Abstract
There is ample evidence that neoadjuvant chemotherapy of breast carcinoma is particularly efficient if the tumor presents signs of either a pre-existent or therapy-induced anticancer immune response. Antineoplastic chemotherapies are particularly beneficial if they succeed in inducing immunogenic cell death, hence converting the tumor into its own therapeutic vaccine. Immunogenic cell death is characterized by a pre-mortem stress response including endoplasmic reticulum stress and autophagy. Based on these premises, we attempted to identify metagenes that reflect an intratumoral immune response or local stress responses in the transcriptomes of breast cancer patients. No consistent correlations between immune- and stress-related metagenes could be identified across several cohorts of patients, representing a total of 1045 mammary carcinomas. Moreover, few if any, of the stress-relevant metagenes influenced the probability of pathological complete response to chemotherapy. In contrast, several immune-relevant metagenes had a significant positive impact on response rates. This applies in particular to a CXCL13-centered, highly reproducible metagene signature reflecting the intratumoral presence of interferon-γ-producing T cells.
Introduction
Accumulating evidence indicates that pre-therapy anticancer immune responses significantly influence disease progression in breast carcinoma patients.Citation1,Citation2 This corollary has also been perceived in the prognosis of other malignancies,Citation3-Citation5 including colorectal carcinomas.Citation6,Citation7 The infiltration of CD8+ lymphocytes in primary breast cancer lesions is positively associated with both overall and relapse-free survival, especially if the tumors lack immunosuppressive CD68+ macrophages.Citation8-Citation10 Similarly, the absence of regulatory T cells (Tregs) in diagnostic breast cancer biopsies has a positive impact on the long-term fate of patients after adjuvant chemotherapy.Citation11,Citation12 A high frequency of infiltrating lymphocytes detected in diagnostic biopsies also constitutes an independent predictive biomarker for the induction of pathological complete responses (pCRs) in locally advanced breast cancers.Citation13-Citation16 In the neoadjuvant setting, chemotherapeutic responses have been associated with the de novo induction of local immune responses. Thus, an amelioration in the intratumoral T-cell ratio of CD8+ T lymphocytes to FOXP3+ Tregs detected after the first cycle of anthracycline-based chemotherapy is sufficient to predict the occurrence of pCR in response to the full chemotherapeutic regimen of at least 6 cycles.Citation17,Citation18 While most the studies have used immunohistochemical methods to investigate the composition of the immune infiltrate in breast cancer, a few have identified immune-relevant transcripts and gene sets or “metagenes” (composed by several genes expressed in Type 1 T helper (TH1) cells and interferon responses) that predict pCRs in each of the breast cancer subtypes investigated in this respect.Citation19-Citation22
One of the mechanisms that could potentially underlie anticancer immune responses post-chemotherapy is that of so-called immunogenic cell death. Anthracyclines, DNA-damaging agents often used in the treatment of breast cancer, stimulate 2 types of premortem stress responses possibly linked to immunogenic cell death. Namely, these include the endoplasmic reticulum (ER) stress response (also called the “unfolded stress response”) and autophagy consisting of the sequestration and lysosomal degradation of cytoplasmic components.Citation18,Citation23-Citation25 Autophagy is required for the cells to release ATP during the apoptotic blebbing phase, and ATP acts as a potent chemoattractant for myeloid cells, including antigen-presenting dendritic cell precursors.Citation26-Citation28 ER stress is required for the exposure of calreticulin thought to act as an eat-me signal for improving the transfer of tumor antigens to antigen-presenting cells.Citation29 Tumors that are deficient in ER stress or in autophagy fail to elicit immune responses in mice post-chemotherapy and the malignancy becomes incurable.Citation24,Citation25 Similarly, therapeutic agents that fail to induce premortem ER stress and autophagy have limited therapeutic potential.Citation30
Based on these premises, we set out to investigate breast cancer microarray data for signs of ER stress, autophagy, or lysosomal transcriptional perturbations, hoping to establish correlations between parameters of cellular stress and local immune responses. From this analysis, we established a series of gene signatures or “metagenes” reflecting correlated gene expression patterns with special reference to the immune response, the ER stress response, autophagy, or lysosomes. We then interrogated correlated the clinical relevance of these metagenes among each other, in several independent cohorts of breast cancers subjected to neo-adjuvant chemotherapy, and evaluated their impact on patient survival post-chemotherapy. Our results reveal the clinical relevance of several immunity-related metagenes, despite the apparently limited impact on local cellular stress responses.
Results and Discussion
Immune- and stress-related metagenes with a high degree of reproducibility across data sets
Among genes known to relate to the immune system, ER-stress, autophagy, or lysosomes (refer to Materials and Methods for the procedure used in the construction of the gene lists), we determined aggregate patterns of coordinately expressed genes (i.e., metagenes) by hierarchical clustering and principal component analyses of the initial data set of 522 breast cancer microarrays accessible in The Cancer Genome Atlas (TCGA). Thresholds were arbitrarily set so that this operation would yield 18–20 metagenes in each of the 4 categories (Tables S1–4, corresponding to genes associated with immunity, ER-stress, autophagy, or the lysosome, respectively). These metagenes were then re-examined within a series of additional microarray data sets profiling cancer types linked to antitumor immunity. Among these validation data sets, 3 that were obtained were composed of breast cancer patient samples (161 samples collected by Bonnefoi et al.,Citation31 184 samples analyzed by Hatzis et al.,Citation32 and 178 advanced, late-stage carcinoma samples in the collection by Tabchy et al.Citation33). Three other additional validation data sets involved colorectal carcinomas or head and neck cancers. This procedure established a ranking of metagenes based on their reproducibility across multiple distinct data sets and immunity-associated cancer types (). Seven of the immune-related metagenes were judged to be reproducible (reproducibility P value < 0.05 for all data sets except 1). The salient genetic components of each reproducible metagene are shown in . The first (and hence most reproducible) immune metagene “CXCL13,” named according to the highest weighted gene in the signature chemokine (C-X-C motif) ligand 13, mainly reflects the presence of effector T cells within the tumor bed. The second and third metagenes (“CTSS” and “S100A4,” respectively) constitute aggregates of genes typically expressed in myeloid cells. And the subsequent ones (CCL8, CXCL9, CXCL1, CCL19) reflect chemokine-related gene signatures. More generally, most of the genes appearing in the list of salient components contributing to immune-relevant metagenes are related to tumor infiltrates, such as CD8B,Citation34 CD80,Citation35 CD86,Citation35 CTLA4,Citation36 PRF1,Citation37 IL-2,Citation38,Citation39 IL-10,Citation39-Citation41 CCL5,Citation42 CCL2,Citation43 CCL19,Citation44 and CXCL9.Citation45 Four metagenes among each of the 3 stress-related categories (ER-stress, autophagy, and lysosomes) yielded reproducible patterns across all data sets ().
Figure 1.P value of metagene reproducibility. Each of the candidate metagenes per category constructed from The Cancer Genome Atlas (TCGA) cohort (listed in Table S1 and named according to the highest weighted gene in the signature) was revaluated in other cohorts, as indicated, and P values were determined by bootstrapping. Metagenes are ranked left to right according to their reproducibility. Box plots of the P values are shown for each group of metagenes per category. Plain and dashed yellow lines delimit highly- (P value < 0.05 for all cohorts), median- (P value < 0.05 for all cohorts except 1) and non-reproducible metagenes.
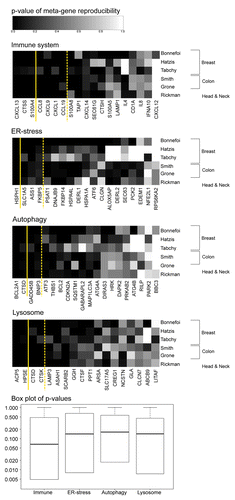
Figure 2. Salient features of reproducible metagenes. Listed are the most important genes contributing to metagenes defined as highly or median-reproducible in . The relative weight of the listed individual mRNA species within each metagene is gray-coded and reflect the correlation coefficient (“component” column of metagene-associated genes listed in Tables S1–4). Shown are only those genes whose coefficient is above 0.14. Genes related to the tumor-associated immune microenvironment are highlighted: red = cytokines, blue = natural killer, green = human leukocyte antigen.
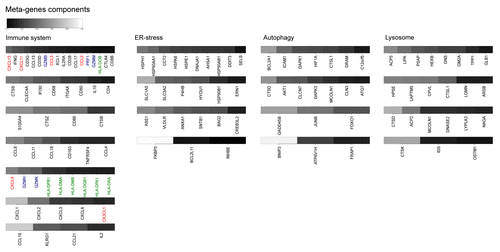
Correlations among immune- and stress-related metagenes
In the subsequent step of this analysis, Pearson’s correlations were calculated between the expression levels of all metagenes, in each of the 4 breast-cancer cohorts studied here (). The immune system-related metagenes yielded preponderantly positive correlations, especially among the most reproducible metagenes (plotted in the upper left corner of each graph in ) and demarcated by the solid yellow lines. No significant negative correlations could be identified among the immune-related genes, indicating that the overall gene expression signature in this category reflects inflammatory and immune reactions as an en bloc (unified) process. These results suggest a failure to identify antagonistic relationships between cells and associated genes reputed to play a positive role in immunosurveillance (such as effector T cells) and immunosuppressive elements (such as myeloid-derived suppressor cells and regulatory T cells). A similar, though less obvious pattern of mostly positive correlations was obtained for lysosome-related metagenes (). In sharp contrast, no clear correlations could be established among ER stress-related metagenes () and autophagy-related genes (). Thus, the principal ER-stress metagene (HSPH1) was not correlated with any of the other reproducible metagenes within this category (SLC1A5, ASS1, FKBP5; ). Moreover, the 2 principal autophagy-related metagenes (BCL2A1, CTSD) were neither correlated with each other nor with the 2 other reproducible metagenes (GADD45B, BNIP3) contained within this category (). Collectively, immune-related and lysosome-related metagenes exhibited more positive internal correlations (higher r-values) than ER stress- and autophagy-related metagenes, especially among the subsets of metagenes that were considered to be reproducible across distinct cancer types (yellow box plots in ). Finally, the reproducibility of the correlations (as evinced by a lower reproducibility P value) across the 4 distinct cohorts of breast cancer patients was also higher for immune-related and lysosome-related metagenes as compared with ER-stress and autophagy-related metagenes (). Altogether, these data suggest that immune-related and lysosome-relevant metagenes reflect rather monolithic processes, while ER-stress and autophagy-related metagenes comprise apparently heterogeneous alterations in gene expression. The relatively low level of correlations among the stress-related metagenes relative to those found among immune-relevant metagenes might also reflect a greater reliance of cellular stress responses on post-transcriptional (rather than transcriptional) changes.
Figure 3. Pearson’s correlations between metagenes within each of the 4 functional categories as determined in 4 distinct breast cancer cohorts. A–D. Reproducibility (P value) calculated based on the variance between metagene data sets within the indicated cohort. E–F. Box plots of correlation matrix (E) elements (or r-values) calculated relative to the TCGA cohort and reproducibility P values (F), either for the complete set of metagenes (in white) or focusing on the subset of reproducible metagenes (in yellow) delimited by yellow lines in A–D (as described in legend). Zero (black) indicates that the pair are uncorrelated, a positive value (red) indicating a correlation and a negative value (green) indicating an inverse relationship.
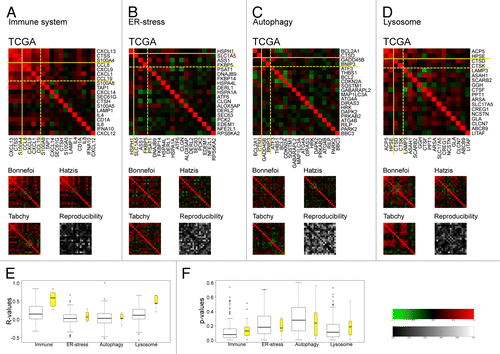
Correlations between immune-related and stress-related metagenes
The principal objective of this study was to explore correlations between immune-related gene expression patterns on one hand and cellular stress responses on the other. Cross correlations between immune- and ER stress-related metagenes were generally weak and poorly reproducible among the 4 breast cancer cohorts with the notable exception of the ER stress-related metagene ALOX5AP (), which regroups 2 pro-inflammatory genes, namely arachidonate 5-lipoxygenase-activating protein (ALOX5AP or FLAP)Citation46 itself and another component of the ALOX5AP metagene G protein-coupled receptor 84 (GPR84), a receptor expressed by immune cells.Citation47 However, the ALOX5AP metagene was not ranked as a reproducible gene across distinct data sets (). The correlation between immune- and autophagy-relevant metagenes was weak as well, with the notable exception of the highly reproducible metagene BCL2A1 (). In contrast, we observed a relatively strong correlation between several immune- and lysosome-related metagenes, especially among the reproducible subsets of metagenes (upper left corner in ). Thus, strong correlations were found between most immune-relevant metagenes and the ACP5 and HPSE metagenes, which both primarily reflect the expression level of lysosomal hydrolases, and the LAMP3 metagene. This may be partly explained by the fact that some lysosomal hydrolases and the lysosomal-associated membrane protein 3 (LAMP3, also called DC-LAMP or CD208) isoform of the LAMP family are strongly expressed by innate immune effectors.Citation48 Altogether, the correlations between metagenes falling into distinct functional categories () tended to be as low as those observed among each of the stress-related categories outside of the immune-related category (compare with ).
Figure 4. Pearson correlations (or r-values) between immune-relevant and stress response-relevant metagenes. A–C. Reproducibility (P value) was calculated based on the variance between data sets and by comparison with a null hypothesis distribution (2000 events). D–E. Box plots (D) of correlation matrix elements (or r-values) calculated relative to the TCGA cohort and reproducibility P values (E) either for the complete set of metagenes (in white) or focusing on reproducible metagenes (in yellow) delimited by yellow lines in A–C.
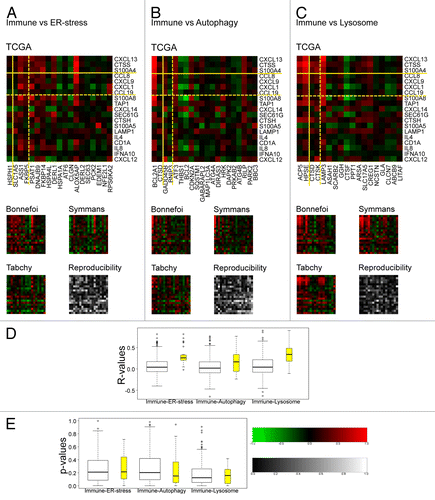
We next turned to another strategy for the identification of possible correlations between immune-related metagenes and cellular stress pathways. Target genes of transcription factors involved in the ER-stress response, including activating transcription factor 4 and 6 (ATF4 and ATF6, respectively), x-box binding protein 1 (XBP1),Citation49 and a regulator of lysosomal/autophagic biogenesis transcription factor EB (TFEB)Citation50 were listed together with hypoxia-inducible factor 1 α subunit (HIF1α) and the pro-inflammatory nuclear factor-κB (NF-κB) as controls. These lists were then used to interrogate the TCGA breast cancer cohort and 5 metagenes corresponding to each of these transcription factors were identified (Table S5). Again, as previously performed for immune- and stress-related metagenes, these transcription-factor associated metagenes were ranked for reproducibility in distinct immune-implicated cancer types (upper panels in and Fig. S1). Subsequently, as shown in (middle panels), these novel metagenes were correlated with the 19 immune-related metagenes initially identified (; Table S1). Finally, these correlations were evaluated for reproducibility within the 4 breast cancer cohorts (lower panels in ). We found that among the ATF4 target metagenes (), 2 (PSAT1 and PTX3) positively correlated with the immune-related metagenes with a high degree of reproducibility, whereas 1 metagene (STC2) reproducibly exhibited a negative correlation with the immune-related metagenes. Among the ATF6 target genes (), only the IL6 metagene, dominated by the gene coding for the pro-inflammatory cytokine interleukin-6, exhibited a highly reproducible positive correlation with immune-related metagenes, whereas 1 metagene (XBP1) negatively correlated with some immune metagenes, albeit with a low level reproducibility. Among XBP1 target genes (), again an IL6-centered metagene (dominated by the genes coding for IL-6, cyclin A1, and RANK ligand) exhibited a positive correlation with immune genes across all 4 breast cancer cohorts. Another XPB1 target metagene (), POU2AF1, comprising several immune-relevant genes, including vascular cell adhesion molecule-1 (VCAM1) activation-induced cytidine deaminase (AICDA) the cell death receptor FAS/APO-1/CD95, the intercellular adhesion molecule-1 (ICAM1), and FK506-binding protein-11 (FKB11), among others, correlated positively with immune-related metagenes in 3 out of 4 breast cancer cohorts. In contrast, an estrogen receptor-1 (ESR1)-dominated XBP1 target metagene negatively correlated with immune-metagenes, confirming the previous observation that estrogen receptor is linked to a reduced infiltration of CD8+ T cells into tumors.Citation10 No significant correlations were detectable between TFEB target metagenes and immune-related metagenes (). Among our controls, we also found 1 HIF1α target metagene (MMP1) and 2 NFκB1 target metagenes (IL-6 and PTX3) that positively correlated with immune metagenes (Fig. S1), likely reflecting the presence of target genes of these factors that are preponderantly expressed by immune cells (Table S5).
Figure 5. Metagenes derived from target genes of stress-responsive transcription factors. A–G. Analysis of metagenes (see Table S5) derived from the downstream targets of ATF4 (A), ATF6 (B), XBP1 (C) and TFEB (D) transcription factors. A–D. Upper panels indicate the P value of metagene reproducibility in 6 distinct cancer cohorts (refer to legend for details). Middle panels display Pearson’s correlations (or r-values) between immune-relevant metagenes and each of the new metagenes within the TCGA cohort. Yellow lines delimit different levels of reproducibility as described in . Lower panels summarize the reproducibility among additional breast cancer cohorts (similar to ). E–G. Box plots of p-value reproducibility (E; derived from the upper panels in A–D), correlation matrix elements (or r-values) calculated relative to the TCGA cohort (F; derived from the middle panels in A–D) and correlation reproducibility (G; derived from the gray-shaded graphs in the lower panels in A–D).
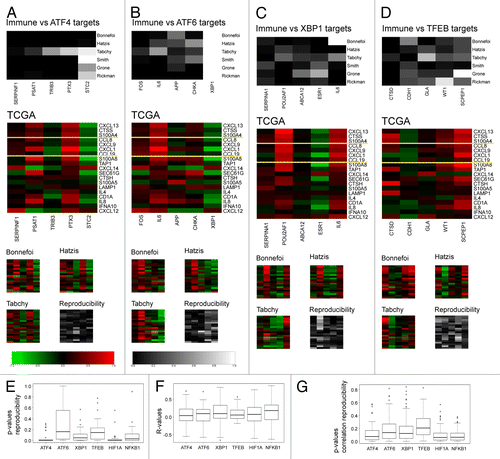
Altogether, it appears that some metagenes of transcription factor targets related to ER stress (ATF4, ATF6, XBP1) correlated with some degree with immune-related metagenes (which is not the case for TFEB target metagenes), although these correlations are comparable to those observed with HIF1α and NFKB1 target metagenes (). Moreover, the reproducibility of ER or lysosome/autophagy-related metagenes is not superior to that observed for HIF1α and NFKB1 target metagenes ().
Prognostic values of immune- and stress-related metagenes across distinct data sets
The ultimate goal of such cancer microarray analyses is their clinical application to prospectively identify biomarkers that can predict the natural progression of the disease or clinical responses to chemotherapy. Therefore, we evaluated the impact of each metagene described in this report on the clinical response of the 3 cohorts for which such data are available. All 3 cohorts received neo-adjuvant chemotherapy, and pathological complete responses (pCR) were evaluated following surgical resection of the tumors. The Bonnefoi collection of breast cancers were locally invasive estrogen receptor-negative carcinomas treated with anthracycline-based neoadjuvant chemotherapy (either epirubicin + fluorouracil + cyclophosphamide or epiribucin plus docetaxel).Citation31 The Hatzis cohort comprised ERBB2-negative breast cancers that received neoadjuvant chemotherapy based on anthracyclines plus either paclitaxel or docetaxel (followed by endocrine therapy if estrogen receptor-positive).Citation32 The Tabchy cohort received neoadjuvant chemotherapy involving fluorouracil plus epirubicin plus cyclophosphamide (FEC) or fluorouracil plus doxorubicin plus cyclophosphamide (FAC), alone or combined with taxanes.Citation33 Among the metagenes that have been defined in this report, rather few correlated with the chemotherapeutic response. Included in this rare subset was the immune-related CXCL13 metagene that exhibited a strong positive correlation with pCR (combined P value of 0.005), and to some degree to CCL8 (combined P value of 0.02) and CXCL9 (combined P value of 0.05), both of which also exhibited positive correlations with pCR although with lower reproducibility than that of the CXCL13 metagene (). Among the ER-stress-related, autophagy-related, and lysosome-related metagenes () no reproducible positive correlations could be identified with the notable exception of the LAMP3 metagene, (combined P value of 0.0002), which most likely reflects the presence of LAMP3-expressing dendritic cells in the tumor bed. Other metagenes could only acquire significant combined P values if their impact on patient survival was measurable in all patient cohorts. This applies to several ER stress-related metagenes (HSPA1A, CLGN, PCK2, NFE2L1) but to only 1 autophagy- (THBS1) and 3 lysosome- (ASAH1, ARSA, GLA) related metagenes. Moreover, no significant correlations were found between the stress-related transcription factor metagenes and patient survival in any of the individual cohorts. Furthermore, it was only after calculation of combined P values for all 4 cohorts that some transcription factor-related metagenes (STC2, XBP1, SERPINA1, ESR1, CDH1, GLA) appeared to correlate with the therapeutic response (; Fig. S1). In conclusion, the “best” metagene predicting pCR after neoadjuvant breast cancer chemotherapy is the CXCL13 metagene that was also the most reproducible metagene across different breast cancer cohorts and immune-implicated cancer types.
Figure 6.A–H. Heat map of significance of differential metagene expression between chemotherapy responding and non-responding patients. Signed log P values were computed for each immune (A), ER-stress (B), autophagy (C) or lysosomal (D) response-relevant metagenes (yellow lines delimit different levels of reproducibility as determined in Figure 1,or transcription factor-determined (E–H) metagenes by one-tailed, unpaired Student’s t tests to compare the transcript levels in tumors that underwent complete pathological responses (cPR) with those that failed to respond to chemotherapy. This calculation was done for each cohort and treatment option separately. The “combined” rows represent the signed log P value obtained by the aggregation of the individual data set values by means of Fisher’s method. The color scale represents log (base 10) of P values, with a positive or negative sign to indicate over (red) or under (green) expression, respectively, in samples from patients with cPR relative to samples from non-responders.
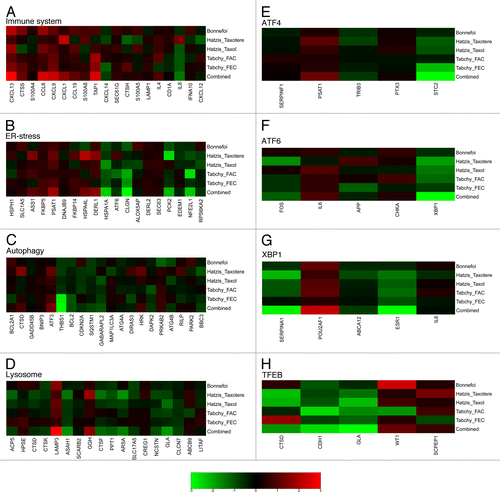
Concluding Remarks
The present study confirms the idea that the expression level of select immune response-related mRNA species may constitute a robust biomarker with the potential to predict chemotherapeutic responses in breast cancer.Citation21 In particular 1 metagene (CXCL13) composed by several genes encoding various chemokines (in decreasing order of importance: CXCL13, CXCL11, CCL12, CLL5, XCL1, CCL17, CCL2, CCL23, CXCL10), interferon-γ (IFNγ), components of the T cell receptor/CD3 complex (CD3G, CD3D, CD3E), a cytotoxic T cell marker (CD8B), a general T cell marker (CD7), the T cell activation marker (CD25A, CTLA4), the co-activation receptor CD28, several components of cytotoxic granules such as perforin-1 (PRF1) and the granzymes B (GZMB) and M (GZMM), as well as the dendritic cell activation marker CD83 (Table S1). Hence, this metagene likely relates to the presence of IFNγ-producing cytotoxic T lymphocytes, a cell type that has previously been reported to play a major role in immunosurveillance.Citation51,Citation52 This particular metagene was the most reproducible one among all immune-related metagenes identified in this study. In addition, constituents of this metagene have been identified as major players in immune cell tumor infiltration in colorectal cancer, such as has been reported for CXCL13,Citation53 CXCL10,Citation54 and CD8B.Citation55
Contrasting with the high degree of clinical relevance and reproducibility of immune-related metagenes, we found that metagenes related to cellular stress responses exhibited a relatively poor degree of reproducibility and failed to predict clinical responses. There are a number of plausible explanations that may underlie this observation. First, stress responses may occur in a transient and spatially limited fashion, despite potentially durable effects on cognate immune responses. Second, although stress responses do impact the transcriptome due to the activation of stress-elicited transcription factors, post-transcriptional adaptations are known to play a major role in the response to stress, as exemplified by eIF2α phosphorylation and dephosphorylation reactions regulation translation in the unfolded protein response.Citation56 Similar post-translational regulatory mechanisms include the acute activation of autophagy by cytoplasmic protein (de)-phosphorylation and (de)-acetylation reactions.Citation57 Hence, the transient nature of stress responses, as well as their limited impact on transcription, may explain the absence of robust interactions between immune-related and stress-related metagenes. Indeed, the few interactions that we detected can be explained by the fact that several stress-related metagenes (such as LAMP3) included genes that are preferentially expressed by immune cells.
If microarray analyses constitute a suboptimal tool for exploring stress responses within tissues, it will be important to explore alternative technologies for measuring such stress responses. Thus, a proteomic approach may offer distinct technical advantages, such as immunohistochemical-based monitoring of eIF2α phosphorylation as a parameter of the ER stress response, an event found to correlate with T cell response within breast cancer tissues post-chemotherapy.Citation18 Similarly, autophagy can be detected immunohistochemically by measuring the aggregation of LC3B cytosolic protein in autophagosomes.Citation58 It will be important to apply such protein-based methods to further establish the chronological, spatial, and clinical correlations between stress responses and anticancer immunosurveillance.
Materials and Methods
Data sets
We used 4 breast cancer data sets for this work. The first one, “TCGA” (downloaded from TCGA website cancergenome.nih.gov), was used as a basis for the construction of meta-genes. The 3 others were chosen because the information regarding patient’s response to treatment was accessible and included: “Bonnefoi”Citation31 GSE6861, “Tabchy”Citation33 GSE20271, and “Hatzis”Citation32 GSE25065. We add 2 data sets of colorectal cancer (“Smith”Citation59,Citation60 GSE17536 and “Grone”Citation61 GSE18088) and 1 of head and neck cancer (“Rickman”Citation62 E-TABM-302) to study the applicability of metagenes to other cancer types ().
For each data set, normalized data were downloaded (except for the Rickman data set for which RMA normalization was applied). For Affymetrix technology, we considered only the best probesets according to Jetset.Citation63
The entire compliment of patients within each data set was generally considered for metagene construction and metagene reproducibility, correlation matrices and correlation matrix reproducibility calculations. In the analysis of metagene correlation with therapeutic response, distinct subgroups of patients were defined according to their inclusion in a particular therapeutic regimen ().
All computations for data analysis (e.g., normalization, statistical tests and figure drawing) were done within R.Citation64
Genes included in distinct functional groups
For the group of immune-related genes, a series of genes specifically expressed in immune cells was complemented by the inclusion of a complete set of genes encoding interferons, cytokines, chemokines and HLA genes (135 genes in total, as indicated in Table S1).
Groups of stress-related genes were extracted from previous publications on ER stress (81 genes, Table S2),Citation65 autophagy (86 genes, Table S3),Citation66 and lysosomes (88 genes, Table S4),Citation67 following removal of (most of) the genes overlapping with the immune-related group. Genes modulated by the transcription factors ATF4 (177 genes), ATF6 (29 genes), XBP1 (173 genes), TFEB (26 genes), HIF1A (299 genes), and NFKB1 (177 genes) were obtained from the IPA database (Ingenuity® Systems, www.ingenuity.com, Table S5), and again genes listed in the immune-related group were removed during this process.
Metagene construction
A metagene was defined as an aggregate of genes whose expression correlates in a series of samples.Citation68 Sets of genes comprising metagenes were obtained by hierarchical clustering of the TCGA data set, fixing the number of metagenes (20 for stress related groups, 5 for transcription factor targets) using the R-functions hclust and cutree to generate the correlation matrix. Gene coefficients inside a meta-gene (Tables S1–4 and Supplementary Material) were obtained by performing principal component analysis on covariance of expression within the set of genes that constituted each metagene using princomp in R. For each metagene, the first principal component was extracted and used as the gene coefficient.
Reproducibility of metagenes
The reproducibility of a metagene () between several data sets was obtained by comparing TCGA with the other breast cancer data sets. More precisely, to calculate reproducibility for a given metagene, in a given data set, a multistep process was chosen and applied as follows:
1. Construct the set of coefficients within the given data set as the first principal component by applying the same procedure as for the initial TCGA-metagene construction, except that the data set to be used does not originate from TCGA.
2. Measure the angle between the coefficient set constructed in 1) with the one the TCGA-defined metagene. Extract the angle from the scalar product between these 2 sets considered as 2 vectors in the vector space of gene components. If the expression of a given gene was not measured in a data set, remove that component when measuring the angle (reducing the dimension of the vector space). If no components remain, the metagene is removed (this occurred in the lysosome-relevant group of genes).
3. Compute the significance of the angle by comparing the computed value with the null hypothesis distribution obtained by randomly selecting (n = 2000) gene sets within the given group (Immune-related genes, ER stress genes, etc.) of identical length compared with the given metagene and computating the angle as described in steps 1 and 2.
Correlation matrices
The correlation matrices of – represent the Pearson’s correlation coefficient of metagene expression with zero (black) indicating the pair are uncorrelated, a positive value (red) indicating a correlation and a negative value (green) indicating an inverse relationship. Covariance matrices are provided in Supplementary Material.
Reproducibility of correlation matrices
Because there is no consensus for measuring correlation reproducibility in statistics, we developed our own method by applying a bootstrapping procedure on the variance of each correlation matrix elements. More precisely, for each matrix element, we computed the variance among the different data sets. We associated a P value by constructing a null hypothesis distribution (2000 events) by shuffling metagenes independently between data sets.
Correlation with treatment responses
Correlations with therapeutic responses were calculated for the 3 breast cancer cohorts for which treatment responses were publicly available. In order to accomplish this, expression of each metagene in each sample was first calculated using gene coefficients. Next, for each metagene per data set, unpaired Student’s t tests were performed (one-tailed, for both over- and under-expression alternative hypotheses), comparing the gene coefficient of tumors with or without cPR. For each metagene, the combined P values were then calculated by means of Fisher’s method for combining probability tests. The log(10) of the smallest P value (from the 2 alternative hypotheses) was chosen with positive and negative signs representing over- and under-expression, respectively, in samples from patients that exhibit cPR relative to patients without cPR. The absolute levels of expression are represented by multiple boxplots in Figure S2.
Abbreviations: | ||
ALOX5AP | = | arachidonate 5-lipoxygenase-activating protein |
ATF4 | = | activating transcription factor 4 |
ATF6 | = | activating transcription factor 6 |
CXCL13 | = | chemokine (C-X-C motif) ligand 13 |
ER | = | endoplasmic reticulum |
FAC | = | fluorouracil plus doxorubicin plus cyclophosphamide |
FEC | = | fluorouracil plus epirubicin plus cyclophosphamide |
HIF1α | = | hypoxia-inducible factor 1α |
LAMP3 | = | lysosomal-associated membrane protein |
pCR | = | pathological complete response |
TCGA | = | The Cancer Genome Atlas |
TH1 | = | Type 1 T helper |
Treg | = | regulatory T cell |
XBP1 | = | x-box binding protein 1 |
Additional material
Download Zip (548.1 KB)Disclosure of Potential Conflicts of Interest
No potential conflicts of interest were disclosed.
Acknowledgments
G.K. is supported by the Ligue contre le Cancer (équipe labelisée); Agence National de la Recherche (ANR); Association pour la recherche sur le cancer (ARC); Cancéropôle Ile-de-France; Institut National du Cancer (INCa); Fondation Bettencourt-Schueller; Fondation de France; Fondation pour la Recherche Médicale (FRM); the European Commission (ArtForce); the European Research Council (ERC); the LabEx Immuno-Oncology; the SIRIC Stratified Oncology Cell DNA Repair and Tumor Immune Elimination (SOCRATE); the SIRIC Cancer Research and Personalized Medicine (CARPEM); and the Paris Alliance of Cancer Research Institutes (PACRI). We thank also TCGA Research Network for generating TCGA data sets.
Citation: Stoll G, Enot D, Mlecnik B, Galon J, Zitvogel L, Kroemer G. Immune-related gene expression patterns predicting the outcome of neoadjuvant chemotherapy. OncoImmunology 2014; 3:e27884; 10.4161/onci.27884
References
- Zitvogel L, Kepp O, Kroemer G. Immune parameters affecting the efficacy of chemotherapeutic regimens. Nat Rev Clin Oncol 2011; 8:151 - 60; http://dx.doi.org/10.1038/nrclinonc.2010.223; PMID: 21364688
- Zitvogel L, Galluzzi L, Smyth MJ, Kroemer G. Mechanism of action of conventional and targeted anticancer therapies: reinstating immunosurveillance. Immunity 2013; 39:74 - 88; http://dx.doi.org/10.1016/j.immuni.2013.06.014; PMID: 23890065
- Angell H, Galon J. From the immune contexture to the Immunoscore: the role of prognostic and predictive immune markers in cancer. Curr Opin Immunol 2013; 25:261 - 7; http://dx.doi.org/10.1016/j.coi.2013.03.004; PMID: 23579076
- Fridman WH, Pagès F, Sautès-Fridman C, Galon J. The immune contexture in human tumours: impact on clinical outcome. Nat Rev Cancer 2012; 12:298 - 306; http://dx.doi.org/10.1038/nrc3245; PMID: 22419253
- Galon J, Angell HK, Bedognetti D, Marincola FM. The continuum of cancer immunosurveillance: prognostic, predictive, and mechanistic signatures. Immunity 2013; 39:11 - 26; http://dx.doi.org/10.1016/j.immuni.2013.07.008; PMID: 23890060
- Galon J, Costes A, Sanchez-Cabo F, Kirilovsky A, Mlecnik B, Lagorce-Pagès C, Tosolini M, Camus M, Berger A, Wind P, et al. Type, density, and location of immune cells within human colorectal tumors predict clinical outcome. Science 2006; 313:1960 - 4; http://dx.doi.org/10.1126/science.1129139; PMID: 17008531
- Galon J, Fridman W-H, Pagès F. The adaptive immunologic microenvironment in colorectal cancer: a novel perspective. Cancer Res 2007; 67:1883 - 6; http://dx.doi.org/10.1158/0008-5472.CAN-06-4806; PMID: 17332313
- DeNardo DG, Brennan DJ, Rexhepaj E, Ruffell B, Shiao SL, Madden SF, Gallagher WM, Wadhwani N, Keil SD, Junaid SA, et al. Leukocyte complexity predicts breast cancer survival and functionally regulates response to chemotherapy. Cancer Discov 2011; 1:54 - 67; http://dx.doi.org/10.1158/2159-8274.CD-10-0028; PMID: 22039576
- Mahmoud SMA, Paish EC, Powe DG, Macmillan RD, Grainge MJ, Lee AH, Ellis IO, Green AR. Tumor-infiltrating CD8+ lymphocytes predict clinical outcome in breast cancer. J Clin Oncol 2011; 29:1949 - 55; http://dx.doi.org/10.1200/JCO.2010.30.5037; PMID: 21483002
- Mahmoud S, Lee A, Ellis I, Green A. CD8(+) T lymphocytes infiltrating breast cancer: A promising new prognostic marker?. Oncoimmunology 2012; 1:364 - 5; http://dx.doi.org/10.4161/onci.18614; PMID: 22737616
- Bates GJ, Fox SB, Han C, Leek RD, Garcia JF, Harris AL, Banham AH. Quantification of regulatory T cells enables the identification of high-risk breast cancer patients and those at risk of late relapse. J Clin Oncol 2006; 24:5373 - 80; http://dx.doi.org/10.1200/JCO.2006.05.9584; PMID: 17135638
- Gobert M, Treilleux I, Bendriss-Vermare N, Bachelot T, Goddard-Leon S, Arfi V, Biota C, Doffin AC, Durand I, Olive D, et al. Regulatory T cells recruited through CCL22/CCR4 are selectively activated in lymphoid infiltrates surrounding primary breast tumors and lead to an adverse clinical outcome. Cancer Res 2009; 69:2000 - 9; http://dx.doi.org/10.1158/0008-5472.CAN-08-2360; PMID: 19244125
- Denkert C, Loibl S, Noske A, Roller M, Müller BM, Komor M, Budczies J, Darb-Esfahani S, Kronenwett R, Hanusch C, et al. Tumor-associated lymphocytes as an independent predictor of response to neoadjuvant chemotherapy in breast cancer. J Clin Oncol 2010; 28:105 - 13; http://dx.doi.org/10.1200/JCO.2009.23.7370; PMID: 19917869
- Loi S. Tumor-infiltrating lymphocytes, breast cancer subtypes and therapeutic efficacy. Oncoimmunology 2013; 2:e24720; http://dx.doi.org/10.4161/onci.24720; PMID: 24073365
- Loi S, Sirtaine N, Piette F, Salgado R, Viale G, Van Eenoo F, Rouas G, Francis P, Crown JP, Hitre E, et al. Prognostic and predictive value of tumor-infiltrating lymphocytes in a phase III randomized adjuvant breast cancer trial in node-positive breast cancer comparing the addition of docetaxel to doxorubicin with doxorubicin-based chemotherapy: BIG 02-98. J Clin Oncol 2013; 31:860 - 7; http://dx.doi.org/10.1200/JCO.2011.41.0902; PMID: 23341518
- West NR, Milne K, Truong PT, Macpherson N, Nelson BH, Watson PH. Tumor-infiltrating lymphocytes predict response to anthracycline-based chemotherapy in estrogen receptor-negative breast cancer. Breast Cancer Res 2011; 13:R126; http://dx.doi.org/10.1186/bcr3072; PMID: 22151962
- Ladoire S, Mignot G, Dabakuyo S, Arnould L, Apetoh L, Rébé C, Coudert B, Martin F, Bizollon MH, Vanoli A, et al. In situ immune response after neoadjuvant chemotherapy for breast cancer predicts survival. J Pathol 2011; 224:389 - 400; http://dx.doi.org/10.1002/path.2866; PMID: 21437909
- Senovilla L, Vitale I, Martins I, Tailler M, Pailleret C, Michaud M, Galluzzi L, Adjemian S, Kepp O, Niso-Santano M, et al. An immunosurveillance mechanism controls cancer cell ploidy. Science 2012; 337:1678 - 84; http://dx.doi.org/10.1126/science.1224922; PMID: 23019653
- Ascierto ML, Kmieciak M, Idowu MO, Manjili R, Zhao Y, Grimes M, Dumur C, Wang E, Ramakrishnan V, Wang XY, et al. A signature of immune function genes associated with recurrence-free survival in breast cancer patients. Breast Cancer Res Treat 2012; 131:871 - 80; http://dx.doi.org/10.1007/s10549-011-1470-x; PMID: 21479927
- Desmedt C, Haibe-Kains B, Wirapati P, Buyse M, Larsimont D, Bontempi G, Delorenzi M, Piccart M, Sotiriou C. Biological processes associated with breast cancer clinical outcome depend on the molecular subtypes. Clin Cancer Res 2008; 14:5158 - 65; http://dx.doi.org/10.1158/1078-0432.CCR-07-4756; PMID: 18698033
- Ignatiadis M, Singhal SK, Desmedt C, Haibe-Kains B, Criscitiello C, Andre F, Loi S, Piccart M, Michiels S, Sotiriou C. Gene modules and response to neoadjuvant chemotherapy in breast cancer subtypes: a pooled analysis. J Clin Oncol 2012; 30:1996 - 2004; http://dx.doi.org/10.1200/JCO.2011.39.5624; PMID: 22508827
- Yau C, Esserman L, Moore DH, Waldman F, Sninsky J, Benz CC. A multigene predictor of metastatic outcome in early stage hormone receptor-negative and triple-negative breast cancer. Breast Cancer Res 2010; 12:R85; http://dx.doi.org/10.1186/bcr2753; PMID: 20946665
- Kroemer G, Galluzzi L, Kepp O, Zitvogel L. Immunogenic cell death in cancer therapy. Annu Rev Immunol 2013; 31:51 - 72; http://dx.doi.org/10.1146/annurev-immunol-032712-100008; PMID: 23157435
- Michaud M, Martins I, Sukkurwala AQ, Adjemian S, Ma Y, Pellegatti P, Shen S, Kepp O, Scoazec M, Mignot G, et al. Autophagy-dependent anticancer immune responses induced by chemotherapeutic agents in mice. Science 2011; 334:1573 - 7; http://dx.doi.org/10.1126/science.1208347; PMID: 22174255
- Panaretakis T, Kepp O, Brockmeier U, Tesniere A, Bjorklund AC, Chapman DC, Durchschlag M, Joza N, Pierron G, van Endert P, et al. Mechanisms of pre-apoptotic calreticulin exposure in immunogenic cell death. EMBO J 2009; 28:578 - 90; http://dx.doi.org/10.1038/emboj.2009.1; PMID: 19165151
- Ma Y, Galluzzi L, Zitvogel L, Kroemer G. Autophagy and cellular immune responses. Immunity 2013; 39:211 - 27; http://dx.doi.org/10.1016/j.immuni.2013.07.017; PMID: 23973220
- Martins I, Wang Y, Michaud M, Ma Y, Sukkurwala AQ, Shen S, Kepp O, Métivier D, Galluzzi L, Perfettini JL, et al. Molecular mechanisms of ATP secretion during immunogenic cell death. Cell Death Differ 2014; 21:79 - 91; http://dx.doi.org/10.1038/cdd.2013.75; PMID: 23852373
- Wang Y, Wang XN. Cell-in-cell: A virgin land of cell biology. Oncoimmunology 2013; 2:e25988; http://dx.doi.org/10.4161/onci.25988; PMID: 24244895
- Obeid M, Tesniere A, Ghiringhelli F, Fimia GM, Apetoh L, Perfettini JL, Castedo M, Mignot G, Panaretakis T, Casares N, et al. Calreticulin exposure dictates the immunogenicity of cancer cell death. Nat Med 2007; 13:54 - 61; http://dx.doi.org/10.1038/nm1523; PMID: 17187072
- Menger L, Vacchelli E, Adjemian S, Martins I, Ma Y, Shen S, Yamazaki T, Sukkurwala AQ, Michaud M, Mignot G, et al. Cardiac glycosides exert anticancer effects by inducing immunogenic cell death. Sci Transl Med 2012; 4:143ra99; http://dx.doi.org/10.1126/scitranslmed.3003807; PMID: 22814852
- Bonnefoi H, Potti A, Delorenzi M, Mauriac L, Campone M, Tubiana-Hulin M, Petit T, Rouanet P, Jassem J, Blot E, et al. Validation of gene signatures that predict the response of breast cancer to neoadjuvant chemotherapy: a substudy of the EORTC 10994/BIG 00-01 clinical trial. Lancet Oncol 2007; 8:1071 - 8; http://dx.doi.org/10.1016/S1470-2045(07)70345-5; PMID: 18024211
- Hatzis C, Pusztai L, Valero V, Booser DJ, Esserman L, Lluch A, Vidaurre T, Holmes F, Souchon E, Wang H, et al. A genomic predictor of response and survival following taxane-anthracycline chemotherapy for invasive breast cancer. JAMA 2011; 305:1873 - 81; http://dx.doi.org/10.1001/jama.2011.593; PMID: 21558518
- Tabchy A, Valero V, Vidaurre T, Lluch A, Gomez H, Martin M, Qi Y, Barajas-Figueroa LJ, Souchon E, Coutant C, et al. Evaluation of a 30-gene paclitaxel, fluorouracil, doxorubicin, and cyclophosphamide chemotherapy response predictor in a multicenter randomized trial in breast cancer. Clin Cancer Res 2010; 16:5351 - 61; http://dx.doi.org/10.1158/1078-0432.CCR-10-1265; PMID: 20829329
- Lesokhin AM, Merghoub T, Wolchok JD. Myeloid-derived suppressor sells and the efficacy of CD8(+) T-cell immunotherapy. Oncoimmunology 2013; 2:e22764; http://dx.doi.org/10.4161/onci.22764; PMID: 23525353
- Ming Lim C, Stephenson R, Salazar AM, Ferris RL. TLR3 agonists improve the immunostimulatory potential of cetuximab against EGFR(+) head and neck cancer cells. Oncoimmunology 2013; 2:e24677; http://dx.doi.org/10.4161/onci.24677; PMID: 23894722
- Demaria S, Pilones KA, Formenti SC, Dustin ML. Exploiting the stress response to radiation to sensitize poorly immunogenic tumors to anti-CTLA-4 treatment. Oncoimmunology 2013; 2:e23127; http://dx.doi.org/10.4161/onci.23127; PMID: 23802063
- Trapani JA, Thia KY, Andrews M, Davis ID, Gedye C, Parente P, Svobodova S, Chia J, Browne K, Campbell IG, et al. Human perforin mutations and susceptibility to multiple primary cancers. Oncoimmunology 2013; 2:e24185; http://dx.doi.org/10.4161/onci.24185; PMID: 23734337
- Vacca P, Martini S, Mingari MC, Moretta L. NK cells from malignant pleural effusions are potent antitumor effectors: A clue for adoptive immunotherapy?. Oncoimmunology 2013; 2:e23638; http://dx.doi.org/10.4161/onci.23638; PMID: 23734317
- Lorvik KB, Haabeth OAW, Clancy T, Bogen B, Corthay A. Molecular profiling of tumor-specific TH1 cells activated in vivo. Oncoimmunology 2013; 2:e24383; http://dx.doi.org/10.4161/onci.24383; PMID: 23762808
- Lindenberg JJ, van de Ven R, Lougheed SM, Zomer A, Santegoets SJ, Griffioen AW, Hooijberg E, van den Eertwegh AJ, Thijssen VL, Scheper RJ, et al. Functional characterization of a STAT3-dependent dendritic cell-derived CD14(+) cell population arising upon IL-10-driven maturation. Oncoimmunology 2013; 2:e23837; http://dx.doi.org/10.4161/onci.23837; PMID: 23734330
- Sung W-W, Lee H. The role of interleukin-10 in the progression of human papillomavirus-associated lung carcinoma. Oncoimmunology 2013; 2:e25854; http://dx.doi.org/10.4161/onci.25854; PMID: 24244909
- Velasco-Velázquez M, Pestell RG. The CCL5/CCR5 axis promotes metastasis in basal breast cancer. Oncoimmunology 2013; 2:e23660; http://dx.doi.org/10.4161/onci.23660; PMID: 23734321
- Li M, Knight DA, A Snyder L, Smyth MJ, Stewart TJ. A role for CCL2 in both tumor progression and immunosurveillance. Oncoimmunology 2013; 2:e25474; http://dx.doi.org/10.4161/onci.25474; PMID: 24073384
- Wong JL, Muthuswamy R, Bartlett DL, Kalinski P. IL-18-based combinatorial adjuvants promote the intranodal production of CCL19 by NK cells and dendritic cells of cancer patients. Oncoimmunology 2013; 2:e26245; http://dx.doi.org/10.4161/onci.26245; PMID: 24228233
- Guirnalda P, Wood L, Goenka R, Crespo J, Paterson Y. Interferon γ-induced intratumoral expression of CXCL9 alters the local distribution of T cells following immunotherapy with Listeria monocytogenes. Oncoimmunology 2013; 2:e25752; http://dx.doi.org/10.4161/onci.25752; PMID: 24083082
- Kennedy BP, Diehl RE, Boie Y, Adam M, Dixon RA. Gene characterization and promoter analysis of the human 5-lipoxygenase-activating protein (FLAP). J Biol Chem 1991; 266:8511 - 6; PMID: 1673682
- Wang J, Wu X, Simonavicius N, Tian H, Ling L. Medium-chain fatty acids as ligands for orphan G protein-coupled receptor GPR84. J Biol Chem 2006; 281:34457 - 64; http://dx.doi.org/10.1074/jbc.M608019200; PMID: 16966319
- Karthaus N, Torensma R, Tel J. Deciphering the message broadcast by tumor-infiltrating dendritic cells. Am J Pathol 2012; 181:733 - 42; http://dx.doi.org/10.1016/j.ajpath.2012.05.012; PMID: 22796439
- Kim R, Emi M, Tanabe K, Murakami S. Role of the unfolded protein response in cell death. Apoptosis 2006; 11:5 - 13; http://dx.doi.org/10.1007/s10495-005-3088-0; PMID: 16374548
- Settembre C, Fraldi A, Medina DL, Ballabio A. Signals from the lysosome: a control centre for cellular clearance and energy metabolism. Nat Rev Mol Cell Biol 2013; 14:283 - 96; http://dx.doi.org/10.1038/nrm3565; PMID: 23609508
- Schreiber RD, Old LJ, Smyth MJ. Cancer immunoediting: integrating immunity’s roles in cancer suppression and promotion. Science 2011; 331:1565 - 70; http://dx.doi.org/10.1126/science.1203486; PMID: 21436444
- Vesely MD, Kershaw MH, Schreiber RD, Smyth MJ. Natural innate and adaptive immunity to cancer. Annu Rev Immunol 2011; 29:235 - 71; http://dx.doi.org/10.1146/annurev-immunol-031210-101324; PMID: 21219185
- Bindea G, Mlecnik B, Tosolini M, Kirilovsky A, Waldner M, Obenauf AC, Angell H, Fredriksen T, Lafontaine L, Berger A, et al. Spatiotemporal dynamics of intratumoral immune cells reveal the immune landscape in human cancer. Immunity 2013; 39:782 - 95; http://dx.doi.org/10.1016/j.immuni.2013.10.003; PMID: 24138885
- Mlecnik B, Tosolini M, Charoentong P, Kirilovsky A, Bindea G, Berger A, Camus M, Gillard M, Bruneval P, Fridman WH, et al. Biomolecular network reconstruction identifies T-cell homing factors associated with survival in colorectal cancer. Gastroenterology 2010; 138:1429 - 40; http://dx.doi.org/10.1053/j.gastro.2009.10.057; PMID: 19909745
- Mlecnik B, Tosolini M, Kirilovsky A, Berger A, Bindea G, Meatchi T, Bruneval P, Trajanoski Z, Fridman WH, Pagès F, et al. Histopathologic-based prognostic factors of colorectal cancers are associated with the state of the local immune reaction. J Clin Oncol 2011; 29:610 - 8; http://dx.doi.org/10.1200/JCO.2010.30.5425; PMID: 21245428
- Harding HP, Zhang Y, Zeng H, Novoa I, Lu PD, Calfon M, Sadri N, Yun C, Popko B, Paules R, et al. An integrated stress response regulates amino acid metabolism and resistance to oxidative stress. Mol Cell 2003; 11:619 - 33; http://dx.doi.org/10.1016/S1097-2765(03)00105-9; PMID: 12667446
- Kroemer G, Mariño G, Levine B. Autophagy and the integrated stress response. Mol Cell 2010; 40:280 - 93; http://dx.doi.org/10.1016/j.molcel.2010.09.023; PMID: 20965422
- Ladoire S, Chaba K, Martins I, Sukkurwala AQ, Adjemian S, Michaud M, Poirier-Colame V, Andreiuolo F, Galluzzi L, White E, et al. Immunohistochemical detection of cytoplasmic LC3 puncta in human cancer specimens. Autophagy 2012; 8:1175 - 84; http://dx.doi.org/10.4161/auto.20353; PMID: 22647537
- Smith JJ, Deane NG, Wu F, Merchant NB, Zhang B, Jiang A, Lu P, Johnson JC, Schmidt C, Bailey CE, et al. Experimentally derived metastasis gene expression profile predicts recurrence and death in patients with colon cancer. Gastroenterology 2010; 138:958 - 68; http://dx.doi.org/10.1053/j.gastro.2009.11.005; PMID: 19914252
- Freeman TJ, Smith JJ, Chen X, Washington MK, Roland JT, Means AL, Eschrich SA, Yeatman TJ, Deane NG, Beauchamp RD. Smad4-mediated signaling inhibits intestinal neoplasia by inhibiting expression of β-catenin. Gastroenterology 2012; 142:562 - , e2; http://dx.doi.org/10.1053/j.gastro.2011.11.026; PMID: 22115830
- Gröne J, Lenze D, Jurinovic V, Hummel M, Seidel H, Leder G, Beckmann G, Sommer A, Grützmann R, Pilarsky C, et al. Molecular profiles and clinical outcome of stage UICC II colon cancer patients. Int J Colorectal Dis 2011; 26:847 - 58; http://dx.doi.org/10.1007/s00384-011-1176-x; PMID: 21465190
- Rickman DS, Millon R, De Reynies A, Thomas E, Wasylyk C, Muller D, Abecassis J, Wasylyk B. Prediction of future metastasis and molecular characterization of head and neck squamous-cell carcinoma based on transcriptome and genome analysis by microarrays. Oncogene 2008; 27:6607 - 22; http://dx.doi.org/10.1038/onc.2008.251; PMID: 18679425
- Li Q, Birkbak NJ, Gyorffy B, Szallasi Z, Eklund AC. Jetset: selecting the optimal microarray probe set to represent a gene. BMC Bioinformatics 2011; 12:474; http://dx.doi.org/10.1186/1471-2105-12-474; PMID: 22172014
- R Core Team. R: A language and environment for statistical computing. (2013). at <http://www.R-project.org/>
- Dombroski BA, Nayak RR, Ewens KG, Ankener W, Cheung VG, Spielman RS. Gene expression and genetic variation in response to endoplasmic reticulum stress in human cells. Am J Hum Genet 2010; 86:719 - 29; http://dx.doi.org/10.1016/j.ajhg.2010.03.017; PMID: 20398888
- Pietrocola F, Izzo V, Niso-Santano M, Vacchelli E, Galluzzi L, Maiuri MC, Kroemer G. Regulation of autophagy by stress-responsive transcription factors. Semin Cancer Biol 2013; 23:310 - 22; http://dx.doi.org/10.1016/j.semcancer.2013.05.008; PMID: 23726895
- Palmieri M, Impey S, Kang H, di Ronza A, Pelz C, Sardiello M, Ballabio A. Characterization of the CLEAR network reveals an integrated control of cellular clearance pathways. Hum Mol Genet 2011; 20:3852 - 66; http://dx.doi.org/10.1093/hmg/ddr306; PMID: 21752829
- Huang E, Cheng SH, Dressman H, Pittman J, Tsou MH, Horng CF, Bild A, Iversen ES, Liao M, Chen CM, et al. Gene expression predictors of breast cancer outcomes. Lancet 2003; 361:1590 - 6; http://dx.doi.org/10.1016/S0140-6736(03)13308-9; PMID: 12747878