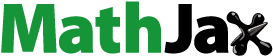
Abstract
Since experiments involving animal models are labor and time intensive, there is an attempt to replace these measurements on animal models with in vitro assays which has higher acceptance in the population concerning ethical issues. In this work, we explore to what extend animal models can be replaced by in vitro assays in the context of a toxicogenomics study. The data from the Japanese Toxicogenomics Project are gene expression profiles measured by microarrays from both in vitro and animal samples. We apply a comprehensive genomic association network analysis in order to study the comparative behavior of the genomic networks for the in vivo vs. in vitro data. The genomic networks are computed based on association scores of gene-gene pairs using a partial least squares modeling of gene expression values adjusted for sacrifice time and dosage. We apply permutation based statistical tests to compare the connectivity of a given gene, as well as a class of genes in the two networks which may be affected by a given drug. The goal is to identify parts of these networks including key genes that are not significantly altered for in vivo vs. in vitro samples for the majority of the drugs.
Introduction
In the last century many drug development projects were based on screening the effects of chemicals in animal models. However, concerns such as animal rights, prohibitive costs and time to perform such experiments have prompted the scientific community to explore the possibility of using in vitro studies instead of in vivo animal models. Undetected toxicity is the primary reason for drug failure. The response of an organism to drug toxicity in some particular organ, especially the liver, can be detected by the changes in gene expression levels. In particular, changes in the expression of some genes can serve as biomarkers for the pre-clinical stages of drug development. Identifying these genes using in vitro gene expression data (for animals) may potentially eliminate the need for using real animals. Hence there is a great interest to replace an animal model with an in vitro study in the field of toxicogenomics.
The biomarker study involving liver toxicity is a unique approach to investigate the drug related liver injury. The Japanese Toxicogenomics Project is one of the largest toxicogenomics studies known as TGGATEs (Genomics-Assisted Toxicity Evaluation System developed by the TGP in Japan). This gene expressions study comprises 150 medical drugs with the organ at which toxicity is measured being the liver. Moreover this gene expression study supplied human and rat in vivo and in vitro studies. We use gene expression data to provide a partial answer to the important question in toxicogenomics whether in vivo microarray expression data based on animal studies can be replaced by in vitro data. The TGP data set contains over 31,000 arrays that measure rat gene expression profiles treated with drugs and profiled by Affymetrix RAE230_2.0 GeneChip®. In a previous analysis of this data, Uehara et al.Citation1 identified the genes commonly upregulated both in vivo and in vitro after treatment with three different drugs: clofibrate, WY-14643 and gemfibrozil. This study was one of the first to create an in vivo–in vitro bridge for the validation of genomic biomarkers with those three compounds. These genomic biomarkers are important factors in predicting the pre-clinical drug toxicity.
In this analysis, we attempt to provide a comprehensive view of the in vivo–in vitro bridging across all the genes (probe sets) for all 131 drugs provided in the CAMDA 2013 challenge data. The in vivo–in vitro bridging genes may help us assess the hepatotoxicity of the drugs using in vitro data. Moreover, our approach is not only to observe the similarities in gene expressions of individual genes marginally but to identify the similarities of the network connectivity of all the similar genes across all the chemicals. As the genes do not act alone and their expressions are not independent of one another, we think that a holistic approach of observing the co-expressions of all the genes should provide better information and potentially identify a set of reliable biomarkers of a toxicity study.
Methodologically, we consider this question from a statistical perspective and apply a significance test to examine if there is a difference between the genomic networks for the two different types (in vivo, in vitro) after accounting for different dosages of a given drug, and sacrifice times of the rats. We conduct this type of analysis for each of the drugs provided in the data set and compare the results. In order to construct the genomic networks followed by identifications of differences and similarities of the networks for the two assay types we use an extension of the framework of a differential network analysis (DNA) described in our earlier work in Gill et al.Citation2 This extension was necessary to incorporate additional sources of variability present in the current data.
Construction of the networks for each data type (in vivo, in vitro) is based on a connectivity score measuring the association between each pair of genes. In order to study the expression pattern and the network structures, appropriate data preprocessing is required to account for type, dose, and sacrifice time effects. There are substantial differences between the expression values of the MAS5 preprocessed data from the in vivo and in vitro samples. Any naive attempt (such as a gene by gene t test) might find that all genes are significantly differentially expressed in the two types. So we incorporate an additional preprocessing step into our linear model (ANOVA) for log-gene expressions itself. Similarly, these effects are included in our model for the computation of the partial least squares (PLS) scores for the network analysis. More precisely, we apply a connectivity score constructed using a PLS regression method that captures the predictability of each gene's expression from a pairing gene after adjusting for other genes and additional covariates (such as dosage and sacrifice time) and thus extending our earlier approach to network constructions and differential network analyses.Citation2-4 We only consider this extension for PLS scores since these scores have been effective in the previous studies, but in principal, scores can be computed based on many other methods such as correlation, principal components regression, ridge regression, the LASSO, etc. However, additional work would be required to adapt these other methods to allow for additional covariates as we do for PLS herein.
The rest of the manuscript is organized as follows. In the Materials and Methods we describe the methodology to construct the network following the PLS regression based association scores. In the Results section we report the results and we conclude with a Conclusion section.
Data
We analyze part of the challenge data set from the Japanese Toxicogenomics Project and compare the MAS5 preprocessed data (downloaded from the CAMDA 2013 website) from the “single dose study in vivo experiment using Sparague-Dawley rats” with the “in vitro study using hepatocytes from Sparague-Dawley rats” for each of 131 drugs. The in vivo data set for each drug has microarray expression values of 31,099 genes for 48 rats at four different dose concentrations (control, low, middle, and high) and four different sampling times (3, 6, 9, and 24 h) with three observations at each combination of the levels for these factors. Also, for the in vitro study, microarray gene expression analysis was performed on the primary cultured cells at multiple time points and different drugs with different dose levels. Consequently the in vitro data set for each drug has microarray expression values of the same genes for 24 rat liver cells at four dose concentrations with the same labels and three different sampling times (2, 8, and 24 h) with two observations at each combination of the levels.
Results
For each of the 131 drugs, tests for differential connectivity of the networks on the set of all 473 bridging genes were performed based on (1) using 1000 permutations based on the L1-distance function and the PLS connectivity scores. No significant differences in the overall connectivity scores of the networks of this set of 473 genes were found for 77 of the 131 drugs at a 5% significance level. These drugs are listed in .
Table 1. Drugs with similar connectivity scores in the two networks are provided
Some of the drugs in this list of 77 drugs are established as causing drug-induced liver injury (DILI) and 41 of these drugs are listed in bold in . Among the drugs labeled as high DILI concern, no significant differences were found in the overall connectivity scores of the two networks for 23 out of these high DILI 41 drugs.
Even among the 18 high DILI drugs for which the set of all genes are significantly different in terms of overall network connectivity, there are many genes that are not significantly different in terms of individual connectivity scores in the two networks at a 5% level. Tests for the significance difference of the connectivity score of each individual gene within the network were performed based on (2) for these 18 drugs, and there were 17 genes that were not differentially connected for at least 80% of the drugs between the two types in vitro vs. in vivo. These genes are shown in .
Table 2. Genes which are not differentially expressed between the networks for at least 80% of the 18 high DILI drugs for which there is a statistically significant difference between the overall connectivity score for in vivo vs. in vitro networks based on the set of all genes
In order to characterize the 473 genes which have shown no significant difference between the in vivo and in vitro types (when analyzed one-by-one marginally) for more than 80% of the drugs we used the functional annotation tool DAVID.Citation5,6 Results of that analysis for the top five functional clusters out of the 473 genes are given in . Most of the genes in the first functional cluster are involved in neuron development, neuron differentiation, neuron projection morphogenesis and cell morphogenesis activities. The genes in the second most important cluster are involved with proteins in cell–cell junctions of multi-cellular species and also most of them are associated with some synaptic activities. The third most important functional cluster of the genes is associated with epidermal growth factor (EGF) proteins. We then form sub-networks for the genes in each functional cluster and then perform the differential network test for the overall connectivity of those genes in the in vivo and in vitro groups for each drug. We report the percentage of drugs for which the difference in the overall connectivity were insignificant (last column of ). These sub-networks had fewer significant differences between the in vivo and in vitro types than the overall networks. As seen in the , the difference between the in vitro and in vivo networks are not statistical significant for at least 87% of the drugs among these top five clusters. On the other hand when we tested the differential connectivity considering all the 473 non-significant genes then we found that the networks were not significant for about 59% (77 out of 131) drugs. So it can be concluded that the expressions of these genes (whether in vivo or in vitro analysis) in these functional clusters will not change significantly even with the treatment of drugs with high DILI potential.
Table 3. The top 5 clusters obtained from the DAVID Functional Annotation Tool
Next we wanted to further identify the genes which were studied by other researchers in liver toxicity studies. We consider a list of 82 genes identified by a previous in vivo rat liver study by Guzelian et al.Citation7 which were found to be significantly downregulated by a steroid (pregnenolone 16α-carboitrile) treatment known to help protect the liver. Of these 82 genes, only 7 genes (rnd3, KIFC2, LRP5, MATN1, CLDN1, DAP1, Lrp11) were common to the TGP data set. For each of the high DILI drugs, a test for differential connectivity of this class of 7 genes was performed, and the difference between the two networks was not statistically significant for 23 out of the 41 high DILI drugs. It shows that these six genes collectively remain unaffected in the presence of 56% of highly toxic drugs with DILI potential. We also report the results of the difference in connectivities of all these genes individually in the presence of other genes in the networks. Tests for differential connectivity of the individual genes were also performed for each of the 41 high DILI drugs. The proportions of drugs for which each gene were not found to be significantly different are reported in .
Table 4A. Proportions of high DILI drugs for which the downregulated genes from Guzelian et al. common to the TGP data set are not differentially expressed
Two of the genes are functionally interesting in the context of the present study. One is LPR5. This can enhance the expression of a critical component of the Wnt signaling pathway which is known to impact osteogenesis in response to mechanical stimulation. Another one is DAP which is known to be involved in mediating interferon-gamma-induced cell death.
In another study, Tugues et al.Citation8 examined expression values for liver endothelial cells in cirrhotic and control rats and identified a set of differentially expressed genes for these two groups. Of these genes, only 9 genes (Mmp23, CAMK2G, GATA3, ankh, CDH1, GPR182, Ptger4, ANXA1, GGT5) were common to the TGP data set. For each of the high DILI drugs, a test for differential connectivity using (1) of this class of 9 genes together was performed, and the difference between the two networks was not statistically significant for 34 out of the 41 high DILI drugs. We also investigated the differential connectivity of each of these genes individually in the networks for 41 high DILI drugs. The proportions of drugs for which each gene were not found to be significantly different using (2) are reported in .
Table 4B. Proportions of high DILI drugs for which the differentially expressed genes (between cirrhotic and control groups) from Tugues et al. common to the TGP data set are not differentially expressed
It shows that these 9 genes that were established to be differentially expressed in cirrhotic and control rats while analyzed marginally showed no significant difference between the in vivo and in vitro networks while treated with about 83% of drugs with high DILI potential.
Lastly, we wanted to illustrate how these sub-networks behave for a given drug. illustrates the constructed in vitro and in vivo networks for the genes in cluster 4 for phenylbutazone, a non-steroidal anti-inflammatory drug (NSAID). The genes are SLIT3 (1391375_at), Odz2 (1375331_at), Nrxn3 (1375653_at), Adam4 (1385244_at), Epha5 (1388265_a_at), SLC22A23 (1371069_at), VCAN (1371007_at), and RELN (1396334_at). For these networks, the test for differential connectivity is not significant (P value is 0.42). All edges in the in vivo network also appear in the in vitro network, and only 4 edges in the in vitro network do not appear in the in vivo network.
Materials and Methods
As stated in the Introduction, we needed to build a preprocessing step for the gene expression data to account for the other factors such as drug dosages and sacrifice times. Without these corrections, the gene expression levels in the two groups may appear to be different due to non-biological causes. illustrates this issue for one of the genes in , whose behavior is not significantly different in the two networks. However, the Box plots of the raw gene expression values appear to be very different (shown for 8 high DILI drugs).
In order to achieve this, we used a nested ANOVA model to assess the effects of TYPE (in vivo, in vitro), drug dose (DOSE), and sacrifice time (SAC) on the expression levels of 31,099 genes for each drug. Specifically, for each drug the mean expression value for the ith observation for the gth gene is modeled as
The nested structure is necessary since the categorical values of DOSE and SAC are only meaningful conditional on the value of TYPE. Some of the possible values of SAC only correspond to one value of TYPE, and even when the values or labels are the same, the effects corresponding to this value should be estimated separately for each TYPE.
Before fitting the ANOVA model we take the logarithm of the centered expression levels; the logarithms of the expression values are centered with respect to all genes of the given type. For each drug, the P values for TYPE effect coefficients are computed for each gene under the assumption that the log-expression values follow a normal distribution with homogeneous error variance. We use these preliminary ANOVA analyses to determine the genes for which the expressions are not significantly different for two different types (in vivo vs. in vitro) at a pairwise type 1 error rate of 0.05. Summarizing the results for all the drugs we find there are 473 genes for which the TYPE effect is not significant for at least 80% of the drugs. In other words, the expression profiles of this common set of genes appear to be similar for many of the drugs. Thus, these 473 genes can be taken as common bridging genes between in vivo and in vitro studies across a great majority of the drugs. However, as the genes do not work independently we want to construct the network of these genes and check their differential behaviors across two types (in vivo, in vitro).
The tests described in this section are based on connectivity score sik which measures the association between the ith and kth genes in a network. Our earlier methodsCitation2 for differential network connectivity are modified to allow for additional covariates such as drug dosages and sacrifice times. We estimate the coefficients for these additional covariates at the same time that the coefficients used to compute the connectivity scores are obtained. Let xi be the centered and scaled expression vector for the ith gene. Gill et al.,Citation2 Pihur et al.,Citation3 and Datta,Citation9 demonstrate the effectiveness of PLS regression and performance in comparison with some other methods. The method of computing the PLS scores that is described in Pihur et al.Citation3 uses separate PLS models
for each gene i. However, in the present context, adjustments for additional effects such as the dose levels and sacrifice times are needed; thus we create additional covariate vectors z1,…,zm based on the levels of the nested covariates and fit a set of linear models of the form
Specifically, zk's indicator variables are constructed such that every possible combination of dose and sacrifice time is included in the model for each network. For the in vivo model, 16 indicators are needed to represent all possible combinations of the 4 doses and 4 sacrifice times; for the in vitro model, 12 indicators are needed for all possible combinations of 4 doses and 3 sacrifice times.
PLS regression is used to estimate the coefficients ai1,…aim,bi1,…bi,i−1,bi,i+1,…bip based on the design matrix formed by the covariates in the PLS model. Here, the method for computing the PLS regression estimates of the regression coefficients and the conversion to PLS scores are described; this is a modified version of the method described in Pihur et al.Citation3 Starting with ℓ = 1,
for fixed PLS latent factors
the method of least squares is applied to the model
to obtain coefficient estimates
for ℓ= 1,2,…,v.
For ℓ = 1,
the matrix X(1) is defined to be the deflated design matrix with columns z1,…,zm,x1,…,xi−1,xi+1,…,xp where p is the number of bridging genes. This matrix is updated using the formula
for ℓ ≥ 2.
The PLS latent factors are linear combinations of the covariates z1,…,zm,x1,…,xi−1,xi+1,…,xp constructed by
where
are the columns of the matrix
and
are the components of the vector
Then the estimates of bi1,…,bi,i−1,…,bi,i+1,…bip are obtained from
for k = 1,…,i−1,i+1,…,p. A symmetrized estimate of regression coefficient bik is taken as the PLS association score
Once the connectivity scores are computed for each network, a permutation test is performed to test for differential connectivity of the class of all the bridging genes together or the test for a single gene in the presence of other genes. Let sik(1) and sik(2) denote the connectivity scores between genes i and k for networks 1 and 2, respectively. The test statistic for the class of all genes F with cardinality f is(1)
(1)
the test statistic for a single gene g is(2)
(2) where D computes the distance between the connectivity scores. We have worked with the L1-distance D(s(1),s(2)) = | s(1) − s(2)| rather than the more commonly used L2-distance leading to a more robust analysis since this measure is less affected by extreme scores. The permutation test is performed by randomly assigning the labels to each observation in the data set formed by combining the observations from both networks. For networks with moderate sample sizes, it is not feasible to consider all possible permutations, so a fixed number of random permutations are selected. There is a natural tradeoff when selecting the number of permutations. A larger number of permutations require more computation time, but it provides a more accurate estimate of the P value.
Conclusion
In this paper we present a comprehensive view of the in vivo-in vitro bridge of the genes using the rat microarray TGP study using all drugs measured in this study. We not only provide the similarity of individual gene expression patterns while analyzing the data marginally for the two groups but also that of the gene-gene association networks under in vivo and in vitro experiments. The systems are scrutinized in terms of overall network connectivity and also in terms of individual gene connectivity in a network (which is entirely different from the marginal analysis of the gene expression individually). We found that the conclusions can be different when analyzing the whole network opposed to a marginal analysis. As the genes do not act alone we think that considering the interplay of genes in terms of a network is more appropriate.
We have used PLS based association scores adjusted for sacrifice time and dosage followed by a permutation based statistical test. Since we are trying to identify genes that are not differentially expressed between in vivo and in vitro studies, a conservative approach in this context will be not to apply a multiple testing P value correction unlike typical gene expression studies. In such studies the goal is to identify genes that are differentially expressed and/or connected under two biological conditions. Interestingly but similar to Uehara et al.1 who studied three of the drugs, none of the bridging genes that we found are involved with cell proliferation and apoptosis.
A potential limitation of our approach is that our findings are based on a specific type of statistical model (e.g., PLS with ANOVA). In the future, we may undertake additional investigations where networks are constructed by fitting other types of predictive models such as LASSOCitation10 or elastic netCitation11 and compare the results.
The findings described here must be considered with care. First of all, we have highlighted the genes (and the portion of the networks) which were not significantly different in the two data types. However it does not imply that in vivo and in vitro studies are completely interchangeable since there are genes that show differential expression and network profiles in the two networks. Furthermore, lack of statistical significance does not necessarily imply that the objects under comparison are indeed equal. Further experimental investigations are needed for a definitive validation of our results in order to justify the use of these genomic biomarkers for pre-clinical drug safety assessment. However, this study provides a rough guideline to find bridging genes for which an in vitro study may indicate the nature of changes of gene expressions in vivo. Our findings hint at the need for additional network level studies replacing differential gene expression studies in the context of other toxicological experiments.
Disclosure of Potential Conflicts of Interest
No potential conflicts of interest were disclosed.
Funding
This research work was partially supported by NIH grant CA 170091-01A1 (Su. Datta).
References
- Uehara T, Ono A, Maruyama T, Kato I, Yamada H, Ohno Y, Urushidani T. The Japanese toxicogenomics project: application of toxicogenomics. Mol Nutr Food Res 2010; 54:218-27; PMID:20041446; http://dx.doi.org/10.1002/mnfr.200900169
- Gill R, Datta S, Datta S. A statistical framework for differential network analysis from microarray data. BMC Bioinformatics 2010; 11:95; PMID:20170493; http://dx.doi.org/10.1186/1471-2105-11-95
- Pihur V, Datta S, Datta S. Reconstruction of genetic association networks from microarray data: a partial least squares approach. Bioinformatics 2008; 24:561-8; PMID:18204062; http://dx.doi.org/10.1093/bioinfor-matics/btm640
- Gill R, Datta S, Datta S. DNA: An R package for differential network analysis. Bioinformation; 10:233-4. PMCID: PMC4070055
- Huang W, Sherman BT, Lempicki RA. Systematic and integrative analysis of large gene lists using DAVID bioinformatics resources. Nat Protoc 2009a; 4:44-57; PMID:19131956; http://dx.doi.org/10.1038/nprot.2008.211
- Huang W, Sherman BT, Lempicki RA. Bioinformatics enrichment tools: paths toward the comprehensive functional analysis of large gene lists. Nucleic Acids Res 2009b; 37:1-13; PMID:19033363; http://dx.doi.org/10.1093/nar/gkn923
- Guzelian J, Barwick JL, Hunter L, Phang TL, Quattrochi LC, Guzelian PS. Identification of genes controlled by the pregnane X receptor by microarray analysis of mRNAs from pregnenolone 16α-carbonitrile-treated rats. Toxicol Sci 2006; 94:379-87; PMID:16997903; http://dx.doi.org/10.1093/toxsci/kfl116
- Tugues S, Morales-Ruiz M, Fernandez-Varo G, Ros J, Arteta D, Muñoz-Luque J, Arroyo V, Rodés J, Jiménez W. Microarray analysis of endothelial differentially expressed genes in liver of cirrhotic rats. Gastroenterology 2005; 129:1686-95; PMID:16285966; http://dx.doi.org/10.1053/j.gastro.2005.09.006
- Datta S. Exploring relationships in gene expressions: a partial least squares approach. Gene Expr 2001; 9:249-55; PMID:11763996
- Tibshirani R. Regression shrinkage and selection via the lasso. J R Stat Soc, B 1996; 58:267-88.
- Zou H, Hastie T. Regularization and variable selection via the elastic net. J R Stat Soc, B 2005; 67:301-20; http://dx.doi.org/10.1111/j.1467-9868.2005.00503.x