Abstract
Post-transcriptional regulation (PTR) of gene expression is now recognized as a major determinant of cell phenotypes. The recent availability of methods to map protein-RNA interactions in entire transcriptomes such as RIP, CLIP and their variants, together with global polysomal and ribosome profiling techniques, are driving the exponential accumulation of vast amounts of data on mRNA contacts in cells, and of corresponding predictions of PTR events. However, this exceptional quantity of information cannot be exploited at its best to reconstruct potential PTR networks, as it still lies scattered throughout several databases and in isolated reports of single interactions. To address this issue, we developed the second and vastly enhanced version of the Atlas of UTR Regulatory Activity (AURA 2), a meta-database centered on mapping interaction of trans-factors with human and mouse UTRs. AURA 2 includes experimentally demonstrated binding sites for RBPs, ncRNAs, thousands of cis-elements, variations, RNA epigenetics data and more. Its user-friendly interface offers various data-mining features including co-regulation search, network generation and regulatory enrichment testing. Gene expression profiles for many tissues and cell lines can be also combined with these analyses to display only the interactions possible in the system under study. AURA 2 aims at becoming a valuable toolbox for PTR studies and at tracing the road for how PTR network-building tools should be designed. AURA 2 is available at http://aura.science.unitn.it.
Introduction
Post-transcriptional regulation of gene expression (PTR) has been object of a rising interest in the latest years, gaining wide recognition as the most important determinant of protein levels and cell phenotypes.Citation1-Citation3 In consequence to this interest, huge amounts of data have been and are being collected every day primarily thanks to the advent of the new high-throughput techniques such as polysomal profiling,Citation3,Citation4 ribosome footprinting,Citation5 and protein-RNA interaction mapping methods such as RIP,Citation6 CLIP and its several variants.Citation7-Citation9This massive quantity of information cannot be exploited at its best, due to the fact that most of it lies isolated and scattered throughout several databases,Citation10 or in published papers reporting single PTR interactions. The lack of a comparative platform hampers the possibility to obtain a comprehensive view of the multiple factors binding to UTRs, which could both enlighten about the biological meaning of their combination and allow us to trace regulatory networks. Integrating complementary data types could therefore generate a relevant potential for the discovery of PTR circuits, significantly contributing to the advancement of the field. The solution we envisaged was to build a unified warehouse for PTR data, augmented with clever data mining tools. We thus originally developed AURA, the Atlas of UTR Regulatory Activity, which we now present in a widely extended and improved second version, AURA 2. AURA 2 is a meta-database of post-transcriptional regulatory interactions, centered on untranslated regions (UTRs) of mRNAs and relying on experimental data as its sole source of information. We decided to exclude predictions (except for two kinds of them, see below) so to give strength to insights and to sustain clues derived from the data integration tools. AURA 2 includes, to mention a few, data such as RBPs and ncRNA binding sites on UTRs, cis-elements like AU-Rich Elements,Citation11 RNA epigenetics data such as m5C and m6A profiles,Citation12,Citation13 alternative polyadenylation profilesCitation14 and variation profiles such as SNPs and somatic mutations in cancer. The result is an environment in which widely different aspects of the same process, PTR, can be observed side by side and employed to derive new functional insights on the regulation of a single gene or a collection of functionally related genes. This wealth of data is complemented by a set of data-mining tools providing novel views on PTR of either individual or groups of genes. Particular attention was given to the interface, which is designed to be easy to use but flexible enough to accommodate advanced usage and complex queries. AURA 2 is intended to be a one-stop broad resource for PTR, targeted at both command-line averse biologists and bioinformaticians.
Results
The strength of a resource such as AURA 2 lies in its ability to be constantly updated and include, in a timely manner, as many as possible data types and records concerning its area of focus. On the other hand, clever exploitation of such a data collection must be empowered by various adequate retrieval and mining features, eventually allowing users to effectively reach the data they need. We will now describe what can be found in AURA 2, both in terms of data types and quantities therein contained and in terms of website features such as search modes, analytical tools and data download options. A graphical summary of AURA 2 capabilities is displayed by .
Figure 1. AURA 2 features. The figure illustrates the main types of information contained in the database (Data) and the most prominent investigation tools available, either for exploring PTR events for a single UTR (UTR Browser, trans-factor search and UTR secondary structure analysis) or for a gene set (PTR network generation, regulatory enrichment computation and co-regulation search). The available data download options are also illustrated (Data download).
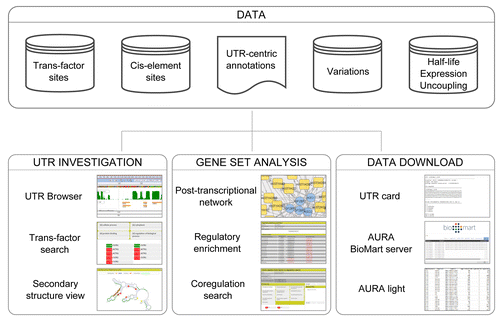
Data types and figures
AURA 2 currently includes data about two model species, namely Homo sapiens and Mus musculus. However, its structure allows for any number of model species to be inserted and other, such as Danio rerio, Caenorhabditis elegans and Saccharomyces cerevisiae, will follow later on. Both included species share a layer of basic annotation that goes from a gene, transcript and UTR model obtained from the UCSC Genome annotationCitation15 to phylogenetic conservation, secondary structure folding and Gene Ontology term associations. Human data also includes two different data sets of transcript half-life measurements, obtained by microarrayCitation16 and high-throughput sequencingCitation17 respectively. Finally, uncoupling between translatome and transcriptome gene expression variations, suggesting the occurrence of post-transcriptional regulation, is quantified and displayed for both human and mouse genes based on a meta-analysis of data sets containing such profiles.Citation3 On top of these annotations, a number of different PTR databases and data sets of various kinds were collected and integrated. We decided to consider only experimentally derived data, thus excluding predictions. This was done with the notable exception of AU-Rich Elements, obtained from AREsite,Citation18 and of the secondary structure of mRNAs, because these predictions are pervasive in mRNAs and their UTRs and can help in formulating interesting hypotheses as complement to the interaction data. While on one side the exclusion of predictions leads to limit the amount of exploitable information, on the other side we believe this choice to give strength and reliability to the PTR networks which can be inferred by means of AURA 2 and the biological hypotheses which can be formulated thereafter. Concerning RBPs and their binding sites on UTRs, AURA 2 contains both data obtained by single mRNA experiments such as luciferase assays (obtained by screening the literature produced for each RBP and extracting regulatory events and binding sites from the related papers), and by means of high-throughput techniques such as the CLIP family of methodsCitation7-Citation9; RBP functional descriptionsCitation19 and binding motif logos from RBPdbCitation20 and CISBP-RNACitation21 are also included when available. Currently, data are available for 158 RBPs, 32 of which in mouse. MicroRNA-mRNA interactions are represented through the inclusion of several data sets aggregating results from low-throughput techniques (mostly obtained through existing databases integration, see Supplemental Table 2), covering 312 microRNAs; high-throughput data sets profiling Argonaute binding sites (see Additional File 1) and microRNA-mRNA interactions derived by CLASHCitation22 are also included. Currently, a total of 985 mapped microRNAs are present in AURA 2. Data for a wide range of cis-element categories were looked for and collected. We included AU-Rich Elements obtained from AREsite,Citation18 ALU repeats,Citation15 alternative polyadenylation events,Citation14 alternative translation initiation sitesCitation23,Citation24 from genome-wide assays, TOP genes annotationCitation25 and a map of hyper-conserved elements in UTRs, that we previously described inCitation26. We also included RNA post-transcriptional modification maps: in particular, A to I RNA editing (from DARNEDCitation27), m5C and m6A methylation were considered in this version in the light of the growing importance attributed to these phenomena.Citation12,Citation13 All these data currently amount to more than 50 thousand regulatory sites. Eventually, in order to allow the user to estimate the potential effects of sequence variation on UTRs and their regulation, SNPs from dbSNPCitation28 and somatic mutations in cancer from COSMICCitation29 were also included in AURA 2 (totaling more than one million variations) and are displayed in independent tracks along with trans-factors and cis-element sites.
Considering trans-factors, cis-elements and variations together, AURA 2 currently contains more than 2.5 million regulatory sites. A complete list of integrated databases can be found in Supplementary Table 1, statistics about AURA 2 are summarized in Supplementary Table 2 and the integrated high-throughput data sets are eventually listed in Supplementary Table 3.
Combined with the wealth of data we just described, AURA 2 offers various search and analytical tools customized for PTR as powerful ways to take advantage of this data warehouse. On one side, its user interface is tuned to deliver an intuitive and seamless search experience to command-line averse biologists; on the other hand, data mining-oriented tools will allow bioinformaticians to query and retrieve large amounts of data quickly and in automated ways.
Search modes and analytical tools
In particular, five search modes are available, enriched by various filters and parameters: along with the classic gene search, providing an overview of all PTR events mediated by the UTRs of a single gene whose name corresponds to the query term, the user can also search for a trans-factor (e.g., an RBP or a ncRNA) in order to obtain all the UTRs which share a regulatory event by this factor; these UTRs can be grouped by Gene Ontology terms in order to provide an immediate clue to functions and processes controlled by this trans-factor through its UTR targets. The other three search methods are named co-regulation, sequence and batch searches. Co-regulation search allows users to retrieve all UTRs controlled at the PTR level by two or more trans-factors: this feature can be extremely useful, for instance, to identify different factors controlling a common process or to detect competing regulation phenomena. Sequence search performs a BLASTCitation30 query against 3′ and 5′ UTRs of the selected organism, permitting to display and browse all matching UTRs and to download results. The last search mode is the batch search, which includes two analytical tools and is offered in three flavors. Users can input a list of genes in which they are interested: the first option consists in selecting and browsing all UTRs of these genes together; secondarily they can build a PTR network in which edges are regulatory relationships (factor F is controlling an mRNA M and so on) and which outlines all factors regulating and possibly shared by the genes in the input list. The last option consists of performing a Fisher’s exact test in order to understand which trans-factors or cis-element categories are significantly enriched in the provided gene set. When combined, these options will provide both a graphical and intuitive way of producing hypotheses on the regulation of thousands of human and mouse mRNAs and a sound statistics indicating which factors may be interesting to pursue for subsequent investigations.
Eventually, interactions displayed by the trans-factor, co-regulation, network generation and regulatory enrichment modes can be filtered for the expression of involved genes in a set of 45 different tissues and 3 cell lines (see Materials and Methods). This powerful feature allows the user to investigate only the potential interactions which are relevant to the system under study.
The UTR Browser
UTRs selected for detailed visualization by any of the search modes described above are then displayed in individual page segments (UTR Browser) including a basic annotation section (showing, for instance, genomic coordinates, length and overall conservation) and a dedicated genome browser. This component, based on JBrowseCitation31, allows the user to zoom and move throughout the UTR sequence, add or remove tracks and rearrange their order to best suit the user’s focus. Along with sequence and conservation tracks, regulatory sites of RBPs, microRNAs, variations and each category of cis-elements are each laid out on independent tracks, for the maximum flexibility in choosing which data to visualize and with which arrangement. Further features accessible through the UTR Browser are gene expression measurements for the gene and the trans-factor at hand, and annotated UTR secondary structure visualization. The former feature takes advantage of several hundred gene expression profiles performed on more than 20 different tissues and stored at the Genotype-Tissue Expression project (GTEx)Citation32: this database is queried on-the-fly for the displayed gene and for the related trans-factor (only when the trans-factor search is used); relevant gene expression measurements are displayed in a pop-up window. The latter feature provides the UTR secondary structure folding (drawn by the VARNA pluginCitation33), annotated with a green gradient for phylogenetic conservation and three different colors for SNPs, RBPs and ncRNA binding sites: users can thus readily understand whether a regulatory event relies on or can be influenced by a particular predicted secondary structure element.
Data download
All of the information displayed in the various UTR Browser parts is also downloadable through the UTR card feature, which consists of a textual, human-readable (but also machine-parseable thanks to its structured layout) collection of all the data regarding the investigated UTR. The card feature is also available for trans-factors such as RBPs and microRNAs and includes, along with basic annotation and descriptions, all the binding sites for the factor and related target UTR data. The complete AURA 2 data set can also be retrieved by downloading the database dump (to replicate the complete database on a local machine), by downloading a reduced version of the database, called AURAlight, which includes only data concerning cis-elements and trans-factors interactions with UTRs in tab-delimited format, or by accessing the AURA 2 Mart. This latter feature is an instance of the federated database system BioMartCitation34 containing all of AURA 2 data. BioMart provides an unique query interface shared by all its instances (called Marts, of which the most famous is probably the Ensembl BioMart): an user which had been already exposed to a Mart will therefore be able to query and retrieve data from the Mart offered by AURA 2 without additional learning efforts. A comparison of AURA 2 features, data content and mining tools with respect to its previous version and other available resources is in Supplementary Materials (Supplementary Text, Supplementary Table 1–2). Furthermore, a user guide to AURA 2 features is also available in Supplementary Materials (Supplementary Text).
Discussion
We think that AURA 2 is a considerable step forward for PTR data integration and PTR network-building capabilities. Nevertheless, interaction data are still available only for a limited number of RBPs and non-coding RNAs: this aspect represents a limit on the possibility to accurately reconstruct regulatory networks. While this issue will be progressively solved with the predictable increase of available data sets (from CLIP and CLIP variants), it must as of now be taken into account when building such networks. It is to be stressed that all data sets present in AURA 2 were included ‘as-is’, without reprocessing data: this choice has been made on the basis of the heterogeneity of the techniques employed to obtain such data. Even if we decided to reprocess all these data sets, we would have needed to employ several analysis protocols, with their inherent biases and limitations. Furthermore, we decided not to exclude any data set on the basis of quality judgments: references to the original publication are available for every and each binding site throughout the database, allowing users to further investigate and eventually decide on the value of the data they wish to exploit. A quality measure for the high-throughput-derived data sets (RIP, CLIPs, etc.) considering properties such as presence of replicates and filtering stringency will likely be subject of future developments in AURA 2. Evidence has been accumulating in the last years to suggest that interactions, both in terms of cooperation and competition, do happen between RBPs and microRNAs.Citation35,Citation36 A small but increasing number of publications are also now indicating that lncRNAs may be involved as well.Citation37 We think that these phenomena will likely gain more importance as they are elucidated and characterized, emphasizing the opportunity of exploiting them to discover biological implications and to promote better network-building approaches in PTR. We plan to integrate this kind of data in AURA 2 and possibly develop some dedicated search and visualization modes to allow the user for seamless and effective study of this interaction processes. Another emerging topic in PTR is RNA epigenetics. As can be seen in AURA 2, genome-wide transcriptome methylation and editing data sets are beginning to appear and the next years will most likely start to reveal the impact of these regulatory processes and the potential interplay with other PTR actors. Integrating from the start these data into the PTR networks we are building will help to better understand the functional effects of RNA epigenetics and improve its characterization. We also aim at expanding AURA 2 by including annotation and data for other model organisms which are relevant in RNA biology, such as C. elegans, S. cerevisiae, D.rerio and D. melanogaster. This will require collaboration with experts of these species to achieve effective and useful data integration. On a broader perspective, being able to involve the PTR research community in maintaining AURA 2 updated and in determining the directions to be taken in its development would be of great value to make it as effective and useful as possible: we indeed are available for establishing new and thriving collaborations toward this aim.
Materials and Methods
Website implementation
The database was designed and implemented on a MySQL Community Server 5.5 (Oracle, Santa Clara, CA, USA). The website is implemented with Python programming languageCitation38 and the Django web framework,Citation39 exploiting JQueryUI graphical components.Citation40 The UTR browser employs JBrowseCitation31 as genome browser to display sequence, and data tracks; UTRs secondary structures are visualized through the VARNA plugin,Citation33 while CytoscapeWebCitation41 is used to display PTR networks. Sequence search is performed by means of a local installation of BLASTCitation30 on a custom database containing 5′ and 3′UTR sequences. The BioMart site was implemented on BioMart 0.8Citation34 by producing a copy of the AURA database containing all the data and employing a database schema adequate for BioMart.
Basic annotation data retrieval
Gene symbols, synonyms and annotations were retrieved from HGNCCitation42 and MGICitation43 for human and mouse respectively. Transcripts and UTRs models, UTR secondary structure folding and phylogenetic conservation data were retrieved from various tracks of the UCSC Genome BrowserCitation15 for both species. Displayed half-life measurements were retrieved from two genome-wide measurements,Citation16,Citation17 obtained through microarray and high throughput sequencing respectively. Uncoupling data was obtained by computing differences in expression variations between translatome and transcriptome profiles in 20 human and mouse data sets, as presented in.3 Links to Human Protein AtlasCitation44 and miRBaseCitation45 for additional trans-factor information, and to PAZARCitation46 for transcriptional regulation data, were composed by plugging-in the trans-factor name in the URL structure of these two websites. Genes associations with Gene Ontology Slim (a reduced version of the complete Gene Ontology tree) terms were retrieved from the Gene Ontology website [4746] for both organisms.
Databases integration
The latest version of integrated databases (listed in Supplementary Table 2) were downloaded in full from their respective websites. When needed, genomic coordinates were converted to the most recent genome assembly version (hg19 for human and mm10 for mouse) and mapped to UTRs. One database, GTExCitation32 was integrated by retrieving expression plot images when requested for a given gene and/or trans-factor.
High-throughput data sets collection
A literature search was performed to retrieve high-throughput data sets for RBPs and ncRNAs, deriving from techniques such as the many variations of RIPCitation6 and CLIP.Citation7-Citation9 Given the wide number of techniques covered by these data sets (thus inherently containing a certain degree of heterogeneity), we decided to insert the data as processed by the authors of each publication: details for each experiment can be accessed through links to the original publications, available on the website. A parallel search focused on identifying studies profiling RNA modifications such as RNA methylations m5C and m6A,Citation12,Citation13 alternative polyadenylationCitation23,Citation24 and translation initiation sitesCitation25 at a genome-wide level. Collected and inserted data sets are listed in Supplementary Table 3. As for the integrated databases, listed in Supplementary Table 1, genomic coordinates were converted to the most recent genome assembly version and mapped to UTRs.
Low-throughput data set building
An extensive, manually curated, RBP-by-RBP literature search was performed in order to collect and extract all RBP-mRNA interactions deriving from mechanistic or single-gene experiments, in papers published from 1994 to 2013. Data obtained from assays producing various degrees of information (protein binding to the mRNA, protein binding to a specific UTR or binding to a defined location in a given UTR) was included; the associated uncertainty of precise binding location was indicated in the UTR browser by graphically coding it with colors and drawing patterns.
Gene expression data set building
HTS-derived gene expression profiles for 45 tissues were retrieved from GTExCitation32, and three further profiles for HeLa, HEK293 and MCF7 cell lines were retrieved from published RNA-seq studies [4847,4948,5049]. RPKMs were computed for these last three data sets by means of CufflinksCitation5150. Expressed genes lists were obtained by filtering these profiles for genes having a RPKM value greater than 0.1.
Abbreviations: | ||
AURA | = | Atlas of UTR Regulatory Activity |
PTR | = | post-transcriptional regulation |
UTR | = | untranslated region of mRNA |
RBP | = | RNA-binding protein |
ncRNA | = | non-coding RNA |
lncRNA | = | long non-coding RNA |
m5C | = | RNA cytosine methylation at C5 position |
m6A | = | RNA adenosine methylation at A6 position |
SNP | = | single nucleotide polymorphism |
CLIP | = | cross-linking and immunoprecipitation |
REST | = | Representational State Transfer |
API | = | Application Programming Interface |
Additional material
Download Zip (281.3 KB)Disclosure of Potential Conflicts of Interest
No potential conflicts of interest were disclosed.
Acknowledgments
We would like to thank Luca Andreetti for reviewing the website graphics and providing helpful advices toward its improvement.
References
- Schwanhäusser B, Busse D, Li N, Dittmar G, Schuchhardt J, Wolf J, Chen W, Selbach M. Global quantification of mammalian gene expression control. Nature 2011; 473:337 - 42; http://dx.doi.org/10.1038/nature10098; PMID: 21593866
- Vogel C, Abreu RdeS, Ko D, Le SY, Shapiro BA, Burns SC, Sandhu D, Boutz DR, Marcotte EM, Penalva LO. Sequence signatures and mRNA concentration can explain two-thirds of protein abundance variation in a human cell line. Mol Syst Biol 2010; 6:400; http://dx.doi.org/10.1038/msb.2010.59; PMID: 20739923
- Tebaldi T, Re A, Viero G, Pegoretti I, Passerini A, Blanzieri E, Quattrone A. Widespread uncoupling between transcriptome and translatome variations after a stimulus in mammalian cells. BMC Genomics 2012; 13:220; http://dx.doi.org/10.1186/1471-2164-13-220; PMID: 22672192
- Melamed D, Eliyahu E, Arava Y. Exploring translation regulation by global analysis of ribosomal association. Methods 2009; 48:301 - 5; http://dx.doi.org/10.1016/j.ymeth.2009.04.020; PMID: 19426805
- Ingolia NT. Genome-wide translational profiling by ribosome footprinting. Methods Enzymol 2010; 470:119 - 42; http://dx.doi.org/10.1016/S0076-6879(10)70006-9; PMID: 20946809
- Keene JD, Komisarow JM, Friedersdorf MB. RIP-Chip: the isolation and identification of mRNAs, microRNAs and protein components of ribonucleoprotein complexes from cell extracts. Nat Protoc 2006; 1:302 - 7; http://dx.doi.org/10.1038/nprot.2006.47; PMID: 17406249
- Ule J, Jensen KB, Ruggiu M, Mele A, Ule A, Darnell RB. CLIP identifies Nova-regulated RNA networks in the brain. Science 2003; 302:1212 - 5; http://dx.doi.org/10.1126/science.1090095; PMID: 14615540
- Hafner M, Landthaler M, Burger L, Khorshid M, Hausser J, Berninger P, Rothballer A, Ascano M Jr., Jungkamp AC, Munschauer M, et al. Transcriptome-wide identification of RNA-binding protein and microRNA target sites by PAR-CLIP. Cell 2010; 141:129 - 41; http://dx.doi.org/10.1016/j.cell.2010.03.009; PMID: 20371350
- König J, Zarnack K, Rot G, Curk T, Kayikci M, Zupan B, Turner DJ, Luscombe NM, Ule J. iCLIP reveals the function of hnRNP particles in splicing at individual nucleotide resolution. Nat Struct Mol Biol 2010; 17:909 - 15; http://dx.doi.org/10.1038/nsmb.1838; PMID: 20601959
- Dassi E, Quattrone A. Tuning the engine: an introduction to resources on post-transcriptional regulation of gene expression. RNA Biol 2012; 9:1224 - 32; http://dx.doi.org/10.4161/rna.22035; PMID: 22995832
- Barreau C, Paillard L, Osborne HB. AU-rich elements and associated factors: are there unifying principles?. Nucleic Acids Res 2005; 33:7138 - 50; http://dx.doi.org/10.1093/nar/gki1012; PMID: 16391004
- Pan T. N6-methyl-adenosine modification in messenger and long non-coding RNA. Trends Biochem Sci 2013; 38:204 - 9; http://dx.doi.org/10.1016/j.tibs.2012.12.006; PMID: 23337769
- Squires JE, Patel HR, Nousch M, Sibbritt T, Humphreys DT, Parker BJ, Suter CM, Preiss T. Widespread occurrence of 5-methylcytosine in human coding and non-coding RNA. Nucleic Acids Res 2012; 40:5023 - 33; http://dx.doi.org/10.1093/nar/gks144; PMID: 22344696
- Derti A, Garrett-Engele P, Macisaac KD, Stevens RC, Sriram S, Chen R, Rohl CA, Johnson JM, Babak T. A quantitative atlas of polyadenylation in five mammals. Genome Res 2012; 22:1173 - 83; http://dx.doi.org/10.1101/gr.132563.111; PMID: 22454233
- Meyer LR, Zweig AS, Hinrichs AS, Karolchik D, Kuhn RM, Wong M, Sloan CA, Rosenbloom KR, Roe G, Rhead B, et al. The UCSC Genome Browser database: extensions and updates 2013. Nucleic Acids Res 2013; 41:D1 D64 - 9; http://dx.doi.org/10.1093/nar/gks1048; PMID: 23155063
- Friedel CC, Dölken L, Ruzsics Z, Koszinowski UH, Zimmer R. Conserved principles of mammalian transcriptional regulation revealed by RNA half-life. Nucleic Acids Res 2009; 37:e115; http://dx.doi.org/10.1093/nar/gkp542; PMID: 19561200
- Tani H, Mizutani R, Salam KA, Tano K, Ijiri K, Wakamatsu A, Isogai T, Suzuki Y, Akimitsu N. Genome-wide determination of RNA stability reveals hundreds of short-lived noncoding transcripts in mammals. Genome Res 2012; 22:947 - 56; http://dx.doi.org/10.1101/gr.130559.111; PMID: 22369889
- Gruber AR, Fallmann J, Kratochvill F, Kovarik P, Hofacker IL. AREsite: a database for the comprehensive investigation of AU-rich elements. Nucleic Acids Res 2011; 39:D1 D66 - 9; http://dx.doi.org/10.1093/nar/gkq990; PMID: 21071424
- UniProt Consortium. Update on activities at the Universal Protein Resource (UniProt) in 2013. Nucleic Acids Res 2013; 41:D1 D43 - 7; http://dx.doi.org/10.1093/nar/gks1068; PMID: 23161681
- Cook KB, Kazan H, Zuberi K, Morris Q, Hughes TR. RBPDB: a database of RNA-binding specificities. Nucleic Acids Res 2011; 39:D1 D301 - 8; http://dx.doi.org/10.1093/nar/gkq1069; PMID: 21036867
- Ray D, Kazan H, Cook KB, Weirauch MT, Najafabadi HS, Li X, Gueroussov S, Albu M, Zheng H, Yang A, et al. A compendium of RNA-binding motifs for decoding gene regulation. Nature 2013; 499:172 - 7; http://dx.doi.org/10.1038/nature12311; PMID: 23846655
- Helwak A, Kudla G, Dudnakova T, Tollervey D. Mapping the human miRNA interactome by CLASH reveals frequent noncanonical binding. Cell 2013; 153:654 - 65; http://dx.doi.org/10.1016/j.cell.2013.03.043; PMID: 23622248
- Fritsch C, Herrmann A, Nothnagel M, Szafranski K, Huse K, Schumann F, Schreiber S, Platzer M, Krawczak M, Hampe J, et al. Genome-wide search for novel human uORFs and N-terminal protein extensions using ribosomal footprinting. Genome Res 2012; 22:2208 - 18; http://dx.doi.org/10.1101/gr.139568.112; PMID: 22879431
- Lee S, Liu B, Lee S, Huang SX, Shen B, Qian SB. Global mapping of translation initiation sites in mammalian cells at single-nucleotide resolution. Proc Natl Acad Sci U S A 2012; 109:E2424 - 32; http://dx.doi.org/10.1073/pnas.1207846109; PMID: 22927429
- Yamashita R, Suzuki Y, Takeuchi N, Wakaguri H, Ueda T, Sugano S, Nakai K. Comprehensive detection of human terminal oligo-pyrimidine (TOP) genes and analysis of their characteristics. Nucleic Acids Res 2008; 36:3707 - 15; http://dx.doi.org/10.1093/nar/gkn248; PMID: 18480124
- Dassi E, Zuccotti P, Leo S, Provenzani A, Assfalg M, D’Onofrio M, Riva P, Quattrone A. Hyper conserved elements in vertebrate mRNA 3′-UTRs reveal a translational network of RNA-binding proteins controlled by HuR. Nucleic Acids Res 2013; 41:3201 - 16; http://dx.doi.org/10.1093/nar/gkt017; PMID: 23376935
- Kiran A, Baranov PV. DARNED: a DAtabase of RNa EDiting in humans. Bioinformatics 2010; 26:1772 - 6; http://dx.doi.org/10.1093/bioinformatics/btq285; PMID: 20547637
- Sherry ST, Ward MH, Kholodov M, Baker J, Phan L, Smigielski EM, Sirotkin K. dbSNP: the NCBI database of genetic variation. Nucleic Acids Res 2001; 29:308 - 11; http://dx.doi.org/10.1093/nar/29.1.308; PMID: 11125122
- Forbes SA, Bindal N, Bamford S, Cole C, Kok CY, Beare D, Jia M, Shepherd R, Leung K, Menzies A, et al. COSMIC: mining complete cancer genomes in the Catalogue of Somatic Mutations in Cancer. Nucleic Acids Res 2011; 39:D1 D945 - 50; http://dx.doi.org/10.1093/nar/gkq929; PMID: 20952405
- Camacho C, Coulouris G, Avagyan V, Ma N, Papadopoulos J, Bealer K, Madden TL. BLAST+: architecture and applications. BMC Bioinformatics 2009; 10:421; http://dx.doi.org/10.1186/1471-2105-10-421; PMID: 20003500
- Skinner ME, Uzilov AV, Stein LD, Mungall CJ, Holmes IH. JBrowse: a next-generation genome browser. Genome Res 2009; 19:1630 - 8; http://dx.doi.org/10.1101/gr.094607.109; PMID: 19570905
- GTEx Consortium. The Genotype-Tissue Expression (GTEx) project. Nat Genet 2013; 45:580 - 5; http://dx.doi.org/10.1038/ng.2653; PMID: 23715323
- Darty K, Denise A, Ponty Y. VARNA: Interactive drawing and editing of the RNA secondary structure. Bioinformatics 2009; 25:1974 - 5; http://dx.doi.org/10.1093/bioinformatics/btp250; PMID: 19398448
- Kasprzyk A. BioMart: driving a paradigm change in biological data management. Database 2011,2011:bar049.
- Chang SH, Hla T. Gene regulation by RNA binding proteins and microRNAs in angiogenesis. Trends Mol Med 2011; 17:650 - 8; http://dx.doi.org/10.1016/j.molmed.2011.06.008; PMID: 21802991
- Sharma S, Verma S, Vasudevan M, Samanta S, Thakur JK, Kulshreshtha R. The interplay of HuR and miR-3134 in regulation of AU rich transcriptome. RNA Biol 2013; 10:1283 - 90; http://dx.doi.org/10.4161/rna.25482; PMID: 23823647
- Yoon JH, Abdelmohsen K, Srikantan S, Yang X, Martindale JL, De S, Huarte M, Zhan M, Becker KG, Gorospe M. LincRNA-p21 suppresses target mRNA translation. Mol Cell 2012; 47:648 - 55; http://dx.doi.org/10.1016/j.molcel.2012.06.027; PMID: 22841487
- Python. (http://www.python.org/)
- Django. (http://www.djangoproject.com/)
- JQuery User Interface. . http://jqueryui.com/
- Lopes CT, Franz M, Kazi F, Donaldson SL, Morris Q, Bader GD. Cytoscape Web: an interactive web-based network browser. Bioinformatics 2010; 26:2347 - 8; http://dx.doi.org/10.1093/bioinformatics/btq430; PMID: 20656902
- HUGO Gene Nomenclature Committee at the European Bioinformatics Institute. . http://www.genenames.org
- Bult CJ, Eppig JT, Blake JA, Kadin JA, Richardson JE, Mouse Genome Database Group. The mouse genome database: genotypes, phenotypes, and models of human disease. Nucleic Acids Res 2013; 41:D1 D885 - 91; http://dx.doi.org/10.1093/nar/gks1115; PMID: 23175610
- Uhlen M, Oksvold P, Fagerberg L, Lundberg E, Jonasson K, Forsberg M, Zwahlen M, Kampf C, Wester K, Hober S, et al. Towards a knowledge-based Human Protein Atlas. Nat Biotechnol 2010; 28:1248 - 50; http://dx.doi.org/10.1038/nbt1210-1248; PMID: 21139605
- Kozomara A, Griffiths-Jones S. miRBase: integrating microRNA annotation and deep-sequencing data. Nucleic Acids Res 2011; 39:D1 D152 - 7; http://dx.doi.org/10.1093/nar/gkq1027; PMID: 21037258
- Portales-Casamar E, Arenillas D, Lim J, Swanson MI, Jiang S, McCallum A, Kirov S, Wasserman WW. The PAZAR database of gene regulatory information coupled to the ORCA toolkit for the study of regulatory sequences. Nucleic Acids Res 2009; 37:D1 D54 - 60; http://dx.doi.org/10.1093/nar/gkn783; PMID: 18971253
- The Gene Ontology. . http://amigo.geneontology.org
- Vanderkraats ND, Hiken JF, Decker KF, Edwards JR. Discovering high-resolution patterns of differential DNA methylation that correlate with gene expression changes. Nucleic Acids Res 2013; 41:6816 - 27; http://dx.doi.org/10.1093/nar/gkt482; PMID: 23748561
- Kishore S, Jaskiewicz L, Burger L, Hausser J, Khorshid M, Zavolan M. A quantitative analysis of CLIP methods for identifying binding sites of RNA-binding proteins. Nat Methods 2011; 8:559 - 64; http://dx.doi.org/10.1038/nmeth.1608; PMID: 21572407
- Cabili MN, Trapnell C, Goff L, Koziol M, Tazon-Vega B, Regev A, Rinn JL. Integrative annotation of human large intergenic noncoding RNAs reveals global properties and specific subclasses. Genes Dev 2011; 25:1915 - 27; http://dx.doi.org/10.1101/gad.17446611; PMID: 21890647
- Trapnell C, Williams BA, Pertea G, Mortazavi A, Kwan G, van Baren MJ, Salzberg SL, Wold BJ, Pachter L. Transcript assembly and quantification by RNA-Seq reveals unannotated transcripts and isoform switching during cell differentiation. Nat Biotechnol 2010; 28:511 - 5; http://dx.doi.org/10.1038/nbt.1621; PMID: 20436464