Abstract
A real-time water quality monitoring (RTWQM) network was established in the province of Newfoundland and Labrador in late 2001. The network has changed the way river health is assessed in the province and a great deal has been learned in recent years about using this innovation in resource management. This paper summarizes three new developments carried out in recent years using RTWQM data. First, regression models are developed using real-time data as a surrogate for the concentration of important indicators of water quality that have traditionally been determined through manual grab sample collection. Second, regression models are developed for the prediction of water temperature and dissolved oxygen at the real-time water quality stations. A graphical approach is presented that links air temperature to these two important indicators of water quality. Third, control charts are investigated as a means of analyzing the data collected by the network. These charts have traditionally been used in the manufacturing and processing industries, where their usefulness as a quality control tool hinges upon the assumption that observations from the process being monitored are independent random variables. RTWQM measurements are autocorrelated over time and this lack of independence poses a challenge for control chart design. While a time-series approach is suitable for studying short subsets of the data (e.g. hourly measurements collected over the course of 3 to 5 days), the resulting chart does not clearly show when the health of an aquatic ecosystem is being threatened. Replacing the traditional control chart limit lines in favor of water quality criterion limits that better represent the concerns of resource managers is a much more suitable approach to analyzing real-time data.
Un rseau de suivi en temps rel de la qualit de l'eau (RTWQM) a t tabli Terre-Neuve-et-Labrador vers la fin de 2001. Le rseau a rvolutionn la faon dont on value la sant des rivires dans la province. De nombreuses connaissances ont t acquises ces dernires annes propos de l'utilisation de cette innovation dans la gestion des ressources. Cette tude rsume trois nouveaux domaines de recherche et dveloppement effectus dans les dernires annes en utilisant des donnes du RTWQM. (1) Des modles de rgression sont dvelopps en utilisant des donnes en temps rel comme substitut pour la concentration d'indicateurs importants de la qualit d'eau qui a t traditionnellement dtermine par le prlvement manuel d'chantillons. (2) Des modles de rgression sont dvelopps afin de prdire la temprature de l'eau et de l'oxygne dissous aux stations en temps rel. Une nouvelle approche graphique est prsente, qui cre un lien visuel entre la temprature de l'air et ces deux indicateurs importants de la qualit de l'eau. (3) Des diagrammes de contrle sont tudis dans le but d'examiner les donnes recueillies par le rseau. Ces diagrammes ont t habituellement utiliss dans les industries manufacturires et de procds - o leur utilit en tant qu'outil de contrle de la qualit repose sur la supposition que les observations du processus examin sont des variables indpendantes et alatoires. Des mesures du RTWQ sont auto-corrles au fil du temps et ce manque d'indpendance pose un dfi considrable pour la conception d'un tableau de contrle. Quoiqu'un modle de srie chronologique soit convenable pour tudier des sous-ensembles de donnes (p. ex. des mesures prises toutes les heures durant trois cinq jours), le tableau qui en rsulte ne montre pas clairement quel moment la sant d'un cosystme aquatique est en danger. Le remplacement de lignes de contrle graphique traditionnel par des critres de qualit de l'eau qui reprsentent mieux les proccupations des gestionnaires des ressources est beaucoup plus adapt l'analyse des donnes en temps rel.
Introduction
The Newfoundland and Labrador real-time water quality monitoring (RTWQM) network was established by the Water Resources Management Division (WRMD) of the provincial Department of Environment and Conservation in late 2001. It is the first of its kind in Canada to be supported by industry partners and is growing on an annual basis. The data are communicated in near real-time and are made available online to the public via the Department of Environment and Conservation's website. While there are other continuous monitoring networks in Canada, for many, the data are generally not publically available and many were not set up following documented protocols. The Newfoundland and Labrador RTWQM network on the other hand is recognized by the United States Geological Survey (USGS) and was set up following the same protocols used by the USGS (Ziegler, Citation2011). This real-time network has changed the way resource managers monitor the health of aquatic ecosystems in the province. The cumbersome analogue instruments of the past have been replaced by in-stream digital sensors capable of recording a range of water quality indicators over long stretches of time. The hand-written historical records of infrequent measurements that rarely gave insight into water quality on both spatial and temporal scales have been replaced by digital records of water quality measured continuously. Frequent visits to remote monitoring sites are no longer required as in-stream measurements are available in real-time.
The data collected by the RTWQM network has required research and development in recent years. The results of three of these are summarized in this paper:
1. | Can real-time measurements of variables like water temperature and specific conductance be used to predict the chemical concentrations of other water quality indicators that are normally obtained through manual sample collection? Investigations were carried out in the development of regression models that use real-time data as a surrogate for physical properties and chemical concentrations obtained from grab samples. Similar models previously developed for networks in the United States (e.g. Christensen et al., Citation2000; Christensen, Citation2001; CitationRasmussen et al., 2011) increased the accuracy of the estimated chemical and suspended-sediment concentrations in the rivers and decreased the amount of time dedicated to manual sample collection activities. | ||||
2. | Can measurements of air temperature be used to predict water temperature and the concentration of dissolved oxygen at the real-time stations? Researchers outside of Newfoundland (Crisp and Howson, Citation1982; Webb, Citation1987; Stefan and Preud'homme, Citation1993; Pilgrim and Stefan, Citation1995; Mohseni et al., Citation1998; Pilgrim et al., Citation1998; Webb et al., Citation2003) have successfully used historical records of air temperature and water quality for the prediction of important indicators of river health like water temperature and dissolved oxygen but such statistical models have never been developed for the Newfoundland real-time network. | ||||
3. | Can control charts be used for monitoring real-time data and can they help resource managers identify the timing of events that threaten water quality? The statistical process control chart is a time series plot of observations with upper and lower limit lines drawn to help users identify the timing of any unwanted changes in a process. While these charts have been used for many years in the manufacturing industry, they have rarely been used for studying environmental engineering data (Manly, Citation1994; MacNally and Hart, Citation1997; Smeti et al., Citation2007). |
Overview of the RTWQM Network
The network consists of 28 monitoring stations across the province, with expansion ongoing. Water temperature, pH, specific conductance, and the concentration of dissolved oxygen are monitored at each station at 15- to 60-minute intervals using Hydrolab Multiprobe Series Datasonde real-time monitors. The Datasonde design has made it an ideal option for monitoring some of the province's more remote rivers. Communications equipment installed at each monitoring station transmits recorded data to an orbiting satellite which then relays the data to a central depository in the United States from which it can be subsequently downloaded, processed, and analyzed by WRMD resource managers. The RTWQM program follows strict quality assurance/quality control/quality assessment procedures to ensure the integrity of the collected data (CitationHarvey et al., 2011).
Data collected at the four stations operated by the WRMD: NF02YL0012-Humber River, NF02YO0121-Peter's River, NF02ZM0178-Leary's Brook, and NF02ZM0009-Waterford River () are considered in this study. The water quality datasets are described in .
Figure 1. Location of observation stations [solid circles] in Newfoundland and Labrador used in this study. Shaded areas indicate watershed boundaries.
![Figure 1. Location of observation stations [solid circles] in Newfoundland and Labrador used in this study. Shaded areas indicate watershed boundaries.](/cms/asset/5ed96656-9cdd-49f7-b229-8215b964ca2b/tcwr_a_10636602_o_f0001.gif)
Table 1. Summary of the data used in this study.
The measurements of water temperature, pH, specific conductance, and the concentration of dissolved oxygen recorded by the Datasonde were linked to real-time measurements of stage (water level) recorded by Environment Canada. Air temperatures at the stations were estimated using hourly measurements recorded at nearby Environment Canada meteorological stations located 5 to 50 kilometres from the real-time water quality stations. No air temperature data are available at the location of the real-time stations. Manual grab samples collected during 20042008 provided measurements of physical properties and chemical concentrations that the Datasonde is not capable of measuring. A summary of the water quality variables recorded in the grab samples collected at the stations is given in .
Table 2. Ranges of sample measurements in relation to the CCME guidelines.
Regression Models for the Prediction of Grab Sample Water Quality
Regression models were developed using datasets consisting of historical records of grab samples paired with real-time measurements collected at the same date and time (i.e. a grab sample collected at the Humber River station on May 15, 2005 at 14:00 was paired to Datasonde measurements collected as close to that hour as possible). If no real-time measurement was obtained within 4 hours of grab sample collection that grab sample was not used in model development.
Model development following the procedure of Helsel and Hirsch (Citation2002) was carried out using Datafit curve fitting software. The best fitting models were determined based on an interpretation of the residual sum of squares, standard error, the probability values for the explanatory variables, Mallow's Cp and the adjusted R2. A summary of the regression models for select grab sample water quality variables of interest to the WRMD is presented in . These models represent the first attempt at developing a method of predicting grab sample variables for the real-time stations and have not been validated with additional data that were not used in model development. These models will be re-evaluated as more grab sample data become available in the future. A complete description of model development can be found in CitationHarvey (2010).
Table 3. Regression model results relating grab sample concentrations to water temperature (WT), specific conductance (SC) and stage (ST).
Alkalinity
Alkalinity represents the sensitivity of a river to acidic inputs. Rivers with an alkalinity of 010 mg/L CaCO3 are highly sensitive to acidic pollution, 1020 mg/L CaCO3 are moderately sensitive, and>20 mg/L CaCO3 are not sensitive (Inventory Resources Committee, Citation1998). Although no statistically significant regression model could be developed using real-time data for Humber River alkalinity, alkalinity levels change very little throughout the year at this station (mean concentration 13.12 mg/L CaCO3). Regression models for Humber River were deemed unnecessary as historical records indicate the health of the river is not under threat (alkalinity remains close to 13 mg/L throughout the year). If future grab sample collections indicate a change in alkalinity it will be necessary to revisit model development for this station in the future.
Alkalinity in the Waterford River grab sample dataset is of more concern for modelling purposes. While the mean concentration is 13.65 mg/L CaCO3, four of the twenty observations are less than 10 mg/L. Acidic inputs at these lower alkalinity levels can potentially cause an immediate change in pH and threaten acid-intolerant forms of aquatic life. Specific conductance and stage were used as independent variables to estimate alkalinity at this station with an adjusted R2 of 0.799.
The alkalinity data in Leary's Brook showed 15 of the 19 observations having alkalinity less than 10 mg/L CaCO3 and seven of those were less than the detection limit of 5 mg/L CaCO3. With such low concentrations of alkalinity, acidic inputs could severely impact the health of the aquatic environment. With about 40% of the data being censored, it was decided that more data are required before developing models for alkalinity in Leary's Brook.
Hardness and Total Dissolved Solids
Hardness is used as a general measure of the amount of calcium, magnesium, and iron present in a water body. The variation in Humber River water hardness is quite limited; similar to alkalinity there is little need to develop a regression model for hardness at this station at this time. Water temperature and specific conductance were used as surrogates for Leary's Brook and Waterford River water hardness.
The concentration of total dissolved solids (TDS) refers to both the inorganic and organic material dissolved in the water. While TDS concentrations in freshwater naturally range from 01000 mg/L, fluctuations in TDS can occur as the result of pollution, such as the spreading of road salt during icy winter conditions (Inventory Resources Committee, Citation1998). TDS concentrations at the real-time stations are less than 1000 mg/L. The concentrations in Leary's Brook and Waterford River are higher than in Peter's and Humber Rivers. Large spikes in TDS during the winter months () at these two urban rivers are likely the result of road salting. Specific conductance was used as an independent variable to estimate TDS in Peter's River (adjusted R2=0.83) and Leary's Brook (adjusted R2=0.85). The model for the Waterford River had the best fit (adjusted R2=0.90, ). Real-time measurements could not be used as a surrogate for Humber River TDS because the range of concentration in the grab samples was too small (2234 mg/L, ).
Figure 2. Grab sample Total Dissolved Solids concentrations collected at Humber River, Peter's River, Leary's Brook and Waterford River.
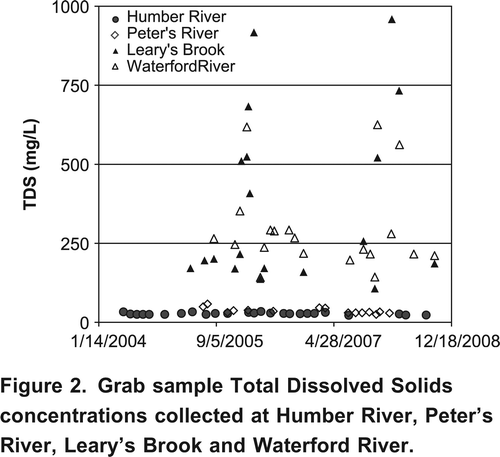
Major Elements
Developing regression models for variables such as the concentration of calcium, chloride, sodium, and sulphate is much easier for smaller urban rivers like Leary's Brook and Waterford River than it is for a larger rural river like the Humber River. Variations in the concentration of major ions are larger in the smaller rivers and linking these variations to RTWQM data is more successful. Chemical inputs into a larger water body like the Humber River are heavily diluted and only rarely shift chemical concentrations away from their natural occurring levels. According to the currently available grab sample data for the Humber River, grab sample chemical concentrations vary little throughout the year and remain well below defined guidelines for the protection of aquatic life.
Metals
Antimony, arsenic, mercury, nickel, and selenium were all undetected in grab samples taken at the real-time stations. The concentrations of barium, chromium, magnesium, and manganese from grab samples show little variation throughout the year (e.g. Waterford River magnesium is in the range of 1.02.5 mg/L). Developing regression models for chemical concentrations that show only limited concentration variation is not meaningful.
The majority of grab sample chromium, copper, lead, and aluminum concentrations were below Canadian Council of Ministers of the Environment (CCME) guidelines for the protection of freshwater aquatic life. Occasionally, grab samples in the historical datasets exceed the CCME guidelines. For example, in the Leary's Brook dataset 18 of the 19 copper samples are in the 0.0010.004 mg/L range and one sample of 0.006 mg/L is greater than the CCME limit of 0.004 mg/L. When there is such little variation in concentration, regression models are not possible, but samples exceeding the guidelines demonstrate the need for ongoing monitoring.
Zinc can be toxic to organisms in aquatic environments. Although the grab samples collected at Humber River, Peter's River, and Waterford River never exceed the CCME limit of 0.03 mg/L, at Leary's Brook five samples were greater than this limit. These measurements all occur during February and March, when specific conductance at the station is high. There is considerable scatter in the zinc measurements; a simple linear regression model using specific conductance has a low adjusted R2 of 0.684. While a logistic or sigmoid function fitted using non-linear regression using specific conductance as an explanatory variable provides a slightly improved fit (adjusted R2=0.750), more data are required to validate a predictive model.
Regression Models for Predicting Water Temperature and Dissolved Oxygen
The ability to monitor and assess water temperature is an essential component of effective resource management as temperature influences a wide range of biological and chemical processes that are present in a river system. A variety of empirical regression models were considered in this research for predicting mean, maximum, and minimum water temperature at the RTWQ stations at the monthly, weekly, and daily time scales in CitationHarvey et al. (2011). A three-parameter logistic model (e.g. Mohseni et al., Citation1998) was found to describe the S-shaped relationship between air and water temperature at the stations, while an exponential model best described the relationship between water temperature and dissolved oxygen.
Regression models for water temperature and DO were brought together using a graphical procedure (). The 3-way plot links user defined air-water temperature and water temperature-DO models in one image. Pairing the regression models through this simple method should prove useful in the ongoing assessment of water quality in rivers monitored by the Newfoundland RTWQ network. All the models developed were validated using data not used for model development (CitationHarvey et al., 2011).
Figure 5. Nomogram linking air temperature to water temperature and then dissolved oxygen. The dotted/dashed line provides an example of its use during the cooling season for the Humber River; an air temperature (16C) links to a water temperature (17C) and then to an expected dissolved oxygen concentration in the river (9 mg/L).
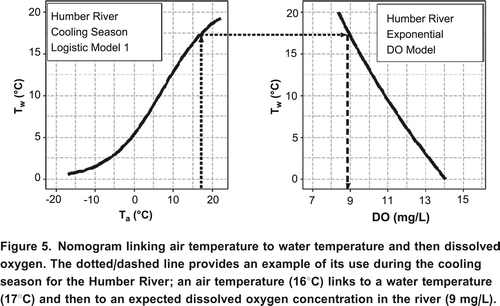
The Modified Control Chart
All physical and chemical processes have a certain amount of inherent natural variation. From a quality control perspective, any process that operates solely in the presence of this noise is said to be in a state of statistical control. However, variations in these processes may make the time series go out of statistical control. A frequently used laboratory method for discovering these departures is a Shewhart control chart ().
Figure 6. The Shewhart control chart uses upper and lower control limits (dashed lines) to identify deviations from the process mean (center line).
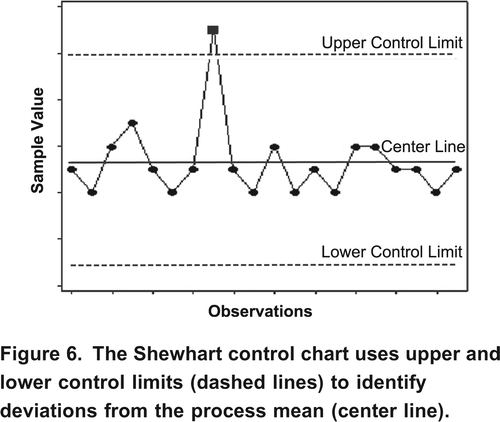
The process mean on the standard Shewhart chart is assumed to be known and the upper and lower control limits are defined according to a set of statistical rules. These control limits help process managers identify variability due to some influence that is not part of the normal process (assignable cause). The sample variations for a process in a state of statistical control will stay within the three-sigma (standard deviation) control limit lines. When a number of points in a row plot outside these lines it is likely that the process has reached an out of control state and actions would have to be taken to correct the problem.
Initial investigations into using the Shewhart chart to study RTWQM data were problematic as control charts only provide useful insights if it can be assumed that observations are independent and identically distributed about some mean level. MacNally and Hart (Citation1997) note that a control chart's effectiveness for assessing environmental data hinges upon the assumption of independence, as the presence of autocorrelation will increase the false alarm rate of the chart (where what looks to be a statistically significant change or problem on the control chart is not really a problem at all). Environmental processes rarely satisfy this set of conditions as observations tend to be highly autocorrelated. For example, while hourly pH may fluctuate over the course of a month, measurements taken an hour apart will usually be very similar.
One approach for handling the autocorrelation problem was proposed by Alwan and Roberts (Citation1988); an autoregressive integrated moving average (ARIMA) model is fit to a set of autocorrelated observations and a control chart is used to study the uncorrelated residuals from that model. The Box-Jenkins methodology is used when determining which ARIMA model would best fit a set of observations, where the type of model to be used is first identified, then the parameters of the model are estimated, and the residuals from the fit of the model are checked for normality, constant variance, and independence (Box et al., Citation1994). While this approach is not suitable for studying large amounts of real-time data (i.e. fitting an ARIMA model to one month of highly autocorrelated data was incredibly difficult), it may be suitable when studying smaller, less autocorrelated subsets of the real-time data. For example, five days of Humber River hourly pH can be modelled by an AR(1) process () and the uncorrelated residuals can then be studied using a standard Shewhart chart (). The control chart () shows two observations outside the upper and lower limits (6.99 and 6.93 pH units).
Figure 8. Uncorrelated residuals from an AR(1) time series model fit to five days of Humber River hourly pH plotted on a traditional Shewhart control chart. The dashed lines show the upper and lower confidence limits, while the center line shows the mean.
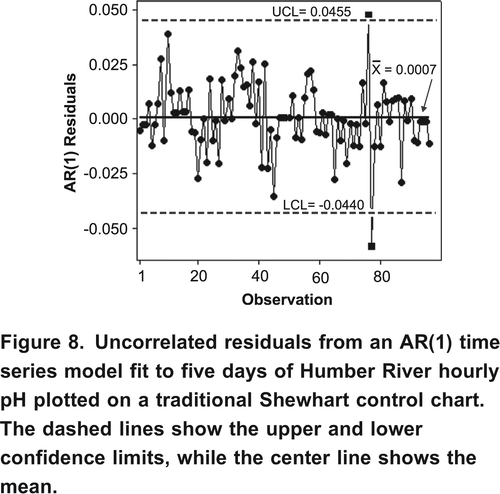
Although the ARIMA approach can be used to remove autocorrelation, there is still the problem that the chart is not telling a resource manager anything useful. The traditional control chart has flagged two points as being problematic based on a predetermined set of statistical rules not according to water quality guidelines. Control charts were never originally designed to study a process with so much natural variation and an entirely new approach to the problem is required as a result.
Figure 9. Modified control chart used to study one month of Humber River hourly pH. The upper and lower control limits (dashed lines) are defined according to CCME guidelines for the protection of aquatic health. Points outside these guidelines can be quickly identified using this simple approach to data screening.
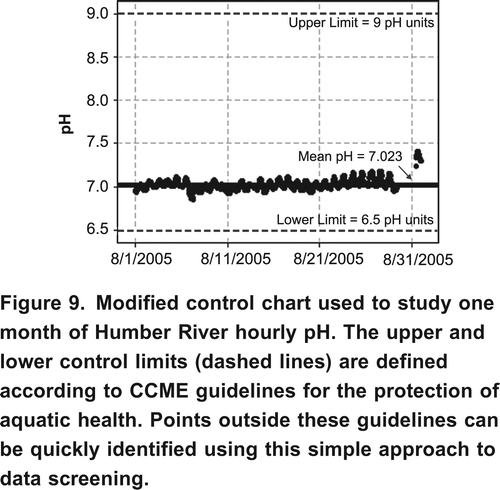
Modified control charts were developed that ignore the statistically defined limits of the traditional control chart in favor of limits defined according to water quality guidelines for the protection of aquatic life. These charts eliminate the need to satisfy the conditions of independence and constant variance and make analyzing highly autocorrelated data simple and straightforward. These modified non-statistical control charts can be used to quickly study long lengths of collected data (e.g. ). The potential for advanced warnings to regulators that a water quality variable is on an upward or downward trend or is getting close to the defined water quality limit, etc. is being explored.
Acknowledgements
Funding for this research was provided by the Natural Sciences and Engineering Research Council of Canada (NSERC), the Newfoundland and Labrador Department of Environment and Conservation, the Institute for Biodiversity, Ecosystem Science and Sustainability (IBES), and the School of Graduate Studies at Memorial University. The insight and assistance of Ms. Renee Paterson of the Department of Environment and Conservation are gratefully acknowledged.
References
- Alwan , L. and Roberts , H. 1988 . Time series modeling for Statistical process control . Journal of Business and Economic Statistics , 6 ( 1 ) : 87 – 95 .
- Crisp , D. and Howson , G. 1982 . Effect of air temperature upon mean water temperature in streams in the North Pennines and English Lake District . Journal of Freshwater Biology , 12 : 359 – 367 .
- Harvey , R. , Lye , L. , Khan , A. and Paterson , R. 2011 . The influence of air temperature on water temperature and dissolved oxygen in Newfoundland rivers . Canadian Water Resources Journal , 36 ( 2 ) : 171 – 192 .
- MacNally , R. and Hart , B. 1997 . Use of CUSUM methods for water-quality monitoring in storages . Journal of Environmental Science and Technology , 31 : 2114 – 2119 .
- Mohseni , O. , Stefan , H. and Erickson , T. 1998 . A nonlinear regression model for weekly stream temperatures . Journal of Water Resources Research , 34 ( 10 ) : 2685 – 2692 .
- Pilgrim , J. , Fang , X. and Stefan , H. 1998 . Stream temperature correlations with air temperature in Minnesota: implications for climate warming . Journal of the American Water Resources Association , 345 ( 5 ) : 1109 – 1121 .
- Smeti , E. , Koronakis , D. and Golfinopolous , S. 2007 . Control charts for the toxicity of finished water-modeling the structure of toxicity . Water Research , 41 ( 12 ) : 2679 – 2689 .
- Stefan , H. and Preud'homme , E. 1993 . Stream temperature estimation from air temperature . Water Resources Bulletin , 29 ( 1 ) : 27 – 45 .
- Webb , B. 1987 . The relationship between air and water temperature for a Devon river . Reports and Transactions of the Devonshire Association for the Advancement of Science , 119 : 197 – 222 .
- Webb , B., P. , Clack , P and Walling , D. 2003 . Water and air temperature relationships in a Devon river system and the role of flow . Hydrological Processes , 17 : 3069 – 3084 .