Abstract
One hypothesis to explain how mutations in the same nuclear envelope proteins yield pathologies focused in distinct tissues is that as yet unidentified tissue-specific partners mediate the disease pathologies. The nuclear envelope proteome was recently determined from leukocytes and muscle. Here the same methodology is applied to liver and a direct comparison of the liver, muscle and leukocyte data sets is presented. At least 74 novel transmembrane proteins identified in these studies have been directly confirmed at the nuclear envelope. Within this set, RT-PCR, western blot and staining of tissue cryosections confirms that the protein complement of the nuclear envelope is clearly distinct from one tissue to another. Bioinformatics reveals similar divergence between tissues across the larger data sets. For proteins acting in complexes according to interactome data, the whole complex often exhibited the same tissue-specificity. Other tissue-specific nuclear envelope proteins identified were known proteins with functions in signaling and gene regulation. The high tissue specificity in the nuclear envelope likely underlies the complex disease pathologies and argues that all organelle proteomes warrant re-examination in multiple tissues.
Introduction
The nuclear envelope (NE) is a double membrane system consisting of the nuclear lamina, inner and outer nuclear membranes and nuclear pore complexes.Citation1 Though historically viewed as little more than a barrier and gatekeeper, recent years have linked NE proteins to functions as disparate as DNA damage repair,Citation2 cell cycle regulation,Citation3 and cell mobility.Citation4 This range of functions is enabled because NE transmembrane proteins (NETs) in the outer membrane connect to the cytoskeleton and NETs/ lamins in the inner membrane interact with chromatin and gene regulatory proteins. Mutations in lamins and NETs, often collectively referred to as the lamina, have been linked to distinct diseases that each exhibit tissue-specific pathologies ranging from muscular dystrophies to neuropathy, dermopathy, lipodystrophy, bone disorders and progeroid aging syndromes.Citation1,Citation5 As the proteins mutated in these disorders are all widely expressed it has been proposed that as yet unidentified tissue-specific partners might mediate the tissue preferences in pathology.Citation1,Citation6,Citation7
Cellular organelles, in general, are thought to be relatively invariant in their integral protein composition, with the exception of the complement of tissue-specific proteins being synthesized in the ER and functioning at the plasma membrane. The first indication that this may not be the case was a proteomic observation that mitochondria isolated from four different mouse tissues exhibited differences in their protein complement.Citation8 Despite the significance of this finding, other organelles have not been similarly analyzed for such tissue specificity. Two recent proteomic determinations of blood leukocyte and muscle NE proteomes using identical methodologies each identified some novel proteins previously not reported at the NE.Citation9,Citation10 Some differences could be due to tissue- and cell-type specificity while others could reflect differences in the methodologies used from earlier studies. Here, we have employed the same methodology used for the blood leukocyte and muscle NEs to determine the liver NE proteome so that all three tissues could be directly compared.
Comparing these NE proteomes, we find surprisingly few proteins common to all three tissues. Tissue differences determined by direct testing of a subset of confirmed NETs by antibody staining of tissue cryosections, tissue western blot and tissue RT-PCR reflected the tissue differences indicated by the proteome data set comparison. Proteomic tissue specificity in the larger data sets also correlated with expression data from a large-scale transcriptome study. Furthermore, comparison of the proteome data with interactome data revealed that proteins indicated to be in complexes often segregated together into particular tissues. Among the subset of proteins with known functions identified here at the NE, gene ontology (GO) functional assignments suggest that the observed tissue differences in NE composition contribute to signaling and gene regulation. It is reasonable to speculate that many among the hundreds of previously uncharacterized proteins identified in the NE will have similarly important tissue-specific contributions.
Results
Nuclear envelope proteomics
Many novel NE proteins were identified in two recent proteomic analyses of rat muscle and human peripheral blood leukocytesCitation9,Citation10 that were not identified in earlier studies of rat liverCitation11 or mouse neuronal tissue culture cells.Citation12 This finding could indicate that the NE proteome differs significantly between tissues or could reflect a combination of moderate tissue differences together with improvements in the mass spectrometry approaches used and/or a tendency for different contaminants to co-fractionate in a particular tissue. To distinguish these possibilities a new analysis of rat liver NEs was engaged that used the same approaches as the recent muscle and leukocyte studies.
Nuclei were first isolated from other cellular organelles by floating contaminating membranes on sucrose, then chromatin was digested and nuclear contents extracted with salt washes and removed by floating on sucrose to generate crude NEs (). These were further purified by alkali or detergent extraction prior to mass spectrometry because isolated NEs at this stage clearly have some chromatin attached and some contamination from other cellular organelles as determined by electron microscopy (−D). Nonetheless, even at this stage, the fractionation had effectively separated the NEs from most expected contaminants as judged by the fact that the ER marker calreticulin and the mitochondrial marker porin were undetectable by Western ().
Figure 1. Liver NE preparations. (A) NEs were isolated from rat liver by first dounce homogenization of the tissue to release nuclei, followed by separation of many contaminating membranes on sucrose gradients and finally digestion and washing away chromatin. These were further extracted with salt and detergent or NaOH to enrich respectively for proteins associated with the insoluble lamin polymer or proteins embedded in the membrane. (B-D) Electron micrographs of NE preparations. Arrowheads point to places where NPCs are inserted in the membrane. Note that images are taken at the step prior to extraction as no discernible structure was left after NaOH or salt and detergent extraction. Thus much cleaner NEs were subject to mass spectrometry analysis. Scale bar 200 nm. (B) Double membrane from particularly clean NE. (C) Most NEs had still chromatin connected. (D) Contaminants were also observed stuck to NEs such as the fragmented mitochondria shown. (E-F) NEs from the pre-extraction stage were analyzed by western blot for expected contaminants. (E) A microsome fraction was separately isolated from the post-nuclear supernatant and similar amounts of total protein loaded. NE proteins lamin A/C and LAP2β were not present in the microsome fraction while the ER marker calreticulin was highly enriched in the microsome fraction compared with the NE fraction. As the ONM is continuous with the ER, the low calreticulin signal in the NE fraction is expected. (F) A mitochondria fraction was isolated by pelleting at 11,000 x g from the post-nuclear supernatant. The mitochondrial marker porin was undetectable in NEs at the level of sensitivity of the LICOR for fluorescence detection, even when 20x more NEs were loaded.
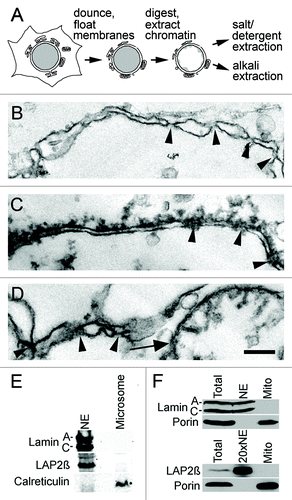
The reason that the ER would be expected to provide the principal transmembrane protein contaminants is because the ER membrane is continuous with the outer nuclear membrane.Citation13 Therefore, some ER proteins will normally reside also in the outer nuclear membrane or, viewed conversely, some NETs likely double as ER proteins. This has already been demonstrated for the NET emerin, which concentrates both in the inner nuclear membrane and in the peripheral ER where it functions in connecting the centrosome to the outer nuclear membrane.Citation14 To better distinguish NE-specific proteins a separate microsomal membrane fractionCitation15 was prepared from the tissue for comparison. NETs enriched in the NE data sets over the microsome data sets could be considered as higher probability candidate NE proteins, though other NETs could still be important because it is estimated that ~40% of proteins occupy multiple cellular compartments.Citation16 Thus NE proteins identified were considered in total or as enriched in NEs > 5-fold over microsomes based on normalized spectral counts.Citation17,Citation18
These NEs and microsomes were then extracted with alkali or detergent treatments to remove many of these presumed contaminants prior to mass spectrometry analysis (). One aliquot of NEs was extracted with 0.1 M NaOH because this breaks most protein-protein interactions without solubilizing membranes and so enriches for transmembrane proteins. Another aliquot was extracted with 500 mM NaCl/1% β-octylglucoside because the detergent will draw the membrane lipids into micelles without perturbing the intermediate filament lamin polymer and so enriches for proteins tightly associated with the lamin polymer. Both aliquots were separately analyzed because some well-characterized NETs distribute to one or the other fraction.Citation11,Citation12 Each extracted fraction was digested with trypsin and soluble peptides were equally divided for use in 4 direct replicate multidimensional protein identification technology (MudPIT)Citation19,Citation20 runs. Remaining insoluble material was further digested with proteinase K at high pH and split for an additional two runs (Fig. S1; Table S1).
The use of both different extraction and different digestion conditions increased the total number of NETs identified. The separate analysis of salt/ detergent extracted NEs resulted in the recovery of 34 additional transmembrane proteins over the NaOH extracted NEs (a 6% increase) using the NE-enriched data set (, top Venn diagram). A similar increase in identified NETs was obtained (5.6%) when including NETs also < 5-fold enriched over microsomes. The sequential digests (trypsin, then proteinase K) were engaged because the NE lamina is defined largely by its insolubility, consisting of both intermediate filament and transmembrane proteins. Thus it was anticipated that associated proteins would be missed that were impervious to the trypsin digestion due to hydrophobic aggregation. This anticipation was justified because 62 additional transmembrane proteins (an 11.5% increase) were uniquely identified in the proteinase K fraction and not in the trypsin fraction using the NE-enriched data set (, bottom Venn diagram). This approach would thus likely benefit other proteomic analyses of transmembrane proteins.
Figure 2. Comparison of replicate MudPIT runs. (A) Both the process of separately analyzing alkali and salt/ detergent extracted NEs and the sequential protease digestions increased the recovery of proteins, particularly NETs. Proportional venn diagrams are shown for the transmembrane proteins identified in all the NaOH or salt/detergent extracted samples and for those identified in all trypsin alone or trypsin followed by proteinase K runs. (B) The same complex sample equally divided yields differences in the identifications for each MudPIT run. However, as the number of runs increases fewer new unique proteins are identified such that the curve plateaus with roughly five replicates. In this experiment liver NEs extracted with 400 mM NaCl, 1% β-octylglucoside were digested with trypsin, insoluble material pelleted and digested with PK. The trypsin sample was split into four equal samples and the PK material into two equal samples and all six samples were separately run on the mass spectrometer.
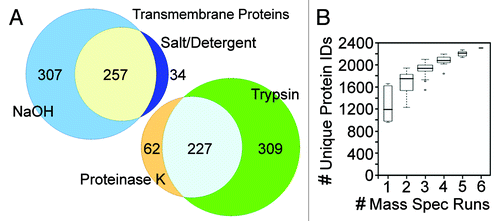
Similarly, engaging multiple replicate runs enabled more comprehensive identification of all proteins in the fractions. The total number of proteins identified from six salt/detergent extracted NE runs was nearly 50% higher than the number identified in any individual run (). As run number increased the number of new identifications dropped, reaching a plateau by ~5 runs where it could be estimated that roughly all proteins in the fractions had been identified. Whereas the earlier liver study that engaged only one run for each extracted fraction identified 1,150 proteins,Citation11 this new liver study identified 2,921 proteins (Table S2).
The finding that so many replicate runs were required for new identifications to reach a plateau is an important observation because it indicates the need for many replicate mass spectrometry runs when investigating complex fractions. The blood leukocyte and muscle studies had respectively engaged 5 and 7 MudPIT runs using the same digestion and run methodology,Citation9,Citation10 so that all three analyses should be fairly comprehensive and could be compared on equal footing. In the case of the blood leukocyte data sets, 5 MudPIT runs were performed for unstimulated leukocytes and an additional 5 MudPIT runs were separately performed for PHA-activated leukocytes, finding some differences between the two statesCitation9; so for purposes of comparison just the PHA-stimulated data sets are used while total NET tallies include both. Before comparing the new liver NE data sets with the blood leukocyte and muscle NE data sets, redundancy due to differences in annotation was removed by converting protein IDs from all 30 MudPIT runs to orthologous gene groups. This yielded 5,222 proteins in total identified among unstimulated and activated leukocyte, muscle and liver NE fractions and 1,037 NETs (Table S3). Proteins were ranked by abundance estimates based on normalized spectral countsCitation17,Citation18 and compared with microsomes to generate the NE-enriched data set containing 4,077 proteins and 598 NETs.
Nuclear envelope tissue specificity
Considerable differences were observed in the total protein complement of the NE when comparing the three data sets. First, proteins were plotted as heat maps using a color coding based on the abundance estimate for a particular tissue using normalized spectral countsCitation17 across all tissues (). Many proteins were uniquely identified in a single tissue. Furthermore, differences in abundance were often observed for those identified in multiple tissues. Though some of those identified in all tissues were highly abundant, surprisingly, quite a few of the tissue-specific NE proteins were more abundant than ones that were ubiquitously expressed. Second, tissue-specific NETs tended to be less conserved than those widely expressed. Analysis of the evolutionary conservation of NETs revealed a statistical correlation such that the more tissue-specific the NET the less it was conserved (). The subset of tissue-specific proteins thus is more likely to have evolved more specific functions. Third, tissue specificity of protein identifications correlated with tissue-specific expression data. NE proteins identified in the various tissues were checked for expression against the high-throughput BioGPS transcriptome database that compared gene expression levels between 80 different human tissues.Citation21,Citation22 Proteins color-coded by their proteomic identification in human blood leukocytes (red), rat muscle (yellow) or rat liver (blue) and the various combinations were plotted according to the tissue preference for their transcript expression in whole human blood (x-axis), human liver (y-axis) or human muscle (z-axis) (). This yielded a clear correlation between mRNA tissue expression and protein identification in our tissue NE fractions across the wider set of proteins identified and underscores that many tissue differences are conserved across species.
Figure 3. Global analysis of NE tissue differences. (A) All proteins identified in all tissues are plotted in the heatmap with TM proteins on the left and soluble proteins on the right. Color-coding is for log-transformed dNSAF values, indicating the relative abundance within a particular tissue with red meaning high abundance and blue low abundance. Raw dNSAF values are given in Table S3. Black indicates absence from a particular data set. The PHA-activated blood leukocyte data set was used, but results are indistinguishable from the separate unstimulated blood leukocyte data set. The differences between tissues and the lack of a correlation between abundance in a tissue and overall abundance further support the tissue specificity. (B) The percent identity between mouse, rat and human homologs was calculated for all NE proteins and the distribution is plotted using variable width Tukey boxplots according to the number of tissues in which each protein was found. The proteins found in all three tissues were significantly more conserved in sequence than the proteins found in just one (Kolmogorov-Smirnov test: D = 0.1547, p value 4.0 x 10−15). (C) NE proteins that were identified in at least 60% of runs compared with data from the BioGPS transcriptome database. Proteins identified in different tissues were color-coded: PHA-activated human blood leukocytes (red), rat muscle (yellow), rat liver (blue), PHA-activated blood leukocytes and muscle (orange), PHA-activated blood leukocytes and liver (purple), muscle and liver (green), all three tissues (brown). These were then plotted according to their level of expression in the different tissues such that those more specifically expressed in human blood, muscle or liver respectively climb along the x-, y- and z-axis. Note that the BioGPS transcriptome database did not have a separate leukocyte-enriched population similar to that used for the proteomic analysis; therefore, whole blood was used for the comparison because expression in this tissue should encompass all the proteins identified in the more restricted blood leukocyte NE data sets.
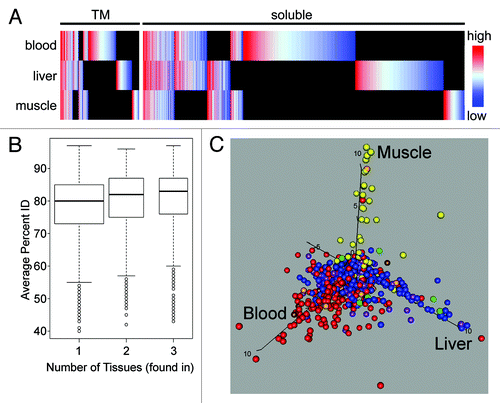
The calculated percentage of NETs shared between the muscle, liver and blood leukocyte NEs was remarkably small—only 16% of the total NETs identified (). Thus the vast majority of NETs identified are distinct in certain tissues. These tissue differences are not driven by species differences because the conservation between the two rat tissues (liver and muscle: 31%) is similar to that between these and the human leukocytes (25% and 27%). Prep-to-prep variation should have been largely averaged out because, due to low yields in isolating clean leukocyte and muscle NEs, at least 12 individual preparations were combined. A value for shared proteins around 10% was maintained whether considering all proteins identified including soluble proteins or considering subsets that represent higher stringency criteria such as the NETs, those with spectral abundance in NEs > 5-fold higher than microsomes or proteins identified in multiple MudPIT runs (). Nonetheless, this number should not be taken as an absolute value because of the inability to distinguish all contaminants obtained during NE isolation.
Figure 4. NE tissue distinctiveness. (A) Numbers of NETs overlapping between rat liver, rat muscle and human PHA-stimulated blood leukocytes expressed using a proportional Venn diagram. For this analysis PHA-stimulated blood leukocytes were considered separately from the leukocytes that were not stimulated (hence fewer than the 1,037 total NETs identified). This was done because protein differences between the two conditions render them almost like separate tissuesCitation9; however, results were similar when comparing either blood leukocyte data set with the other tissues. (B) The small amount of overlap between tissues for the NE proteome is maintained for higher stringency protein subsets. Plotting the percentage of proteins shared between all three tissues yields a value ~10% that is maintained when considering higher stringency subsets: NET, transmembrane proteins; 5x, 5-fold enriched over microsomes by abundance estimates (dNSAF); Multi, appeared in multiple MudPIT runs for the same tissue. (C) Proportional Venn diagram of transmembrane proteins found in NE data sets that had GO-targeting annotations associated with other organelles. As in A, just the PHA-stimulated blood leukocytes are shown for the comparison. Many more proteins were identified in multiple tissues among this set compared with the total set of NETs shown in . (D) The percentage of total proteins in a particular NE tissue data set that have GO-targeting annotations for each organelle is plotted by each contaminating organelle (cyto-mbv is cytoplasmic membrane bound vesicles). Most organelles represented less than 3% contamination of any NE preparation. Moreover, the amount was similar for proteins identified in individual or multiple NE tissue data sets. Only mitochondria and ER GO-targeting annotated proteins were found more commonly in all NE data sets. Standard deviations are shown. Note that the error bar for mitochondria contaminants in 2-tissues goes above the graph scale: the standard deviation value is 10.96.
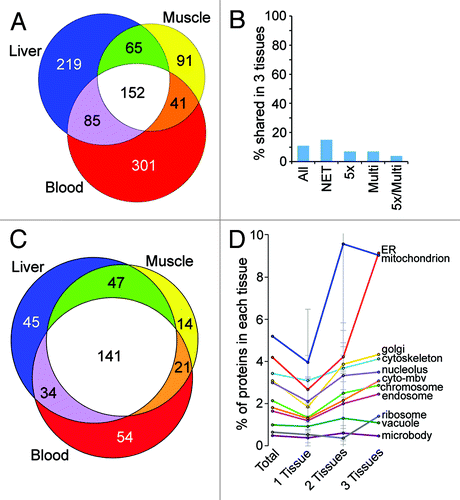
To estimate the level of possible contamination acquired during cell fractionation, the complete data sets (5,222 proteins) were searched for proteins with gene ontology (GO)-localization terms for other cellular organelles (particularly membrane bound organelles). The tissue distribution of this subset of NE proteins that have previously been linked to other organelles was the opposite of that for the whole data sets, with the largest fraction being shared by all three tissues for both the total protein set (not shown) and for 356 NETs (). Thus, if these proteins were considered as contaminants it would only increase the amount of tissue specificity among the remaining proteins.
If the tissue differences were due to a particular organelle more readily co-purifying with NEs in one tissue vs. another, then contaminants from that organelle would be expected to accumulate in a particular tissue. This was not the case for any organelle (). Instead the percentage of these potential contaminants in each tissue was roughly equal for individual organelles, whether considering those identified exclusively in one tissue, in any two tissues or in all three tissues.
Proteins with GO-localization terms for any individual organelle generally represented only 0.5 to 3% of total proteins in any tissue data set with the exception of ER and mitochondria (). This could indicate contamination due to a favored relationship between the NE and these organelles. Indeed, as noted before, the ER is continuous with the NECitation13 and also mitochondria are observed in invaginations of the NE.Citation23 However, the fact that only a fraction of the known proteins from these organelles were identified in the NE preparations is perhaps more consistent with this subset of proteins having functions in both organelles. Interestingly, a large number of these proteins were associated with multiple GO-localization terms, further consistent with their identification as valid NE components.
If viewing those proteins enriched in the ER and mitochondria as more likely to be contaminants, then the use of the enriched data set (with normalized spectral abundance in the NE data sets > 5-fold over the microsome data sets) should yield high confidence in identifications. Mitochondrial proteinsCitation8 accounted for 3% of those in the NE data sets: subtracting those left 3,946 proteins in the mixed enriched data set of which 571 were putative NETs. Because not all NETs were exclusive to the NE, we refer to individual NETs using their gene names.
Direct confirmation of NET tissue specificity
The only way to verify if a protein is a true NE component is through direct testing by microscopy for targeting to the NE. The validity of these data sets is supported by the confirmation either with tagged fusions or antibodies thus far of 87 NETs (Table S4). All of the original 13 NETs met the enrichment criterion of being > 5-fold enriched in the NE compared with microsomes and nearly 90% of the new NETs meeting this criterion that have been tested are now confirmed. Strikingly, over half of the 74 new NETs confirmed to at least partially accumulate at the NE were < 5-fold enriched compared with microsomal proteins based on normalized spectral counts—in fact 8 had very similar levels. Interestingly, 95% of the proteins tested in the < 5-fold enriched set were validated as targeting to the NE. Thus many proteins in the data sets clearly occupy multiple cellular compartments, as expected from the continuity between the ER and outer nuclear membrane.
Several proteins identified in only one of the extraction conditions were confirmed at the NE, further validating the methodology of using different extractions and sequential digests to increase identification of membrane proteins. NET33/SCARA5 and Tmem70 were identified only in the proteinase K digested data sets while NET34/SLC39A14 and NET62/MCAT were identified only in the salt/ detergent extracted data sets and another 16 proteins were identified only in the NaOH extracted/ trypsin digested data sets.
Tissue specificity was directly tested for several NETs by investigating transcript and protein levels and antibody staining in different tissues. Transcript levels of 64 putative and confirmed NETs were compared by RT-PCR in 8 human tissues (; Fig. S2 and Table S4). Though some were ubiquitously expressed, many NET transcripts were only detected in a subset of the tissues examined. This tissue expression largely matched the tissue identification by mass spectrometry. Tissue differences at the protein level were confirmed by western blot with NET antibodies comparing rat liver, heart, leg muscle and thymus lysates (). Equal amounts of each tissue were resolved on the same gels for western blotting and the signals for bands corresponding to each NET were directly quantified from fluorophores conjugated to the antibodies. The fraction for each tissue from the total signal from all tissues combined is plotted.
Figure 5. Experimental confirmation of NET tissue-specificity. (A) RT-PCR from human tissue RNA confirms that several of the NETs identified by MudPIT in a particular tissue have messages preferentially transcribed in that tissue and often absent from a subset of other tissues. NETs shown are grouped according to the tissue of highest expression (muscle, liver or blood leukocytes), but some were found in multiple data sets. Peptidylprolyl isomerase A (PPIA) was used as a loading control. (B) Protein tissue specificity assessed by western blot. Equal amounts of protein from lysates of rat liver, heart, muscle and thymus were compared on the same blots for the levels of different NETs. NET levels were quantified using fluorescently labeled secondary antibodies using a LI-COR system. The total signal for all four tissues was calculated and the fraction of the total signal in each tissue is colored in the plot. The average values from three blots are shown. (C) Cryosections of rat heart or leg muscle, liver and spleen were stained with NET antibodies. Nuclear rim staining was only observed in the tissue where the NET was identified by proteomics: C17orf62 was more abundant in blood leukocytes, but identified in all tissues. Sometimes cytoplasmic staining was also observed, consistent with NETs occupying multiple cellular locations; however, most of the appearance of NETs in the cytoplasm in other tissues comes from increasing exposure times in order to see the background. Bars 10 µm.
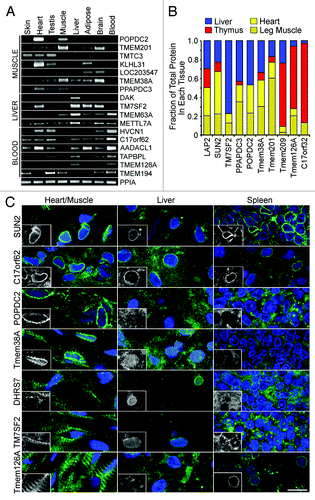
Finally, antibodies generated to several of the confirmed NETs identified in liver, muscle and/ or leukocytesCitation9,Citation10,Citation24 were tested on cryosections from rat muscle, liver and spleen (). With the exception of C17orf62, which was identified in all three tissues, NE staining was only observed in the tissue from which the novel NET was identified. For example POPDC2 and Tmem38A were both identified only in muscle and a rim staining around the nucleus as defined by DAPI staining for DNA was observed in the muscle cryosections, but not in the liver or spleen cryosections. To ensure that no weak nuclear rim staining was occurring, the exposure times were much longer in the tissues where rim staining was not observed giving the appearance of high background. Similarly nuclear rim staining is only observed in liver for DHRS7 and TM7SF2 that were identified only in the liver data sets and Tmem126A that was identified uniquely in the leukocytes appeared at the nuclear rim only in spleen (). Importantly, it can be observed that even within a tissue staining was restricted to only certain cell types. Tissues are made up of multiple cell types and, accordingly, Tmem38A strongly stains only 3 of the 6 nuclei in the image shown. The cryosection data importantly demonstrate that tissue-specificity applies to NE residence, even if some moderate expression is observed in another tissue.
Functional consequences of NE tissue differences
General protein characteristics such as isoelectric point, transmembrane topology and prevalence of coiled-coils did not vary between NETs identified in different tissues (data not shown). At the same time the previously suggested tendency for NETs to have higher isoelectric pointsCitation25 was supported, suggesting that these data sets can be used to extract general characteristics of NETs.
A further support of both the tissue distinctiveness of the NE and its functional importance is that the components of a particular protein complex were often found together in one tissue while being absent from the other tissues. NE proteins from the different tissue data sets were searched for their occurrence in complexes listed in the HPRD database at Johns Hopkins University. For each complex for which a component was found in the NE data sets the proportion of complex components identified in the NE of each tissue was plotted (). Though few complexes were recovered in their entirety in all three tissues, in many cases a complex was fully identified in one tissue but not identified at all or only partially identified in other tissues. In these latter cases the complex might contain different components in different tissues. These same characteristics were observed when considering only complexes containing transmembrane components ().
Figure 6. Differences in NE functional composition in different tissues. (A) Proteins identified in at least 60% of runs for a particular NE fraction were searched for their inclusion in the Johns Hopkins HPRD database of annotated protein complexes and then for each complex the proportion of complex components found in each tissue was calculated. Data are shown for the 352 complexes for which at least 75% of components were found in the data sets and the percentage for each tissue is plotted additively with liver in blue, muscle in yellow and blood leukocytes in red. The maximum proportion for any individual complex is 1; therefore a complex fully intact in all three tissues would have a value of 3. For many complexes all components were found in only one or two of the tissues. (B) The percentage of complex components found in each tissue is similarly plotted, but restricted to those complexes containing a NET. (C-F) Within the subset of proteins in NE data sets with GO-annotations, the fraction with a particular functional annotation was calculated. Similar fractions were calculated against all “nuclear”-annotated proteins in the GO-database. The relative ratio of NE/nuclear fractions was then calculated, setting a 1:1 ratio to 0 so that positive values are fold-relative enrichment and negative are fold-relative deficiency at the NE compared with the whole nucleus. Relative enrichment thus indicates a function that is more enriched at the NE compared with other functions associated with the nucleus as opposed to indicating a concentration at the NE. Liver, muscle and unstimulated and activated blood leukocyte data sets are represented by blue, yellow, pink and red bars, respectively. (C) General functions such as nuclear transport, signaling and ion transport were relatively enriched at the periphery. (D) More specific RNA functions revealed some tissue differences in relative NE enrichment. (E) Certain epigenetic functions were relatively enriched at the NE in particular tissues. (F) Some signaling functions were relatively enriched at the NE in certain tissues. For example, Wnt signaling was relatively enriched at the NE in liver while being strongly deficient at the NE in unstimulated blood leukocytes.
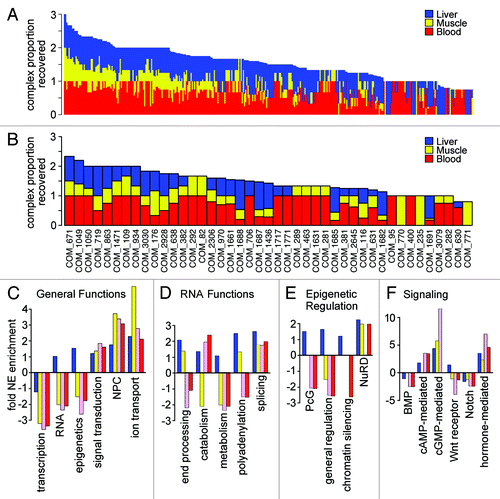
Another possible reason for NE tissue specificity is suggested by the subset of proteins identified at the NE that have Gene Ontology (GO)Citation26 functional annotations. To determine if particular nuclear GO-functions were partly accumulating at the NE so that they become relatively enriched at the NE, the fraction of our NE proteins with a particular functional annotation was compared with the fraction of total GO-annotated nuclear proteins with the same functional annotations. Thus a positive value for this ratio indicates relative enrichment at the NE such that the percentage of NE proteins devoted to a particular function is greater than the percentage of GO-nuclear proteins devoted to that same function. Negative values indicate relative enrichment in the nucleoplasm (−F). All GO-functional annotations used were experimentally verified.
General functional categories yielded expected distributions, e.g., DNA and RNA functions were relatively enriched in the nucleoplasm while transport functions were relatively enriched at the NE (). However, more specific functions varied in relative NE enrichment, some according to the tissues sampled. Although as expected general RNA functions were more nucleoplasmic, those proteins involved in splicing were relatively enriched at the NE (). This does not mean that splicing preferentially occurs at the NE compared with the nucleoplasm (it is clearly more nucleoplasmic by localization studies), but that among GO functions splicing occurs more often at the NE than other GO functions. The relative enrichment of some splicing functions at the NE is perhaps due to splicing factors that remain associated with mRNAs during transport through the nuclear pore complex. Other GO-functions had tissue-specific differences in their relative functional enrichment at the NE. Polyadenylation functions, for example, were relatively enriched at the NE only in liver and muscle, but not in leukocytes.
Although most epigenetic regulatory functional groupings were not relatively enriched at the NE, certain silencing factors became relatively enriched at the NE in certain tissues. Polycomb group proteins were only relatively enriched at the NE in liver while NuRD was relatively enriched in all but unstimulated blood leukocytes (). Similarly, several proteins involved in functional groupings for signaling pathways were also relatively enriched at the NE (). Of note, a large difference was observed between unstimulated and PHA-stimulated blood leukocytes in cGMP-mediated signaling, Wnt receptor signaling was only relatively enriched in liver and BMP signaling was more relatively enriched in the nucleoplasm in blood leukocytes than in liver and muscle.
Discussion
The high degree of tissue specificity in the NE proteome observed here was not expected. However, it is not surprising in retrospect when considering that the three tissues compared are all made up of many different cell types that have striking differences in nuclear size, shape and the amount of dense chromatin at the NE. For example, in addition to hepatocytes liver contains biliary epithelia, sinusoidal cells, Kupffer cells and stellate cells as well as connective tissue, veins and arteries and muscle composition is similarly diverse. Though the blood cells were determined to be roughly 70% leukocytes, there were also many other cell types present. Applying the same bioinformatic analysis to a previously published data set of mitochondria from different tissuesCitation8 indicates that the NE has at least 3-fold more tissue specificity than mitochondria. However, even the differences observed for mitochondria are large enough that these studies together strongly argue for evaluation of the protein complement of all organelles in different tissues. Furthermore the functional implications of this work underscore the importance of considering the possibility of tissue-specific mediators when studying the function of most well-characterized proteins.
As roughly a third of the exome is predicted to encode transmembrane proteins, it is important to develop improved methods for their detection in proteomic analyses. Most such approaches have focused on chemically improving resolution of membrane proteins on 2D gels, but it is generally assumed that LC/LC/MS/MS approaches avoid the losses inherent in 2D gels.Citation27,Citation28 While this is certainly true to some extent, the greater than 10% increase in NET identifications we observe by using multiple extraction and digestion conditions strongly argue that proteomic studies still tend to under-represent transmembrane proteins and provides a simple approach that can increase membrane protein identifications. Our results also underscore the importance of engaging multiple replicate runs for complex fractions. Though some of the differences between liver NE proteins identified in this study compared with a previous MudPIT analysis of liver NEsCitation11 might be attributed to improvements in peptide fragmentation and identification in the mass spectrometers used, within this study the replicate runs and sequential digests roughly doubled the number of identifications. Thus the identification of 2,921 liver NE proteins in this study compared with 1,150 in the previous study is apparently mostly due to these two procedural changes since the purification procedures used to isolate NEs from liver were identical in both studies.
The extreme sensitivity of mass spectrometry often yields identification of even minor contaminants in a sample. As cellular fractions are inherently impossible to purify to homogeneity, this has led to a tendency to use cutoffs based on abundance estimates as an effective in silica purification step. While this makes sense for most soluble proteins, that 95% of NETs tested from the < 5-fold enriched set were confirmed as targeting at least in part to the NE argues that a separate, less stringent cutoff may be appropriate for transmembrane proteins.
The reason that close to half of the total NETs identified were not > 5-fold enriched at the NE is likely because they have multiple cellular localizations. This is consistent with separate high-throughput observations that 40% of all proteins have multiple cellular localizationsCitation16 as well as the physical structure of the NE in association with both mitochondria and ER.Citation13,Citation23 Thus it is not surprising that three tissue-specific proteins in the data sets previously published as mitochondrial and ER proteins (MARCHV, Tmem70 and Tmem38A;Citation29-Citation31) were confirmed as NETs in the inner nuclear membrane by super-resolution microscopy.Citation9,Citation10 Even the well characterized and NE-enriched NET emerin has now been found, in addition to its predominant inner nuclear membrane localization, also to associate with the centrosome in the outer nuclear membrane, the cytoplasm in myotubes and interstitial discs in cardiac tissue.Citation14,Citation32,Citation33
In keeping with this tendency for multiple cellular localizations, the < 5-fold enriched set includes several proteins now confirmed at the NE by others that have characterized functions in small molecule transport or signaling (Table S4;Citation34-Citation38). The exclusion limit of the nuclear pore complexes allows for small molecules and ions to exchangeCitation39; thus, the nucleus likely needs a variety of membrane transporters to maintain ion levels and pH. Notably, in muscle, where strong calcium fluxes during contraction could result in leakage into the nucleus that might damage the genome, there was an abundance of calcium transporters and associated proteins identified in the NE data sets that could clear the ion from the nucleus (Table S3). The identification of signaling molecules identified in the NE by direct testingCitation36,Citation38,Citation40,Citation41 supports the wider findings from the GO-functional term analysis of tissue-specific accumulation of signaling molecules in the NE. The specific observations regarding some NE accumulation of Wnt and BMP signaling proteins in certain tissues () reinforce separate observations that Smads and β-catenin interact with the NETs MAN1 and emerinCitation42,Citation43 and, perhaps more importantly, provide an explanation for how mutation of these ubiquitously expressed NETs can lead to tissue-specific disease pathologies.
The identification of chromatin binding/modifying proteins in association with the NE is also supported by various individual observations in the literature (e.g., LAP2β with BAF and HDAC3,Citation44,Citation45 hALP1 with SUN1,Citation46 emerin with lmo7,Citation47 MeCP2 and HP1 with LBRCitation48,Citation49), though this study provides the first large-scale sampling of such proteins at the NE. Interestingly, the NuRD complex we find to be relatively enriched at the NE in certain tissues () is involved in progeria defects caused by NE mutations.Citation50 Thus known proteins in these data sets fit with the current literature for the NE influencing genome functions and these functions are tissue-specific. This indicates the likelihood that some of the 419 new NE proteins lacking GO-annotations (including both soluble proteins and NETs) also will contribute to genome functions. This likelihood is supported by findings that several NETs with unknown functions identified in the leukocyte data sets could alter genome organization.Citation9 More compelling, though, is the observation that even a protein with a known and distinct function identified in our data sets—the Na,K-ATPase βm-subunit—has been confirmed at the NE and found to serve a secondary function as a co-regulator of transcription.Citation51,Citation52
NETs and their soluble partners that accumulate at the NE only in certain tissues could explain the focused tissue pathology in NE diseases. For example a muscle-specific NET that complexes with Lamin A or Emerin could be lost from the NE with mutations in these proteins linked to Emery-Dreifuss muscular dystrophy.Citation53,Citation54 Differences between tissues in NE signaling pathways or chromatin organization/ gene regulation indicated here could result in particular tissues having greater susceptibility to disruption of specific functions with particular NE mutations. In keeping with this idea, the NuRD complex and signaling proteins indicated here to vary at the NE between tissues have been linked to various NE diseases and proteins.Citation42,Citation43,Citation50 The primary deficits in heritable diseases tend to localize in a particular tissue and moreover within a particular organelle. Thus the subcellular location and tissue distribution of proteins linked to disease are important in constructing a model for how their mutation can lead to pathology. This study suggests that to better understand such diseases all cell organelles should be analyzed for tissue specificity in their proteomes.
Materials and Methods
Preparation and MudPIT analysis of NEs
Rat liver NEs and microsomes were prepared and analyzed by MudPIT as described in references Citation55 and Citation56 The human blood leukocyte and rat muscle NE preparation and data sets are described in detail in.Citation9,Citation10 All protein pellets were solubilized in 0.1 M TRIS-HCl, pH 8.5, 8 M urea, 5 mM TCEP. Iodoacetamide was added to 10 mM for 30 min and endoproteinase Lys-C and trypsin digestion performed as above. Samples were centrifuged 30 min at 17,500 × g. Supernatants (“Ti” digests) were analyzed by MudPIT while pellets were resuspended in 0.1 M Na2CO3 pH 11.5, 8 M urea, 5 mM TCEP for 30 min, then 10 mM iodoacetamide 30 min and then digested with proteinase K 4 h at 37°CCitation57 and also analyzed by MudPIT (“PK” digests).
Importantly in all cases at least five separate MudPIT runsCitation19,Citation20 were engaged for each preparation. During the course of a fully automated chromatography, 15 120 min cycles (Table S1) of increasing salt concentrations followed by organic gradients slowly released peptides directly into the mass spectrometer.Citation56 Three different elution buffers were used: 5% acetonitrile, 0.1% formic acid (Buffer A); 80% acetonitrile, 0.1% formic acid (Buffer B); and 0.5 M ammonium acetate, 5% acetonitrile, 0.1% formic acid (Buffer C). The last five (out of 15) chromatography steps consisted in a high salt wash with 100% Buffer C followed by the acetonitrile gradient. The distal application of a 2.5 kV voltage electrosprayed the eluting peptides directly into ion trap mass spectrometers equipped with a nano-LC electrospray ionization source (ThermoFinnigan). Each full MS scan (from 400 to 1,600 m/z) was followed by five (LTQ) MS/MS events using data-dependent acquisition where the first most intense ion was isolated and fragmented by collision-induced dissociation (at 35% collision energy), followed by the second to 5th most intense ions. The raw data from each run is available at the Proteome Commons Tranche repository through the links given in Table S1.
Data analysis
RAW files were extracted into ms2 file formatCitation58 using RAW_Xtract v.1.0.Citation59 MS/MS spectra—including the liver NEs and ER/microsomes data fromCitation11—were queried for peptide sequence information using SEQUESTTM v.27 (rev.9)Citation60 against 28,400 rat proteins (non-redundant NCBI sequences on July 10, 2006), plus 197 human and mouse homologs of previously identified NETsCitation9-Citation11 and 172 sequences from usual contaminants (e.g., human keratins, IgGs, proteolytic enzymes, etc.). In addition, sequences for proteins we had annotated as NETs using previous database releases were added to these databases. Finally, to estimate false discovery rates, each non-redundant protein entry was randomized. The resulting “shuffled” sequences were added to the database and searched at the same time as the “forward” sequences, leading to a total search space of 57,538 and 60,828 sequences for the rat and mouse databases (Table S1). MS/MS spectra were searched without specifying differential modifications. To account for carboxamidomethylation by IAM, +57 Da were added statically to cysteine residues for all the searches. No enzyme specificity was imposed during searches, setting a mass tolerance of 3 amu for precursor ions and of ± 0.5 amu for fragment ions.
Spectrum/peptide matches were selected using DTASelectCitation61 and only retained if peptides were at least 7 amino acids long and their ends had to comply with the specificity of the proteolytic enzymes used, when appropriate. For trypsin-digested samples, peptides had to be fully tryptic, while for samples that had been chemically cleaved with CNBr prior to trypsin digestion (previously acquired mouse NE data set), Methionine or Lysine or Arginine had to be present before the N-terminus and at the C-terminus of the peptide sequences. In both cases, the DeltCn had to be at least 0.08, with a minimum XCorr of 1.8 for singly-, 2.0 for doubly- and3.0 for triply-charged spectra and a maximum Sp rank of 10. For the proteinase K-digested samples, no specific peptide ends were imposed, but the DeltCn cut-off was increased to 0.15,Citation62 while XCorr minima were increased to 2.5 for doubly- and3.5 for triply-charged spectra. SEQUEST parameters for the spectrum to peptide matches for all detected proteins from rat liver NE and microsomal membranes are provided in Tables S2A and S2B, respectively. Results from different runs were compared and merged using CONTRASTCitation61 (Table S2C). Proteins that were subset of others were removed. NSAF7 (Tim Wen) was used to create the final report (Table S2C) on all detected proteins across the different runs, calculate their respective Normalized Spectral Abundance Factor (NSAF) values and estimate false discovery rates (FDR).
Spectral FDR was calculated as:
Protein level FDR was calculated as:
Under these criteria the final FDRs at the protein and peptide levels were 2.8 ±1.5% and 0.4 ±0.2%, respectively.
To estimate relative protein levels, distributed normalized spectral abundance factors (dNSAFs) were calculated for each non-redundant protein or protein group, as described in:Citation18
with
in which shared spectral counts (sSpC) were distributed based on spectral counts unique to each protein i (uSpC) divided by the sum of all unique spectral counts for the M protein isoforms that shared peptide j with protein i (Tables S2C and S3).
Antibodies and western blotting
Antibodies used: GAPDH (Enogene, E1C604), Calreticulin (Cell Signaling, 2891S), Calnexin (Stressgen, SPA-860), lamin A (3262), NET antibodies were rabbit polyclonals generated to peptides from human sequences (Millipore) LAP2β (06–1002), SUN2 (06–1038), TMTC3 (06–1009), TM7SF2 (06–1026), TMEM126A (06–1037), TMEM201 (06–1013), C17orf62 (06–1033), C17orf32 (06–1035), PPAPDC3 (06–1025), TMEM38A (06–1005), POPDC2 (06–1007), TMEM209 (06–1020), DHRS7 (06–1027).
Rat tissue lysates were prepared by grinding tissues under liquid nitrogen, adding sample buffer (100 mM Tris pH 6.8, 4 M Urea, 2% SDS, 50 mM DTT and 15% sucrose) and heating at 65°C 10 min followed by sonibath sonication. Loading was normalized with GAPDH antibodies. Mitochondria were prepared by pelleting a liver post-nuclear supernatant at 11,000x g 15 min and lysing in sample buffer. To increase lamina solubility, liver NE and microsomes were incubated on ice in 50 mM TRIS-HCl pH 7.4, 150 mM NaCl, 2 mM MgCl2, 0.2% NP-40 with protease inhibitors, then heated at 65°C for 2 min and sonicated in a 4°C sonibath. Protein concentrations were determined by Bradford assay before adding sample buffer.
For and blots after quantification of protein levels in the lysates, equal amounts of protein were added for NEs and microsomes. For blots, mitochondrial lysates were loaded so that porin levels matched those in total cell lysates and NE lysates were loaded so that the lamin levels matched those in total cell lysates. Blots shown in were run according to standard procedures visualizing bands with ECL reagent. For , protein bands were visualized and quantified with IR800-conjugated secondary antibodies using a LI-COR Odyssey and median background subtraction and averages from three independent blots are plotted.
RT-PCR
All human total tissue RNAs for RT-PCR reactions were obtained from Stratagene except for peripheral blood leukocytes (PBL). In this case RNA was isolated using Trizol from cells prepared as for the blood leukocyte proteomics. Reactions were performed with 10 ng of the tissue RNAs using the Titan one tube RT-PCR system (Roche) according to manufacturer’s instructions, except that dNTP concentration was increased to 500 µM and MgCl2 to 3 mM. Typical reaction conditions were 30 min reverse transcription at 50°C, 2 min denaturation at 94°C, then 24 cycles of 94°C for 30 sec, 60°C for 30 sec and 68°C for 45 sec. Peptidylprolyl isomerase A (PPIA) was used as a loading control and reactions were typically repeated at least three times when notable differences were observed.
Immunofluorescence microscopy
For cryosections, fresh rat tissues cut into 2–3 mm cubes were embedded in Optimal Cutting Temperature Compound (Tissue-Tek) and snap-frozen in liquid nitrogen. Sections were cut on a Leica CM 1900 Cryostat at 6–8 μm thickness and fixed in -20°C methanol. After rehydration, sections were incubated with NET antibodies O/N at 4°C followed by 2° antibodies as above. Images were recorded using an SP5 laser confocal system with 63× oil 1.4 NA objective (Leica). Micrographs were saved as TIFF files and prepared for figures using Photoshop 8.0.
Bioinformatics analysis
Proteins identified by SEQUEST were first mapped to an Ensembl gene. Human/Rat/Mouse orthologous groups were identified with Ensembl release 48Citation63 to remove redundancy and false variation that might have resulted from differences in human and rodent gene assignments. Orthologous group IDs were sorted according to run criteria (e.g., appearance in runs for different tissues, membrane helix status and 5x higher dNSAF values in NEs vs microsomes) and compared using Venn diagrams to measure the level of tissue distinctness. Area proportional Venn diagrams were generated using Venn Diagram Plotter v1.4 from PNNL, US Department of Energy (http://omics.pnl.gov/software/VennDiagramPlotter.php).
Basic properties of proteins listed in supplemental tables were calculated using BioPerl modulesCitation64 or EMBOSS.Citation65 PSORTII was used for the prediction of nuclear localization signals.Citation66 For prediction of transmembrane spans it is important to note that a very stringent set of criteria was used for those annotated as “TM” in the supplemental tables and used for the numbers generated comparing NETs in various figures. Only proteins that had predictions by TM-HMM version 2.0cCitation67 AND which had no signal peptide (SP) prediction if they had only one predicted membrane span were used for this analysis. However, some NETs confirmed at the NE (including one of the original pre-proteomics NETs) did not have membrane spans predicted using these criteria. The detailed listing of TM-HMM and SP predictions is given in Table S3.
To plot heatmaps the log2 of the dNSAF scores for NETs within a particular tissue were z-transformed to standardize between experiments.
Comparison of expression levels in different tissues was done by downloading microarray signal data from BioGPS at http://biogps.gnf.org/Citation21,Citation22 and calculating the fold-expression over the median value from a wide variety of mouse tissues tested in this transcriptome database. For just those proteins appearing in ~60% or more of MudPIT runs were considered.
Proteins were searched against the human protein reference database (HPRD, Johns Hopkins University) to identify protein complexes. These were compared between the individual NE data sets to determine how many complex components were identified in each tissue and then restricted to those that had at least 75% of complex components identified between all data sets.
Biologically interesting gene ontology (GO)-terms and their corresponding child terms were retrieved from the mySQL database http://amigo.geneontology.org.Citation26 To ensure a fair comparison for term enrichment, only human-mapped genes in our data set were considered. These were compared with the genomic data set of human Ensembl genes using BioMart (http://www.biomart.org/) as well as those GO-defined as having nuclear localization. Only experimentally verified GO-functional annotations were used including EXP (Inferred from EXperiment), IDA (Inferred from Direct Assay) andIPI (Inferred from Physical Interaction). For a given GO-term, the fraction of genes containing that term or any of the child terms was calculated for all data sets. The fold-difference was calculated by dividing this fractional value from our data set of interest by the value from the reference group. GO terms were also used to identify potential contaminants as proteins with GO-targeting annotations for other organelles as follows. GO:0016023, cytoplasmic membrane-bounded vesicle; GO:0005794, Golgi apparatus; GO:0005739, mitochondrion; GO:0005773, vacuole; GO:0005768, endosome; GO:0005783, endoplasmic reticulum; GO:0042579, microbody; GO:0005856, cytoskeleton; GO:0005694, chromosome; GO:0005730, nucleolus; GO:0005840, ribosome.
Additional material
Download Zip (31.8 MB)Disclosure of Potential Conflicts of Interest
No potential conflicts of interest were disclosed.
Acknowledgments
This study was supported by the Wellcome Trust 076616 and 095209 (E.C.S.) and The Stowers Institute for Medical Research (L.F.). The Wellcome Trust Centre for Cell Biology is supported by core funding from the Wellcome Trust 077707. D.G.B. is a Darwin Trust student. We thank S. Mitchell for assistance with electron microscopy and D. Tollervey and W.C. Earnshaw for comments and discussion.
Supplemental Materials
Supplemental materials may be found here:
http://www.landesbioscience.com/journals/nucleus/article/22257/
References
- Dauer WT, Worman HJ. The nuclear envelope as a signaling node in development and disease. Dev Cell 2009; 17:626 - 38; http://dx.doi.org/10.1016/j.devcel.2009.10.016; PMID: 19922868
- Oza P, Jaspersen SL, Miele A, Dekker J, Peterson CL. Mechanisms that regulate localization of a DNA double-strand break to the nuclear periphery. Genes Dev 2009; 23:912 - 27; http://dx.doi.org/10.1101/gad.1782209; PMID: 19390086
- Korfali N, Srsen V, Waterfall M, Batrakou DG, Pekovic V, Hutchison CJ, et al. A flow cytometry-based screen of nuclear envelope transmembrane proteins identifies NET4/Tmem53 as involved in stress-dependent cell cycle withdrawal. PLoS One 2011; 6:e18762; http://dx.doi.org/10.1371/journal.pone.0018762; PMID: 21533191
- Lee JS, Hale CM, Panorchan P, Khatau SB, George JP, Tseng Y, et al. Nuclear lamin A/C deficiency induces defects in cell mechanics, polarization andmigration. Biophys J 2007; 93:2542 - 52; http://dx.doi.org/10.1529/biophysj.106.102426; PMID: 17631533
- Foisner R, Aebi U, Bonne G, Gruenbaum Y, Novelli G. 141st ENMC International Workshop inaugural meeting of the EURO-Laminopathies project “Nuclear Envelope-linked Rare Human Diseases: From Molecular Pathophysiology towards Clinical Applications”, 10-12 March 2006, Naarden, The Netherlands. Neuromuscul Disord 2007; 17:655 - 60; http://dx.doi.org/10.1016/j.nmd.2007.04.003; PMID: 17587579
- Schirmer EC, Gerace L. The nuclear membrane proteome: extending the envelope. Trends Biochem Sci 2005; 30:551 - 8; http://dx.doi.org/10.1016/j.tibs.2005.08.003; PMID: 16125387
- Wilkie GS, Schirmer EC. Guilt by association: the nuclear envelope proteome and disease. Mol Cell Proteomics 2006; 5:1865 - 75; http://dx.doi.org/10.1074/mcp.R600003-MCP200; PMID: 16790741
- Mootha VK, Bunkenborg J, Olsen JV, Hjerrild M, Wisniewski JR, Stahl E, et al. Integrated analysis of protein composition, tissue diversity andgene regulation in mouse mitochondria. Cell 2003; 115:629 - 40; http://dx.doi.org/10.1016/S0092-8674(03)00926-7; PMID: 14651853
- Korfali N, Wilkie GS, Swanson SK, Srsen V, Batrakou DG, Fairley EA, et al. The leukocyte nuclear envelope proteome varies with cell activation and contains novel transmembrane proteins that affect genome architecture. Mol Cell Proteomics 2010; 9:2571 - 85; http://dx.doi.org/10.1074/mcp.M110.002915; PMID: 20693407
- Wilkie GS, Korfali N, Swanson SK, Malik P, Srsen V, Batrakou DG, et al. Several novel nuclear envelope transmembrane proteins identified in skeletal muscle have cytoskeletal associations. Mol Cell Proteomics 2011; 10:M110 - , 003129; http://dx.doi.org/10.1074/mcp.M110.003129; PMID: 20876400
- Schirmer EC, Florens L, Guan T, Yates JR 3rd, Gerace L. Nuclear membrane proteins with potential disease links found by subtractive proteomics. Science 2003; 301:1380 - 2; http://dx.doi.org/10.1126/science.1088176; PMID: 12958361
- Dreger M, Bengtsson L, Schöneberg T, Otto H, Hucho F. Nuclear envelope proteomics: novel integral membrane proteins of the inner nuclear membrane. Proc Natl Acad Sci U S A 2001; 98:11943 - 8; http://dx.doi.org/10.1073/pnas.211201898; PMID: 11593002
- Callan HG, Tomlin SG. Experimental studies on amphibian oocyte nuclei. I. Investigation of the structure of the nuclear membrane by means of the electron microscope. Proc R Soc Lond B Biol Sci 1950; 137:367 - 78; http://dx.doi.org/10.1098/rspb.1950.0047; PMID: 14786306
- Salpingidou G, Smertenko A, Hausmanowa-Petrucewicz I, Hussey PJ, Hutchison CJ. A novel role for the nuclear membrane protein emerin in association of the centrosome to the outer nuclear membrane. J Cell Biol 2007; 178:897 - 904; http://dx.doi.org/10.1083/jcb.200702026; PMID: 17785515
- Walter P, Blobel G. Preparation of microsomal membranes for cotranslational protein translocation. Methods Enzymol 1983; 96:84 - 93; http://dx.doi.org/10.1016/S0076-6879(83)96010-X; PMID: 6656655
- Foster LJ, de Hoog CL, Zhang Y, Zhang Y, Xie X, Mootha VK, et al. A mammalian organelle map by protein correlation profiling. Cell 2006; 125:187 - 99; http://dx.doi.org/10.1016/j.cell.2006.03.022; PMID: 16615899
- Paoletti AC, Parmely TJ, Tomomori-Sato C, Sato S, Zhu D, Conaway RC, et al. Quantitative proteomic analysis of distinct mammalian Mediator complexes using normalized spectral abundance factors. Proc Natl Acad Sci U S A 2006; 103:18928 - 33; http://dx.doi.org/10.1073/pnas.0606379103; PMID: 17138671
- Zhang Y, Wen Z, Washburn MP, Florens L. Refinements to label free proteome quantitation: how to deal with peptides shared by multiple proteins. Anal Chem 2010; 82:2272 - 81; http://dx.doi.org/10.1021/ac9023999; PMID: 20166708
- Washburn MP, Wolters D, Yates JR 3rd. Large-scale analysis of the yeast proteome by multidimensional protein identification technology. Nat Biotechnol 2001; 19:242 - 7; http://dx.doi.org/10.1038/85686; PMID: 11231557
- Wolters DA, Washburn MP, Yates JR 3rd. An automated multidimensional protein identification technology for shotgun proteomics. Anal Chem 2001; 73:5683 - 90; http://dx.doi.org/10.1021/ac010617e; PMID: 11774908
- Su AI, Cooke MP, Ching KA, Hakak Y, Walker JR, Wiltshire T, et al. Large-scale analysis of the human and mouse transcriptomes. Proc Natl Acad Sci U S A 2002; 99:4465 - 70; http://dx.doi.org/10.1073/pnas.012025199; PMID: 11904358
- Wu C,, Orozco C, Boyer J, Leglise M, Goodale J, Batalov S, et al. BioGPS: an extensible and customizable portal for querying and organizing gene annotation resources. Genome Biol 2009; 10:R130; http://dx.doi.org/10.1186/gb-2009-10-11-r130; PMID: 19919682
- Lui PP, Chan FL, Suen YK, Kwok TT, Kong SK. The nucleus of HeLa cells contains tubular structures for Ca2+ signaling with the involvement of mitochondria. Biochem Biophys Res Commun 2003; 308:826 - 33; http://dx.doi.org/10.1016/S0006-291X(03)01469-4; PMID: 12927793
- Malik P, Korfali N, Srsen V, Lazou V, Batrakou DG, Zuleger N, et al. Cell-specific and lamin-dependent targeting of novel transmembrane proteins in the nuclear envelope. Cell Mol Life Sci 2010; 67:1353 - 69; http://dx.doi.org/10.1007/s00018-010-0257-2; PMID: 20091084
- Ulbert S, Platani M, Boue S, Mattaj IW. Direct membrane protein-DNA interactions required early in nuclear envelope assembly. J Cell Biol 2006; 173:469 - 76; http://dx.doi.org/10.1083/jcb.200512078; PMID: 16717124
- Carbon S, Ireland A, Mungall CJ, Shu S, Marshall B, Lewis S, AmiGO Hub, Web Presence Working Group. AmiGO: online access to ontology and annotation data. Bioinformatics 2009; 25:288 - 9; http://dx.doi.org/10.1093/bioinformatics/btn615; PMID: 19033274
- Helbig AO, Heck AJ, Slijper M. Exploring the membrane proteome--challenges and analytical strategies. J Proteomics 2010; 73:868 - 78; http://dx.doi.org/10.1016/j.jprot.2010.01.005; PMID: 20096812
- Savas JN, Stein BD, Wu CC, Yates JR 3rd. Mass spectrometry accelerates membrane protein analysis. Trends Biochem Sci 2011; 36:388 - 96; PMID: 21616670
- Cízková A, Stránecký V, Mayr JA, Tesarová M, Havlícková V, Paul J, et al. TMEM70 mutations cause isolated ATP synthase deficiency and neonatal mitochondrial encephalocardiomyopathy. Nat Genet 2008; 40:1288 - 90; http://dx.doi.org/10.1038/ng.246; PMID: 18953340
- Karbowski M, Neutzner A, Youle RJ. The mitochondrial E3 ubiquitin ligase MARCH5 is required for Drp1 dependent mitochondrial division. J Cell Biol 2007; 178:71 - 84; http://dx.doi.org/10.1083/jcb.200611064; PMID: 17606867
- Yazawa M, Ferrante C, Feng J, Mio K, Ogura T, Zhang M, et al. TRIC channels are essential for Ca2+ handling in intracellular stores. Nature 2007; 448:78 - 82; http://dx.doi.org/10.1038/nature05928; PMID: 17611541
- Lattanzi G, Ognibene A, Sabatelli P, Capanni C, Toniolo D, Columbaro M, et al. Emerin expression at the early stages of myogenic differentiation. Differentiation 2000; 66:208 - 17; http://dx.doi.org/10.1111/j.1432-0436.2000.660407.x; PMID: 11269947
- Wheeler MA, Warley A, Roberts RG, Ehler E, Ellis JA. Identification of an emerin-beta-catenin complex in the heart important for intercalated disc architecture and beta-catenin localisation. Cell Mol Life Sci 2010; 67:781 - 96; http://dx.doi.org/10.1007/s00018-009-0219-8; PMID: 19997769
- Arteaga MF, Gutiérrez R, Avila J, Mobasheri A, Díaz-Flores L, Martín-Vasallo P. Regeneration influences expression of the Na+, K+-atpase subunit isoforms in the rat peripheral nervous system. Neuroscience 2004; 129:691 - 702; http://dx.doi.org/10.1016/j.neuroscience.2004.08.041; PMID: 15541890
- Bkaily G, Nader M, Avedanian L, Jacques D, Perrault C, Abdel-Samad D, et al. Immunofluorescence revealed the presence of NHE-1 in the nuclear membranes of rat cardiomyocytes and isolated nuclei of human, rabbit andrat aortic and liver tissues. Can J Physiol Pharmacol 2004; 82:805 - 11; http://dx.doi.org/10.1139/y04-119; PMID: 15523538
- Cruttwell C, Bernard J, Hilly M, Nicolas V, Tunwell RE, Mauger JP. Dynamics of the inositol 1,4,5-trisphosphate receptor during polarisation of MDCK cells. Biol Cell 2005; http://dx.doi.org/10.1042/BC20040503; PMID: 15730344
- Erickson ES, Mooren OL, Moore-Nichols D, Dunn RC. Activation of ryanodine receptors in the nuclear envelope alters the conformation of the nuclear pore complex. Biophys Chem 2004; 112:1 - 7; http://dx.doi.org/10.1016/j.bpc.2004.06.010; PMID: 15501570
- Klein C, Gensburger C, Freyermuth S, Nair BC, Labourdette G, Malviya AN. A 120 kDa nuclear phospholipase Cgamma1 protein fragment is stimulated in vivo by EGF signal phosphorylating nuclear membrane EGFR. Biochemistry 2004; 43:15873 - 83; http://dx.doi.org/10.1021/bi048604t; PMID: 15595842
- Paine PL, Moore LC, Horowitz SB. Nuclear envelope permeability. Nature 1975; 254:109 - 14; http://dx.doi.org/10.1038/254109a0; PMID: 1117994
- Maissel A, Marom M, Shtutman M, Shahaf G, Livneh E. PKCeta is localized in the Golgi, ER and nuclear envelope and translocates to the nuclear envelope upon PMA activation and serum-starvation: C1b domain and the pseudosubstrate containing fragment target PKCeta to the Golgi and the nuclear envelope. Cell Signal 2006; 18:1127 - 39; http://dx.doi.org/10.1016/j.cellsig.2005.09.003; PMID: 16242915
- Deng M, Hochstrasser M. Spatially regulated ubiquitin ligation by an ER/nuclear membrane ligase. Nature 2006; 443:827 - 31; http://dx.doi.org/10.1038/nature05170; PMID: 17051211
- Lin F, Morrison JM, Wu W, Worman HJ. MAN1, an integral protein of the inner nuclear membrane, binds Smad2 and Smad3 and antagonizes transforming growth factor-beta signaling. Hum Mol Genet 2005; 14:437 - 45; http://dx.doi.org/10.1093/hmg/ddi040; PMID: 15601644
- Markiewicz E, Tilgner K, Barker N, van de Wetering M, Clevers H, Dorobek M, et al. The inner nuclear membrane protein emerin regulates beta-catenin activity by restricting its accumulation in the nucleus. EMBO J 2006; 25:3275 - 85; http://dx.doi.org/10.1038/sj.emboj.7601230; PMID: 16858403
- Furukawa K. LAP2 binding protein 1 (L2BP1/BAF) is a candidate mediator of LAP2-chromatin interaction. J Cell Sci 1999; 112:2485 - 92; PMID: 10393804
- Somech R, Shaklai S, Geller O, Amariglio N, Simon AJ, Rechavi G, et al. The nuclear-envelope protein and transcriptional repressor LAP2beta interacts with HDAC3 at the nuclear periphery andinduces histone H4 deacetylation. J Cell Sci 2005; 118:4017 - 25; http://dx.doi.org/10.1242/jcs.02521; PMID: 16129885
- Chi YH, Haller K, Peloponese JM Jr., Jeang KT. Histone acetyltransferase hALP and nuclear membrane protein hsSUN1 function in de-condensation of mitotic chromosomes. J Biol Chem 2007; 282:27447 - 58; http://dx.doi.org/10.1074/jbc.M703098200; PMID: 17631499
- Holaska JM, Rais-Bahrami S, Wilson KL. Lmo7 is an emerin-binding protein that regulates the transcription of emerin and many other muscle-relevant genes. Hum Mol Genet 2006; 15:3459 - 72; http://dx.doi.org/10.1093/hmg/ddl423; PMID: 17067998
- Guarda A, Bolognese F, Bonapace IM, Badaracco G. Interaction between the inner nuclear membrane lamin B receptor and the heterochromatic methyl binding protein, MeCP2. Exp Cell Res 2009; 315:1895 - 903; http://dx.doi.org/10.1016/j.yexcr.2009.01.019; PMID: 19331822
- Ye Q, Worman HJ. Interaction between an integral protein of the nuclear envelope inner membrane and human chromodomain proteins homologous to Drosophila HP1. J Biol Chem 1996; 271:14653 - 6; http://dx.doi.org/10.1074/jbc.271.25.14653; PMID: 8663349
- Pegoraro G, Kubben N, Wickert U, Göhler H, Hoffmann K, Misteli T. Ageing-related chromatin defects through loss of the NURD complex. Nat Cell Biol 2009; 11:1261 - 7; http://dx.doi.org/10.1038/ncb1971; PMID: 19734887
- Pestov NB, Korneenko TV, Zhao H, Adams G, Kostina MB, Shakhparonov MI, et al. The betam protein, a member of the X,K-ATPase beta-subunits family, is located intracellularly in pig skeletal muscle. Arch Biochem Biophys 2001; 396:80 - 8; http://dx.doi.org/10.1006/abbi.2001.2599; PMID: 11716465
- Pestov NB, Ahmad N, Korneenko TV, Zhao H, Radkov R, Schaer D, et al. Evolution of Na,K-ATPase beta m-subunit into a coregulator of transcription in placental mammals. Proc Natl Acad Sci U S A 2007; 104:11215 - 20; http://dx.doi.org/10.1073/pnas.0704809104; PMID: 17592128
- Stewart CL, Roux KJ, Burke B. Blurring the boundary: the nuclear envelope extends its reach. Science 2007; 318:1408 - 12; http://dx.doi.org/10.1126/science.1142034; PMID: 18048680
- Worman HJ, Bonne G. “Laminopathies”: a wide spectrum of human diseases. Exp Cell Res 2007; 313:2121 - 33; http://dx.doi.org/10.1016/j.yexcr.2007.03.028; PMID: 17467691
- Florens L, Korfali N, Schirmer EC. Subcellular fractionation and proteomics of nuclear envelopes. Methods Mol Biol 2008; 432:117 - 37; http://dx.doi.org/10.1007/978-1-59745-028-7_8; PMID: 18370014
- Florens L, Washburn MP. Proteomic analysis by multidimensional protein identification technology. Methods Mol Biol 2006; 328:159 - 75; PMID: 16785648
- Wu CC, MacCoss MJ, Howell KE, Yates JR 3rd. A method for the comprehensive proteomic analysis of membrane proteins. Nat Biotechnol 2003; 21:532 - 8; http://dx.doi.org/10.1038/nbt819; PMID: 12692561
- McDonald WH, Tabb DL, Sadygov RG, MacCoss MJ, Venable J, Graumann J, et al. MS1, MS2 andSQT-three unified, compact andeasily parsed file formats for the storage of shotgun proteomic spectra and identifications. Rapid Commun Mass Spectrom 2004; 18:2162 - 8; http://dx.doi.org/10.1002/rcm.1603; PMID: 15317041
- Venable JD, Dong MQ, Wohlschlegel J, Dillin A, Yates JR. Automated approach for quantitative analysis of complex peptide mixtures from tandem mass spectra. Nat Methods 2004; 1:39 - 45; http://dx.doi.org/10.1038/nmeth705; PMID: 15782151
- Eng J, McCormack A. Yates Jr. An Approach to Correlate Tandem Mass Spectral Data of Peptides with Amino Acid Sequences in a Protein Database. J Am Soc Mass Spectrom 1994; 5:976 - 89; http://dx.doi.org/10.1016/1044-0305(94)80016-2
- Tabb DL, McDonald WH, Yates JR 3rd. DTASelect and Contrast: tools for assembling and comparing protein identifications from shotgun proteomics. J Proteome Res 2002; 1:21 - 6; http://dx.doi.org/10.1021/pr015504q; PMID: 12643522
- Zybailov BL, Florens L, Washburn MP. Quantitative shotgun proteomics using a protease with broad specificity and normalized spectral abundance factors. Mol Biosyst 2007; 3:354 - 60; http://dx.doi.org/10.1039/b701483j; PMID: 17460794
- Flicek P, Aken BL, Beal K, Ballester B, Caccamo M, Chen Y, et al. Ensembl 2008. Nucleic Acids Res 2008; 36:Database issue D707 - 14; http://dx.doi.org/10.1093/nar/gkm988; PMID: 18000006
- Stajich JE, Block D, Boulez K, Brenner SE, Chervitz SA, Dagdigian C, et al. The Bioperl toolkit: Perl modules for the life sciences. Genome Res 2002; 12:1611 - 8; http://dx.doi.org/10.1101/gr.361602; PMID: 12368254
- Rice P, Longden I, Bleasby A. EMBOSS: the European Molecular Biology Open Software Suite. Trends Genet 2000; 16:276 - 7; http://dx.doi.org/10.1016/S0168-9525(00)02024-2; PMID: 10827456
- Nakai K, Horton P. PSORT: a program for detecting sorting signals in proteins and predicting their subcellular localization. Trends Biochem Sci 1999; 24:34 - 6; http://dx.doi.org/10.1016/S0968-0004(98)01336-X; PMID: 10087920
- Krogh A, Larsson B, von Heijne G, Sonnhammer EL. Predicting transmembrane protein topology with a hidden Markov model: application to complete genomes. J Mol Biol 2001; 305:567 - 80; http://dx.doi.org/10.1006/jmbi.2000.4315; PMID: 11152613