Abstract
The vinegar flies Drosophila subobscura and D. obscura frequently serve as study organisms for evolutionary biology. Their high morphological similarity renders traditional species determination difficult, especially when living specimens for setting up laboratory populations need to be identified. Here we test the usefulness of cuticular chemical profiles collected via the non-invasive method near-infrared spectroscopy for discriminating live individuals of the two species. We find a classification success for wild-caught specimens of 85%. The species specificity of the chemical profiles persists in laboratory offspring (87–92% success). Thus, we conclude that the cuticular chemistry is genetically determined, despite changes in the cuticular fingerprints, which we interpret as due to laboratory adaptation, genetic drift and/or diet changes. However, because of these changes, laboratory-reared specimens should not be used to predict the species-membership of wild-caught individuals, and vice versa. Finally, we demonstrate that by applying an appropriate cut-off value for interpreting the prediction values, the classification success can be immensely improved (to up to 99%), albeit at the cost of excluding a considerable portion of specimens from identification.
Introduction
Drosophila obscura and D. subobscura (Diptera: Drosophilidae) are closely related species of the D. obscura group,Citation1 with a wide distribution in the Palaearctic. Both are generalists and co-occur broadly in the colline and alpine zone.Citation2 They are frequently used species in evolutionary-biological studies (for review see refs. Citation3–Citation7). Accurate species identification of living specimens of both sexes is difficult, as the two species are morphologically highly similar,Citation8 with considerable intraspecific variation in the diagnostic characters.Citation9 The problem is aggravated by the need to keep to a minimum the anesthesia by CO2, to avoid reduced longevity and fecundity.Citation10,Citation11 For introducing wild-caught individuals to the laboratory with the aim to retain genetic variation, a rapid and non-destructive method for species identification with the potential for high throughput would thus be desirable as an alternative to morphology-based methods.
Insect cuticular layers contain complex mixtures of hydrocarbons (CHCs), many of which are synthesized by the insect itself, i.e., supposedly genetically determined.Citation12 In addition to their central role in the prevention of desiccation,Citation13 CHCs are important for chemical communication, for example in mate choiceCitation14,Citation15 and social behavior.Citation16 The idea that the bouquet of CHCs will thus be species specific led researchers to enquire into their usefulness in species identificationCitation17,Citation18 and various examples of success exist.Citation19 We decided to test the usefulness of near-infrared spectroscopy (NIRS) to discriminate between D. subobscura and D. obscura: Previous studies suggested differences in desiccation resistance between the two species,Citation8 and, possibly due to a lack of interspecific mate recognition, no hybridization between them has been reported as yet,Citation20 both of which may involve CHC species specificity.
NIRS characterizes chemical patterns qualitatively and quantitatively based primarily on C-H, N-H, O-H stretching vibrations.Citation21 It is thus a useful tool for the characterization of biological material, and is, also owing to its non-destructive and non-invasive nature, becoming common practice in ecologyCitation22 and entomology.Citation23,Citation24 Of relevance here, it was successfully used in the identification of (non-drosophilid) dipterans.Citation25-Citation27
The objectives of this study were to determine if NIRS (1) can be used to discriminate living D. obscura and D. subobscura specimens by using multivariate chemometrics, and (2) whether calibration models elaborated for wild-caught specimens and for specimens from different laboratory-reared generations can be cross-applied. Cross-applicability would reduce significantly the effort needed for establishing the identification of specimens with such differing backgrounds. However, it needs to be kept in mind that genetic and phenotypic changes can arise from evolution in a novel environment.Citation28 The CHC bouquet, in particular, can evolve due to changes in the ambient thermal regime and in diet compositionCitation29 but can also change due to acquisition of hydrocarbons from food.Citation30
Results
Statistical parameters of the partial least squares (PLS) calibration models (number of PLS factors used, coefficient of determination (r2) and standard error of cross validation (SECV)) and the classification results for the validation sets are listed in . The calibration models had r2 values between 0.43 and 0.63, and SECV values between 0.33 and 0.40. The correct classification for the validation sets ranged between 85% and 92%; the best prediction results were achieved for the eighth lab-reared generation (F8), with 90% for F8 males (F8 min) and 92% for F8 females (F8f).
Table 1. Classification results of Drosophila subobscura and D. obscura based on PLS regression models developed from near-infrared spectra (500–2200 nm).
We then explored how the exclusion of prediction values around 1.5 influenced classification rate and loss of specimens, by symmetrically excluding values below and above 1.5, decreasing and increasing in steps of 0.02 to the extremes of 1.0 and 2.0, respectively. Exclusion of values between 1.4 and 1.6 resulted in, for example, the F8 females in an increase of the correct classification from 92% to 96% and in the exclusion of 10% of specimens (). For the other models and validation sets, the corresponding values were similar at increases of correct classification to 90–91% and 9–11% of individuals excluded (); for our data set sizes, we found this to represent an acceptable compromise across models between accuracy and number of specimens excluded. When values 1.30–1.70 and 1.20–1.80 were excluded, the success rate for F8f increased to 97% and 99%, but the portion of specimens identified dropped to 78% and 65%, respectively ().
Figure 1. The portion of correctly classified specimens depends on the exclusion of spectra with ambiguous prediction values, exemplified by the F8 females. The thin line shows the increasing loss of individuals with increasing range of excluded prediction values, the bold line shows the corresponding increase in correct classification. Exclusion ranges in boxes are discussed in the text.
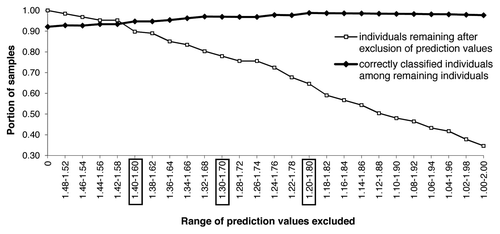
Wavelengths important for the identification of D. subobscura and D. obscura were identified from the PLS regression coefficients, with wavelengths showing very high or very low coefficients being more important. There were peaks occurring in all of the five calibration models and peaks that were important only in single models. shows the regression-coefficient plot for F8f.
Figure 2. PLS regression coefficient used for identifying important wavelengths for classification of Drosophila subobscura and D. obscura females from the F8.
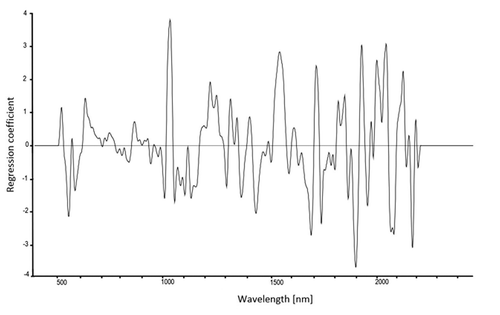
When calibration models created for one group were used to predict validation sets from the other groups, the classification success ranged from 56% to 83% (; prediction values between 1.4 and 1.6 excluded).
Table 2. Correct classification rate (%) for validation sets performed on the different calibration models to test their cross-applicability (classification values 1.4–1.6 excluded).
Discussion
Here we show that NIRS can be used to distinguish between Drosophila subobscura and D. obscura with an accuracy of 85% to 92% using PLS analysis, when using the full range of prediction values. This indicates that the composition of CHCs may differ between the two species. We cannot directly relate NIR-spectral differences to CHCs, and also the visible spectral range was relevant to successful PLS models (see further down), but we assume that CHC composition contributed significantly to species differences (compare refs. Citation14–Citation19). The prediction results for the wild-caught flies were comparable to those obtained for laboratory-reared specimens, in line with the notion that hydrocarbon profiles are more genetically than environmentally determinedCitation31 – although the two species were reared under the same conditions, differences in the cuticular profiles persisted and were detectable by NIRS. These findings contrast the NIRS study by Mayagaya et al.Citation25 who predicted two Anopheles species reared in the laboratory with an accuracy of almost 100%, and field-collected specimens with 80% accuracy. Including both wild-caught flies and laboratory-reared flies (from all generations) in the same model did not improve our prediction results, the best models resulting in 82% and 79% prediction success for females and males, respectively (S. Fischnaller, unpubl.). However, from the practical point of view of setting up breeding lines based on identification via NIRS, our error rates are not fatal, given that Drosophila obscura and D. subobscura do not hybridize.Citation20 Hence, no interspecific gene flow is expected for unintentionally heterospecific cultures, and the identification procedure can be repeated in consecutive generations.
The lower rate of correct classification in our study as compared with the work by Rodriguez-Fernandez et al.,Citation27 who used nine Diptera species, could be caused by a closer phylogenetic relatedness of our species as well as by our including multiple populations in the sample – genetic diversity was found to be very high across other wild populations of D. subobscura.Citation28 Furthermore, we included individuals of all ages, and thus likely both unmated and mated individuals, in our calibration and validation sets. NIRS is sensitive to the age of individuals, and thus used for age-grading of various insects,Citation25,Citation32,Citation33 and Everaerts et al.Citation34 showed that in Drosophilidae, in both females and males, CHC changes occur during mating. The variation introduced by either or both of these effects may possibly have impeded greater success of our calibration procedures.
One way to improve classification is the exclusion of specimens with prediction values around 1.5 (, ). This procedure was suggested by Sikulu et al.Citation26 in general, but to our knowledge the trade-off between increase of classification success and loss of specimens has not yet been explored in a quantitative manner. We suggest that such exploration be adopted as a standard procedure in NIRS species-identification studies. Depending on the demands for the specific project, researchers could thus prioritise either classification success or number of specimens identified in a controlled manner. Another way of improving accuracy with our species could be to scan just wings. Using the pulled-out right wings of thawed males in NIRS analysis enabled us to distinguish D. subobscura from D. obscura with 100% accuracy (n = 50 males per species; data not shown). This is in line with the findings from Shevtsova et al.Citation35 who found high interspecific variation in the wing interference patterns of Drosophilidae. Scanning just wings of live specimens is very difficult to put into praxis, however, due to the need for standardised positioning of wings on the one hand and minimum CO2 exposure of specimens at the other (S. Fischnaller, unpubl.). Exploring this possibility in depth remains subject to future exploration.
Examination and comparison of the regression coefficient plots indicated that there are peaks important to species discrimination that are common to all five calibration models. The region around 510, 540 and 610 nm indicates that there are differences between the two species in the visible region, possibly caused by variation in cuticle thickness, bristles and/or pigmentation.Citation35 The region of 1050–1070 nm indicates vibration of water molecules at the third overtone, as well as occurrence of molecules containing N-H functional groups (ref. Citation36, also used for the interpretation of the subsequently listed wavelengths). Peaks at 1370–1390 nm (CH2 second overtone, and water), 1720–1730 nm (CH3 first overtone), 1810–1840 nm and 1870 nm (C-H first overtone, water), and 2140–2180 nm (N-H and O-H combination bands) also contributed to all calibration models.
Our study suggests that wild-caught specimens of our species should not be used to identify laboratory-reared specimens, and vice versa, due to excessive failure rates (). This contrasts the findings of Mayagaya et al.Citation25 of 79% correctly classified wild-caught Anopheles when using models based on laboratory-reared individuals. Our low success rate is supported by absorption peaks in the regression coefficients exclusive to just one of the calibration models (e.g., 1025 nm, 1460 nm in Wm; 1500 nm, 2050 nm in F1 min; 1770 nm in F1f; 2000 nm in F8f). In other words, chemical differences led to the observed generation specificity of the models. Toolson and Kuper-SimbronCitation29 reported for Drosophila pseudoobscura that maintenance in the laboratory leads to physiological and biochemical changes. They reported a shift in the cuticular composition even for the first generation of large populations reared in the laboratory, and explained it by changes of selective pressure and fitness advantages under novel environmental factors. Especially in small populations genetic drift can additionally increase the genetic differentiation across populations (D. subobscura: see refs. Citation28, Citation37). Also, hydrocarbon profiles can change in a non-inherited manner due to acquisition of food-derived hydrocarbons (ant example: ref. Citation30). Thus, changes in the metabolic profiles – either due to genetic or environmental changes – may have altered the recorded NIRS data across generations, impeding the use of calibration models generated for one generation in the others. Future research should aim to pinpoint potential non-inherited contributions as well as assess if this problem ceases in later generations which would indicate that it is due to rapid laboratory adaptationCitation5,Citation38 or whether larger population sizes diminish it which would indicate that it is due to genetic drift (but note that our population sizes were in line with general practice, e.g., FryCitation39).
In conclusion, there are three main findings to our study: First, near-infrared spectroscopy proved a useful tool for the identification of living Drosophila flies. Second, we could not cross-apply models and validation sets among field-caught and lab-reared individuals and across generations, indicating changes due to laboratory adaptation, genetic drift and/or diet changes. Third, classification rates could be considerably improved by excluding prediction values around 1.5, suggesting that researchers should consider excluding a particular range of prediction values depending on their research question. Our study thus underscores the enormous potential of the NIRS technique to species identification (e.g., refs. Citation24, Citation25, Citation26, Citation40, Citation41), and indicates that it could become an important tool also for the delimitation of species in integrative taxonomy,Citation42 as well as in other biological fields.Citation43
Materials and Methods
Insects
Specimens were collected from six different locations in North Tyrol (Austria) during August and September 2010. To represent a wide range of habitats, the collection sites were chosen from various altitudes between 570 and 2000 min above sea level (). The minimum and maximum distances between populations were 2 and 60 km, respectively. Collecting was done by net sweeping over baits of fermented bananaCitation44 in the evening hours from 5 to 7 p.m. The field-caught flies were transported alive to the laboratory and anaesthetised with CO2 for morphology-based species identification. CO2 exposure length for species identification, as well as for spectra collection (see below), was kept to a minimum and never exceeded four minutes per specimen. Flies that were identified as D. subobscura or D. obscura according to Bächli and BurlaCitation9 were used to set up breeding lines for each location sampled. All lines were kept at a minimum census size of 60 individuals on an artificial diet (corn-meal, sugar, agar, yeast, Tegosept) and at a photoperiod of 12/12 h (light/dark) at 19°C.
Table 3. Sampling data for field-collected Drosophila obscura and D. subobscura.
Data collection
Spectra were collected from anaesthetised flies using a Labspec® 5000 Portable Vis/NIR Spectrometer (350–2,500 nm; ASD Inc.) by placing flies individually on their backs on a 9 cm diameter Spectralon plate. The 3 mm diameter bifurcated fiber-optic probe was positioned about 2 mm above the specimen, focusing on the abdomen. The spectrometer automatically calculated and saved the average spectrum of 30 collected spectra of each individual. Background reference (the baseline) was measured using a separate 3 cm diameter Spectralon plate to avoid contamination. All field-caught individuals as well as 251 randomly chosen individuals of the F1 and 421 of the F8 of the breeding lines were sexed and scanned. We thus included a wide range of individual ages in our sample.
Data analysis
All recorded spectra were converted into Galactic spectrum file format using ASD ViewSpecPro. Spectra used for the calibration sets were pre-processed by mean-centring and analyzed using PLS regression and leave-one-out cross validationCitation45,Citation46 implemented in GRAMS software PLS/IQ. Spectra were generally very noisy below 500 nm and above 2200 nm and these regions were excluded from further analysis. Calibration models were elaborated separately for males (m) and females (f), because females can be easily distinguished from males and because Drosophila sexes differ in their CHC-profiles.Citation34 We performed models for the following five groups: (1) the wild, field-collected males, referred to as “Wm” (due to the low number of field-caught D. obscura females, no model could be created for this group), (2) the first lab-reared generation, referred to as “F1m” and (3) “F1f,” and (4) the eighth lab-reared generation, referred to as “F8m” and (5) “F8f.”
The training sets for each calibration model contained 70 spectra (35 of each species). A two-way comparison in PLS analysis was made by assigning integer values 1 and 2 to D. subobscura and D. obscura, respectively. Independent validation sets, treated as “unknown” specimens, were then classified on the basis of the calibration model in each group. Spectra predicted to have a class value of ≤ 1.5 were considered to belong to D. subobscura, those with a predicted value of ≥ 1.5 to D. obscura. The numbers of PLS regression factors to be used in the prediction models were determined by examining the values of the predicted residual sum of squaresCitation46 and the classification results of the independent validation sets. Accuracy of the calibration models was examined by checking the r2 indicating the closeness of fit between NIRS and reference data, the SECV of the leave-one-out procedure, and by calculating the prediction results using the validation sets—the most rigorous indicator of model quality.Citation47 Spectral residuals, which were possibly due to technical problems such as movement of insufficiently anaesthetised specimens, were discarded from the sample. Such outliers were detected by visual examination of the spectra using spekwin32 (F. Menges “Spekwin32—free software for optical spectroscopy”- Vers.1.71.5, 2010, http://www.effemm2.de/spekwin/) and by examination of the leverage and studentised residuals plots generated in GRAMS (compare ref. Citation48).
Abbreviations: | ||
CHCs | = | cuticular hydrocarbons |
NIRS | = | near-infrared spectroscopy |
PLS | = | partial least squares |
Wm | = | field-collected males |
F1 | = | first lab-reared generation |
F8 | = | eighth lab-reared generation |
r2 | = | coefficient of determination |
SECV | = | standard error of cross validation |
Acknowledgments
We thank Heike Perlinger and Clemens Folterbauer for assistance in the laboratory, Alexander Rief for sampling assistance, Regina Medgyesy for help in compiling literature, Gerhard Bächli for help with morphological species identification, and two anonymous referees for constructive criticism. This research was funded by the University of Innsbruck; FMS was supported by FWF P 23949-B17.
Disclosure of Potential Conflicts of Interest
No potential conflicts of interest were disclosed.
References
- Gao JJ, Watabe HA, Aotsuka T, Pang JF, Zhang YP. Molecular phylogeny of the Drosophila obscura species group, with emphasis on the Old World species. BMC Evol Biol 2007; 7:87; http://dx.doi.org/10.1186/1471-2148-7-87; PMID: 17555574
- Bächli G. Quantitative methods for population analysis applied to a Drosophila (Diptera, Drosophilidae) collection. Aquilo (Zool) 1979; 20:33 - 40
- Santos M, Iriarte PF, Céspedes W, Balanyà J, Fontdevila A, Serra L. Swift laboratory thermal evolution of wing shape (but not size) in Drosophila subobscura and its relationship with chromosomal inversion polymorphism. J Evol Biol 2004; 17:841 - 55; http://dx.doi.org/10.1111/j.1420-9101.2004.00721.x; PMID: 15271084
- Matos M, Simões P, Duarte A, Rego C, Avelar T, Rose MR. Convergence to a novel environment: comparative method versus experimental evolution. Evolution 2004; 58:1503 - 10; PMID: 15341152
- Simões P, Santos J, Fragata I, Mueller LD, Rose MR, Matos M. How repeatable is adaptive evolution? The role of geographical origin and founder effects in laboratory adaptation. Evolution 2008; 62:1817 - 29; http://dx.doi.org/10.1111/j.1558-5646.2008.00423.x; PMID: 18489721
- Zivanovic G, Mestres F. Changes in chromosomal polymorphism and global warming: The case of Drosophila subobscura from Apatin (Serbia). Genet Mol Biol 2011; 34:489 - 95; PMID: 21931524
- Dolgova O, Rego C, Calabria G, Balanyà J, Pascual M, Rezende EL, et al. Genetic constraints for thermal coadaptation in Drosophila subobscura.. BMC Evol Biol 2010; 10:363; http://dx.doi.org/10.1186/1471-2148-10-363; PMID: 21108788
- Koch R. Tagesperiodik der Aktivität und der Orientierung nach Wald und Feld von Drosophila subobscura und Drosophila obscura.. Z Vgl Physiol 1967; 54:353 - 94; http://dx.doi.org/10.1007/BF00298224
- Bächli G, Burla H. Insecta Helvetica: Fauna. Diptera: Drosophilidae. 1st edn. Zürich: Schweizerische Entomologische Gesellschaft, 1985.
- Barron AB. Anaesthetising Drosophila for behavioural studies. J Insect Physiol 2000; 46:439 - 42; http://dx.doi.org/10.1016/S0022-1910(99)00129-8; PMID: 12770207
- Champion de Crespigny FE, Wedell N. The impact of anaesthetic technique on survival and fertility in Drosophila.. Physiol Entomol 2008; 33:310 - 5; http://dx.doi.org/10.1111/j.1365-3032.2008.00632.x
- Blomquist GJ, Bagnères AG. Introduction: history and overview of insect hydrocarbons. In: Blomquist GJ, Bagnères AG, eds. Insect hydrocarbons: Biology, biochemistry, and chemical ecology. New York: Cambridge University Press, 2010:3-19.
- Toolson EC. Effects of rearing temperature on cuticle permeability and epicuticular lipid composition in Drosophila pseudoobscura.. J Exp Zool 1982; 222:249 - 53; http://dx.doi.org/10.1002/jez.1402220307
- Cobb M, Jallon JM. Pheromones, mate recognition and courtship stimulation in the Drosophila melanogaster species sub-group. Anim Behav 1990; 39:1058 - 67; http://dx.doi.org/10.1016/S0003-3472(05)80778-X
- Mas F, Jallon JM. Sexual isolation and cuticular hydrocarbon differences between Drosophila santomea and Drosophila yakuba.. J Chem Ecol 2005; 31:2747 - 52; http://dx.doi.org/10.1007/s10886-005-7570-5; PMID: 16132336
- Dahanukar A, Ray A. Courtship, aggression and avoidance: pheromones, receptors and neurons for social behaviors in Drosophila. Fly (Austin) 2011; 5:58 - 63; PMID: 20962580
- Carlson DA, Service MW. Differentiation between species of the Anopheles gambiae Giles complex (Diptera: Culicidae) by analysis of cuticular hydrocarbons. Ann Trop Med Parasitol 1979; 73:589 - 92; PMID: 539859
- Lockey KH. Insect hydrocarbon classes: Implication for chemotaxonomy. Insect Biochem 1991; 21:91 - 7; http://dx.doi.org/10.1016/0020-1790(91)90068-P
- Bagnères AG, Wicker-Thomas C. Chemical taxonomy with hydrocarbons. In: Blomquist GJ, Bagnères AG, eds. Insect hydrocarbons: Biology, biochemistry, and chemical ecology. New York: Cambridge University Press, 2010:3-19.
- Koske T. Artkreuzungsversuche in der obscura-Gruppe der Gattung Drosophila.. Mol Genet Genomics 1953; 85:373 - 81; http://dx.doi.org/10.1007/BF00309674
- Miller CE. Chemical principles of near-infrared technology. In: Williams P, Norris K, eds. Near-infrared technology in the agricultural. 2nd edn. St. Paul, USA: American Association of Cereal Chemists Inc, 2001:19-37.
- Foley JW, McIlwee A, Lawler I, Aragones L, Woolnough AP, Berding N. Ecological applications of near-infrared reflectance spectroscopy – a tool for rapid, cost-effective prediction of the composition of plant and animal tissues and aspects of animal performance. Oecologia 1998; 116:293 - 305; http://dx.doi.org/10.1007/s004420050591
- Pasquini C. Near infrared spectroscopy: fundamentals, practical aspects and analytical applications. J Braz Chem Soc 2003; 14:198 - 219; http://dx.doi.org/10.1590/S0103-50532003000200006
- Klarica J, Bittner L, Pallua J, Pezzei C, Huck-Pezzei V, Dowell FE, et al. Near-infrared imaging spectroscopy as a tool to discriminate two cryptic Tetramorium ant species. J Chem Ecol 2011; 37:549 - 52; http://dx.doi.org/10.1007/s10886-011-9956-x; PMID: 21537901
- Mayagaya VS, Michel K, Benedict MQ, Killeen GF, Wirtz RA, Ferguson HM, et al. Non-destructive determination of age and species of Anopheles gambiae s.l. using near-infrared spectroscopy. Am J Trop Med Hyg 2009; 81:622 - 30; http://dx.doi.org/10.4269/ajtmh.2009.09-0192; PMID: 19815877
- Sikulu M, Killeen GF, Hugo LE, Ryan PA, Dowell KM, Wirtz RA, et al. Near-infrared spectroscopy as a complementary age grading and species identification tool for African malaria vectors. Parasit Vectors 2010; 3:49; http://dx.doi.org/10.1186/1756-3305-3-49; PMID: 20525305
- Rodríguez-Fernández JI, De Carvalho CJB, Pasquini C, De Lima KMG, Moura MO, Arízaga GGC. Barcoding without DNA? Species identification using near infrared spectroscopy. Zootaxa 2011; 2933:46 - 54
- Simões P, Pascual M, Santos J, Rose MR, Matos M. Evolutionary dynamics of molecular markers during local adaptation: a case study in Drosophila subobscura.. BMC Evol Biol 2008; 8:66 - 77; http://dx.doi.org/10.1186/1471-2148-8-66; PMID: 18302790
- Toolson EC, Kuper-Simbron R. Laboratory evolution of epicuticular hydrocarbon composition and cuticular permeability in Drosophila pseudoobscura: effects on sexual dimorphism and thermal-acclimation ability. Evolution 1989; 43:468 - 73; http://dx.doi.org/10.2307/2409222
- Liang D, Silverman J. “You are what you eat”: diet modifies cuticular hydrocarbons and nestmate recognition in the Argentine ant, Linepithema humile.. Naturwissenschaften 2000; 87:412 - 6; http://dx.doi.org/10.1007/s001140050752; PMID: 11091966
- Mullen SP, Mendelson TC, Schal C, Shaw KL. Rapid evolution of cuticular hydrocarbons in a species radiation of acoustically diverse Hawaiian crickets (Gryllidae: trigonidiinae: Laupala). Evolution 2007; 61:223 - 31; http://dx.doi.org/10.1111/j.1558-5646.2007.00019.x; PMID: 17300441
- Perez-Mendoza J, Dowell FE, Broce AB, Throne JE, Wirtz RA, Xie F, et al. Chronological age-grading of house flies by using near-infrared spectroscopy. J Med Entomol 2002; 39:499 - 508; http://dx.doi.org/10.1603/0022-2585-39.3.499; PMID: 12061447
- Reeves WK, Peiris KH, Scholte EJ, Wirtz RA, Dowell FE. Age-grading the biting midge Culicoides sonorensis using near-infrared spectroscopy. Med Vet Entomol 2010; 24:32 - 7; http://dx.doi.org/10.1111/j.1365-2915.2009.00843.x; PMID: 20377729
- Everaerts C, Farine JP, Cobb M, Ferveur JF. Drosophila cuticular hydrocarbons revisited: mating status alters cuticular profiles. PLoS One 2010; 5:e9607; http://dx.doi.org/10.1371/journal.pone.0009607; PMID: 20231905
- Shevtsova E, Hansson C, Janzen DH, Kjærandsen J. Stable structural color patterns displayed on transparent insect wings. Proc Natl Acad Sci U S A 2011; 108:668 - 73; http://dx.doi.org/10.1073/pnas.1017393108; PMID: 21199954
- Coates J. Interpretation of infrared spectra, a practical approach. In: Meyers RA, ed. Encyclopedia of Analytical Chemistry. Chichester: John Wiley & Sons Ltd, 2000:10815-37.
- Matos M, Avelar T, Rose MR. Variation in the rate of convergent evolution: adaptation to a laboratory environment in Drosophila subobscura.. J Evol Biol 2002; 15:673 - 82; http://dx.doi.org/10.1046/j.1420-9101.2002.00405.x
- Matos M, Rose MR, Rocha Pité MT, Rego C, Avelar T. Adaptation to the laboratory environment in Drosophila subobscura.. J Evol Biol 2000; 13:9 - 19; http://dx.doi.org/10.1046/j.1420-9101.2000.00116.x
- Fry JD. Detection of ecological trade-offs using selection experiments. Ecology 2003; 84:1672 - 8; http://dx.doi.org/10.1890/0012-9658(2003)084[1672:DETUSE]2.0.CO;2
- Aldrich BT, Maghirang EB, Dowell FE, Kambhampati S. Identification of termite species and subspecies of the genus Zootermopsis using near-infrared reflectance spectroscopy. J Insect Sci 2007; 7:1 - 7; http://dx.doi.org/10.1673/031.007.1801; PMID: 20307237
- Cole TJ, Ram MS, Dowell FE, Omwega CO, Overholt WA, Ramaswamy SB. Near-infrared spectroscopic method to identify Cotesia flavipes and Cotesia sesamiae (Hymenoptera: Braconidae). Ann Entomol Soc Am 2003; 96:865 - 9; http://dx.doi.org/10.1603/0013-8746(2003)096[0865:NSMTIC]2.0.CO;2
- Schlick-Steiner BC, Steiner FM, Seifert B, Stauffer C, Christian E, Crozier RH. Integrative taxonomy: a multisource approach to exploring biodiversity. Annu Rev Entomol 2010; 55:421 - 38; http://dx.doi.org/10.1146/annurev-ento-112408-085432; PMID: 19737081
- Cruickshank RH, Munck L. It's barcoding Jim, but not as we know it. Zootaxa 2011; 2933:55 - 6
- Bächli G, Schatzmann E, Haring E. On some population parameters of drosophilids in Switzerland (Diptera, Drosophilidae). Mitt Schweiz Entomol Ges 2008; 81:243 - 60
- Wold S, Sjöström M, Eriksson L. PLS-regression: a basic tool of chemometrics. Chemometr Intell Lab 2001; 58:109 - 30; http://dx.doi.org/10.1016/S0169-7439(01)00155-1
- Abdi H. Partial least squares regression and projection on latent structure regression (PLS Regression). . WIREs Comput Stat 2010; 2:97 - 106
- Kessler W. Kalibrieren, Validieren, Vorhersagen. In: Kessler W, ed. Multivariate Datenanalyse: für die Pharma-, Bio- und Prozessanalytik. Weinheim: Wiley-VCH Verlag GmbH & Co.KgaA, 2007:153-181.
- Martens H, Naes T. Multivariate calibration by data compression. In: Williams P, Norris K, eds. Near-infrared technology in the agricultural. 2nd edn. St. Paul, USA: American Association of Cereal Chemists Inc, 2001:19-37.