Abstract
Allele-specific (AS) assessment of chromatin has the potential to elucidate specific cis-regulatory mechanisms, which are predicted to underlie the majority of the known genetic associations to complex disease. However, development of chromatin landscapes at allelic resolution has been challenging since sites of variable signal strength require substantial read depths not commonly applied in sequencing based approaches. In this study, we addressed this by performing parallel analyses of input DNA and chromatin immunoprecipitates (ChIP) on high-density Illumina genotyping arrays. Allele-specificity for the histone modifications H3K4me1, H3K4me3, H3K27ac, H3K27me3, and H3K36me3 was assessed using ChIP samples generated from 14 lymphoblast and 6 fibroblast cell lines. AS-ChIP SNPs were combined into domains and validated using high-confidence ChIP-seq sites. We observed characteristic patterns of allelic-imbalance for each histone-modification around allele-specifically expressed transcripts. Notably, we found H3K4me1 to be significantly anti-correlated with allelic expression (AE) at transcription start sites, indicating H3K4me1 allelic imbalance as a marker of AE. We also found that allelic chromatin domains exhibit population and cell-type specificity as well as heritability within trios. Finally, we observed that a subset of allelic chromatin domains is regulated by DNase I-sensitive quantitative trait loci and that these domains are significantly enriched for genome-wide association studies hits, with autoimmune disease associated SNPs specifically enriched in lymphoblasts. This study provides the first genome-wide maps of allelic-imbalance for five histone marks. Our results provide new insights into the role of chromatin in cis-regulation and highlight the need for high-depth sequencing in ChIP-seq studies along with the need to improve allele-specificity of ChIP-enrichment.
Introduction
Genome-wide association studies have revealed that ~93% of variants associated to complex traits lie in non-coding DNA, with an enrichment in DNase I hypersensitive sites (DHSs) shown to mark cis-regulatory elements, such as enhancers and promoters, suggesting the predominance of cis-regulatory mechanisms in mediating the common genetic risk associated with complex disease.Citation1 Allelic expression (AE) studies that associate phased genetic variants (e.g., SNPs) with allelic differences in transcription have proven to be powerful methods for specifically identifying cis-regulatory SNPs (cis-rSNPs). Paternal and maternal alleles function as within-sample controls, allowing for the assessment of regulatory variants independently of trans-acting effects.Citation2 In a recent comparison of expression quantitative trait loci (eQTL) vs. AE-mapping studies using the same samples, AE showed equivalent power to detect cis-rSNPs with an up to 8-fold smaller sample size compared with the eQTL approach.Citation3 Despite these recent advances in cis-rSNP detection, fine-mapping of cis-rSNPs to single causal variants is complicated by the fact that cis-rSNPs commonly lie in haplotype blocks of high linkage disequilibrium (LD). Since regulatory elements, where cis-rSNPs are likely to lie, are tightly correlated with chromatin state (e.g., histone modifications), it has been suggested that allele-specific characterization of the chromatin landscape could provide one tool for precisely identifying causal AE-mapped cis-rSNPs, which locally affect allelic chromatin state, and thereby associate to allelic differences in expression of nearby transcripts.Citation4,Citation5
The rapidly decreasing costs of next-generation sequencing along with the development of reagents to study DNA-protein interactions and other regulatory phenomena have allowed ENCODE and the Roadmap Epigenomics Project to map a diverse array of epigenetic marks in a range of cultured cell-lines and primary tissues.Citation6,Citation7 Studies utilizing this and other similar data sets have highlighted the role of open chromatin regions, histone modifications and transcription factor binding sites in regulating gene expression and/or disease risk, with variants in these regions frequently enriched for eQTLs and/or GWAS SNPs.Citation1,Citation8-Citation10 Open chromatin regions have previously been studied using DNase I, an enzyme which selectively cuts at nucleosome-depleted areas of the genome.Citation11 More recently, the DNase I protocol has been combined with next generation sequencing, in a procedure known as DNase-seq, to generate genome-wide maps of DNase I Hypersensitive Sites (DHSs).Citation12 Furthermore, in a recent study, Degner et al. performed DNase-seq on 70 LCL cell lines, from the HapMap Yoruba (YRI) population, which they combined with genotyping data to map variants affecting DHSs, by measuring DNase I hypersensivity as a quantitative trait, and termed these variants DNase I-sensitivity Quantitative Trait Loci (dsQTLs).Citation13
In theory, the most direct method of measuring a variant’s potential cis-regulatory effect on chromatin state is to assess differences in allelic chromatin activity within individuals heterozygous for the variant in a method analogous to AE-mapping. In this case, confounding effects due to environmental and trans-acting factors are removed. These factors should affect both alleles equally and are thus controlled for by comparing differences in binding between alleles within an individual. An early study piloted genome-wide assessment of allelic differences in RNA polymerase II (RNAP II) ChIP samples using genotyping arrays, as a method for uncovering imprinted genes and other allele-specific binding events. This proof-of-concept study applied low density genotyping arrays (~300K SNPs) and employed one ChIP antibody (RNAP II), therefore providing results primarily on the technical feasibility of the approach.Citation14 While a number of recent studies have investigated allelic bias in ChIP-seq data, the analyses are confined to a short list of sites, where sufficient read-depth exists to determine statistically significant differences in allelic binding.Citation15,Citation16 Higher read depths have been proposed as a potential long-term solution.Citation16 However, sequencing to sufficient depth to yield “full allelic resolution” is currently costly. Based on a recent study using high-depth ChIP-seq data from the comparatively small Drosophila genome, it is estimated that >400 million mapped reads would be required to reach saturation of all H3K4me1 peaks in the human genome.Citation17
Given genotyping arrays’ sensitivity to detect low levels of DNA (provided by target DNA amplification), and the recent availability of high density chips, containing probes for up to 5 million SNPs, we reasoned that an updated version of ChIP-SNP, using a diversity of informative post-translational modifications of histones in ChIP coupled with high-density genotyping arrays, would enable a cost-effective assessment of allelic chromatin state globally, at high resolution, for the evaluation of common regulatory variants associated to complex traits. Using the high-density, genome-wide AS-ChIP data, as well as allelic expression data generated in-house from 4 populations comprising 3 distinct cell-types, we were able to characterize allelic chromatin around allelically expressed transcripts for the first time, using data from the same cell-type/population. We identify a previously uncharacterized H3K4me1 anti-correlation at the TSS of allelically-expressed transcripts. We also demonstrate the heritability, population-dependent and cell-type specific components of allelic chromatin. In addition, we observe enrichments of DNase I hypersensitive sites, under genetic control, in allelic-chromatin domains suggesting a shared regulatory mechanism. Finally, we test allelic-chromatin domains for enrichment of disease-associated GWAS SNPs, and observe a LCL-specific enrichment of autoimmune-disease associated GWAS SNPs, with no enrichment in FBs.
Results
Allele-specific ChIP reveals an unprecedented number of allelically regulated SNPs
Cell-lines derived from 20 individuals from 3 populations were assessed via allele-specific ChIP: 2 trios of fibroblast (FB) cell-lines of European ancestry, 1 trio and 4 unrelated lymphoblastoid cell lines (LCLs) from the 1000 Genomes (1000G) CEU population (Utah residents of European ancestry), and 1 trio and 4 unrelated LCLs from the 1000G YRI population (Yoruban in Ibadan, Nigeria) (). The LCL samples were specifically chosen according to the availability of publicly available, high coverage, whole genome sequence data.Citation18,Citation19 Furthermore, our selection of trios from multiple cell-types and populations allowed us to assess the heritable, population-dependent and cell-type specific components of allelic-chromatin. ChIP was performed on all samples for the histone modifications: H3K4me1 (enhancers) and H3K4me3 (promoters). Ten individuals were further assayed for H3K27ac (active chromatin), H3K27me3 (inactive chromatin), and H3K36me3 (transcriptional elongation). ChIP samples were assessed on high-density Illumina genotyping arrays along with cDNA and gDNA samples, allowing for the assessment of ~4.4 million SNPs per sample (). For heterozygous SNPs, an AI value was calculated as previously described,Citation2 such that AI is equivalent to variation from bi-allelic 0.50:0.50 ratio. For example, an AI value of 0.05 would represent a 0.55:0.45 normalized allelic ratio, which corresponds to an approximately 1.2-fold difference in binding affinity. On average, 548 453 heterozygous SNPs per AS-ChIP sample met signal intensity cutoffs (see methods) for allelic-imbalance calculation, with 404621 being the lowest number of heterozygous SNPs assessed for any sample analyzed. On average, 22% of these SNPs had absolute AI values of at least 0.05 (), with 5% greater than 0.1, corresponding to a 1.5-fold difference. For full details on the number of SNPs analyzed for each sample, see Table S1.
Figure 1. AS-ChIP reveals high resolution maps of allelic imbalance for 5 histone modifications (A) Twenty cell lines from 3 cohorts comprising 2 distinct cell-types, 2 ancestral populations and 3 parent-child trios underwent AS-ChIP analysis. Fibroblast (FB) cell-lines came from 2 trios of Caucasian ancestry. LCL cell-lines came from 2 ‘1000 Genomes’ populations, CEU (Utah residents of European ancestry) and YRI (Individuals of Yoruban ancestry in Ibadan, Nigeria). From each of these two populations we selected 1 trio and 4 unrelated children (for which all parents’ phased genotypes were available for phasing). Ovals indicate females and rectangles indicate males. (B) Chromatin immunoprecipitation for each of the 5 histone modifications: H3K4me1 (enhancer), H3K4me3 (promoter), H3K27me3 (inactive chromatin), H3K27ac (active chromatin), H3K36me3 (transcriptional elongation). ChIP samples were run along with cDNA and gDNA from each cell-line, on high density Illumina genotyping arrays, covering ~4.4 million common SNPs. By assessing the signal ratio coming from the X and Y channel for each SNP we were able to calculate allelic imbalance values for heterozygous SNPs. (C) An example of AS-ChIP AI data for the paternally-expressed imprinted gene PLAGL1 in the fibroblast cell-line GM02456, shown on the UCSC genome browser track. Red upwards bars correspond to AI in favor of the paternal allele at the given SNP, with blue bars indicating AI in favor of the maternal allele.
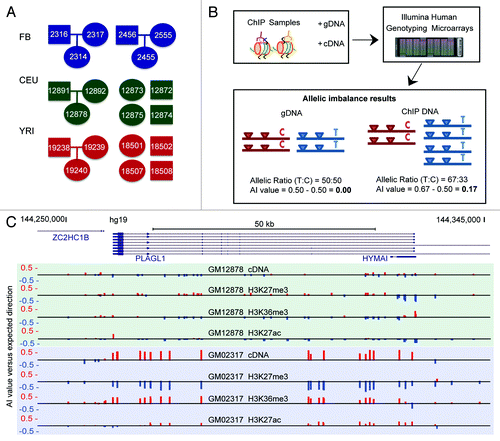
Table 1. Number of heterozygous SNPs with minimum AI of 0.05 by assay
The AS-ChIP approach is validated by independent assays and techniques
Reproducibility between genotyping arrays using different technologies was assessed for H3K4me1 for 3 individuals: GM12938, GM19239, GM19240 (Fig. S1). The Pearson correlation (r2) of unequal allelic ratios was high, ranging from 0.52–0.61 among technical replicates, P < 2.2E-16. Please note that all P values are not corrected for multiple-testing unless otherwise stated. To assess the biological reproducibility of our AS-ChIP experiments we performed 3 biological replicate experiments using cells from GM19238, GM19239 and GM19240, which were each grown in cell-culture twice, 3 months apart. Each time, cells were subjected to ChIP protocol using H3K4me1 antibody followed by allele-specific assessment bias using genotyping Illumina BeadChips. The correlation (r2) was again high, ranging from 0.73 to 0.81 among biological replicates, P < 2.2E-16 (Fig. S2).
Next, we assessed the sensitivity and specificity of the AS-ChIP method by generating high-depth ChIP-seq data for H3K4me1 and H3K4me3 using the same LCL individuals we had performed AS-ChIP on. The purpose of this analysis was to validate the AS-ChIP technique at sites for which we had high ChIP-seq signal, enabling us to determine the conditions for selection of allelic chromatin domains at high sensitivity and specificity (Fig. S3). On average, the sequence coverage was 150 M reads per experiment (~10 times higher than average ENCODE ChIP-seq data, see Table S1). We compared average phased AI across 10kb windows (minimum 2 SNPs) in our AS-ChIP data, with high-confidence allelic ChIP-seq sites. These sites were selected using the following criteria: ≥ 20 reads, binomial P < 0.001, with alternate allele greater than reference allele (to avoid spurious biases due to reference allele bias). These conservative criteria served the goal to evaluate AS-ChIP data against “golden standard” allelic-sites identified by ChIP-seq. In order to investigate specificity of AS-ChIP we also identified sites in our ChIP-seq data where we had high-confidence of equivalent bi-allelic binding. We specifically chose average AI cutoffs across measured windows with at least 2 sites) so as to achieve high-specificity in comparison with high confidence allelic ChIP-seq sites (>99%), while still maintaining reasonable sensitivity (>50%) (Fig. S3). A range of cutoffs were selected to select the optimal criteria: ≥20 reads, and binomial P > 0.99, for ChIP-seq and AI > 0.05 for H3K4me3 and >0.04 for H3K4me1 for at least 2 sites in the AS-ChIP. These high-specificity sites, termed allelic-chromatin domains, were subsequently used in downstream analyses. Descriptive statistics for the domains are shown in Table S3.
Characteristics of allelic-chromatin proximal to allelically expressed transcripts
We hypothesized that allelic-chromatin studies should help to infer the regulatory chromatin mechanisms underlying AE transcripts. We thus initially sought to characterize whether there is a general pattern to the allelic-chromatin landscape around these loci, with respect to allelic expression. First, we looked at a well-established imprinted gene to use as an example. We chose PLAGL1 (NM_0010809056), a mono-allelically expressed transcript in fibroblasts, where only the paternal allele of isoform 1 is expressed.Citation20 In LCLs, both the maternal and paternal alleles of this transcript are expressed.Citation20 This gene thus represents an ideal locus for characterizing the role of allelic-chromatin in regulating allelic expression as we can expect to observe mono-allelic imprinted expression and bi-allelic expression at the same locus. If allelic chromatin underlies allelic expression then we would expect to see allelic chromatin imbalance in FBs and not in LCLs. UCSC browser tracks displaying allelic imbalance values for cDNA indeed indicate a strong allelic imbalance for the fibroblast cell lines, and virtually no imbalance for the LCL cell-lines (). The individuals GM12878 (LCL) and GM02317 (FB) are shown as examples, as both cell lines are derived from Caucasian adult females. Chromatin marks H3K27ac and H3K36me3 are imbalanced strongly in the direction of the active (paternal) allele across the length of the transcript. While H3K36me3 is a known marker of transcriptional elongation, H3K27ac is a mark associated to active promoters and enhancers, we found at active gene loci. In concordance with previous non-allelic characterizations, H3K27me3 is highly imbalanced toward the inactive, in this case maternal, allele across the length of the transcript. Conversely, in LCLs we see little evidence of allelic-chromatin around the gene body of PLAGL1, consistent with the cDNA data showing that bi-allelic transcription is occurring in these cell-lines (). ENCODE ChIP-seq tracks for GM12878 and NHLF at the same loci are shown in Figure S4. In addition to PLAGL1, we examined 4 additional imprinted loci (L3MBTL, KCNQ1, MEG3, ZNF331), where we had sufficient SNPs to assess AE and AS-ChIP, in at least one fibroblast and at least one LCL individual. Notably, in these additional loci we observed allelic chromatin patterns, including H3K27ac marking transcriptional elongation, similar to that described in PLAGL1 (Figs. S5–8).
We next sought to characterize the allelic-chromatin landscape across all transcripts for which we have strong evidence of heritable AE mappable to common SNPs. We used an in-house generated list of 3513 transcripts shown to demonstrate differential AE in the 3 population cohorts from which we had obtained the cell-lines used in AS-ChIP assays: Yoruban HapMap LCLs (YRI) n = 55, Utah HapMap LCLs (CEU) n = 63 and Caucasian primary fibroblasts (FB) n = 70 (Adoue et al., Molecular Systems Biology, in review). Briefly the list of 3513 transcripts was obtained by assessing transcripts for AE in each of the populations, using Illumina genotyping arrays, as previously reportedCitation2 with a Fisher’s exact test, requiring that mapped loci be below the threshold of 1% FDR, used to improve mapping resolution for shared loci across populations. We then selected AS-ChIP data from samples heterozygous for cis-regulatory variants associated to differential AE of these genes. Plotting ChIP AI relative to the direction expected based on AE mapping across all 3513 transcripts, we observed global evidence of a predictable allelic-chromatin landscape around the TSS and gene body of AE genes (). For the most part, the allelic chromatin data behaved as expected based on previous non-allelic descriptions of the 5 histone marks with regards to transcription; however a number of unexpected findings were observed. Both H3K36me3 and H3K27ac modifications showed a strong AI in the direction of the most expressed allele along the gene body. The histone mark H3K27me3 showed a strong imbalance in the opposite direction along the gene body and to a lesser extent, upstream and downstream of the gene, essentially reflecting the opposite AI as H3K27ac. Promoter mark H3K4me3 showed significant (P = 1.59E-5, T-Test) AI positively correlating with allelic expression, in the first intron, and to a lesser degree across the gene body and upstream of the TSS. Remarkably, we observed a strong allelic signal around the TSS of AE genes for H3K27me3, H3K4me1 (and to a lesser non-significant extent, H3K36me3) where higher signal intensity coincided with the under-expressed allele.
Figure 2. Global characterization of allelic chromatin modifications around transcripts with differential allelic expression Allelic imbalance plotted for the 5 histone modifications across 3513 transcripts demonstrating allelic expression: (A) H3K4me1, (B) H3K4me3, (C) H3K27ac, (D) H3K36me3, (E) H3K27me3. Colored lines indicate average AI calculated using AI data for 25 bins of 10 kb, 2 kb upstream of TSS, 1st exon, 1st intron (1st 2 kb max), rest of transcript, last exon, 2 kb downstream of TES, and 25 bins of 10 kb further upstream of TSS. Grey plots show the –log (P value) for each bin, calculated via T-test, using as a baseline AI value the mean AI of the 2bins at the 250 kb upstream and downstream of TSS and TES respectively. Gray dotted lines indicate where P = 0.05 (corrected for multiple testing).
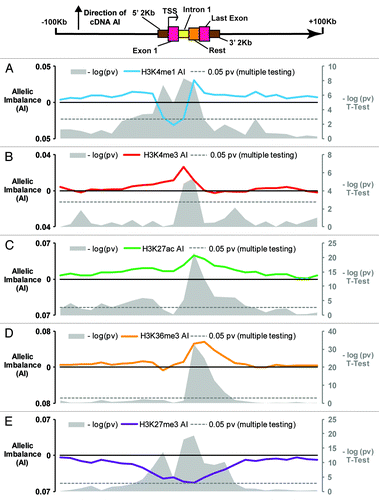
H3K4me1/cDNA anti-correlated signal at TSS reflect allelic regulatory activity
One of the more striking findings was the strong anti-correlation between H3K4me1 and H3K4me3 at the TSS of AE-transcripts (). While a slight depletion of H3K4me1 to baseline levels has previously been observed for highly active transcripts using ChIP-seq,Citation21 a strong allelic anti-correlation was highly unexpected, and no previous explorations of the functional role of H3K4me1 anti-correlation exist to our knowledge. H3K4me1 signal was anti-correlated with H3K4me3 for many AE-mapped cis-rSNP candidate sites. With a smaller list of sites we observed the same profiles in allelic ChIP-seq data. In order to validate true signals, we randomly selected 26 sites with significant allelic biases in ChIP-seq (binomial test P < 0.01) for validation via Sanger-sequencing (). Overlapping regions were amplified by PCR followed by purification and sequencing using the same samples used for ChIP-seq assays. Of the 26 sites, 15 had sufficient amplification and sequencing depth for allele-specific assessment of both H3K4me1 and H3K4me3. The expected allelic bias was successfully validated in 93% of randomly chosen sites (14/15) (Fig. S9).
Table 2. Significant allelic bias validation using Sanger sequencing
We observed a large H3K4me1/me3 anti-correlated signal with sharp enrichment in the region between 0 and 2kb downstream of the TSS (Fig. S10). This is in agreement with chromatin signals which show higher enrichment in the 2 kb immediately downstream of the TSSs rather than upstreamCitation22,Citation23 and supports an active allelic regulatory activity of H3K4me3 at the promoter of an overexpressed allele. In fact, this specific signal appears to be a sensitive indicator of allelically regulated promoters; when such signals were detected at +/− 2kb from the promoter, the average AE (toward H3K4me3 overrepresented allele) was 1.46-fold (95% CI 1.4 – 1.6), which was statistically higher (P = 1.5E-4) than the average AE of an allelically regulated transcript without such a pattern (1.25-fold, 95% CI 1.24 – 1.26). Furthermore, SNPs demonstrating specific H3K4me1/H3K4me3 anti-correlated signals were more likely to be fine-mapped cis-rSNPs, as determined based on our in-house AE data from the same population vs. that of any other heterozygous SNP located +/−2kb from TSS of same transcripts (CHITEST, P = 3.10E-4). We sought to investigate whether the H3K4me1/H3K4me3 signal was significantly enriched for cis-rSNPs near the TSS of allelically expressed genes. In order to better understand the allelic activity occurring proximal to the TSS, we generated AS-ChIP profiles for histone marks in a +/−5kb window from the TSS with a resolution of 100bp. Depletion of H3 nucleosomes and H3K4me3 within 100 bp upstream of the TSS has been shown, suggesting that nucleosomes are depleted near the TSS to facilitate binding of transcriptional machinery including RNAP II and associated transcription factors.Citation24 However, the very specific allelic signals of the different histone marks suggest more complex regulation, particularly for H3K4me1, which showed a strong and extended signal +/−1kb on both sides of TSS. The fact that a substantial proportion of H3K4me1/me3 anti-correlated signal localizes to the TSS of AE-loci suggests regulatory activity for such profiles.
Shared AS-ChIP regions between Individuals
To assess the heritability, cell-type specificity, and population specific effects of allelic-chromatin, we performed a test of enrichment for pairwise-overlaps between individuals for the AS-ChIP domains for H3K4me1 and H3K4me3. Enrichment was tested by calculating the ratio of AS-ChIP domains which overlapped between individuals divided by the number of control windows of equal size, randomly shuffled (using BEDtools)Citation25 in the genome which also overlapped (see methods). shows a heatmap of these enrichments for both H3K4me1 () and H3K4me3 (). To assess whether these enrichments were stronger in directly related (parent-child) relationships vs. unrelated individuals, we performed a one-tailed student’s T-test comparing parent-child enrichment values and unrelated enrichment values from the heat maps in between individuals from the same cell-type and population. We found for both H3K4me1 and H3K4me3 significantly higher enrichment values overall in directly related individuals (H3K4me1 P < 0.01, H3K4me3 P < 0.001) (). In addition, we see a highly significant enrichment in unrelated individuals from the same cell-type and population vs. unrelated individuals from the same-cell-type but different populations (YRI vs. CEU) (H3K4me1- P < 10E-5, H3K4me3 P < 10E-5). This suggests that in addition to directly heritable/ transmission-type effects on allelic-chromatin, population-level variation affecting allelic-chromatin also exists. Moreover, we see the strongest enrichments (H3K4me1- P < 10E-5, H3K4me3 P < 10E-5) in unrelated individuals from the same cell-type vs. unrelated individuals from different cell-types, indicating that cell-type specific effects on allelic-chromatin are likely greater than population-dependent/ heritable effects (). Given that gene density varies in the genome, the individual pair-wise enrichments may be overestimated; however, this does not affect the comparison of pair-wise enrichments between groups. Finally, we detected little enrichment of allelic-chromatin domains with distance to TSS compared with random SNPs (Fig. S11). Ultimately these results suggest that allelic chromatin is in part controlled by genetic and developmental factors, although we cannot rule out the contribution of environmental effects.
Figure 3. H3K4me1 and H3K4me3 allelic chromatin domains pairwise overlap demonstrates cell-type specificity, population-specificity and heritability (A–B) Heat maps of enrichment of pair-wise overlap between individuals for (A) H3K4me1 and (B) H3K4me3 allelic-chromatin domains, vs. shuffled control regions of equal size. Along the top and side axes are each of the AS-ChIP individuals, labeled by population and whether they are in a trio by their relationship: daughter (D), mother (M), father (F), or unrelated (U). (C-D) Bar charts for H3K4me1 (C) and H3K4me3 (D), showing mean enrichment for pair-wise overlap is significantly higher within cell-types among unrelated individuals from same population (purple) vs. across cell-types. Also pair-wise overlap is significantly higher among unrelated individuals within the same population (purple), vs. across populations, same cell-type, unrelated individuals (green). Finally pair-wise overlap is significantly higher among related individuals from same cell-type and population (purple), vs. unrelated individuals from same cell-type and population (green). * = P < 0.01, ** = P < 0.001, *** = P < 10E-5.
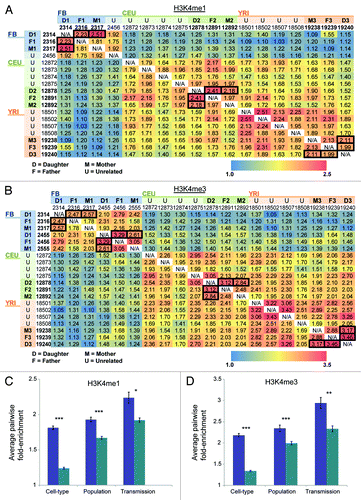
dsQTL–positive DNase peaks preferentially overlap allelic chromatin domains
Given the high correlation of histone modifications with DNase I-accessibility, we sought to identify whether allelic-chromatin domains preferentially overlap DHSs. Given the recent mapping of a large subset of DHSs to dsQTLs, we sought also to investigate this enrichment specifically in DHSs under genetic control, i.e., those domains which would be expected to show allelic imbalance for an individual heterozygous for the corresponding dsQTL. We obtained lists of called DHS peaks generated by ENCODE (http://ebi.edu.au/ftp/software/software/ensembl/encode/integration_data_jan2011/) for 17 LCL samples that underwent DNase-sequencing.Citation26 For all DHSs present in at least 9 individuals, and with a P value less than 1E-5, intersection with dsQTL associated DHSs was performed using BEDtools.Citation25 dsQTLs were obtained from Degner et al.Citation13 DHSs were termed dsQTL-positive, when Degner et al., had identified an overlapping DHS under genetic control from a SNP. All DHSs which did not overlap a DHS reported by Degner et al. as being associated to a SNP, were termed dsQTL-negative. A total of 3358 combined DHSs were found to be dsQTL positive and 23 024 were dsQTL negative. Overlap with H3K4me1 and H3K4me3 allelic-chromatin domains was performed for both DHS subsets. Enrichments were performed using the Monte-Carlo genome-wide enrichment procedure described in the methods. Enrichment for dsQTL-negative DHSs in both LCL populations (CEU, YRI) was significant when overlapped with H3K4me3 allelic chromatin regions (P < 10E-5). However, greater enrichments were found for dsQTL positive DHSs for H3K4me1 and H3K4me3 in both LCL populations (CEU, YRI), with the greatest levels of enrichment occurring in allelic chromatin domains generated from YRI individuals (). The strong enrichment of allelic chromatin domains in dsQTL-positive DHSs vs. dsQTL-negative DHSs suggests a shared regulatory mechanism for these marks. These enrichments were not significant in the FB population (P > 0.05) and were most significant in the YRI population (P < 10E-5). Given that the DHS/dsQTL data was generated in YRI individuals, and CEU and YRI are both LCL samples, it would appear that differences in enrichment are affected by population, but are primarily cell-type dependent. This is in alignment with previous studies of cell-type and population-dependent cis-regulatory effects.Citation27-Citation29
Figure 4. dsQTL–positive DNase peaks preferentially overlap allelic chromatin domains Enrichment of DHS peaks in H3K4me1 and H3K4me3 allelic-chromatin domains from each of the 3 populations (CEU, FB and YRI). Dark blue indicates dsQTL positive DHS peak overlap, and light blue indicates dsQTL negative DHS peaks overlap. n.s = P > 0.05, ** = P < 0.001, *** = P < 10E-5.
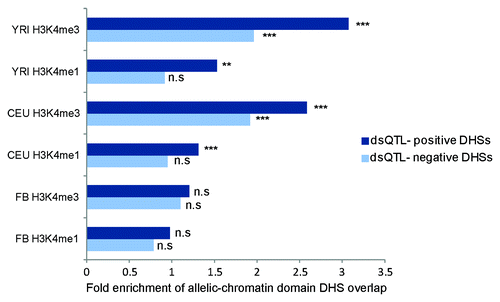
Enrichment of disease-associated variants in allelic-chromatin domains
The GWAS catalogCitation30 includes 11 760 complex trait-genetic variant associations. Given our initial hypothesis that regions of allelic chromatin would be likely candidates for harboring genetic variants associated to complex disease, we sought to test this hypothesis by intersecting our allelic-chromatin domains with SNPs reported by the GWAS catalog as having evidence of association with complex traits and/or diseases (P < 1E-6). For each of the three populations for which we performed AS-ChIP, we intersected allelic-chromatin regions (H3K4me1 and H3K4me3 separately) that appeared in at least two individuals with SNPs reported in the GWAS catalog. Compared with 10 000 randomly shuffled control regions of equal size, we observed significant enrichment in our allelic-chromatin regions for GWAS SNPs in all 3 populations for both marks (P < 0.01 - YRI-H3K4me3, FB H3K4me1; P < 10E-5 – YRI H3K4me1, CEU H3K4me1, CEU H3K4me1, FB H3K4me3) (). Moreover, we see the greatest enrichments, over 2.5-fold, for autoimmune-related GWAS SNPs lying in allelic-chromatin regions mapped in LCLs. Meanwhile, there was no enrichment for these SNPs in the FB-derived allelic-chromatin domains (P > 0.05), indicating that this effect is specific to LCLs and likely other immune cell-types. The FB meanwhile showed no disease-type specific GWAS enrichment in our analyses. This could be due to a dearth of GWAS data for fibroblast-relevant diseases, or to the way in which diseases have been categorized. Disease categorization was performed using the same categories previously used to assess GWAS hit enrichment in DHS regions.Citation1
Figure 5. Enrichment of disease-associated variants in allelic chromatin domains. Enrichment of GWAS lead SNPs from GWAS catalog in allelic-chromatin domains, vs. control regions of equal size. Green, red and blue bars indicate YRI, CEU and FB and populations respectively with darker bars being H3K4me3 domains and pale bars being H3K4me1 domains. n.s = P > 0.05, * = P < 0.01, ** = P < 0.001, *** = P < 10E-5.
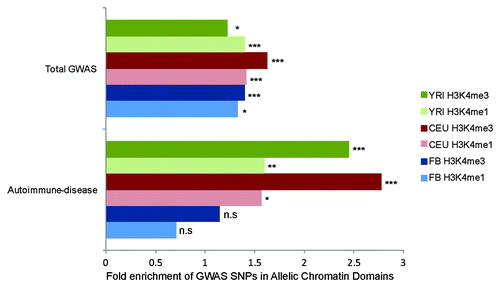
Allelic-chromatin suggests allelic transcription in unannotated regions
As we investigated the AS-ChIP data more closely, we noticed a large amount of allelic chromatin signal suggestive of transcription (e.g., H3K36me3 anti-correlated with H3K27me3) in intergenic regions - those not annotated in GENCODE/ENCODE V17 as overlapping regions with any known coding/non-coding transcripts. An example is shown in , in which we see strong anti-correlated H3K36me3/H3K27me3 allelic binding across a region of several hundred Kb in length. Notably this region is entirely unannotated in the latest GENCODE release; however, a few sporadic ESTs are found in the area, revealing the possibility of weak transcription. Remarkably, despite the lack of nearby genes, 3 GWAS hits lie in this region (rs6894216, rs7731657, and rs4836519), which are associated with d-amphetamine response, asthma and inflammatory bowel disease respectively, suggesting that this region has an important biological function.Citation31-Citation33 To systematically investigate allelic chromatin globally in unannotated regions, we created allelic-chromatin domains using H3K27me3 and H3K36me3 AS-ChIP data, as we had previously seen H3K27me3 and H3K36me3 anti-correlation as a strong marker of allelic expression. Briefly, we defined a domain as a genomic region with a minimum of 3 consecutive AS-ChIP SNPs for a given mark, in the same allelic direction, with a minimum AI of 0.10, up to a maximum of 100Kb. We combined the allelic chromatin domains of H3K36me3 and H3K27me3, by looking for overlapping H3K36me3 and H3K27me3 domains. Domains where AI was in the same direction were defined as positively correlated, and those in opposite allelic directions as anti-correlated (Tables S4 and S5).
Figure 6. Allelic-chromatin suggests allelic transcription in an unannotated region overlapping GWAS SNPs Example of H3K27me3 and H3K36me3 allelic-chromatin suggestive of transcription in intergenic region of genome on chromosome 5 in UCSC genome browser for GM02316. Red and blue bars correspond to phased AI in opposite directions. Tracks above show all human ESTs in the region, a lack of any annotation in GENCODE V17 Comprehensive annotation and 3 GWAS SNPs, in the GWAS catalog. Below are ChIP-seq tracks from ENCODE showing low signal for H3K27me3 and H3K36me3 in NHLF (Normal Human Lung Fibroblast).
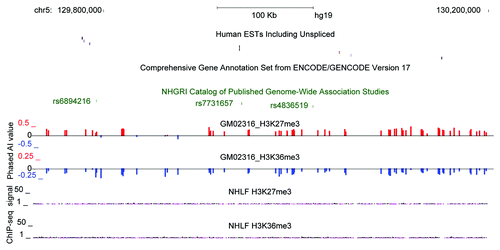
While the vast majority of H3K36me3 and H3K27me3 AS-ChIP data points behaved as expected, (e.g., we see an anti-correlation of H3K36me3 and H3K27me3), with H3K36me3 positively correlated with expression AI and H3K27me3 anti-correlated with expression AI, there was a subset of cases where an unexpected relationship between histone marks was observed (Tables S2 and S3).
For a subset of regions (385 for LCLs, 1483 for FBs), we observed a positive correlation between H3K27me3 and H3K36me3 AI regions, counter to expectation (Tables S2 and S3). While the majority of allelic chromatin regions are found in association with known transcriptional units (mRNA transcripts, lincRNA transcripts etc.), a subset is located entirely in unannotated intergenic regions (49 in LCLs and 234 in FBs). These sites do not overlap any known coding transcripts or lincRNAs based on GENCODE V17, indicating a substantial number of potentially functional transcripts may still be unannotated due to weak expression levels. Two examples are illustrated (Fig. S12).
Discussion
We undertook a genome-wide study of allelic chromatin in order to draw further insights into the cis-regulatory mechanisms governing the predominance of the genetic risk associated to complex disease. Using high density-genotyping arrays capable of assaying common genetic variation down to below 0.5 percent minor allele frequency, we obtained high resolution, genome-wide allelic ChIP data. Five histone marks were selected for AS-ChIP, giving us a wide range of regulatory states to explore. The number of SNPs that were analyzed for histone-modification allele-specificity is a vast improvement on previous ChIP-seq based AS approaches. Using this large data set of AS-binding sites, we were able to combine nearby SNPs into domains of high specificity from which we observed heritability of allelic chromatin, as well as cell-type and population dependent effects. Notably, among the 3 variables studied, cell-type appeared to have the greatest effect on allele-specific chromatin.
We have previously shown that CEU lymphoblastoid lines, in particular, are not fully polyclonal,Citation2,Citation34 which in principle could render random allelic eventsCitation35 detectable in our assay. Furthermore, our earlier analyses in these cells suggested that a small fraction of rare autosomal monoallelic expression could be attributable to aberrant methylation, though most monoallelic expression was shown to be due to heritable sequence variation.Citation20 In line with this we now show that allelic chromatin states are overlapping heritably controlled DHSs, enriched among GWAS SNPs in cell type specific manner and that heritability of AS-chromatin is similar across polyclonal (fibroblast) and oligoclonal (CEU lymphoblasts) cell lines. Consequently, random chromatin silencing appears to have minor impact in AS-ChIP even in cultured cells.
Previously, ChIP-seq studies examining the enrichment of key histone methylation marks around the TSSs of transcripts identified consistent patterns of histone methylation at the promoter region of active genes.Citation21,Citation36 Our assessment of allelic-chromatin around the TSS and gene bodies of allelically expressed transcripts, allows us to sensitively characterize the histone modification patterns associated to expression, free from trans-acting effects.
Our AS-ChIP data shows that allelically expressed genes harness allelic enrichments of histone posttranslational modifications commonly thought to have punctate patterns of enrichments by ChIP-seq. (e.g., H3K27ac). This is due to the observation of relative rather than absolute signal in the highly amplified chromatin inherent to the BeadChip sample processing. In comparison, using ChIP-seq. weak positive allele-specific correlations have earlier been observed between allelically transcribed genes and H3K27ac.Citation7 Not previously characterized, but uncovered via our AS-ChIP analyses here, is allele-specific H3K4me1 binding at the TSS of allelically expressed genes in the opposite direction to allelic expression. Functional validation of H3K4me1 anti-correlated binding at the TSS suggests that this is a powerful mark for predicting highly, allelically imbalanced transcript expression. These data suggest that some properties of known histone modifications will be discovered in following years by improving read depth and technologies.
We find that our allelic-chromatin domains preferentially overlap DHSs under genetic control by dsQTLs, strongly suggesting that our AS-ChIP allows the identification of domains under genetic control. This is in agreement with a shared genetic mechanism controlling both chromatin accessibility and histone modifications. We also identify an overall enrichment of GWAS SNPs in allelic chromatin domains from all 3 populations (FB, CEU, and YRI), with an autoimmune disease specific enrichment in LCL (YRI/CEU) derived allelic chromatin domains. Previous studies have used allele-specific formaldehyde-assisted isolation of regulatory elements (FAIRE) and allelic ChIP-seq to investigate allelic chromatin.Citation37-Citation39 Allele-specific FAIRE in LCLs was notable in the identification of an allele-specific regulatory polymorphism coinciding with a GWAS SNP for HDL-C. Our allelic-chromatin data likewise shows enrichment with GWAS SNPs, and we agree with their conclusion that allelic-chromatin studies have the potential to aid in the identification of causal SNPs associated with disease by GWAS.Citation39
Recently, researchers using high-depth RNA-seq experiments in 15 diverse human cell-lines, found evidence that up to 75% of the human genome is capable of being expressed.Citation40 The functional significance of the vast majority of these previously unannotated transcripts, most of which are expressed at extremely low levels, is not yet known.Citation40 Our identification of allelic chromatin regions indicative of allelic transcription, often coinciding with allelic cDNA regions, in regions not previously annotated by ENCODE/GENCODE, indicates that a subset of these low-level, previously unannotated transcripts are being controlled by sequence-specific cis-regulatory mechanisms. Moreover, our discovery of multiple GWAS SNPs in one of these unannotated allelic-chromatin domains points toward functionality of a subset of these regions. Previously unannotated transcripts, which are regulated by cis-regulatory processes, which we identify as being associated with allelically imbalanced chromatin states, may constitute ideal targets for future functional studies of transcripts expressed at low levels.
Our demonstration of allelic assessment of histone modifications opens up the possibility of performing allelic-ChIP mapping studies using a larger number of individuals, to associate variants with the allelic imbalance of chromatin marks. Given the large increase in power of allelic-analyses previously demonstrated using AE-mapping vs. eQTL studies,Citation3 allelic-ChIP mapping presents a potentially cost-effective means of identifying common genetic variants associated to regulation of specific chromatin states. Combining this information with cis-rSNP data and GWAS data, may be a powerful means to improve our understanding of the specific mechanisms underlying common genetic polymorphisms associated to complex disease.
This study’s findings indicate the utility of allelic tools to investigate genome-wide cis-regulatory mechanisms at high-density. As next-generation sequencing technologies continue to progress, allelic studies examining a range of epigenomic features should be increasingly feasible, and as demonstrated by us, may reveal previously unappreciated phenomena associated with genetic and epigenetic control of gene expression.
Materials and Methods
Cell-culture
Six fibroblast cell-lines (GM02314, GM02316, GM02317, GM02455, GM02456, GM02555), and 14 HapMap lymphoblastoid cell-lines (GM19238, GM19239, GM19240, GM18507, GM12891, GM12892, GM12878, GM12873, GM12872, GM12875, GM12874, GM1801, GM18502, GM18508) were used in the AS-ChIP experiments. All cell-lines were obtained from Coriell. Primary fibroblast cells were immortalized as described previously,Citation41 in order to grow cells to the quantity needed for generating large quantities of histone ChIP DNA for direct assessment on genotyping arrays. Following immortalization, cells were subsequently grown in medium containing a-MEM (SigmaAldrich) supplemented with 2 mmol/l L-glutamine, 100 U/ml penicillin, 100 mg/ml streptomycin, and 10% fetal bovine serum (SigmaAldrich) at 37 °C with 5% CO2. At 70 to 80% confluence, the cells were harvested and stored at -70 °C until RNA and DNA extraction. LCLs were grown to log phase (106 cells/ml maximum density) in 40 ml of media. gDNA and RNA were extracted from cell lysates and a dscDNA synthesis protocol was applied as previously described.Citation41
Antibodies
The following antibodies were used for ChIP: H3K4me1 (Diagenode; #pAb-037–050), H3K4me1 (Abcam; H3K4me3 (Diagenode; #pAb-003–050), H3K27ac (Abcam; #ab4729), H3K27me3 (Millipore; #07–449), H3K36me3 (Abcam, #ab9050).
Chromatin immunoprecipitation
After harvesting, cells were immediately cross-linked with 1% formaldehyde at room temperature for 10 min before quenching with glycine for 5 min (125 mM glycine). The cells were then washed twice with ice-cold PBS. Cells were collected after each wash by centrifugation at 2000 g for 5 min. Cell pellets were flash frozen and stored at −80 °C. Frozen pellets were thawed and cells were lysed in Farnham lysis buffer (5 mM PIPES pH8.0, 85 mM KCl, 0.5% NP-40, and protease inhibitors) for 10 min on ice. After centrifugation and a wash with 1 ml of RIPA buffer (50 mM TRIS-HCl pH8, 150 mM NaCl, 1% NP-40, 0.5% sodium deoxycholate, 0.1% SDS, and protease inhibitors), lysates were diluted with 500 μl of RIPA buffer before sonication. Cells were sonicated in non-stick tubes under conditions optimized to yield soluble chromatin fragments in a size range of 100 to 250 base pairs. Chromatin from 40 million cells was sonicated for 10 min using a Branson 250 sonicator at 20% power amplitude (pulses: 10 s on and 30 s off). Lysate was cleared by centrifuging at 12,000 g for 10 min at 4 °C to eliminate cellular debris. Chromatin was then flash frozen and stored at –80 °C.
Before each immunoprecipitation, chromatin was pre-cleared with 50 μl of prewashed Protein A-magnetic beads (Invitrogen; 100–02D) to avoid non-specific binding. Immunoprecipitation was performed for 12 h by rotation at 4 °C in 500 μl of chromatin/RIPA buffer supplemented with protease inhibitor cocktails (Roche; 04 693 159 001) and PMSF. We used 10 to 100 million cells and 2 to 20 μg of antibody for each assay: After overnight incubation, samples were rotated with 100 μl of prewashed Protein A-magnetic beads at 4 °C for 1 h. The beads were then collected by brief centrifugation at 2000 g following by the use of a magnetic rack. Beads were washed five times with 1 ml of LiCl wash buffer (100mM Tris pH7.5, 500mM LiCl, 1% NP-40, 1% sodium deoxycholate) by re-suspending the beads and keeping them on ice for 10 min. Bound chromatin was then eluted from the beads using 200 μl of Elution buffer (50 mM TRIS-HCl, pH 8.0, 10 mM EDTA, 1.0% SDS) by incubation at 65 °C for 1 h while vortexing every 15 min, followed by centrifugation at 14 000 g at room temperature for 3 min. The eluted chromatin and the “input” samples were then incubated at 65 °C overnight after adding 0.2M of NaCl to remove cross-linking. Samples were subsequently treated with RNase A at 37 °C for 30 min and then proteinase K at 55 °C for 1 h. Immunoprecipitated DNA was then purified using QIAquick PCR Purification Kit (QIAGEN; 28104) and eluted in 30 μl of elution buffer.
Validation
Enrichments of known binding regions for each histone modification were validated using real-time PCR experiments for each antibody, against input DNA. Six primer pairs were used to assess ChIP enrichment, designed to genomic sites known to bind to H3K4me1, H3K4me3, H3K27ac, H3K27me3, H3K36me3 or none of them, see Table S6.
Library preparation for ChIP-seq assays were carry out using Paired-End DNA Sample Prep Kit V1 (Illumina; PE-102–1001) and sequenced using the Illumina Genome Analyzer II (2x76bp) or HiSeq Sequencing System (2x100bp).
Samples prepared for AS-ChIP assays were then treated like double-stranded cDNA samples and assessed on Illumina BeadChips. The panel we used consisted of 7 individuals for ChIP-seq: 4 YRI (GM19238, GM19239, GM19240, and GM18507) and 3 CEU (GM12891, GM12892, and GM12878); and 14 individuals for AS-ChIP assays: 7 YRI (GM19238, GM19239, GM19240, GM18507, GM18508, GM18501, and GM18502) and 7 CEU (GM12891, GM12892, GM12878, GM12872, GM12873, GM12874, and GM12875). All are HapMap samples.
Random sites with significant allelic biases (P binomial test < 0.01) were selected for validation with sanger sequencing. Overlapping regions were amplified by PCR followed by purification and sequencing using the same samples used for ChIP-seq assays.
Genotyping and AI analysis
AE and differential chromatin binding biases analysis was performed on IlluminaHuman1M-Duo, HumanOmni2.5-Quad and HumanOmni2.5-Quad-SBeadChips (LCLs) and Illumina Human Omni5- BeadChips (FB) (see Table S1 for complete details). Approximately 200 ng of genomic DNA and 50–300 ng double-stranded cDNA or 6 ng to 1 ug ChIP sample was used for the parallel genotyping, AE and allelic ChIP analysis on Illumina Human BeadChip was performed according to the manufacturer’s instructions (Illumina Inc.). Genotypes in genomic DNA were extracted using BeadStudio.
The parallel assessment of genomic DNA and cDNA/ChIP DNA heterozygote ratios was performed essentially as described earlier for allelic expression analysis.Citation2,Citation41 We utilized the Xraw and Yraw signal intensities; however, since the variances in the two channels are not same (i.e., it is a function of total intensity from both channels) we corrected this variation through normalization to allow comparison between gDNA and cDNA allele ratios. In this study, we only normalized β ratio (Xraw/[Xraw+Yraw]) from heterozygous SNPs with total intensity (Xraw+Yraw) higher than the threshold value of 1000. The normalization process can be briefly summarized in the following steps: (1) The β ratio was calculated along with the total intensity in log10 scale for all heterozygous SNPs. (2) All data points with greater than 1000 in total intensity were divided into 50 intensity bins. (3) A fitted curve from the median β ratio in each bin was computed using a polynomial regression model (quadratic regression) y = b1x+b2x2+a where y is expected β ratio from the curve and x is log10 scaled total intensity. (4) From the fitted curve, the expected β ratio based on total intensity was calculated. (5) The final normalized β ratio equals (βobs−βexpected+0.5). Following normalization, all median β ratio values in all intensity bins should be close, if not equal, to 0.5. Plots of signal intensity are shown in Figure S13.
GWAS-enrichment-analysis
GWAS catalog was obtained from https://www.genome.gov/admin/gwascatalog.txt on December 3rd, 2013.Citation30 Autoimmune GWAS SNPs were selected based on Maurano et al. GWAS disease categorizations.Citation1 Duplicate SNPs were then removed. We avoided the use of SNPs in linkage disequilibrium (LD) with GWAS SNPs as this would over-count for enrichments at sites with high LD. Enrichment was performed according to below genome-wide enrichment analysis.
ChIP-seq initial-processing and assessment of allelic imbalance
Sequence data was generated by the Illumina HiSeq 2000. The runs were processed using Illumina’s CASAVA 1.8 software. The resulting phred33 encode 100 b.p. paired-end fastq filesRaw reads were trimmed for quality (phred33 ≥ 30) and length (n ≥ 32) using Trimmomatic v. 0.22. Illumina adaptor sequences were also clipped off using the palindrome mode from this software. The filtered reads were aligned to the hg19 reference genome using BWA v. 0.61.
For all 1000G SNPs with a minimum of 20 overlapping reads, allelic imbalance was assessed using a binomial test, comparing reference and alternate alleles. To ensure observed allelic imbalance was not due to reference bias, only cases where the alternate allele possessed greater read counts vs. the reference allele, were included in the analysis.
Genome-wide enrichment analysis
Enrichment analysis was performed using 10 000 Monte Carlo (MC) simulations, where regions were shuffled within the same chromosome arm (centromere was excluded from this analysis). The mean number of bases overlapping in the 10 000 MC simulations was used as a base-line to calculate enrichment. P values were calculated by dividing the number of MC simulations which had at least as much overlap as the observed overlap by the total 10 000 simulations performed. MC simulations were performed using the Genomic Hyperbrowser track analysis tools.Citation42
Data availability
The allele-specific ChIP data discussed in this publication have been deposited in NCBI's Gene Expression OmnibusCitation43 and are accessible through GEO Series accession number GSE51272. (http://www.ncbi.nlm.nih.gov/geo/query/acc.cgi?acc=GSE51272). ChIP-seq data has also been deposited in GEO, and is accessible though GEO Series accession number GSE53837 (http://www.ncbi.nlm.nih.gov/geo/query/acc.cgi?acc=GSE53837).
Abbreviations: | ||
1000G | = | 1000 Genomes Project |
AE | = | allelic expression |
AI | = | allelic imbalance |
AS | = | allele-specific, ChIP-seq, ChIP-sequencing |
ChIP | = | chromatin immunoprecipitation |
cis-rSNPs | = | cis-regulatory SNPs |
DHS | = | DNase I hypersensitive site |
DNase-seq | = | DNase I sequencing |
dsQTL | = | DNase I-sensitive quantitative trait locus |
EDTA | = | ethylenediaminetetraacetic acid |
ENCODE | = | Encylopedia of DNA elements |
eQTL | = | expression quantitative trait loci |
EST | = | expression sequence tag |
FB | = | fibroblasts |
FAIRE | = | formaldehyde assisted isolation of regulatory elements |
GWAS | = | Genome Wide Association Study |
GEO | = | Gene Expression Omnibus |
CEU | = | HapMap Caucasian population |
YRI | = | HapMap Yoruban population |
LD | = | linkage disequilibrium |
lincRNAs | = | long interegenic non-coding RNAs |
LCL | = | Lymphoblastoid Cell Line |
MNC | = | monocytes |
MC | = | Monte Carlo |
PMSF | = | phenylmethylsulfonyl fluoride |
PCR | = | polymerase chain reaction |
RNAP II | = | RNA polymerase II |
RNA-seq | = | RNA-sequencing |
SNP | = | Single Nucleotide Polymorphism |
TES | = | Transcription End Site |
TSS | = | Transcription Start Site |
UTR | = | untranslated region |
Additional material
Download Zip (3.2 MB)Disclosure of Potential Conflicts of Interest
No potential conflicts of interest were disclosed.
References
- Maurano MT, Humbert R, Rynes E, Thurman RE, Haugen E, Wang H, Reynolds AP, Sandstrom R, Qu H, Brody J, et al. Systematic localization of common disease-associated variation in regulatory DNA. Science 2012; 337:1190 - 5; http://dx.doi.org/10.1126/science.1222794; PMID: 22955828
- Ge B, Pokholok DK, Kwan T, Grundberg E, Morcos L, Verlaan DJ, Le J, Koka V, Lam KCL, Gagné V, et al. Global patterns of cis variation in human cells revealed by high-density allelic expression analysis. Nat Genet 2009; 41:1216 - 22; http://dx.doi.org/10.1038/ng.473; PMID: 19838192
- Almlof JC, Lundmark P, Lundmark A, Ge B, Maouche S, Goring HH, Liljedahl U, Enstrom C, Brocheton J, Proust C, et al. Powerful identification of cis-regulatory SNPs in human primary monocytes using allele-specific gene.
- Pastinen T. Genome-wide allele-specific analysis: insights into regulatory variation. Nat Rev Genet 2010; 11:533 - 8; http://dx.doi.org/10.1038/nrg2815; PMID: 20567245
- Ernst J, Kheradpour P, Mikkelsen TS, Shoresh N, Ward LD, Epstein CB, Zhang X, Wang L, Issner R, Coyne M, et al. Mapping and analysis of chromatin state dynamics in nine human cell types. Nature 2011; 473:43 - 9; http://dx.doi.org/10.1038/nature09906; PMID: 21441907
- Bernstein BE, Stamatoyannopoulos JA, Costello JF, Ren B, Milosavljevic A, Meissner A, Kellis M, Marra MA, Beaudet AL, Ecker JR, et al, The NIH Roadmap Epigenomics Mapping Consortium. The NIH Roadmap Epigenomics Mapping Consortium. Nat Biotechnol 2010; 28:1045 - 8; http://dx.doi.org/10.1038/nbt1010-1045; PMID: 20944595
- Bernstein BE, Birney E, Dunham I, Green ED, Gunter C, Snyder M, ENCODE Project Consortium. An integrated encyclopedia of DNA elements in the human genome. Nature 2012; 489:57 - 74; http://dx.doi.org/10.1038/nature11247; PMID: 22955616
- Schaub MA, Boyle AP, Kundaje A, Batzoglou S, Snyder M. Linking disease associations with regulatory information in the human genome. Genome Res 2012; 22:1748 - 59; http://dx.doi.org/10.1101/gr.136127.111; PMID: 22955986
- Li MJ, Wang LY, Xia Z, Sham PC, Wang J. GWAS3D: Detecting human regulatory variants by integrative analysis of genome-wide associations, chromosome interactions and histone modifications. Nucleic Acids Res 2013; 41:W150-8; PMID: 23723249
- Mercer TR, Edwards SL, Clark MB, Neph SJ, Wang H, Stergachis AB, John S, Sandstrom R, Li G, Sandhu KS, et al. DNase I-hypersensitive exons colocalize with promoters and distal regulatory elements. Nat Genet 2013; 45:852 - 9; http://dx.doi.org/10.1038/ng.2677; PMID: 23793028
- Noll M. Internal structure of the chromatin subunit. Nucleic Acids Res 1974; 1:1573 - 8; http://dx.doi.org/10.1093/nar/1.11.1573; PMID: 10793712
- Song L, Crawford GE. DNase-seq: a high-resolution technique for mapping active gene regulatory elements across the genome from mammalian cells. Cold Spring Harb Protoc 2010; 2010:t5384; http://dx.doi.org/10.1101/pdb.prot5384; PMID: 20150147
- Degner JF, Pai AA, Pique-Regi R, Veyrieras J-B, Gaffney DJ, Pickrell JK, De Leon S, Michelini K, Lewellen N, Crawford GE, et al. DNase I sensitivity QTLs are a major determinant of human expression variation. Nature 2012; 482:390 - 4; http://dx.doi.org/10.1038/nature10808; PMID: 22307276
- Maynard ND, Chen J, Stuart RK, Fan J-B, Ren B. Genome-wide mapping of allele-specific protein-DNA interactions in human cells. Nat Methods 2008; 5:307 - 9; PMID: 18345007
- Rozowsky J, Abyzov A, Wang J, Alves P, Raha D, Harmanci A, Leng J, Bjornson R, Kong Y, Kitabayashi N, et al. AlleleSeq: analysis of allele-specific expression and binding in a network framework. Mol Syst Biol 2011; 7:522; http://dx.doi.org/10.1038/msb.2011.54; PMID: 21811232
- Mikkelsen TS, Ku M, Jaffe DB, Issac B, Lieberman E, Giannoukos G, Alvarez P, Brockman W, Kim TK, Koche RP, et al. Genome-wide maps of chromatin state in pluripotent and lineage-committed cells. Nature 2007; 448:553 - 60; http://dx.doi.org/10.1038/nature06008; PMID: 17603471
- Bonn S, Zinzen RP, Girardot C, Gustafson EH, Perez-Gonzalez A, Delhomme N, Ghavi-Helm Y, Wilczyński B, Riddell A, Furlong EEM. Tissue-specific analysis of chromatin state identifies temporal signatures of enhancer activity during embryonic development. Nat Genet 2012; 44:148 - 56; http://dx.doi.org/10.1038/ng.1064; PMID: 22231485
- Abecasis GR, Altshuler D, Auton A, Brooks LD, Durbin RM, Gibbs RA, Hurles ME, McVean GA, 1000 Genomes Project Consortium. A map of human genome variation from population-scale sequencing. Nature 2010; 467:1061 - 73; http://dx.doi.org/10.1038/nature09534; PMID: 20981092
- Abecasis GR, Auton A, Brooks LD, DePristo MA, Durbin RM, Handsaker RE, Kang HM, Marth GT, McVean GA, 1000 Genomes Project Consortium. An integrated map of genetic variation from 1,092 human genomes. Nature 2012; 491:56 - 65; http://dx.doi.org/10.1038/nature11632; PMID: 23128226
- Morcos L, Ge B, Koka V, Lam KC, Pokholok DK, Gunderson KL, Montpetit A, Verlaan DJ, Pastinen T. Genome-wide assessment of imprinted expression in human cells. Genome Biol 2011; 12:R25; http://dx.doi.org/10.1186/gb-2011-12-3-r25; PMID: 21418647
- Barski A, Cuddapah S, Cui K, Roh T-Y, Schones DE, Wang Z, Wei G, Chepelev I, Zhao K. High-resolution profiling of histone methylations in the human genome. Cell 2007; 129:823 - 37; http://dx.doi.org/10.1016/j.cell.2007.05.009; PMID: 17512414
- Cheng C, Yan K-K, Yip KY, Rozowsky J, Alexander R, Shou C, Gerstein M. A statistical framework for modeling gene expression using chromatin features and application to modENCODE datasets. Genome Biol 2011; 12:R15; http://dx.doi.org/10.1186/gb-2011-12-2-r15; PMID: 21324173
- Ha M, Ng DW, Li W-H, Chen ZJ. Coordinated histone modifications are associated with gene expression variation within and between species. Genome Res 2011; 21:590 - 8; http://dx.doi.org/10.1101/gr.116467.110; PMID: 21324879
- Hassan AH, Neely KE, Vignali M, Reese JC, Workman JL. Promoter targeting of chromatin-modifying complexes. Front Biosci 2001; 6:D1054 - 64; http://dx.doi.org/10.2741/Hassan; PMID: 11532604
- Quinlan AR, Hall IM. BEDTools: a flexible suite of utilities for comparing genomic features. Bioinformatics (Oxford, England) 2010; 26:841 - 2; http://dx.doi.org/10.2741/Hassan; PMID: 11532604
- Neph S, Vierstra J, Stergachis AB, Reynolds AP, Haugen E, Vernot B, Thurman RE, John S, Sandstrom R, Johnson AK, et al. An expansive human regulatory lexicon encoded in transcription factor footprints. Nature 2012; 489:83 - 90; http://dx.doi.org/10.1038/nature11212; PMID: 22955618
- Stranger BE, Montgomery SB, Dimas AS, Parts L, Stegle O, Ingle CE, Sekowska M, Smith GD, Evans D, Gutierrez-Arcelus M, et al. Patterns of cis regulatory variation in diverse human populations. PLoS Genet 2012; 8:e1002639; http://dx.doi.org/10.1371/journal.pgen.1002639; PMID: 22532805
- Dimas AS, Deutsch S, Stranger BE, Montgomery SB, Borel C, Attar-Cohen H, Ingle C, Beazley C, Gutierrez Arcelus M, Sekowska M, et al. Common regulatory variation impacts gene expression in a cell type-dependent manner. Science 2009; 325:1246 - 50; http://dx.doi.org/10.1126/science.1174148; PMID: 19644074
- Kwan T, Grundberg E, Koka V, Ge B, Lam KCL, Dias C, Kindmark A, Mallmin H, Ljunggren O, Rivadeneira F, et al. Tissue effect on genetic control of transcript isoform variation. PLoS Genet 2009; 5:e1000608; http://dx.doi.org/10.1371/journal.pgen.1000608; PMID: 19680542
- Hindorff LA, MacArthur J, Morales J, Junkins HA, Hall PN, Klemm AK, Manolio TA. A Catalog of Published Genome-Wide Association Studies. 2013.
- Hart AB, Engelhardt BE, Wardle MC, Sokoloff G, Stephens M, de Wit H, Palmer AA. Genome-wide association study of d-amphetamine response in healthy volunteers identifies putative associations, including cadherin 13 (CDH13). PLoS One 2012; 7:e42646; http://dx.doi.org/10.1371/journal.pone.0042646; PMID: 22952603
- Choudhry S, Taub M, Mei R, Rodriguez-Santana J, Rodriguez-Cintron W, Shriver MD, Ziv E, Risch NJ, Burchard EG. Genome-wide screen for asthma in Puerto Ricans: evidence for association with 5q23 region. Hum Genet 2008; 123:455 - 68; http://dx.doi.org/10.1007/s00439-008-0495-7; PMID: 18401594
- Jostins L, Ripke S, Weersma RK, Duerr RH, McGovern DP, Hui KY, Lee JC, Schumm LP, Sharma Y, Anderson CA, et al, International IBD Genetics Consortium (IIBDGC). Host-microbe interactions have shaped the genetic architecture of inflammatory bowel disease. Nature 2012; 491:119 - 24; http://dx.doi.org/10.1038/nature11582; PMID: 23128233
- Cotton AM, Ge B, Light N, Adoue V, Pastinen T, Brown CJ. Analysis of expressed SNPs identifies variable extents of expression from the human inactive X chromosome. Genome Biol 2013; 14:R122; http://dx.doi.org/10.1186/gb-2013-14-11-r122; PMID: 24176135
- Gimelbrant A, Hutchinson JN, Thompson BR, Chess A. Widespread monoallelic expression on human autosomes. Science 2007; 318:1136 - 40; http://dx.doi.org/10.1126/science.1148910; PMID: 18006746
- Wang Z, Zang C, Rosenfeld JA, Schones DE, Barski A, Cuddapah S, Cui K, Roh T-Y, Peng W, Zhang MQ, et al. Combinatorial patterns of histone acetylations and methylations in the human genome. Nat Genet 2008; 40:897 - 903; http://dx.doi.org/10.1038/ng.154; PMID: 18552846
- Kadota M, Yang HH, Hu N, Wang C, Hu Y, Taylor PR, Buetow KH, Lee MP. Allele-specific chromatin immunoprecipitation studies show genetic influence on chromatin state in human genome. PLoS Genet 2007; 3:e81; http://dx.doi.org/10.1371/journal.pgen.0030081; PMID: 17511522
- McDaniell R, Lee B-K, Song L, Liu Z, Boyle AP, Erdos MR, Scott LJ, Morken MA, Kucera KS, Battenhouse A, et al. Heritable individual-specific and allele-specific chromatin signatures in humans. Science 2010; 328:235 - 9; http://dx.doi.org/10.1126/science.1184655; PMID: 20299549
- Smith AJP, Howard P, Shah S, Eriksson P, Stender S, Giambartolomei C, Folkersen L, Tybjærg-Hansen A, Kumari M, Palmen J, et al. Use of allele-specific FAIRE to determine functional regulatory polymorphism using large-scale genotyping arrays. PLoS Genet 2012; 8:e1002908; http://dx.doi.org/10.1371/journal.pgen.1002908; PMID: 22916038
- Djebali S, Davis CA, Merkel A, Dobin A, Lassmann T, Mortazavi A, Tanzer A, Lagarde J, Lin W, Schlesinger F, et al. Landscape of transcription in human cells. Nature 2012; 489:101 - 8; http://dx.doi.org/10.1038/nature11233; PMID: 22955620
- Grundberg E, Adoue V, Kwan T, Ge B, Duan QL, Lam KCL, Koka V, Kindmark A, Weiss ST, Tantisira K, et al. Global analysis of the impact of environmental perturbation on cis-regulation of gene expression. PLoS Genet 2011; 7:e1001279; http://dx.doi.org/10.1371/journal.pgen.1001279; PMID: 21283786
- Sandve GK, Gundersen S, Johansen M, Glad IK, Gunathasan K, Holden L, Holden M, Liestøl K, Nygård S, Nygaard V, et al. The Genomic HyperBrowser: an analysis web server for genome-scale data. Nucleic Acids Res 2013; 41:W133-41; http://dx.doi.org/10.1093/nar/gkt342; PMID: 23632163
- Edgar R, Domrachev M, Lash AE. Gene Expression Omnibus: NCBI gene expression and hybridization array data repository. Nucleic Acids Res 2002; 30:207 - 10; http://dx.doi.org/10.1093/nar/30.1.207; PMID: 11752295