersected the list of methylated probes that discriminated between tumor and normal tissue identified with the F-test with the list of methylated regions that discriminated between tumor and normal tissue identified with 450K-bumphuntig.
E. mRNA expression arrays
Total RNA was isolated from normal (UPPP) tissue (n = 16) and Head and Neck Squamous Cell Carcinoma (HNSCC) tissue (n = 16) samples by using Tri-reagent. cDNA was made, and hybridized to Affymetrix GeneST1.0 Arrays (Affymetrix) according to manufacturer’s instructions. Six of these samples were also sequenced with MDB-seq. The data obtained from CEL files was background corrected with RMA, quantile normalized before an ANOVA was used to determine the Fold Change difference in log-transformed intensities between Tumor and Normal samples.
Analysis of functional annotation
Enrichment analysis of functional themes (Analysis of Functional Annotation, AFA) was performed to capture biological processes over-represented in the various conditions under investigation. This unbiased computational approach, conceptually similar to Gene Set Enrichment Analysis (GSEA),Citation65 enables the interpretation of genome-wide data through the identification and visualization of information encompassing distinct biological concepts, and was previously used successfully to integrate and interpret both differential gene expression and methylation data.Citation25,Citation66 A chi-square test of independence was applied to test whether each Functional Gene Set (FGS) was over-represented in any of the gene list associated with any of the investigated contrasts/conditions (e.g., gene associated with methylated promoters in HNSCC). In the present study, individual, non-redundant genes, as annotated in the NCBI Entrez gene database (R/Bioconductor package org.Hs.eg.db version 2.4.6) were used as the total gene space, and contingency tables were used to identify gene sets over-represented in the investigated conditions. Correction for multiple hypothesis testing was obtained separately for each FGS collection, by applying the Benjamini and Hochberg methodCitation67 as implemented in the multtest R/Bioconductor package. Overall, this approach is analogous to Gene Set Enrichment Analysis (GSEA),Citation65,Citation68,Citation69 and has already been successfully applied in other studies.Citation66,Citation70 The heatmaps’ color bar represents the negative log10 False Discovery Rate (FDR). For each gene set collection the sets for which at least one condition showed FDR < 0.01 were reported. The top 150 conditions were reported when too many gene sets where retrieved.
AFA was used to compare biological themes enriched in the following gene lists: (1) mutated genes; (2) genes with hyper-methylated promoters; (3) genes showing hyper-methylation outside the greater promoter region; (4) genes upregulated in cancer when compared with normal; and (5) genes downregulated in cancer when compared with normal. Gene set enrichment was assessed by testing for gene set over-representation with a chi-square test, because each gene list was obtained from diverse analyses using different methods. In the AFA analysis each gene was counted only once, while using the totality of the genes annotated to the NCBI Entrez gene database as the background gene space. For this reason there was no confounding with the number of probes or the length of the genes (i.e., each gene was counted only once irrespective to the number of probes or its length). The GO categories reported in Table S6 encompass different biological processes and contain a fairly large number of distinct genes. Such genes show variable length, from short to long transcripts, and all possible and disparate genomic arrangements, with genes located in both “gene-rich” and “gene-poor” genomic regions.
Validation of genomic and epigenomic alterations
Quantitative methylation specific PCR (qMSP) in the prevalence cohort
qMSP was used for validation in a Prevalence cohort of 76 tumors in which we had previously identified somatic mutations in TP53, NOTCH1, CDKN2A, PIK3CA, FBXW7, and HRAS and in 19 UPPP normal control tissue samples. We examined promoter methylation in three of the genes that were included in our final list of mutated and methylated genes and that were methylated in at least 40% of the Discovery Cohort samples: PAX1, PAX5, PLCB1, and ZIC4. Bisulfite-modified DNA was used as a template for fluorescence-based real-time PCR, as previously described.Citation71
Contingency tables of mutational and methylation events in TCGA data set
Publicly available HNSCC Illumina 450K methylation and exome sequencing data was downloaded from the TCGA website (http://cancergenome.nih.gov) and the cBioPortal for Cancer Genomics (www.cbioportal.org/public-portal/) using R (v3.0.0). Publicly available exome mutation data for TP53 and NOTCH1 and β values for all PAX1 and PAX5 450K array probes were extracted for all HNSCC samples analyzed by the TCGA project that had paired methylation and mutation data for the genes of interest (n = 279). Only the 450K probes located in TSS1500, TSS200, and 1st exon, as per the manufacturer’s annotation, were used to create the contingency tables for methylation and mutation analyses. Contingency tables were used to examine the association between exonic mutations of TP53, CDKN2A, HRAS, FBXW7, and NOTCH1 and promoter methylation of ZIC4, PAX1, PAX5, and PLCB1. The MacNemar test for paired data was implemented in R (v3.0.0) to evaluate the association between mutations and promoter methylation.
Quantitative real-time reverse transcription PCR
HNSCC RNA samples from the Discovery cohort and from an independent cohort were assessed for PAX5, PAX1, and GAPDH expression levels using quantitative real-time reverse transcription (RT)-PCR (TaqMan). Reverse transcription was performed with random hexamer primers and Superscript II Reverse Transcriptase (Invitrogen Corp.) according to manufacturer’s instructions. Quantitative RT-PCR was then performed on the Applied Biosystems 7900 Sequence Detection Instrument (Applied Biosystems) using TaqMan expression assays (Life Technologies).
Functional studies in cell lines
Human HNSCC cell lines with known p53 status were cultured to determine PAX5 methylation and expression levels. Human HNSCC cell lines 022 (p53 wt), 22A (p53 mut), and 22B (p53 mut) were selected for functional studies based on their expression and methylation results (Fig. S13). Cell growth conditions were maintained at 37 °C in an atmosphere of 5% CO2.
Transient transfection, PAX5 inhibition or overexpression, and cell proliferation assay
We knocked down PAX5 in the 22B cell line using the ON-TARGETplus Pool of siRNAs against PAX5 and a non-targeting Pool of siRNAs (Thermo Scientific) as control. Cells were seeded in 96-well plates and allowed to grow until approximately 70% confluence. Cells were transfected with siRNA using Lipofectamine RNAiMAX Reagent (Invitrogen) and cell metabolic activity was determined every 24 h using the CCK-8 colorimetric assay (Dojindo). Values are mean ± SEM for pentaplicates of cultured cells. The transfection efficiency was confirmed by quantitative real-time RT-PCR as described above and normalized to GAPDH at 48-h time point. Pax5 knockdown in 22B cells showed a modest increase in cell proliferation compared with the control.
Forced expression of PAX5 in 022 and 22A cell lines was performed. The pCMV-Entry-EV (control) and the (Myc-DDK-tagged)-Human paired box 5 (PAX5) from ORIGENE were transfected into cells (FUGENE HD Promega) which were then seeded in 96-well plates and allowed to grow until approximately 70% confluence. Cell metabolic activity was determined every 24 h using the CCK-8 colorimetric assay (Dojindo). Values are mean ± SEM for pentaplicates of cultured cells. The transfection efficiency was confirmed by quantitative real-time RT-PCR as described above and normalized to GAPDH at the 48-h time point. 022 and 22A cells exhibited a dramatic decrease in cell proliferation after forced expression of PAX5 48h after transfection compared to controls.
Additional material
Download Zip (1.2 MB)Disclosure of Potential Conflicts of Interest
J.A.C. is the Director of Research of the Milton J. Dance Head and Neck Endowment. V.E.V. is a co-founder of Inostics and Personal Genome Diagnostics and is a member of their Scientific Advisory Boards. V.E.V. owns Inostics and Personal Genome Diagnostics stock, which is subject to certain restrictions under University policy. The Johns Hopkins University in accordance with its conflict of interest policies is managing the terms of this arrangement.
Acknowledgments
The authors wish to thank Rafael Irizarry for his supervision in our use and interpretation of minfi and bumphunter analytic platforms. We also want to thank A. Jaffe, M. Haffner, T. Mosbruger, S. Wheelan, L. Danilova, C. Talbot and A. Jedlicka for their expert assistance.
Financial Support
National Cancer Institute grants U01CA84986 and K01CA164092 and CA121113; National Institute of Dental and Craniofacial Research grants P50DE019032 Head and Neck Cancer SPORE, and RC2 DE20957, and the Commonwealth Fund supported this research. The funders have no role in study design, data collection and analysis, decision to publish, or preparation of the manuscript.
References
- Agrawal N, Frederick MJ, Pickering CR, Bettegowda C, Chang K, Li RJ, Fakhry C, Xie TX, Zhang J, Wang J, et al. Exome sequencing of head and neck squamous cell carcinoma reveals inactivating mutations in NOTCH1. Science 2011; 333:1154 - 7; http://dx.doi.org/10.1126/science.1206923; PMID: 21798897
- Stransky N, Egloff AM, Tward AD, Kostic AD, Cibulskis K, Sivachenko A, Kryukov GV, Lawrence MS, Sougnez C, McKenna A, et al. The mutational landscape of head and neck squamous cell carcinoma. Science 2011; 333:1157 - 60; http://dx.doi.org/10.1126/science.1208130; PMID: 21798893
- Westra WH. The changing face of head and neck cancer in the 21st century: the impact of HPV on the epidemiology and pathology of oral cancer. Head Neck Pathol 2009; 3:78 - 81; http://dx.doi.org/10.1007/s12105-009-0100-y; PMID: 20596995
- Kim GB, Wang Z, Liu YY, Stavrou S, Mathias A, Goodwin KJ, Thomas JM, Neville DM. A fold-back single-chain diabody format enhances the bioactivity of an anti-monkey CD3 recombinant diphtheria toxin-based immunotoxin. Protein Eng Des Sel 2007; 20:425 - 32; http://dx.doi.org/10.1093/protein/gzm040; PMID: 17693455
- Poeta ML, Manola J, Goldwasser MA, Forastiere A, Benoit N, Califano JA, Ridge JA, Goodwin J, Kenady D, Saunders J, et al. TP53 mutations and survival in squamous-cell carcinoma of the head and neck. N Engl J Med 2007; 357:2552 - 61; http://dx.doi.org/10.1056/NEJMoa073770; PMID: 18094376
- Ang KK, Harris J, Wheeler R, Weber R, Rosenthal DI, Nguyen-Tân PF, Westra WH, Chung CH, Jordan RC, Lu C, et al. Human papillomavirus and survival of patients with oropharyngeal cancer. N Engl J Med 2010; 363:24 - 35; http://dx.doi.org/10.1056/NEJMoa0912217; PMID: 20530316
- Kelloff GJ, Lippman SM, Dannenberg AJ, Sigman CC, Pearce HL, Reid BJ, Szabo E, Jordan VC, Spitz MR, Mills GB, et al, AACR Task Force on Cancer Prevention. Progress in chemoprevention drug development: the promise of molecular biomarkers for prevention of intraepithelial neoplasia and cancer--a plan to move forward. Clin Cancer Res 2006; 12:3661 - 97; http://dx.doi.org/10.1158/1078-0432.CCR-06-1104; PMID: 16778094
- Myers MF, Chang MH, Jorgensen C, Whitworth W, Kassim S, Litch JA, Armstrong L, Bernhardt B, Faucett WA, Irwin D, et al. Genetic testing for susceptibility to breast and ovarian cancer: evaluating the impact of a direct-to-consumer marketing campaign on physicians’ knowledge and practices. Genet Med 2006; 8:361 - 70; http://dx.doi.org/10.1097/01.gim.0000223544.68475.6c; PMID: 16778598
- Whitworth A. New research suggests access, genetic differences play role in high minority cancer death rate. J Natl Cancer Inst 2006; 98:669; http://dx.doi.org/10.1093/jnci/djj223; PMID: 16705120
- Svatek RS, Lee JJ, Roehrborn CG, Lippman SM, Lotan Y. The cost of prostate cancer chemoprevention: a decision analysis model. Cancer Epidemiol Biomarkers Prev 2006; 15:1485 - 9; http://dx.doi.org/10.1158/1055-9965.EPI-06-0221; PMID: 16896037
- Guerrero-Preston R, Soudry E, Acero J, Orera M, Moreno-López L, Macía-Colón G, Jaffe A, Berdasco M, Ili-Gangas C, Brebi-Mieville P, et al. NID2 and HOXA9 promoter hypermethylation as biomarkers for prevention and early detection in oral cavity squamous cell carcinoma tissues and saliva. Cancer Prev Res (Phila) 2011; 4:1061 - 72; http://dx.doi.org/10.1158/1940-6207.CAPR-11-0006; PMID: 21558411
- Carvalho AL, Chuang A, Jiang WW, Lee J, Begum S, Poeta L, Zhao M, Jerónimo C, Henrique R, Nayak CS, et al. Deleted in colorectal cancer is a putative conditional tumor-suppressor gene inactivated by promoter hypermethylation in head and neck squamous cell carcinoma. Cancer Res 2006; 66:9401 - 7; http://dx.doi.org/10.1158/0008-5472.CAN-06-1073; PMID: 17018594
- Demokan S, Chang X, Chuang A, Mydlarz WK, Kaur J, Huang P, Khan Z, Khan T, Ostrow KL, Brait M, et al. KIF1A and EDNRB are differentially methylated in primary HNSCC and salivary rinses. Int J Cancer 2010; 127:2351 - 9; http://dx.doi.org/10.1002/ijc.25248; PMID: 20162572
- Andrades P, Asiedu C, Ray P, Rodriguez C, Goodwin J, McCarn J, Thomas JM. Islet yield after different methods of pancreatic Liberase delivery. Transplant Proc 2007; 39:183 - 4; http://dx.doi.org/10.1016/j.transproceed.2006.10.016; PMID: 17275501
- Settle K, Posner MR, Schumaker LM, Tan M, Suntharalingam M, Goloubeva O, Strome SE, Haddad RI, Patel SS, Cambell EV 3rd, et al. Racial survival disparity in head and neck cancer results from low prevalence of human papillomavirus infection in black oropharyngeal cancer patients. Cancer Prev Res (Phila) 2009; 2:776 - 81; http://dx.doi.org/10.1158/1940-6207.CAPR-09-0149; PMID: 19641042
- Leemans CR, Braakhuis BJ, Brakenhoff RH. The molecular biology of head and neck cancer. Nat Rev Cancer 2011; 11:9 - 22; http://dx.doi.org/10.1038/nrc2982; PMID: 21160525
- Morris LG, Kaufman AM, Gong Y, Ramaswami D, Walsh LA, Turcan Ş, Eng S, Kannan K, Zou Y, Peng L, et al. Recurrent somatic mutation of FAT1 in multiple human cancers leads to aberrant Wnt activation. Nat Genet 2013; 45:253 - 61; http://dx.doi.org/10.1038/ng.2538; PMID: 23354438
- Scholtens D, Vidal M, Gentleman R. Local modeling of global interactome networks. Bioinformatics 2005; 21:3548 - 57; http://dx.doi.org/10.1093/bioinformatics/bti567; PMID: 15998662
- Serre D, Lee BH, Ting AH. MBD-isolated Genome Sequencing provides a high-throughput and comprehensive survey of DNA methylation in the human genome. Nucleic Acids Res 2010; 38:391 - 9; http://dx.doi.org/10.1093/nar/gkp992; PMID: 19906696
- Irizarry RA, Ladd-Acosta C, Wen B, Wu Z, Montano C, Onyango P, Cui H, Gabo K, Rongione M, Webster M, et al. The human colon cancer methylome shows similar hypo- and hypermethylation at conserved tissue-specific CpG island shores. Nat Genet 2009; 41:178 - 86; http://dx.doi.org/10.1038/ng.298; PMID: 19151715
- Mena E, Turkbey B, Mani H, Adler S, Valera VA, Bernardo M, Shah V, Pohida T, McKinney Y, Kwarteng G, et al. 11C-Acetate PET/CT in localized prostate cancer: a study with MRI and histopathologic correlation. J Nucl Med 2012; 53:538 - 45; http://dx.doi.org/10.2967/jnumed.111.096032; PMID: 22343504
- Feng J, Liu T, Zhang Y. Using MACS to identify peaks from ChIP-Seq data. Current protocols in bioinformatics / editoral board, Andreas D Baxevanis [et al] 2011; Chapter 2:Unit 2 14.
- Jaffe AE, Murakami P, Lee H, Leek JT, Fallin MD, Feinberg AP, Irizarry RA. Bump hunting to identify differentially methylated regions in epigenetic epidemiology studies. Int J Epidemiol 2012; 41:200 - 9; http://dx.doi.org/10.1093/ije/dyr238; PMID: 22422453
- Zhang X, Cruz FD, Terry M, Remotti F, Matushansky I. Terminal differentiation and loss of tumorigenicity of human cancers via pluripotency-based reprogramming. Oncogene 2013; 32:2249 - 60, e1-21; http://dx.doi.org/10.1038/onc.2012.237; PMID: 22777357
- Kortenhorst MS1, Wissing MD, Rodríguez R, Kachhap SK, Jans JJ, Van der Groep P, Verheul HM, Gupta A, Aiyetan PO, van der Wall E, et al. Analysis of the genomic response of human prostate cancer cells to histone deacetylase inhibitors. Epigenetics 2013; 8:907 - 20; http://dx.doi.org/10.4161/epi.25574; PMID: 23880963
- Stuart ET, Haffner R, Oren M, Gruss P. Loss of p53 function through PAX-mediated transcriptional repression. EMBO J 1995; 14:5638 - 45; PMID: 8521821
- O’Brien P, Morin P Jr., Ouellette RJ, Robichaud GA. The Pax-5 gene: a pluripotent regulator of B-cell differentiation and cancer disease. Cancer Res 2011; 71:7345 - 50; http://dx.doi.org/10.1158/0008-5472.CAN-11-1874; PMID: 22127921
- Moelans CB, Verschuur-Maes AH, van Diest PJ. Frequent promoter hypermethylation of BRCA2, CDH13, MSH6, PAX5, PAX6 and WT1 in ductal carcinoma in situ and invasive breast cancer. J Pathol 2011; 225:222 - 31; http://dx.doi.org/10.1002/path.2930; PMID: 21710692
- Torlakovic E, Slipicevic A, Robinson C, DeCoteau JF, Alfsen GC, Vyberg M, Chibbar R, Flørenes VA. Pax-5 expression in nonhematopoietic tissues. Am J Clin Pathol 2006; 126:798 - 804; http://dx.doi.org/10.1309/XEC7JMW9YRM74RNO; PMID: 17050077
- Liu W, Li X, Chu ES, Go MY, Xu L, Zhao G, Li L, Dai N, Si J, Tao Q, et al. Paired box gene 5 is a novel tumor suppressor in hepatocellular carcinoma through interaction with p53 signaling pathway. Hepatology 2011; 53:843 - 53; http://dx.doi.org/10.1002/hep.24124; PMID: 21319196
- Li X, Cheung KF, Ma X, Tian L, Zhao J, Go MY, Shen B, Cheng AS, Ying J, Tao Q, et al. Epigenetic inactivation of paired box gene 5, a novel tumor suppressor gene, through direct upregulation of p53 is associated with prognosis in gastric cancer patients. Oncogene 2012; 31:3419 - 30; http://dx.doi.org/10.1038/onc.2011.511; PMID: 22105368
- Cobaleda C, Schebesta A, Delogu A, Busslinger M. Pax5: the guardian of B cell identity and function. Nat Immunol 2007; 8:463 - 70; http://dx.doi.org/10.1038/ni1454; PMID: 17440452
- Mullighan CG, Goorha S, Radtke I, Miller CB, Coustan-Smith E, Dalton JD, Girtman K, Mathew S, Ma J, Pounds SB, et al. Genome-wide analysis of genetic alterations in acute lymphoblastic leukaemia. Nature 2007; 446:758 - 64; http://dx.doi.org/10.1038/nature05690; PMID: 17344859
- Mandel EM, Grosschedl R. Transcription control of early B cell differentiation. Curr Opin Immunol 2010; 22:161 - 7; http://dx.doi.org/10.1016/j.coi.2010.01.010; PMID: 20144854
- Todd DJ, Lee AH, Glimcher LH. The endoplasmic reticulum stress response in immunity and autoimmunity. Nat Rev Immunol 2008; 8:663 - 74; http://dx.doi.org/10.1038/nri2359; PMID: 18670423
- Chen Z, Xiao Y, Zhang J, Li J, Liu Y, Zhao Y, Ma C, Luo J, Qiu Y, Huang G, et al. Transcription factors E2A, FOXO1 and FOXP1 regulate recombination activating gene expression in cancer cells. PLoS One 2011; 6:e20475; http://dx.doi.org/10.1371/journal.pone.0020475; PMID: 21655267
- Palmisano WA, Crume KP, Grimes MJ, Winters SA, Toyota M, Esteller M, Joste N, Baylin SB, Belinsky SA. Aberrant promoter methylation of the transcription factor genes PAX5 alpha and beta in human cancers. Cancer Res 2003; 63:4620 - 5; PMID: 12907641
- Lagergren A, Manetopoulos C, Axelson H, Sigvardsson M. Neuroblastoma and pre-B lymphoma cells share expression of key transcription factors but display tissue restricted target gene expression. BMC Cancer 2004; 4:80; http://dx.doi.org/10.1186/1471-2407-4-80; PMID: 15544702
- Bolós V, Grego-Bessa J, de la Pompa JL. Notch signaling in development and cancer. Endocr Rev 2007; 28:339 - 63; http://dx.doi.org/10.1210/er.2006-0046; PMID: 17409286
- Mani S, Szymańska K, Cuenin C, Zaridze D, Balassiano K, Lima SC, Matos E, Daudt A, Koifman S, Filho VW, et al. DNA methylation changes associated with risk factors in tumors of the upper aerodigestive tract. Epigenetics 2012; 7:270 - 7; http://dx.doi.org/10.4161/epi.7.3.19306; PMID: 22430803
- Wall DS, Mears AJ, McNeill B, Mazerolle C, Thurig S, Wang Y, Kageyama R, Wallace VA. Progenitor cell proliferation in the retina is dependent on Notch-independent Sonic hedgehog/Hes1 activity. J Cell Biol 2009; 184:101 - 12; http://dx.doi.org/10.1083/jcb.200805155; PMID: 19124651
- Landsman L, Parent A, Hebrok M. Elevated Hedgehog/Gli signaling causes beta-cell dedifferentiation in mice. Proc Natl Acad Sci U S A 2011; 108:17010 - 5; http://dx.doi.org/10.1073/pnas.1105404108; PMID: 21969560
- Forastiere A, Koch W, Trotti A, Sidransky D. Head and neck cancer. N Engl J Med 2001; 345:1890 - 900; http://dx.doi.org/10.1056/NEJMra001375; PMID: 11756581
- Manley NR, Capecchi MR. The role of Hoxa-3 in mouse thymus and thyroid development. Development 1995; 121:1989 - 2003; PMID: 7635047
- Rodrigo I, Hill RE, Balling R, Münsterberg A, Imai K. Pax1 and Pax9 activate Bapx1 to induce chondrogenic differentiation in the sclerotome. Development 2003; 130:473 - 82; http://dx.doi.org/10.1242/dev.00240; PMID: 12490554
- Mammucari C, Tommasi di Vignano A, Sharov AA, Neilson J, Havrda MC, Roop DR, Botchkarev VA, Crabtree GR, Dotto GP. Integration of Notch 1 and calcineurin/NFAT signaling pathways in keratinocyte growth and differentiation control. Dev Cell 2005; 8:665 - 76; http://dx.doi.org/10.1016/j.devcel.2005.02.016; PMID: 15866158
- Sang L, Roberts JM, Coller HA. Hijacking HES1: how tumors co-opt the anti-differentiation strategies of quiescent cells. Trends Mol Med 2010; 16:17 - 26; http://dx.doi.org/10.1016/j.molmed.2009.11.001; PMID: 20022559
- Mill P, Mo R, Fu H, Grachtchouk M, Kim PC, Dlugosz AA, Hui CC. Sonic hedgehog-dependent activation of Gli2 is essential for embryonic hair follicle development. Genes Dev 2003; 17:282 - 94; http://dx.doi.org/10.1101/gad.1038103; PMID: 12533516
- Cillo C, Cantile M, Faiella A, Boncinelli E. Homeobox genes in normal and malignant cells. J Cell Physiol 2001; 188:161 - 9; http://dx.doi.org/10.1002/jcp.1115; PMID: 11424082
- Schubert M, Yu JK, Holland ND, Escriva H, Laudet V, Holland LZ. Retinoic acid signaling acts via Hox1 to establish the posterior limit of the pharynx in the chordate amphioxus. Development 2005; 132:61 - 73; http://dx.doi.org/10.1242/dev.01554; PMID: 15576409
- Koop D, Holland ND, Sémon M, Alvarez S, de Lera AR, Laudet V, Holland LZ, Schubert M. Retinoic acid signaling targets Hox genes during the amphioxus gastrula stage: insights into early anterior-posterior patterning of the chordate body plan. Dev Biol 2010; 338:98 - 106; http://dx.doi.org/10.1016/j.ydbio.2009.11.016; PMID: 19914237
- Turkbey B, Mani H, Aras O, Ho J, Hoang A, Rastinehad AR, Agarwal H, Shah V, Bernardo M, Pang Y, et al. Prostate cancer: can multiparametric MR imaging help identify patients who are candidates for active surveillance?. Radiology 2013; 268:144 - 52; http://dx.doi.org/10.1148/radiol.13121325; PMID: 23468576
- Turkbey B, Mani H, Aras O, Rastinehad AR, Shah V, Bernardo M, Pohida T, Daar D, Benjamin C, McKinney YL, et al. Correlation of magnetic resonance imaging tumor volume with histopathology. J Urol 2012; 188:1157 - 63; http://dx.doi.org/10.1016/j.juro.2012.06.011; PMID: 22901591
- Shah V, Turkbey B, Mani H, Pang Y, Pohida T, Merino MJ, Pinto PA, Choyke PL, Bernardo M. Decision support system for localizing prostate cancer based on multiparametric magnetic resonance imaging. Med Phys 2012; 39:4093 - 103; http://dx.doi.org/10.1118/1.4722753; PMID: 22830742
- Dal Molin M, Matthaei H, Wu J, Blackford A, Debeljak M, Rezaee N, Wolfgang CL, Butturini G, Salvia R, Bassi C, et al. Clinicopathological correlates of activating GNAS mutations in intraductal papillary mucinous neoplasm (IPMN) of the pancreas. Ann Surg Oncol 2013; 20:3802 - 8; http://dx.doi.org/10.1245/s10434-013-3096-1; PMID: 23846778
- Bock C, Tomazou EM, Brinkman AB, Müller F, Simmer F, Gu H, Jäger N, Gnirke A, Stunnenberg HG, Meissner A. Quantitative comparison of genome-wide DNA methylation mapping technologies. Nat Biotechnol 2010; 28:1106 - 14; http://dx.doi.org/10.1038/nbt.1681; PMID: 20852634
- Yegnasubramanian S, Wu Z, Haffner MC, Esopi D, Aryee MJ, Badrinath R, He TL, Morgan JD, Carvalho B, Zheng Q, et al. Chromosome-wide mapping of DNA methylation patterns in normal and malignant prostate cells reveals pervasive methylation of gene-associated and conserved intergenic sequences. BMC Genomics 2011; 12:313; http://dx.doi.org/10.1186/1471-2164-12-313; PMID: 21669002
- Zhang Y, Liu T, Meyer CA, Eeckhoute J, Johnson DS, Bernstein BE, Nusbaum C, Myers RM, Brown M, Li W, et al. Model-based analysis of ChIP-Seq (MACS). Genome Biol 2008; 9:R137; http://dx.doi.org/10.1186/gb-2008-9-9-r137; PMID: 18798982
- Feng J, Liu T, Qin B, Zhang Y, Liu XS. Identifying ChIP-seq enrichment using MACS. Nat Protoc 2012; 7:1728 - 40; http://dx.doi.org/10.1038/nprot.2012.101; PMID: 22936215
- Jaenisch R, Bird A. Epigenetic regulation of gene expression: how the genome integrates intrinsic and environmental signals. Nat Genet 2003; 33:Suppl 245 - 54; http://dx.doi.org/10.1038/ng1089; PMID: 12610534
- Hansen KD, Timp W, Bravo HC, Sabunciyan S, Langmead B, McDonald OG, Wen B, Wu H, Liu Y, Diep D, et al. Increased methylation variation in epigenetic domains across cancer types. Nat Genet 2011; 43:768 - 75; http://dx.doi.org/10.1038/ng.865; PMID: 21706001
- Lister R, Pelizzola M, Dowen RH, Hawkins RD, Hon G, Tonti-Filippini J, Nery JR, Lee L, Ye Z, Ngo QM, et al. Human DNA methylomes at base resolution show widespread epigenomic differences. Nature 2009; 462:315 - 22; http://dx.doi.org/10.1038/nature08514; PMID: 19829295
- Bibikova M, Barnes B, Tsan C, Ho V, Klotzle B, Le JM, Delano D, Zhang L, Schroth GP, Gunderson KL, et al. High density DNA methylation array with single CpG site resolution. Genomics 2011; 98:288 - 95; http://dx.doi.org/10.1016/j.ygeno.2011.07.007; PMID: 21839163
- Aryee MJ, Jaffe AE, Corrada-Bravo H, Ladd-Acosta C, Feinberg AP, Hansen KD, Irizarry RA. Minfi: a flexible and comprehensive Bioconductor package for the analysis of Infinium DNA methylation microarrays. Bioinformatics 2014; http://dx.doi.org/10.1093/bioinformatics/btu049; PMID: 24478339
- Kim SY, Volsky DJ. PAGE: parametric analysis of gene set enrichment. BMC Bioinformatics 2005; 6:144; http://dx.doi.org/10.1186/1471-2105-6-144; PMID: 15941488
- Tyekucheva S, Marchionni L, Karchin R, Parmigiani G. Integrating diverse genomic data using gene sets. Genome Biol 2011; 12:R105; http://dx.doi.org/10.1186/gb-2011-12-10-r105; PMID: 22018358
- Benjamini Y, Drai D, Elmer G, Kafkafi N, Golani I. Controlling the false discovery rate in behavior genetics research. Behav Brain Res 2001; 125:279 - 84; http://dx.doi.org/10.1016/S0166-4328(01)00297-2; PMID: 11682119
- Subramanian A, Tamayo P, Mootha VK, Mukherjee S, Ebert BL, Gillette MA, Paulovich A, Pomeroy SL, Golub TR, Lander ES, et al. Gene set enrichment analysis: a knowledge-based approach for interpreting genome-wide expression profiles. Proc Natl Acad Sci U S A 2005; 102:15545 - 50; http://dx.doi.org/10.1073/pnas.0506580102; PMID: 16199517
- Daniel VC, Marchionni L, Hierman JS, Rhodes JT, Devereux WL, Rudin CM, Yung R, Parmigiani G, Dorsch M, Peacock CD, et al. A primary xenograft model of small-cell lung cancer reveals irreversible changes in gene expression imposed by culture in vitro. Cancer Res 2009; 69:3364 - 73; http://dx.doi.org/10.1158/0008-5472.CAN-08-4210; PMID: 19351829
- Ross AE, Marchionni L, Vuica-Ross M, Cheadle C, Fan J, Berman DM, Schaeffer EM. Gene expression pathways of high grade localized prostate cancer. Prostate 2011; http://dx.doi.org/10.1002/pros.21373; PMID: 21360566
- Hoque MO, Begum S, Topaloglu O, Chatterjee A, Rosenbaum E, Van Criekinge W, Westra WH, Schoenberg M, Zahurak M, Goodman SN, et al. Quantitation of promoter methylation of multiple genes in urine DNA and bladder cancer detection. J Natl Cancer Inst 2006; 98:996 - 1004; http://dx.doi.org/10.1093/jnci/djj265; PMID: 16849682