Abstract
The Pfam database groups regions of proteins by how well hidden Markov models (HMMs) can be trained to recognize similarities among them. Conservation pressure is probably in play here. The Pfam seed training set includes sequence and structure information, being drawn largely from the PDB. A long standing hypothesis among intrinsically disordered protein (IDP) investigators has held that conservation pressures are also at play in the evolution of different kinds of intrinsic disorder, but we find that predicted intrinsic disorder (PID) is not always conserved across Pfam domains. Here we analyze distributions and clusters of PID regions in 193024 members of the version 23.0 Pfam seed database. To include the maximum information available for proteins that remain unfolded in solution, we employ the 10 linearly independent Kidera factors1–3 for the amino acids, combined with PONDR4 predictions of disorder tendency, to transform the sequences of these Pfam members into an 11 column matrix where the number of rows is the length of each Pfam region. Cluster analyses of the set of all regions, including those that are folded, show 6 groupings of domains. Cluster analyses of domains with mean VSL2b scores greater than 0.5 (half predicted disorder or more) show at least 3 separated groups. It is hypothesized that grouping sets into shorter sequences with more uniform length will reveal more information about intrinsic disorder and lead to more finely structured and perhaps more accurate predictions. HMMs could be trained to include this information.
Table 10. Members from 618 unique mammalian Pfam 23.0 domainsa that are 100% PID.
Introduction
Intrinsically disordered proteins (IDPs) and regions (IDRs) have biological activities that, at least for part of the time, require the absence of stable 3-dimensional or secondary structure under physiological conditions.Citation5-Citation17 Numbers estimating the amount of intrinsic disorder in proteins are stunning; about 43% of known mammalian protein sequence is in predicted intrinsic disorder (PID).Citation18-Citation21 Between 35 and 51% of eukaryotic proteins have been predicted to contain IDRs that span 40 or more residues.Citation22 Between 25 and 30% of eukaryotic proteins have been predicted to be half intrinsically disordered or more.Citation23 More than 70% of signaling proteins, and most of the cancer-associated proteins have been predicted to contain long disordered regions.Citation24
DisprotCitation25,Citation26 is the repository for experimentally verified and annotated IDP data. Sequence/structure information in Disprot has been used by members of the IDP community to build more than 50 different methods for predicting regions of intrinsic disorder in proteins,Citation27-Citation31 and to estimate statistics for the accuracy of these methods. Nine of these predictors, along with predictions for 10,429,761 sequences in 1,765 proteomes from 1,256 distinct species, are available on the D2PCitation2 site.Citation32
Direct experimental evidence for the existence of IDPs and IDRs comes primarily from the protein data bank (PDB)Citation53 where NMR solution structures show conformational ensembles that clearly indicate dynamic disorder. Many PDB entries contain segments of protein sequence that are completely missing from X-ray and neutron diffraction crystal structures, but as is well-known by crystallographers, these segments can correspond to structured regions that are unobserved for a variety of technical reasons. For this reason, intrinsic disorder cannot be assigned to residues in crystallized proteins solely because they are not located.
Nevertheless, 7% of the crystal structures in the PDB, which is highly selective for ordered proteins, have been assigned to IDRs longer than 10 residues.Citation54,Citation55 IDPs and proteins with significant IDRs continue to resist crystallization, a situation that is unlikely to change.Citation56,Citation57 With few exceptions, crystal structures of largely disordered proteins have been obtained only from relatively small isolated sections of biologically active IDPs, many of them co-crystallized with, or covalently bound to, much larger structured molecular complexes. Even under these conditions many residues in the IDRs cannot be located in the electron density, and the crystallized complex may not represent the interaction in solution.Citation58
Solution NMR data from a variety of chemical shift, relaxation, and heteronuclear NOE measurements yield unique information about the spectrum of conformational disorder and dynamics in proteins that is less specific but more accurate than that obtained from diffraction measurements.Citation59 Taken together, and supported by circular dichroism, vibrational spectroscopy, chromatography, and small angle scattering methods, these measurements provide certainty about the extent of static and dynamic disorder in IDPs and IDRs,Citation59,Citation60 and this information is recorded in the Disprot database.Citation25 There are many biophysical techniques that can be used to characterize dynamic structure of IDPs, and many of these methods have been the subjects of focused reviews and books.Citation61-Citation67
The p53 and 14–3-3 proteins provide 2 particularly striking examples of the biological activity of intrinsically disordered proteins.Citation13 The p53 protein has several different IDRs that bind to different partners, and some IDRs that each bind in different conformations to several different partners, an association termed “one-to-many.” 14-3-3 on the other hand is a structured protein that binds many different intrinsically disordered partners in associations termed “many-to-one”Citation13.
The Pfam-A databaseCitation68 is a curated collection of biologically conserved, and for many—functional, regions in proteins. Pfam sequences are grouped in large part by function and used to train hidden Markov models (HMMs) that are used to find similar regions in proteins where there is no protein based evidence of biological activity. The training set of sequences, Pfam-A.seed, contains regions from proteins that have been experimentally validated. It is this set that has been used in the present study to relate biological function with intrinsic disorder.
There have been 2 previous studies of IDRs in Pfam domains. Recent work,Citation69 looked at 71,974 version 22.0 Pfam-A seed members of 6,857 unique domains, limited to those that included GO annotations or had at least one literature citation. 12.14% of the domains had greater than 50% predicted disorder, and 4.15% were fully (95–100%) disordered. The high percentage of fully disordered domains was attributed to the uneven length distribution of domains, with somewhat shorter domains dominating at high percentage of disorder.Citation69 Earlier, 40% of Pfam domains were shown to contain conserved protein fragments that were predicted to be disordered (conserved disorder predictions, CDPs).Citation70 These CDPs were found in proteins from all domains/kingdoms of life, including viruses, with eukaryota having one order of magnitude more proteins containing long disordered regions than did archaea and bacteria. Functional analyses revealed that CDP regions frequently participate in signaling, regulation, and interaction with DNA/RNA and other proteins, common in ribosomal proteins.Citation71 In the present work these findings reported earlier are reexamined in detail with some new results.
Here we analyze the distribution of intrinsic disorder in Pfam domain sequences using the 10 dimensional space provided by the Kidera factors for the 20 naturally occurring amino acidsCitation1-Citation3,Citation72-Citation76 combined with PONDRCitation4 predictions of intrinsic disorder. The Kidera factors have been developed expressly to describe properties of the amino acid residues that may be related to protein folding with a minimum number of parameters. They are derived from a multivariate statistical analysis beginning with 188 quantitative measurements of the amino acids available in 1985. Because this set of factors contains most of the measurable information relating the amino acids, it is possible to estimate the numerical values for amino acids where measurements may be missing.
Four of the Kidera factors, helix/bend preference, side-chain size, extended structure preference, and hydrophobicity are essentially pure factors (). Each one has been derived from a cluster of measurements of the same property. For example, the cluster for hydrophobicity contains only the relatively large set of measurements related to amino acid solubility.
Table 1. Labels and abbreviations used here for the Kidera factorsCitation1
The remaining 6 factors consist of weighted linear combinations of different measurements, labeled for convenience by the name of the most heavily weighted component.Citation3 Where factors appear to have names related to similar properties, the similarity is in name only. For example, the vectors composed of the 10 factors for extended structure preference (ext) and flat extended preference (fle) for each of the 20 amino acids are themselves orthonormal. Likewise, the similarly named pairs hel/alp and siz/psb are also orthonormal (); there is no correlation between these factors.
As indicated by the authors, the Kidera factors are orthonormal by design to avoid problems arising from incompleteness and correlation (they are normalized and their inner product is zero.) They do not contain information about interactions as may arise from an analysis of a length of sequence for periodicity or interactions.
Information about interactions can be derived from a sliding window analysis of any of the 10 factors. As we show here, an average over sets of orthonormal factors can yield correlated results with reduced information content. However, an analysis of periodicity in sliding windows can also increase information content.
Some of the factors included in the original Kidera data set have been included in the set used to train the PONDR predictors used to make predictions here. These include the hydrophobicity scales from Kyte and DoolittleCitation35 and from Rose (for predicting turns in globular proteins),Citation77 and side chain volume.Citation78 Also included in PONDR training were charge (K+RD-E), aromatic count (W + F + Y), and coordination number,Citation79 which correlate strongly with hydrophobicity and side chain volume. Side chain volume, related to the convenience named partial specific volume Kidera factor, does not come close to being a pure physical property ( and ref. Citation3) and by itself is incomplete. Other factors included in PONDR training depend on the Kidera information in lengths of sequence and include a flexibility index calculated from a sliding window,Citation80 the hydrophobic periodicity moments from Eisenberg, Weiss, and Terwilliger,Citation81,Citation82 codon number,Citation83 and alphabet size.Citation84 These scales are derived from a small part of the information contained in the Kidera data.
The PONDR predictors continue to be among the most accurate available,Citation85,Citation86 suggesting that hydrophobicity, may be among the principle physical properties of the amino acids determining the tendency of proteins to evolve with functions in intrinsically disordered states. However, we show here that all 10 of the Kidera factors contribute to a clustering of different types of predicted intrinsic disorder in Pfam domains, and that plots of PONDR VSL2b predictor scores against hydrophobicity have a spread and appearance that is similar to that seen in plots of the VSL2b scores against any of the other Kidera factors. The inclusion of the Kidera factors in the training of disorder predictors could hypothetically increase the information content available to an analysis of intrinsic protein disorder by several fold.
Results and Discussion
The results are shown and annotated in the figures and tables. Note that while includes the 15‒20% of Pfam members that contained no PID, subsequent figures exclude members with 0% PID by simply excluding data below 2% from the plot.
Figure 1. End effects do not contribute significantly to disorder distributions, as is shown here and in in a comparison of the distribution of percent predicted disorder, as a function of the percentage of predicted disorder in 2% wide bins, in all Pfam seed proteins where domain members start or end within 19, 29, and 39 residues of the whole seed protein ends. All predictions in this work were performed on whole proteins, not on isolated domains, so sequence end prediction artifacts are restricted to Pfam domains at the N or C-terminus of proteins. When all proteins where end effects may affect prediction are removed, the prevalence of 100% predicted disordered domain members, shown here at 100% and in previous work,Citation33 does not significantly decrease (). The inset shows the distribution of lengths for 100% predicted intrinsically disordered (PID) Pfam sequences. shows the quantiles. The mean length of 100% PID Pfam sequences is 82 residues. The mean length for PID regions in whole mammalian proteins in the Pfam seed set is 16 residues ().
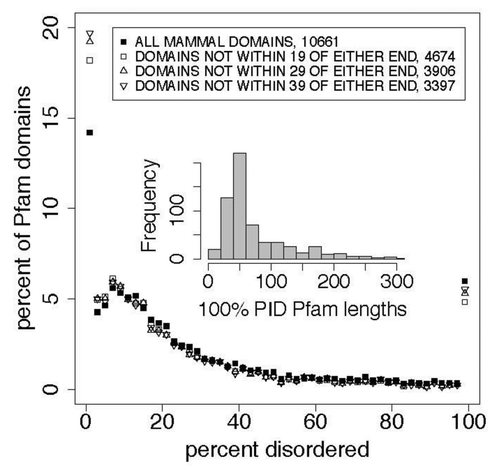
The 10 Kidera factors are listed in . Testing the hypothesis that intrinsic protein disorder preference, like helix preference, is a fundamental physical property of the amino acids that was not included when the Kidera factors were calculated, a singular value decomposition of the 20 by 11 matrix composed of the Kidera factors and the frequencies of occurrence of the amino acids in IDPs yielded only 10 non-zero eigenvalues; the disorder preferences of the amino acids are linear combinations of the Kidera factors.
Of the 618 100% PID members of Pfam domains, 176 start at residues 1 through 19 of the parent protein. The VSL2b predictor is biased to assign disordered structure to the first 20 residues of a protein or isolated segment. We tested the hypothesis that about 176 members may be incorrectly predicted to be 100% disordered and that this error biased our calculations. The results, shown in and , indicate that end effects do not contribute significantly to the 100% PID group.
Table 2. Comparison of distributions for percent predicted disorderCitation1 in mammalian Pfam seed members that are more than 19 or 29 residues from the N and C protein termini ()
The sequence length mean of 100% PID Pfam sequences is 82, the median is just below 50, and includes many above 150. This broad and skewed distribution distorts the cluster analysis performed here to some extent where averages of Kidera factors taken over long sequences tend toward their central value of zero, while in shorter sequences there is a greater chance that a particular type of intrinsic disorder may be isolated, in a way that is analogous to searching for segments of helix, β-strand, or turn in folded structures. Earlier work finding 3 distinct albeit overlapping flavors of disorderCitation52 evaluated amino acid composition in windows 41 residues long. The effects of our use of a homogeneous distribution of lengths here are discussed below where we evaluate the high dimensional analysis of our data.
and show the distributions of PID in several proteomes compared with that in Human Pfam seed proteins. There are more long PID regions in the proteome than in the Pfam seeds for Humans. About 4% of the chimp, mouse, and human proteome whole proteins are 100% PID. Lower phylogenetic domains have smaller proportions of 100% disordered Pfam domains, and of disorder overall, in the following order: mammals > other eukaryota > viruses > bacteria > archaea. The median length of disordered regions in this 100% disordered group is 59 residues. Distributions of disorder in each of the 5 phylogenetic domains have positions that differ at the 0.999 level with p values less than 1 × 10−10 .
Figure 2. In whole proteins there appears to be a predominance of 100% PID over what is found in the Pfam seed database for humans, with more (here) in mouse and chimp than in human proteins. This cannot be attributed to an uneven distribution of Pfam domain or PID lengths, and suggests that the Pfam seed database excludes some IDPs. This is shown here in comparisons of the distribution of percent predicted disorder in the human proteome with the PanTroglodytes chimpanzee and MusMusculus mouse proteomes, and with human proteins chosen as sources for Pfam seed members (red). shows statistics for these distributions. Proteins with 0% disorder are not plotted here. We note that the distribution for mouse is shifted 1% to the left of that for chimp and human proteomes while 100% PID is highest for the mouse.
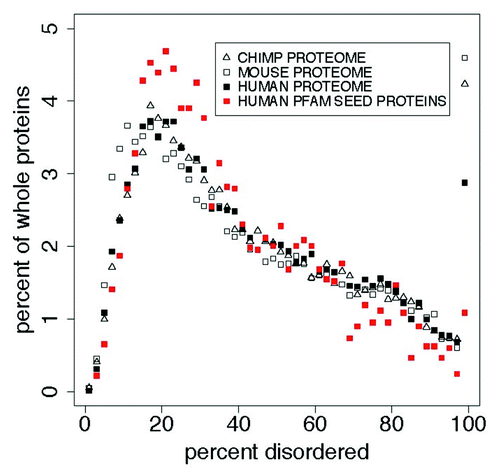
Table 3. Comparison of distributions for percent disorderCitation1 in the proteomes for human, PanTroglodytes chimpanzee, and MusMusculus mouse ()
Overall, 28% of Pfam members with 100% PID are derived from whole proteins that are 100% PID, and Mammalian domains in the Pfam seed database contain 27% PID. This is 13.8% less than in their source proteins and 16% less than in mammalian proteomes. This can be seen, with the additional 3.1%, in and . We hypothesize that this is an artifact of low complexity sequence filtering in the selection of Pfam seeds, by accident or design. The null hypothesis, that each distribution does not differ from any other, can be rejected at the 0.999 level.
, and and , show the distributions of PID for Pfam seed members (regions of proteins) and the whole proteins from which seeds are derived.
Figure 3. Left: A comparison of the distribution of percent PID in Pfam seed domain members and Pfam whole proteins for mammals and other eukaryota in 2% wide bins show 2 things. About 1.7% of whole proteins and 6% of Pfam members are predicted to be 100% PID, but whole proteins contain significantly more PID overall. Again, whole proteins contain 28% of the Pfam sequences predicted to be 100% disordered, and do not fall into the category where uneven length distribution of domains accounts for 100% disordered Pfam domains. Also, predicted disorder is estimated to be 13% lower in Pfam domains than in Pfam whole proteins, shown in , “est dif.” Proteins and domains with 0% disorder are not plotted here. Right: Here, the median length of predicted 100% disordered whole proteins is about 70 residues longer than that of predicted 100% disordered Pfam domains (), and in the median length of Pfam domains is much larger than the median length of predicted intrinsically disordered regions. Clearly, some 100% PID Pfam sequences derive from whole proteins where PID extends beyond the ends of the Pfam segments as proposed earlier,Citation33 but there is no obvious reason why this classifies the significant category of predicted entirely disordered Pfam sequences as an artifact.
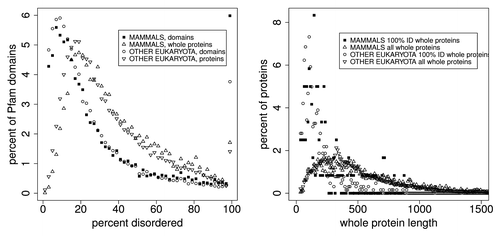
Table 5. Comparison of quantiles and means for the distributions of length in 100% PID: for Pfam seed members and whole proteins,Citation1 also shown in , right, and inset
, and and , show the distributions for percent disorder in Pfam members, Pfam member length, predicted intrinsic disorder (ID) length in whole proteins, and numbers of ID regions in whole proteins. The inset in each plot shows the quartiles for each phylogenetic domain.
Figure 4. Distributions of Pfam seed sequences for mammals, other eukaryota, viruses, bacteria, and archaea, showing, upper left: the percent of Pfam domains as a function of the percentage of predicted disorder in 2% wide bins, and the spike in the number of domains that are 100% disordered (see also ). Upper right: Pfam domain sequence lengths for mammals are 12 to 40 residues shorter than they are in other eukaryota, viruses, bacteria, and archaea, but the order of domain sequence lengths does not follow the order of 100% PID, as also shown in under “shift.” The mean domain length is 145 residues (). Lower left: Intrinsically disordered region (IDR) lengths (distinct from Pfam domain lengths) for mammals are 1–5 residues longer than in other domains/kingdoms of life, also shown in , and the order of ID length follows the order of 100% PID. Most predicted intrinsically disordered regions are shorter than 10 residues, much shorter than the median Pfam domain sequence length. Lower right: there are significantly fewer IDRs in mammals than in viruses, and marginally fewer than in other eukaryota. The median is near 2, and some proteins are predicted to have more than 20 IDRs.
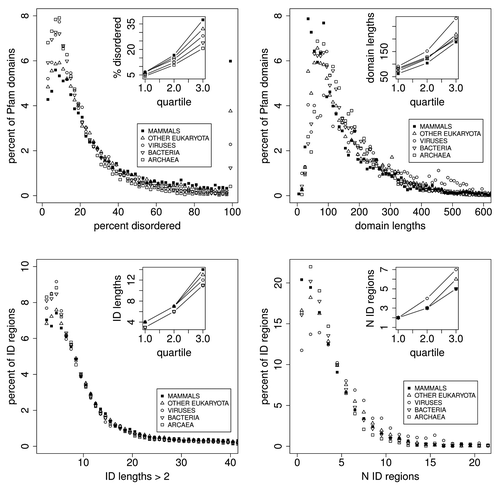
Table 6. Comparison of distributions for percent predicted disorder in Pfam seed membersCitation1 (, upper left) comparing Mammals, Eukaryota, Viruses, Bacteria, and Archaea
Table 7. Comparisons of distributions for Pfam seed member lengthsCitation1 also shown in upper right, for mammals, eukaryota, viruses, bacteria, and archaea, showing that there are small but significant differences, and that the mean length for 100% PID members in mammalian proteins (top row of this table) is 60 residues shorter than that for mammalian Pfam member lengthsCitation2
Most striking is the spike at the right side of top left indicating that there are more Mammalian Pfam members 100% PID than there are in any other 2% wide bin of the data. There are 618 100% PID Mammalian Pfam members in this set. Our initial hypothesis here—that this set of PID sequences was characterized by factors that differed from those in other PID sequences—could not be supported.
Also shown in top right and bottom left, while most PID sequences are shorter than 10 residues, very few Pfam members are this short, and the mean Pfam member length is 145 residues ().
shows that each 100% PID Pfam member clearly derives from a PID region that is longer than the Pfam member. However, there is no compelling evidence here that the presence of entirely disordered Pfam members is an artifact, and does not have a special evolutionary significance, perhaps conferring an advantage to “higher” or “lower” phylogenetic domains. The evidence against artifact is also not especially compelling: the mean length of Pfam domain members, 145 residues, is much longer here than the mean length of PID regions, 16 residues. Pfam member lengths for mammals are 12–40 residues shorter than they are in other eukaryota, viruses, bacteria, and archaea. There appears to be a predominance of 100% PID in the eukaryotic proteome that is also seen in Pfam domain members. The Pfam seed database appears to exclude some IDPs. Predicted disorder is estimated here to be 13% lower in Pfam domains than in Pfam whole proteins. Twenty-eight percent of Pfam members that are 100% PID are derived from whole proteins that are 100% PID. Statistics alone cannot resolve this question.
shows CH/CDF scatter plotsCitation34 for the distributions of predicted disorder in Pfam-A version 23.0 members in mammals (10,660 Pfam members), other eukaryota (71,765), viruses (6,360), bacteria (101,959), and archaea (12,721). The CH/CDF plot shows, for all practical purposes, the VSL2b disorder prediction score on the x axis and hydropathy on the y axis. This can be seen in the scatter plot matrix in the lower right where CH, hydropathy,Citation35 CDF, and the VSL2b scores for mammals are all plotted against each other. CDF and VSL2b are highly correlated, as are CH and hydropathy.
Figure 5. CH/CDF plotsCitation34 for mammals, other eukaryota, viruses, bacteria, and archaea. The scatterplot matrix (bottom right) shows density (number of sequences) as a function of CH, hydrophobicity,Citation35 CDF, and VSL2b,Citation36 while the off-diagonal plots show the correlations between each of these parameters. The off-diagonal plots: (1) corresponding to CH/CDF on the diagonal duplicate the plot for all Pfam seed sequences for Mammals (top left), and (2) corresponding to CH or CDF on the one hand and hydrophobicity or VSL2b on the other, show the high correlation between CH and hydrophobicity, and between CDF and VSL2b. Only 2 or 3 clearly separate clusters are evident here, but the cluster analyses of the Mammalian data, shown in ‒ reveal much more information.
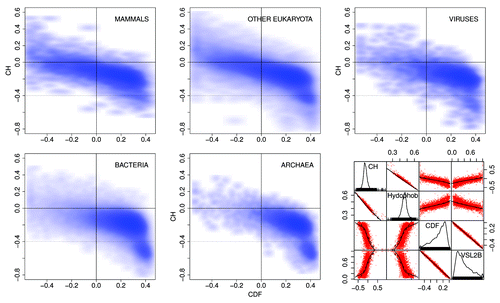
The CH/CDF plots are divided into 6 sections. Density in the lower and middle right hand sections of the CH/CDF plots corresponds to mostly ordered Pfam members, while density in the middle and upper left hand sections represents mostly disordered Pfam members. As can be seen, density shifts from the lower right to the upper left as plots progress from archaea, bacteria, viruses, and other eukaryota, to mammals. The grouping in the lower right hand side of the plots for archaea and bacteria in , corresponding to proteins with no predicted intrinsic disorder, is not included in the cluster analyses below.
shows more detailed structure than can be seen in the . Each plot here contrasts data from all of the phylogenetic domains, and shows the differences between domains more clearly than can be seen in . Here the percent of Pfam members is on the Y axis, and the percent of predicted disorder in each region is on the X axis in 5% wide bins. We note that in each sub-plot the lines for all of the domains tend to cross at 1 point, giving the appearance of an isosbestic point, suggesting that there are at least 2 independent states in each of the 5 sections. A cluster analysis of these sections (not shown) indicates 2 components in each section, with the exception of the “spot” representing ordered proteins. Second, in the upper left quadrant, there are more eukaryota Pfam members in the 95–100% disordered group than in any other 5% wide group.
Figure 6. Pfam sequences plotted in each section of are plotted here as a function of percent disorder in each sequence, correlated with but not the same as the VSL2b parameter. We note that the ordering of phylogenetic domains, with respect to increasing PID, is preserved with the exception of the upper right quadrant where the sample size is too small to be significant. The shifts in PID from 1 domain to the next are more quantitative here, and each “quadrant” appears to represent 2 distinct states.
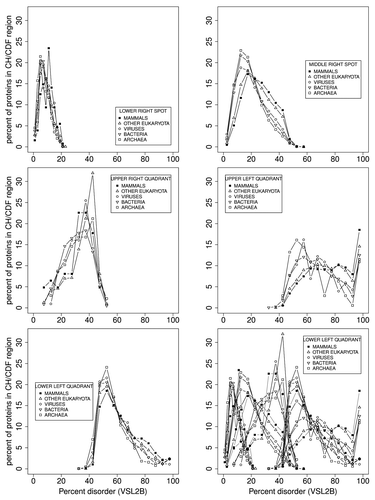
As discussed above, some methods of predicting intrinsically disordered structure are subject to end effects, such that, for VSL2b here, N-and C-terminals are predicted to be disordered when they are possibly not. This problem probably accounts for part of the approximately 20 percent false positive rate observed for some disorder predictors. As can be seen in the top left frame of , corresponding to the lower-right spot in bottom center, in that group of proteins that are the least disordered, only 2–4% are predicted to have no disorder at all. End effects, at least here, partly account for this.
and show structure-function feature maps for 3 examples of the 618 mammalian proteins found here with 100% predicted disordered Pfam members, chosen on the basis of their known involvement in human disease and presented in order of length. The program that generates these figures was written for this work and is now part of the Disprot PONDR predictors available on the Disprot site.Citation40
Figure 7. Structure-function maps of 2 100% PID whole proteins, each dominated by 1 Pfam domain, are shown above with Pfam sequences marked in green. Many similar examples exist where parts of these proteins have been crystallized, but in the presence of SDS or in co-crystals with partner molecules, illustrating the induced conformation nature of IDPs. X-ray crystal structure is marked in black. NMR solution structure is marked in gray. PF05160 DSS1 HUMAN has been co-crystallized in complexes: 1iyj,Citation37 1mje,Citation38 and 1miuCitation39). No pdb evidence of structure yet exists for PF04440 DBND1 HUMAN. These plots of PONDR prediction results are available on the Disprot site.Citation40
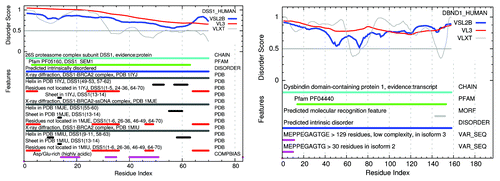
Figure 8. A structure-function map of APC HUMAN shows an example of a protein with a mix of PID and structure with multiple Pfam domains (green), most predicted to be mostly disordered (blue). X-ray crystal structures (black) also include many residues not observed in these structured segments (red). The longest fragment of APC HUMAN to be obtained in large quantities is seen to be 100% disordered in the NMR solution structure (also red). Many of the X-ray structures for APC HUMAN have been co-crystalized with other molecules, suggesting that these conformations are induced by the binding of an IDP with a structured partner.
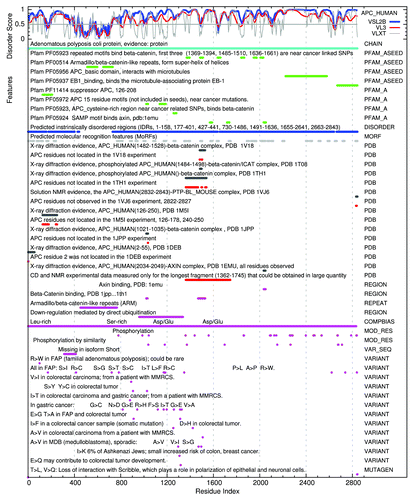
Included in each figure are the IDR prediction profiles from the PONDR VSL2b, VL3, and VXLT methods,Citation4 markers for: Pfam family and domain members, predicted IDRs based on VSL2b, predicted molecular recognition features based on the VXLT profiles, regions of these proteins represented in the protein data bank by X-ray crystal diffraction and NMR evidence both for order and disorder, sites of phosphorylation and methylation, and for sequence variations related to human disease. These are described below with a comparison to other members of the same Pfam family.
PF05160: DSS1 HUMAN plays a role in ubiquitin-dependent proteolysis, interacts with the C-terminal of BRCA2, and is involved in split hand-split foot malformation.Citation87 Other members of this family predicted here to be 100% disordered are DSS1MOUSE-3-63, SEM1-DROME-15-75, and SEM11ARATH-8-70. The following members of the PfamA.seed set predicted to be less disordered are: Q9LIY2ORYSJ-9-97, 86%, SEM1-YEAST-19-84, 67%, and DSS1-SCHPO-2-66, 91%. In a limited sample some non-seed members show even lower amounts of PID, but we cannot say that it is generally true that some family members have widely differing amounts of PID.
PF04440: DBND1 HUMAN-14-153 binds to α- and β-dystrobrevin in muscle and brain, and genetic variation is thought to be associated with Schizophrenia.Citation88 Other members of this family in the seed set: DBND1-MOUSE-14-155, 94% PID, and DBND2HUMAN-100-254, 99% PID.
PF05923: APC HUMAN, Found repeated in the mid region of the adenomatous polyposis proteins (APCs), near many cancer-linked SNPs. These repeats bind β-catenin.Citation89 Most other V23.0 seed members of this family in human are 100% PID: 13691394, 1840-1866, 2006-2031, and 1948-1973, but 1485-1510 is 85% PID and 1636-1661 is 27% PID.
The Pfam V23.0 seed set appears to be accurate with respect to most of the IDPs we have sampled. However, there are, as with all prediction methods, some inconsistencies, and we note that we are applying a prediction to a prediction here.
shows, on the right, a parallel analysis plotCitation41,Citation42 indicating that there are probably 3 independent types of mostly or entirely disordered Pfam members where differences are based only on the averaged physical properties of their amino acids, and VSL2b scores are greater than 0.5. Likewise, on the left, we cannot reject the null hypothesis that there are 5 types of Pfam members in mammals based on the averaged physical characteristics. There are 6 when archaea are included (not shown). These groups are in addition to the HMM classifications.
Figure 9. Retention and rejection in the Glorfeld Principle Component AnalysisCitation41-Citation45 of Pfam sequence information represented by the 10 Kidera factors. Left: 5 components are retained from 10532 mammalian Pfam domains. Six components are retained when archaea are included (not shown). Right: 3 components are retained when the sample is restricted to 1913 members here where the VSL2b parameter is greater than 0.5 (more than half of each member is predicted to be disordered). As can be seen in the figures below, these components form well separated groups with very little overlap.
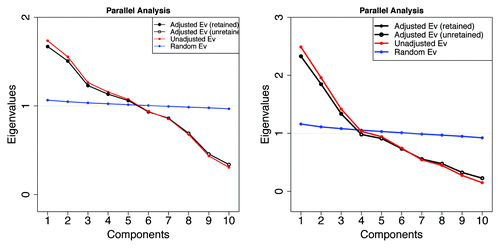
In an earlier study aimed specifically at finding different flavors of intrinsic protein disorder in the Swissprot database, Vucetic et al.Citation52 found 3 groups characterized by their amino acid composition. These groups, composed of predicted intrinsically disordered regions divided into windows 41 residues long, were related to function. It is not possible to compare those groups to the ones seen in this work with respect to physical properties. The question here is: how do the clusters found here relate to Pfam families and function. There is substantial evidence that functional intrinsic disorder, particularly in long sequences, is composed of multiple shorter structural features, such as those found in MoRFs.Citation90,Citation91 To be meaningful, the mapping of types of disorder to function or Pfam family should include a windowing analysis, similar to that performed earlier, of shorter segments that includes the kind of periodic features used to train PONDR in this respect.
shows a pairs graphCitation46 (a scatterplot of all factors against all others) of the mean Kidera factors for Pfam members where the average VSL2b score is greater than 0.5, indicating that the sequence is likely to be more than half disordered. As in , red and green delineate 100% PID members from others that are between 50 and 100% PID. Members that are 100% PID show visually distinct clusters. This is where the VSL2b score, the predictor of intrinsic disorder, is highest. The 100% PID members here appear above the correlation line in the ext vs fle plot, and in the lower left of the dbe vs alp plot. However, these are not particularly special cases; there is some degree of separation in the predictions of partly and completely disordered structure by all pairs of factors, indicating again that all factors contribute to this distinction. Also, in the scatterplot matrix figure below, where the VSL2b score is included and the color distinction is made for clusters and not for degrees of disorder, the VSL2b score does not appear to be well correlated with either color mapping or with the overall trend of the scatter. Another way of viewing the clustering, and non-clustering, of 100% PID Pfam members is shown in the dendrogram plots below.
Figure 10. A pairs graphCitation46 shows scatterplot, regression lines, and typographically scaled absolute values of the correlation coefficients between the mean Kidera factors for the 1913 members where the mean VSL2b parameters are greater than 0.5 (half disordered or more). Points for domains that are 100% PID are red, while all others are green, showing several visually distinct clusters in each of the 2-dimensional plots in a way that is almost impossible with 2-dimensional projections of high-dimensional objects, and the corresponding dendrogram shown in , right, does not reveal the level of structure shown here. Helix/bend (hel) and extended structure preferences (ext and fle) are negatively correlated, and the 2 extended structure preferences are positively correlated, as can be seen also in . This is expected, but other correlations are relatively small. The Kidera factors themselves have zero correlation.
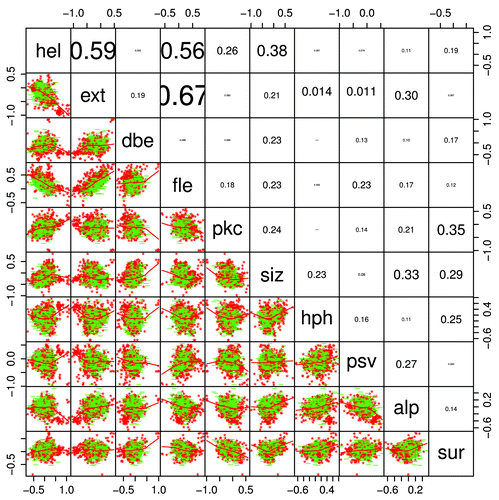
Note that although the Kidera factors themselves have zero correlation, some factors averaged over the entire lengths of Pfam sequences do show substantial correlation, albeit with large variance. There is a positive correlation here between average ext (extended) and fle (flat extended) factors, and a negative correlation between these and the average hel (helix) factors for entire Pfam sequences. These correlations appear again in .
Figure 11. A biplotCitation47 of the data calculated from 10572 Pfam sequences and 10 mean Kidera factors shows a 2-dimensional projection of 3 of the 5 principle components, vectors of equal length representing the Kidera factors (), and 100% PID sequences colored in red. Note that the apparent correlation between hph and sur is caused by the projection into 2 dimensions, and that the actual correlation is 0.25 (). IDPs appear to arise here from a variety of combinations of average factors. These distinctions become even more evident when only shorter Pfam sequences, or windows of uniformly shorter segments, are chosen (not shown). As Pfam sequences become longer, the means of the Kidera factors tend toward the central value, zero, masking the diversity of IDP types in Pfam domains. We anticipate that, for example, a cluster will appear along the hel (helix) or alp (occurrence in α region) axis in an analysis that includes windows of 20 residues each, and that shorter windows may yield a cluster along the dbe (double-bend preference) axis.
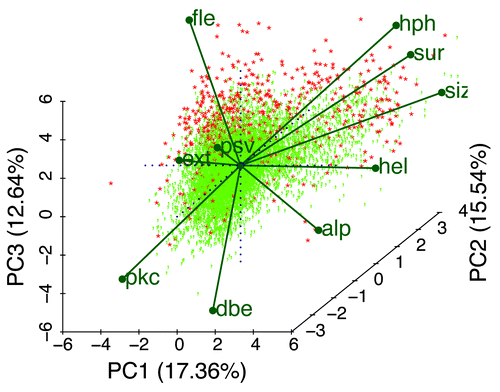
shows a PCA biplot of all Pfam members here scored by the principle components of the 10 mean Kidera factors for the members. Each point represents an identifiable member with eigenvalues or coefficients corresponding to how much each component contributes to the variance in the original data. The 10 labeled vectors are projections of the mean Kidera axes onto the 2-dimensional plot shown here, indicating their contributions, however difficult to see in only 2 dimensions, to the 3 principle components represented here. The positively correlated average extended- and flat-extended factors point away from the average helix factor to which they are negatively correlated, and these are somewhat aligned along the PC1 and (for fle) PC3 axes. Note that 100% PID members, in red, are clustered primarily along the fle axis, but that some occupy positions at the far ends of other factors. All of the Kidera factors appear to contribute to the preference for types of order or disorder.
A better visualization of how all 10 factors contribute to the principle components, particularly when there are more than 3 PCs, can be obtained by rotating the PCA biplot in 2- and 3-dimensional projections in real time using the RGgobi and (for Windows only) BiplotGUI R packages.Citation92,Citation93 Both there, as well as in static plots shown here, individual points can be labeled with their accession numbers to better explore these relationships.
shows a partial least squares and principal component regressionCitation48 of the mean VSL2b score against the 10 mean Kidera factors for all Pfam members included here. Each point on the left represents a Pfam member scaled by 6 eigenvectors of the PCA decomposition. Plots of the regression coefficients on the right show that only 5 components show noticeable differences, so the regression here is essentially in 5 dimensions. The distribution of lengths in Pfam members here is broad, causing the mean values of the Kidera factors to have a limited value, tending toward zero a central value where lengths are long.
Figure 12. A partial least squares and principal component regressionCitation48 of the mean VSL2b factor against the 10 mean Kidera factors for 10572 Pfam sequences, left, shows a prediction of the mean VSL2b factor for Pfam sequences using “leave one out” validation, with 100% PID sequences in red. Here the x axis represents mean VSL2b scores calculated directly from the VSL2b predictions for each sequence (these are just mean VSL2b scores) and the y axis represents predictions from the multivariate linear regression on 6 principle components of the Kidera factors (see Methods). We hypothesize that the nonlinearity and spread of the data here is partly due to errors in the VSL2b predictions themselves, that the Kidera factors more accurately represent the tendency to disorder, and that the spread will narrow in an analysis of smaller uniform windows of sequence. Five components yield the same results, consistent with the results shown in , and here to the right. The plot on the right shows the regression coefficients for these 6 sets of principle components. Here the sets containing 5 and 6 components have nearly the same coefficients, indicating, as was also shown using a different analysis in , that the sixth component contributes little to the information contained in the Kidera factor averages. We hypothesize here that convergence will shift to more coefficients when smaller uniform windows are analyzed.
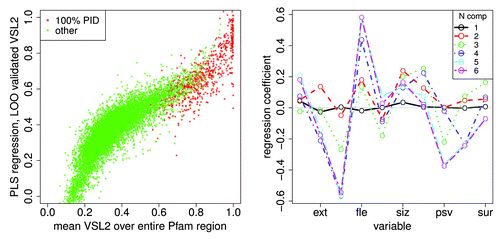
shows dendrogramsCitation49 of the mean Kidera and VSL2b factors for the complete 10532 member set, and for the 1913 member set that includes only members with mean VSL2b scores above 0.5. It appears to be clear that 100% PID members are in distinct groups. Depending on where the y axis line is drawn, it is possible to find between 5 and 7 clusters here. However, in the 1,913 member subset, although we know that there are only 3 distinct clusters (, right), it is difficult to distinguish them here. 100% PID members appear to be distributed randomly among several distinct groups.
Figure 13. The dendrogramCitation49 on the left shows the mean Kidera and VSL2b factors for 10532 Pfam members, with clustered 100% PID members in red. Five clusters are obtained here by drawing a horizontal line crossing six of the vertical markers near y = 2.1. The marker on the far left is an outlier. Vertical lines at the bottom overlap considerably. The dendrogram on the right shows the same mean factors for 1913 Pfam members where the VSL2b mean is above 0.5 and sequences are half PID or more. Three clusters are obtained here by intersecting 3 vertical markers at y = 2.5. The 100% PID members plotted in red are not well clustered, indicating that here we cannot say they are different from those that are half PID. When sequences are chosen to be on the order of 10 residues, distinct clusters do appear (not shown).
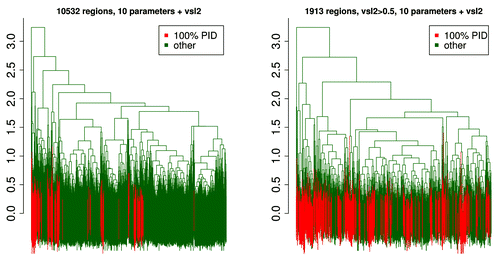
We now turn to a principle component analysis (PCA) of the Kidera factors averaged over entire Pfam sequence lengths, in below where the mean VSL2b scores are excluded, and in where they are included to show an almost complete lack of correlation with any single mean Kidera factor. shows a bivariate cluster plot,Citation50 showing only 2 components, of the 1,913 member subset where 4 components are retained to show the tendency of long members to have the same Kidera factor means, reducing the dimensionality available for discriminating between disorder types. The 4 clusters have the same colors in both the cluster and silhouette plots. While values around 0.2 in silhouette plots are low, indicating weak clustering, these values become larger as longer members are excluded.
Figure 14. Left: A bivariate cluster plotCitation50 of a Partitioning Around Medoids (PAM)Citation51 for k = 4 clusters shows 3 clusters with almost no overlap, and 1 cluster with considerable overlap (black). The observations near zero tend to be from long Pfam sequences where means in the distance matrix tend to the central value. This calculation included the mean VSL2b factors and the 10 mean Kidera factors for 1913 Pfam members where the mean VSL2b factor is greater than 0.5. When k is set to 3, the black and blue points are combined into one group. Again, individual Pfam members can be identified here. Right: A silhouette plot of the same PAM object using the same colors also shows 3 mostly non-overlapping groups and one with significant overlap. Silhouette widths: near 1 indicate well clustered groups, near 0 indicates that observations lay between 2 clusters, and negative means that observations overlap or are in the wrong cluster. Colors, but not numbers, correspond to the same groups in each plot. The same observations with the same colors are also plotted in . We note that overlap in the previous workCitation52 was greater than 70%.
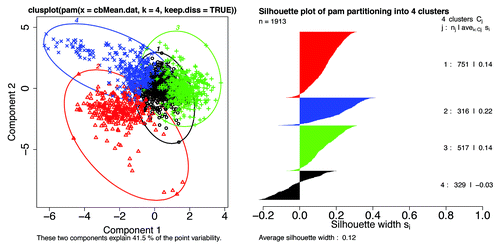
Figure 15. Scatterplot matrix of the mean VSL2b factors and 10 mean Kidera factors for 1913 Pfam members where the mean VSL2b factor is greater than 0.5. Points plotted here have the same colors used in the PAM cluster analysisCitation50 plotted in . The scatter plot shown here for VSL2b (vsl) and hydrophobicity (hph) is essentially the same plot shown in , with the exception that here only the left side of is represented. Note that the cluster identified in red is most prominent where size is plotted against the other parameters, and that it runs parallel to the VSL2b axis, indicating that sequences in this cluster containing large amino acids are present in both ordered and PID Pfam members. The cluster identified in green is most prominent when helix preference is plotted against other parameters, marking sequences with low mean helix preference, and for blue sequences with high mean helix preference.
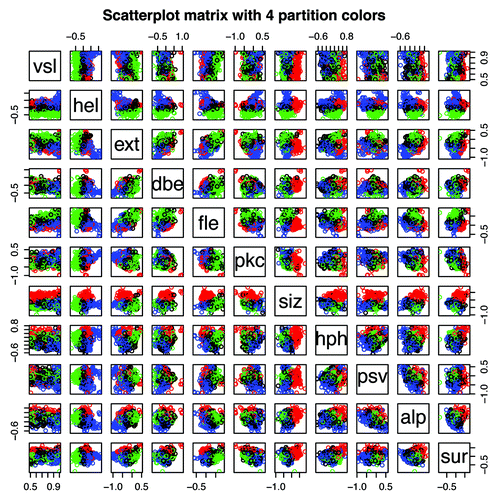
The distribution of residue lengths has a distorting effect on cluster plots. While residue lengths of most PID sequences are less than 10 (4), the mean length of 100% PID Pfam sequences is 82, the median is less than 50, and many are longer than 150 (). These much longer sequences tend to have mean Kidera factors near zero, even though they may contain regions that vary from this and from each other significantly. Presently not well supported by the statistics, both the blue and red clusters on the left () can be divided again to form 5 well separated clusters, and we think these will become more significant as a windowing scheme is imposed on the size of cluster members and the members colored in black become redistributed.
shows a scatterplot matrix for the 1913 member set where mean VSL2b factors greater than 0.5. Members plotted here have the same colors used in the PAM cluster analysisCitation50 plotted in , but no PCA is performed here. Again, these plots take on different characteristics as sequence lengths become more uniform (not shown).
Perhaps the most notable feature of this figure: the cross-plot for vsl and hph, showing the relationship between VSL2b score and hydrophobicity, is essentially the same as is shown in the top left of where CDF is less than zero and CH is between -0.4 and 0.6. Note that the cross plots for vsl against all of the other Kidera factors show a similar spread of data, indicating that hydrophobicity is not the only factor important in predicting intrinsic disorder.
As in , a positive correlation can be seen here between extended and flat extended structure, but here there is also a clear separation of clusters (colors) along the axis of the correlation. Also, the negative correlation between the 2 extended factors and helix shows a separation of clusters.
Note in this figure that amino acid size (siz) and partial specific volume (psv) have very different distributions plotted against predicted disorder (vsl). At least from this perspective, size divides both folded and disordered Pfam sequences along the vsl axis in a very clear way, while volume, one of the factors used in PONDR training, does not appear to discriminate. Likewise, hydrophobicity (hph), while showing more of a separation of the three primary clusters, does not show a direct relationship with vsl. The single Kidera factor that appears to be in the most direct relationship with vsl here is pkc where, on the top line of plots, blue predominates in the upper right and green somewhat in the lower left.
The Kidera factors contain most of what is known about the statistically countable and experimentally measurable physical characteristics of the amino acids, effectively increasing the information content of the sequence by 10-fold. Principle component and cluster analyses of the averages of these factors for each Pfam member, included those that are folded, reveal 6 separate groups. In the subset of Pfam members where the VSL2b disorder predictor is greater than 0.5 at least 3 groups are found, despite the homogeneous distribution of Pfam member lengths.
In the earlier study aimed specifically at finding different flavors of intrinsic protein disorder, Vucetic et al.Citation52 found three groups characterized by their amino acid composition in windows 41 residues long in predicted intrinsically disordered regions in 80,000 sequences of the Swissprot database. It is not possible to compare those groups to the ones seen in this work with respect to physical properties. However, we can observe that the overlap of clusters in the previous work is considerable, while here in the overlap of 1913 sequences is very small but the clustering is weak in the usual interpretation of the silhouette plot.
Where sequences are long the average of the Kidera factors tend toward zero, limiting the capacity of the analyses to discriminate. It is hypothesized that grouping sequences into smaller subsets with more uniform lengths will reveal more information, and lead to more finely structured and perhaps more accurate predictions of intrinsic disorder. Adding additional information derived from sliding window calculations based on the physical properties, related to interactions such as is provided by hydrophobic moment,Citation81 and flexibility,Citation4,Citation80 strengthens predictor training, particularly for features important for molecular recognition.Citation90,Citation91
and show the percent of non-mammal single and multiple cell Eukaryotes, respectively, with unique Pfam members that are 100% PID. It has been shown previously that the average fractions of disordered residues in unicellular and multicellular Eukaryotes are about the same, with unicellular eukaryotes having more scatter (ref. Citation21 and ). We observe here, in a relatively small sample size, that single cell eukaryotes are predicted to have fewer species with 100% intrinsically disordered Pfam domains than multiple cell eukaryota. This may be an artifact of the selection of Pfam seed sequences in these 2 groups, or it may have some evolutionary significance.
Table 8. Percent of single cell eukaryotaa from unique Pfam domains 100% PID
Table 9. Percent of non-mammal multiple cell Eukaryota with unique Pfam membersa 100% PID
Conclusions
A standing hypothesis among IDP investigators proposes that intrinsically disordered protein (IDP) has evolved into different classes that can be identified by physical characteristics or functions, analogous to those identified by HMMs in the Pfam database. We analyze distributions and clusters of PID in 193024 members of the version 23.0 Pfam seed database, representing 12456 unique domains, families, and repeats. Of these sequences, 616 mammalian members associated with 315 biological functions, and 120 of the parent whole protein set, are 100% PID.
Exploring ways to find the maximum information content in intrinsically disordered proteins independent of sequence information used to train HMMs, we applied the 10 linearly independent Kidera factorsCitation1 containing most of the variance in the physical properties of the amino acids to a transformation and analysis of sequence in Pfam training set members. The hypothesis that intrinsic protein disorder exists in multiple forms with preferences analogous to those for protein secondary structure, as is evidenced in the success of MoRF predictorsCitation90,Citation91 is supported by this analysis. There are 3 principle conclusions:
(1) Each of the 10 orthonormal Kidera factors contributes to intrinsic protein disorder. The PONDR predictors used here, while still among the most accurate, included less than 2 of the Kidera factors in training. The inclusion of all 10 Kidera factors could hypothetically increase the information available to a predictor by more than 5-fold.
(2) We identify 3 clearly separate and non-overlapping clusters of intrinsically disordered Mammalian Pfam sequences where the VSL2b score for disorder is not clearly correlated with cluster parameters, and where HMM training or sliding window calculations are not involved. The distributions of length in these Pfam sequences are broad and skewed, so these 3 clusters are groupings of intrinsically disordered Pfam sequences (or families), and not of types of intrinsic disorder. A sliding window analysis limiting sequence size to uniform lengths characteristic of MoRFs and other features important to IDP function could hypothetically increase the number of non-overlapping clusters several fold and reveal a meaningful correlation between disorder cluster type and function. The next step is to train an intrinsic disorder predictor against the Kidera transformed Disprot Database, with the addition of these other methods for recognizing patterns in sequence.
(3) While mammalian proteins in the Pfam seed database contain 40% PID sequence here, only 27% of the Pfam members in this set contain PID regions, suggesting that the Pfam database is missing functionally important PID protein domains.
Methods
The Kidera factors consist of 10 linearly independent vectors derived from an eigenvalue—eigenvector decomposition of statistically and experimentally determined physical properties of the amino acids. The frequencies of occurrence of each amino acid in experimentally known intrinsic disorder, from the Disprot database, can be added to the Kidera matrix to make 11 columns, but a singular value decomposition of this matrix shows only 10 linearly independent vectors; the preference for intrinsic disorder is contained in the 10 Kidera factors. Our 3 letter abbreviations for these factors are shown in .
This analysis included 193024 members of the version 23.0 Pfam seed database,Citation68 representing 12456 unique domains, families, and repeats. The term “members” here refers to individual Pfam domain member sequences and not to entire Pfam domains. The term “domains” here is used for both Pfam domains and phylogenetic domains, but the distinction is spelled out. The term “regions” here refers to parts of proteins, or parts of Pfam members. The term “sequence” here refers to protein in whole or part, and if in part, whether it is isolated or not is spelled out.
Whole protein sequences represented in the Pfam seed set, and the human, mouse, and chimp proteomes were obtained from Uniprot.Citation19
Predictions of intrinsic disorder were performed on 193024 whole proteins using predictors VSL2b,Citation36 VL3,Citation94 and a method that is predictive of molecular recognition features, VLXT.Citation90,Citation91,Citation95 The VSL2b predictor used for the statistical results here compares well with other methods, available on the D2PCitation2 site.Citation32
Statistics, cluster, factor, and principle component analyses, were calculated using R core and contributed packages.Citation41,Citation46-Citation50,Citation92,Citation96,Citation97 The R implementation of the Wilcoxon rank sum test with continuity correction is used to compare distributions, testing the null hypothesis that the distributions of x and y differ by a location shift of “mu.” The alternative is that they differ by some other 1- or 2-sided location shift. Rejection of a null hypothesis in some cases sampled here may have no practical significance if other larger effects are present, such as the possibility that populations of Pfam domains may contain artifacts of HMM training methods, as it appears to be a factor here with respect to low complexity sequences.
Cluster analyses are notoriously difficult to validate. Clusters can appear at random in nature. Confidence intervals on the results of a singular value or eigenvector–eigenvalue decomposition can be calculated when the standard error in the data noise is known because the scale of the original data are maintained through the transformation and eigenvectors can be compared with noise.Citation98,Citation99 Again, even if the level of the noise were known here, other larger effects may render it meaningless.
To obtain some statistical confidence in our results, a parallel analysisCitation41,Citation42 was performed using an eigenvalue-eigenvector decomposition and Glorfelds principle component analysisCitation45 of the 10532-Pfam-member by 10-mean-Kidera-factor matrix. Each Pfam family or domain member was represented by a vector of the means of the 10 Kidera factors listed in over each entire Pfam region. Paran options included 1000 and 5000 iterations, giving the same results at the 99th percentile. A common factor analysis (not shown) retained seven factors from the 10532 sample set. No real difference was seen in the number of eigenvalues retained when VSL2b scores are added to the Kidera set.
A partial least squares and principal component regression, plsr,Citation48 of the mean VSL2b factor against the 10 mean Kidera factors for 10572 Pfam sequences were calculated using the following model: vsl hel + ext + dbe + 〉e + pkc + siz + hph + psv + alp + sur.
Structure and function information extracted from Uniprot database or fasta format files is combined with predictions of intrinsic disorder here to provide graphical representation of the relationships between these features. This program is available on the Disprot database prediction site.Citation100
Acknowledgments
Supported by Department of Defense High Performance Computing Modernization Program project number ODEFN26263101 and USU project C02928.
Disclosure of Potential Conflicts of Interest
No potential conflicts of interest were disclosed.
References
- Kidera A, Konishi Y, Oka M, Ooi T, Scheraga HA. Statistical analysis of the physical properties of the 20 naturally occurring amino acids. J Protein Chem 1985; 4:23 - 55; http://dx.doi.org/10.1007/BF01025492
- Rackovsky S. Quantitative organization of the known protein x-ray structures. I. Methods and short-length-scale results. Proteins 1990; 7:378 - 402; http://dx.doi.org/10.1002/prot.340070409; PMID: 2381907
- Rackovsky S, Scheraga HA. On the information content of protein sequences. J Biomol Struct Dyn 2011; 28:593 - 4, discussion 669-74; http://dx.doi.org/10.1080/073911011010524957; PMID: 21142228
- Romero P, Obradovic Z, Li X, Garner EC, Brown CJ, Dunker AK. Sequence complexity of disordered protein. Proteins 2001; 42:38 - 48; http://dx.doi.org/10.1002/1097-0134(20010101)42:1<38::AID-PROT50>3.0.CO;2-3; PMID: 11093259
- Wright PE, Dyson HJ. Intrinsically unstructured proteins: re-assessing the protein structure-function paradigm. J Mol Biol 1999; 293:321 - 31; http://dx.doi.org/10.1006/jmbi.1999.3110; PMID: 10550212
- Dunker AK, Lawson JD, Brown CJ, Williams RM, Romero P, Oh JS, Oldfield CJ, Campen AM, Ratliff CM, Hipps KW, et al. Intrinsically disordered protein. J Mol Graph Model 2001; 19:26 - 59; http://dx.doi.org/10.1016/S1093-3263(00)00138-8; PMID: 11381529
- Uversky VN, Gillespie JR, Fink AL. Why are “natively unfolded” proteins unstructured under physiologic conditions?. Proteins 2000; 41:415 - 27; http://dx.doi.org/10.1002/1097-0134(20001115)41:3<415::AID-PROT130>3.0.CO;2-7; PMID: 11025552
- Dyson HJ, Wright PE. Intrinsically unstructured proteins and their functions. Nat Rev Mol Cell Biol 2005; 6:197 - 208; http://dx.doi.org/10.1038/nrm1589; PMID: 15738986
- Wright PE, Dyson HJ. Linking folding and binding. Curr Opin Struct Biol 2009; 19:31 - 8; http://dx.doi.org/10.1016/j.sbi.2008.12.003; PMID: 19157855
- Dunker AK, Brown CJ, Lawson JD, Iakoucheva LM, Obradović Z. Intrinsic disorder and protein function. Biochemistry 2002; 41:6573 - 82; http://dx.doi.org/10.1021/bi012159+; PMID: 12022860
- Dunker AK, Silman I, Uversky VN, Sussman JL. Function and structure of inherently disordered proteins. Curr Opin Struct Biol 2008; 18:756 - 64; http://dx.doi.org/10.1016/j.sbi.2008.10.002; PMID: 18952168
- Dunker AK, Cortese MS, Romero P, Iakoucheva LM, Uversky VN. Flexible nets. The roles of intrinsic disorder in protein interaction networks. FEBS J 2005; 272:5129 - 48; http://dx.doi.org/10.1111/j.1742-4658.2005.04948.x; PMID: 16218947
- Oldfield CJ, Meng J, Yang JY, Yang MQ, Uversky VN, Dunker AK. Flexible nets: disorder and induced fit in the associations of p53 and 14-3-3 with their partners. BMC Genomics 2008; 9:Suppl 1 S1; http://dx.doi.org/10.1186/1471-2164-9-S1-S1; PMID: 18366598
- Dosztányi Z, Chen J, Dunker AK, Simon I, Tompa P. Disorder and sequence repeats in hub proteins and their implications for network evolution. J Proteome Res 2006; 5:2985 - 95; http://dx.doi.org/10.1021/pr060171o; PMID: 17081050
- Tompa P. Intrinsically unstructured proteins. Trends Biochem Sci 2002; 27:527 - 33; http://dx.doi.org/10.1016/S0968-0004(02)02169-2; PMID: 12368089
- Tompa P. The interplay between structure and function in intrinsically unstructured proteins. FEBS Lett 2005; 579:3346 - 54; http://dx.doi.org/10.1016/j.febslet.2005.03.072; PMID: 15943980
- Uversky VN, Dunker AK. Understanding protein non-folding. Biochim Biophys Acta 2010; 1804:1231 - 64; http://dx.doi.org/10.1016/j.bbapap.2010.01.017; PMID: 20117254
- Xie H, Vucetic S, Iakoucheva LM, Oldfield CJ, Dunker AK, Uversky VN, Obradovic Z. Functional anthology of intrinsic disorder. 1. Biological processes and functions of proteins with long disordered regions. J Proteome Res 2007; 6:1882 - 98; http://dx.doi.org/10.1021/pr060392u; PMID: 17391014
- UniProt Consortium. Uniprot. Nucleic Acids Res 2007; 35:Database issue D193 - 7; PMID: 17142230
- Ward JJ, Sodhi JS, McGuffin LJ, Buxton BF, Jones DT. Prediction and functional analysis of native disorder in proteins from the three kingdoms of life. J Mol Biol 2004; 337:635 - 45; http://dx.doi.org/10.1016/j.jmb.2004.02.002; PMID: 15019783
- Xue B, Dunker AK, Uversky VN. Orderly order in protein intrinsic disorder distribution: disorder in 3500 proteomes from viruses and the three domains of life. J Biomol Struct Dyn 2012; 30:137 - 49; http://dx.doi.org/10.1080/07391102.2012.675145; PMID: 22702725
- Dunker AK, Obradovic Z, Romero P, Garner EC, Brown CJ. Intrinsic protein disorder in complete genomes. Genome Inform Ser Workshop Genome Inform 2000; 11:161 - 71; PMID: 11700597
- Oldfield CJ, Cheng Y, Cortese MS, Brown CJ, Uversky VN, Dunker AK. Comparing and combining predictors of mostly disordered proteins. Biochemistry 2005; 44:1989 - 2000; http://dx.doi.org/10.1021/bi047993o; PMID: 15697224
- Iakoucheva LM, Brown CJ, Lawson JD, Obradović Z, Dunker AK. Intrinsic disorder in cell-signaling and cancer-associated proteins. J Mol Biol 2002; 323:573 - 84; http://dx.doi.org/10.1016/S0022-2836(02)00969-5; PMID: 12381310
- Sickmeier M, Hamilton JA, LeGall T, Vacic V, Cortese MS, Tantos A, Szabo B, Tompa P, Chen J, Uversky VN, et al. Disprot: the database of disordered proteins. Nucleic Acids Res 2007; 35:Database issue D786 - 93; http://dx.doi.org/10.1093/nar/gkl893; PMID: 17145717
- Vucetic S, Obradovic Z, Vacic V, Radivojac P, Peng K, Iakoucheva LM, Cortese MS, Lawson JD, Brown CJ, Sikes JG, et al. DisProt: a database of protein disorder. Bioinformatics 2005; 21:137 - 40; http://dx.doi.org/10.1093/bioinformatics/bth476; PMID: 15310560
- He B, Wang K, Liu Y, Xue B, Uversky VN, Dunker AK. Predicting intrinsic disorder in proteins: an overview. Cell Res 2009; 19:929 - 49; http://dx.doi.org/10.1038/cr.2009.87; PMID: 19597536
- Ferron F, Longhi S, Canard B, Karlin D. A practical overview of protein disorder prediction methods. Proteins 2006; 65:1 - 14; http://dx.doi.org/10.1002/prot.21075; PMID: 16856179
- Bourhis JM, Canard B, Longhi S. Predicting protein disorder and induced folding: from theoretical principles to practical applications. Curr Protein Pept Sci 2007; 8:135 - 49; http://dx.doi.org/10.2174/138920307780363451; PMID: 17430195
- Dosztányi Z, Sándor M, Tompa P, Simon I. Prediction of protein disorder at the domain level. Curr Protein Pept Sci 2007; 8:161 - 71; http://dx.doi.org/10.2174/138920307780363406; PMID: 17430197
- Dosztányi Z, Tompa P. Prediction of protein disorder. Methods Mol Biol 2008; 426:103 - 15; http://dx.doi.org/10.1007/978-1-60327-058-8_6; PMID: 18542859
- Oates ME, Romero P, Ishida T, Ghalwash M, Mizianty MJ, Xue B, Dosztányi Z, Uversky VN, Obradovic Z, Kurgan L, et al. D²P²: database of disordered protein predictions. Nucleic Acids Res 2013; 41:Database issue D508 - 16; http://d2p2.pro http://dx.doi.org/10.1093/nar/gks1226; PMID: 23203878
- Tompa P, Fuxreiter M, Oldfield CJ, Simon I, Dunker AK, Uversky VN. Close encounters of the third kind: disordered domains and the interactions of proteins. Bioessays 2009; 31:328 - 35; http://dx.doi.org/10.1002/bies.200800151; PMID: 19260013
- Huang F, Oldfield C, Meng J, Hsu WL, Xue B, Uversky VN, Romero P, Dunker AK. Subclassifying disordered proteins by the CH-CDF plot method. Pac Symp Biocomput 2012; •••:128 - 39; PMID: 22174269
- Kyte J, Doolittle RF. A simple method for displaying the hydropathic character of a protein. J Mol Biol 1982; 157:105 - 32; http://dx.doi.org/10.1016/0022-2836(82)90515-0; PMID: 7108955
- Obradovic Z, Peng K, Vucetic S, Radivojac P, Dunker AK. Exploiting heterogeneous sequence properties improves prediction of protein disorder. Proteins 2005; 61:Suppl 7 176 - 82; http://dx.doi.org/10.1002/prot.20735; PMID: 16187360
- Yang H, Jeffrey PD, Miller J, Kinnucan E, Sun Y, Thoma NH, Zheng N, Chen PL, Lee WH, Pavletich NP. BRCA2 function in DNA binding and recombination from a BRCA2-DSS1-ssDNA structure. Science 2002; 297:1837 - 48; http://dx.doi.org/10.1126/science.297.5588.1837; PMID: 12228710
- Yang H, Jeffrey PD, Miller J, Kinnucan E, Sun Y, Thoma NH, Zheng N, Chen PL, Lee WH, Pavletich NP. BRCA2 function in DNA binding and recombination from a BRCA2-DSS1-ssDNA structure. Science 2002; 297:1837 - 48; http://dx.doi.org/10.1126/science.297.5588.1837; PMID: 12228710
- Yang H, Jeffrey PD, Miller J, Kinnucan E, Sun Y, Thoma NH, Zheng N, Chen PL, Lee WH, Pavletich NP. BRCA2 function in DNA binding and recombination from a BRCA2-DSS1-ssDNA structure. Science 2002; 297:1837 - 48; http://dx.doi.org/10.1126/science.297.5588.1837; PMID: 12228710
- The PONDR and PONDR-FIT predictors, http://www. disprot.org/metapredictor.php.
- Dinno A. paran: Horn’s Test of Principal Components/Factors, r package version 1.5.1 (2012). URL http://CRAN.R-project.org/package=paran
- Dinno A. Gently clarifying the application of Horns parallel analysis to principal component analysis versus factor analysis, http://doyenne.com/Software/files/PA_ for_PCA_vs_FA.pdf, unpublished manuscript (September 2012).
- Dinno A. Exploring the sensitivity of Horns Parallel Analysis to the distributional form of simulated data. Multivariate Behav Res 2009; 44:362 - 88; http://dx.doi.org/10.1080/00273170902938969; PMID: 20234802
- Horn JL. A rationale and a test for the number of factors in factor analysis. Psychometrika 1965; 30:179 - 85; http://dx.doi.org/10.1007/BF02289447; PMID: 14306381
- Glorfeld LW. An improvement on horns parallel analysis methodology for selecting the correct number of factors to retain. Educ Psychol Meas 1995; 55:377 - 93; http://dx.doi.org/10.1177/0013164495055003002
- R Core Team. R: A Language and Environment for Statistical Computing, R Foundation for Statistical Computing, Vienna, Austria, ISBN 3-900051-07-0 (2012). URL http://www.R-project.org/
- Faria JC, Demetrio CGB. bpca: Biplot of Multivariate Data Based on Principal Components Analysis., UESC and ESALQ, Ilheus, Bahia, Brasil and Piracicaba, Sao Paulo, Brasil (2012).
- Mevik B-H, Wehrens R, Liland KH. pls: Partial Least Squares and Principal Component regression, r package version 2.3-0 (2011). URL http://CRAN.R-project.org/package=pls
- Witten DM, Tibshirani R. sparcl: Perform sparse hierarchical clustering and sparse k-means clustering, r package version 1.0.3 (2013). URL http://CRAN.R-project.org/package=sparcl
- M. Maechler, P. Rousseeuw, A. Struyf, M. Hubert, K. Hornik, cluster: Cluster Analysis Basics and Extensions, r package version 1.14.3 — For new features, see the ’Changelog’ file (in the package source) (2012).
- Kaufman L, Rousseeuw PJ. Finding Groups in Data: An Introduction to Cluster Analysis, Wiley Series in Probability and Statistics, John Wiley and sons, Hoboken, New Jersey, 1990, 2005.
- Vucetic S, Brown CJ, Dunker AK, Obradovic Z. Flavors of protein disorder. Proteins 2003; 52:573 - 84; http://dx.doi.org/10.1002/prot.10437; PMID: 12910457
- Berman HM, Battistuz T, Bhat TN, Bluhm WF, Bourne PE, Burkhardt K, Feng Z, Gilliland GL, Iype L, Jain S, et al. The protein data bank. Acta Crystallogr D Biol Crystallogr 2002; 58:899 - 907; http://dx.doi.org/10.1107/S0907444902003451; PMID: 12037327
- Le Gall T, Romero PR, Cortese MS, Uversky VN, Dunker AK. Intrinsic disorder in the Protein Data Bank. J Biomol Struct Dyn 2007; 24:303 - 428; PMID: 17206847
- Radivojac P, Obradovic Z, Smith DK, Zhu G, Vucetic S, Brown CJ, Lawson JD, Dunker AK. Protein flexibility and intrinsic disorder. Protein Sci 2004; 13:71 - 80; http://dx.doi.org/10.1110/ps.03128904; PMID: 14691223
- Sedzik J, Kirschner DA. Is myelin basic protein crystallizable?. Neurochem Res 1992; 17:157 - 66; http://dx.doi.org/10.1007/BF00966794; PMID: 1371603
- Oldfield CJ, Xue B, Van YY, Ulrich EL, Markley JL, Uversky VN, Dunker AK. Utilization of protein intrinsic disorder knowledge in structural proteomics. Biochim Biophys Acta 2013; 1834:487 - 98; http://dx.doi.org/10.1016/j.bbapap.2012.12.003; PMID: 23232152
- Tompa P, Fuxreiter M. Fuzzy complexes: polymorphism and structural disorder in protein-protein interactions. Trends Biochem Sci 2008; 33:2 - 8; http://dx.doi.org/10.1016/j.tibs.2007.10.003; PMID: 18054235
- Dyson HJ, Wright PE. Unfolded proteins and protein folding studied by NMR. Chem Rev 2004; 104:3607 - 22; http://dx.doi.org/10.1021/cr030403s; PMID: 15303830
- Dyson HJ, Wright PE. Elucidation of the protein folding landscape by NMR. Methods Enzymol 2005; 394:299 - 321; http://dx.doi.org/10.1016/S0076-6879(05)94011-1; PMID: 15808225
- Daughdrill GW, Pielak GJ, Uversky VN, Cortese MS, Dunker AK. Chapter 8. natively disordered proteins, in: J. Buchner, T. Kiefhaber (Eds.), Protein Folding Handbook, Wiley-VCH, Verlag GmbH & Co. KGaA, Weinheim, Germany, 2005, pp. 271–353.
- Eliezer D. Biophysical characterization of intrinsically disordered proteins. Curr Opin Struct Biol 2009; 19:23 - 30; http://dx.doi.org/10.1016/j.sbi.2008.12.004; PMID: 19162471
- Iakoucheva LM, Kimzey AL, Masselon CD, Smith RD, Dunker AK, Ackerman EJ. Aberrant mobility phenomena of the DNA repair protein XPA. Protein Sci 2001; 10:1353 - 62; http://dx.doi.org/10.1110/ps.40101; PMID: 11420437
- Jensen MR, Salmon L, Nodet G, Blackledge MJ. Defining conformational ensembles of intrinsically disordered and partially folded proteins directly from chemical shifts. J Am Chem Soc 2010; 132:1270 - 2; http://dx.doi.org/10.1021/ja909973n; PMID: 20063887
- Longhi S, Uversky VN, eds. Instrumental Analysis of Intrinsically Disordered Proteins: Assessing Structure and Conformation, John Wiley & Sons, Inc., Hoboken, NJ, 2010.
- Uversky VN, Dunker AK, eds. Experimental Tools for the Analysis of Intrinsically Disordered Protein: Vol. II, Humana Press, Totowa, NJ, 2012.
- V. N. Uversky, A. K. Dunker, A multiparametric analysis of intrinsically disordered proteins: Looking at intrinsic disorder through compound eyes, Analytical Chemistry. 84 (5) 20962104 84 (2012) 2096–2104.
- Finn RD, Mistry J, Tate J, Coggill P, Heger A, Pollington JE, Gavin OL, Gunasekaran P, Ceric G, Forslund K, et al. The Pfam protein families database. Nucleic Acids Res 2010; 38:Database issue D211 - 22; http://dx.doi.org/10.1093/nar/gkp985; PMID: 19920124
- Tompa P, Fuxreiter M, Oldfield CJ, Simon I, Dunker AK, Uversky VN. Close encounters of the third kind: disordered domains and the interactions of proteins. Bioessays 2009; 31:328 - 35; http://dx.doi.org/10.1002/bies.200800151; PMID: 19260013
- Chen JW, Romero P, Uversky VN, Dunker AK. Conservation of intrinsic disorder in protein domains and families: I. A database of conserved predicted disordered regions. J Proteome Res 2006; 5:879 - 87; http://dx.doi.org/10.1021/pr060048x; PMID: 16602695
- Chen JW, Romero P, Uversky VN, Dunker AK. Conservation of intrinsic disorder in protein domains and families: II. functions of conserved disorder. J Proteome Res 2006; 5:888 - 98; http://dx.doi.org/10.1021/pr060049p; PMID: 16602696
- Rackovsky S. Beyond supersecondary structure: the global properties of protein sequences. Methods Mol Biol 2013; 932:107 - 14; http://dx.doi.org/10.1007/978-1-62703-065-6_7; PMID: 22987349
- Solis AD, Rackovsky SR. Fold homology detection using sequence fragment composition profiles of proteins. Proteins 2010; 78:2745 - 56; http://dx.doi.org/10.1002/prot.22788; PMID: 20635424
- Rackovsky S. Global characteristics of protein sequences and their implications. Proc Natl Acad Sci U S A 2010; 107:8623 - 6; http://dx.doi.org/10.1073/pnas.1001299107; PMID: 20421501
- Rackovsky S. Sequence physical properties encode the global organization of protein structure space. Proc Natl Acad Sci U S A 2009; 106:14345 - 8; http://dx.doi.org/10.1073/pnas.0903433106; PMID: 19706520
- Kuznetsov IB, Rackovsky S. CFP: a web-server for constructing sequence-based protein conformational flexibility profiles. Bioinformation 2009; 4:176 - 8; http://dx.doi.org/10.6026/97320630004176; PMID: 20461153
- Rose GD. Prediction of chain turns in globular proteins on a hydrophobic basis. Nature 1978; 272:586 - 90; http://dx.doi.org/10.1038/272586a0; PMID: 643051
- Janin J. Surface and inside volumes in globular proteins. Nature 1979; 277:491 - 2; http://dx.doi.org/10.1038/277491a0; PMID: 763335
- Xie Q, Arnold GE, Romero P, Obradovic Z, Garner E, Dunker AK. The Sequence Attribute Method for Determining Relationships Between Sequence and Protein Disorder. Genome Inform Ser Workshop Genome Inform 1998; 9:193 - 200; PMID: 11072335
- Vihinen M, Torkkila E, Riikonen P. Accuracy of protein flexibility predictions. Proteins 1994; 19:141 - 9; http://dx.doi.org/10.1002/prot.340190207; PMID: 8090708
- Eisenberg D, Weiss RM, Terwilliger TC. The hydrophobic moment detects periodicity in protein hydrophobicity. Proc Natl Acad Sci U S A 1984; 81:140 - 4; http://dx.doi.org/10.1073/pnas.81.1.140; PMID: 6582470
- Eisenberg D, Weiss RM, Terwilliger TC. The helical hydrophobic moment: a measure of the amphiphilicity of a helix. Nature 1982; 299:371 - 4; http://dx.doi.org/10.1038/299371a0; PMID: 7110359
- Nirenberg M, Leder P, Bernfield M, Brimacombe R, Trupin J, Rottman F, O’Neal C. RNA codewords and protein synthesis, VII. On the general nature of the RNA code. Proc Natl Acad Sci U S A 1965; 53:1161 - 8; http://dx.doi.org/10.1073/pnas.53.5.1161; PMID: 5330357
- Romero P, Obradovic Z, Dunker AK. Folding minimal sequences: the lower bound for sequence complexity of globular proteins. FEBS Lett 1999; 462:363 - 7; http://dx.doi.org/10.1016/S0014-5793(99)01557-4; PMID: 10622726
- Xue B, Dunbrack RL, Williams RW, Dunker AK, Uversky VN. PONDR-FIT: a meta-predictor of intrinsically disordered amino acids. Biochim Biophys Acta 2010; 1804:996 - 1010; http://dx.doi.org/10.1016/j.bbapap.2010.01.011; PMID: 20100603
- Peng ZL, Kurgan L. Comprehensive comparative assessment of in-silico predictors of disordered regions. Curr Protein Pept Sci 2012; 13:6 - 18; http://dx.doi.org/10.2174/138920312799277938; PMID: 22044149
- Crackower MA, Scherer SW, Rommens JM, Hui CC, Poorkaj P, Soder S, Cobben JM, Hudgins L, Evans JP, Tsui LC. Characterization of the split hand/split foot malformation locus SHFM1 at 7q21.3-q22.1 and analysis of a candidate gene for its expression during limb development. Hum Mol Genet 1996; 5:571 - 9; http://dx.doi.org/10.1093/hmg/5.5.571; PMID: 8733122
- Straub RE, Jiang Y, MacLean CJ, Ma Y, Webb BT, Myakishev MV, Harris-Kerr C, Wormley B, Sadek H, Kadambi B, et al. Genetic variation in the 6p22.3 gene DTNBP1, the human ortholog of the mouse dysbindin gene, is associated with schizophrenia. Am J Hum Genet 2002; 71:337 - 48; http://dx.doi.org/10.1086/341750; PMID: 12098102
- Nakagawa H, Murata Y, Koyama K, Fujiyama A, Miyoshi Y, Monden M, Akiyama T, Nakamura Y. Identification of a brain-specific APC homologue, APCL, and its interaction with beta-catenin. Cancer Res 1998; 58:5176 - 81; PMID: 9823329
- Disfani FM, Hsu WL, Mizianty MJ, Oldfield CJ, Xue B, Dunker AK, Uversky VN, Kurgan L. MoRFpred, a computational tool for sequence-based prediction and characterization of short disorder-to-order transitioning binding regions in proteins. Bioinformatics 2012; 28:i75 - 83; http://dx.doi.org/10.1093/bioinformatics/bts209; PMID: 22689782
- Uversky VN, Shah SP, Gritsyna Y, Hitchcock-DeGregori SE, Kostyukova AS. Systematic analysis of tropomodulin/tropomyosin interactions uncovers fine-tuned binding specificity of intrinsically disordered proteins. J Mol Recognit 2011; 24:647 - 55; http://dx.doi.org/10.1002/jmr.1093; PMID: 21584876
- Lang DT, Swayne D, Wickham H, Lawrence M. rggobi: Interface between R and GGobi, r package version 2.1.19 (2012). URL http://CRAN.R-project.org/package=rggobi
- la Grange A, le Roux N, Gardner-Lubbe S. Biplotgui: Interactive biplots in r. J Stat Softw 2009; 30:1 - 37; http://www.jstatsoft.org/v30/i12 PMID: 21666874
- Obradovic Z, Peng K, Vucetic S, Radivojac P, Brown CJ, Dunker AK. Predicting intrinsic disorder from amino acid sequence. Proteins 2003; 53:Suppl 6 566 - 72; http://dx.doi.org/10.1002/prot.10532; PMID: 14579347
- Goh GK, Dunker AK, Uversky VN. Protein intrinsic disorder and influenza virulence: the 1918 H1N1 and H5N1 viruses. Virol J 2009; 6:69; http://dx.doi.org/10.1186/1743-422X-6-69; PMID: 19493338
- Buckner J, Seligman M, Wilson J. gputools: A few GPU enabled functions, r package version 0.26 (2011). URL http://CRAN.R-project.org/package=gputools
- Swayne DF, Buja A, Temple Lang D. Exploratory visual analysis of graphs in GGobi, in: J. Antoch (Ed.), CompStat: Proceedings in Computational Statistics, 16th Symposium, Physica-Verlag, 2004.
- Lawson CL, Hanson RJ. Solving Least Squares Problems, Classics in Applied Mathematics, SIAM, 1995, http://dx.doi.org/10.1137/1.9781611971217.
- Williams RW. Estimation of protein secondary structure from the laser Raman amide I spectrum. J Mol Biol 1983; 166:581 - 603; http://dx.doi.org/10.1016/S0022-2836(83)80285-X; PMID: 6864791
- Protein disorder predictors, http://www.disprot.org/ predictors.php.