Abstract
Uncertainty in hydrometric data is a fact of life. Basic assumptions about the nature of this uncertainty are necessary in every analysis of hydrometric data, and an understanding of the variability of uncertainty can facilitate the effective use of hydrologic information. For most of the twentieth century there has been little change in hydrometric methods and many analysts explicitly or implicitly assume that the uncertainty has not changed over the period of record. We argue that there is substantial variability in the magnitude of uncertainty in published streamflow records that is not transparent to data users. Quantifying uncertainty is particularly important in the context of the current changes in hydrometric technology and in the increasing integration of data sets from multiple providers. We recommend best practices for identifying uncertainty in field notes and propagating that observational uncertainty through the data production process. We suggest both field and reanalysis studies that could be undertaken to improve understanding of hydrometric uncertainty. We also recommend improvements in management practices, including preservation of relevant metadata and a suitable period of overlap for new and old observing systems to allow assessment of the effects of changing technology.
L'incertitude entourant les donnes hydromtriques est un fait concret. Les hypothses de base quant la nature de cette incertitude s'avrent ncessaires pour chaque analyse des donnes hydromtriques et une comprhension de la variabilit de l'incertitude peut faciliter l'utilisation efficace des donnes hydrologiques. Pendant la majeure partie du vingtime sicle, ces mthodes ont connu peu de changements et de nombreux analystes posent comme hypothse, de manire explicite ou implicite, que l'incertitude n'a pas volu au cours de la priode de relev. Nous faisons valoir qu'il existe une variabilit considrable dans l'ampleur de l'incertitude lie aux enregistrements des dbits des cours d'eau publis, laquelle n'est pas transparente aux yeux des utilisateurs de donnes. Il est particulirement important de quantifier l'incertitude dans le contexte des changements actuels qui touchent les technologies hydromtriques et de l'intgration croissante des ensembles de donnes provenant de fournisseurs multiples. Nous recommandons les pratiques exemplaires pour la dtermination de l'incertitude dans les notes de terrain et la diffusion de cette incertitude observationnelle par l'entremise du processus de production de donnes. Nous proposons la possibilit d'entreprendre la fois des tudes sur le terrain et de nouvelles analyses dans le but d'amliorer la comprhension de l'incertitude hydromtrique. Nous recommandons galement d'apporter des amliorations aux pratiques de gestion, notamment la conservation des mtadonnes pertinentes et le recours une priode approprie de chevauchement des anciens et nouveaux systmes d'observation pour faciliter lvaluation des effets de lvolution de la technologie.
Introduction
Production of streamflow records involves a variety of inputs, typically periodic measurements of stream discharge and continuous records of stream stage. Each of the measured inputs is inexact, and the component inaccuracies contribute to an aggregate error in the published streamflow record. This aggregate error includes effects associated with the skill of the hydrographer, accuracy and precision of all instruments used, environmental factors that influence the quality of measurement, the validity of the derivation method (e.g., the development of a rating curve for estimating discharge from stage), and the rigor with which systematic errors are identified, quantified and corrected prior to publishing the data. It is improbable that all systematic errors be known and have been corrected and that all random sources of error happen to cancel each other at the specific time of observation. Therefore, the presence of uncertainty in streamflow data is, like death and taxes, amongst the few certainties in life.
In this paper, we first present arguments for the production and publication of quantitative uncertainty estimates for data values in a hydrometric record. We then draw upon our experiences as hydrometric technologists with the Water Survey of Canada (WSC) to illustrate some sources of uncertainty, and their space-time variability, that are present in historic hydrometric records but not transparent to data users. The paper ends with recommendations for advancing methods for quantification and propagation of uncertainty in the context of a hydrometric data quality management framework. These recommendations include considerations for the development of best practices for identifying uncertainty in the original field observations, management of technological and procedural change, and preservation of metadata needed to discover cause and effect relations between hydrometric practice and discharge uncertainty.
Arguments for Quantifying Uncertainty in Hydrometric Records
Streamflow data from major hydrometric agencies are typically provided with an indicator (in the form of a symbol, flag or grade associated with each value) that may be loosely interpreted as a categorical measure of uncertainty. For example, WSC uses a symbol of B to indicate periods when ice cover influences the relation between stage and discharge, and E to indicate periods when the discharge values have been generated by a method that deviates from that normally used at a station. Data that are unqualified by such an indicator are often assumed to have low uncertainty, while qualified data are generally deemed to have higher uncertainty. This solution, while pragmatic and effective for screening data that have errors that are not identically distributed with respect to the main population, is fundamentally flawed, because categorical indications of data quality are not based on an estimate of uncertainty but rather an acknowledgement of a deviation in the method used to produce the data. For example, data in a WSC record graded with an E may in one instance be well informed by redundant (i.e., highly correlated) data sources, whereas in a different instance the estimate may be guided by information that is only weakly correlated to the target signal (e.g., streamflow from a catchment that is fundamentally different from the target catchment). Similarly, data graded with a B may in some cases be based on recorded stage combined with relatively frequent discharge measurements to generate shift corrections to the rating curve, while in others they may be based on subjective interpolation supported by a single measurement during the ice-cover period, which can last six months or more at northern sites.
Because there is no universal convention on data grading, each hydrometric data provider must decide whether, and how, to identify data that do not conform to the typical production process. As is clear from the examples in the preceding paragraph, the correct interpretation of data grades requires that the analyst have a high degree of familiarity with the data production process for each location. In reality, we have observed that many analysts simply ignore the data grades and process the data as if they conform to the assumption that all errors are randomly distributed and follow a common probability distribution. Data from agencies such as U.S. Geological Survey (USGS) and WSC are often assumed by analysts to be accurate and precise to within 5% at the 95% confidence interval even though neither agency would make that claim.
Many analyses, such as the identification of hydrologic change associated with land use or climatic fluctuations, require long records (typically several decades) with consistent data quality. However, pressures on network managers to reduce costs or re-allocate resources often result in records that are too short and fragmented to be of use. The need to mitigate for spatial and temporal gaps in hydrometric records has led to the development of advanced statistical methods in regional analysis to combine information from many, relatively short, records to generate robust estimates for ungauged catchments and to extend records at formerly gauged sites. In such a process, a quantitative estimate of uncertainty could potentially be used to weight the contribution of each data value used as a predictor, thus reducing the influence of information from sites or periods with higher uncertainties.
Inter-comparability of data is particularly relevant given the development of interoperability standards. A memorandum of understanding between the World Meteorological Organization (WMO) and the Open GeoSpatial Consortium on development of the WaterML 2.0 data standard is likely to lead to a future in which hydrometric data from any data provider are potentially discoverable and accessible by data users. Data sharing may soon become the rule rather than the exception. In the context of regional analysis using data obtained through data sharing, data could have inconsistent but unknown patterns of uncertainty over time or among stations. An uninformed analyst, working on the assumption that all published records conform to a uniform standard, would have no basis for downweighting data values with higher uncertainties, thus reducing the robustness of the derived records.
Continuous quantification of time-series uncertainty would also be valuable in the calibration and testing of streamflow models. Particularly when an automated calibration procedure is used, model parameters could be distorted by forcing a simulated hydrograph to fit streamflow values with high uncertainty (Beven and Westerberg, Citation2011). Given a quantitative uncertainty estimate, each streamflow value could be weighted in the calculation of the loss function so as to put more weight on the values with greater certainty.
Publication of quantitative estimates of uncertainty is also needed to address changes in methodology that could influence the variance of discharge records around the true values (i.e., precision) and their bias (i.e., accuracy). The presence of bias is particularly important in the assessment of hydrologic change. For example, the earlier periods in many of the longer hydrometric records in western Canada were based on manual stage measurements. Particularly in melt-dominated hydrologic regimes, the shift from manual to continuous stage records could introduce a spurious shift in streamflow records that would be confounded with the underlying true signal associated with environmental change.
Sources of Uncertainty in Streamflow Records
The typical approach to producing streamflow records involves three components: (1) observations of stream stage, (2) periodic measurements of streamflow, and (3) development of a rating curve to convert stage to discharge. If the uncertainties in these three components can be quantified, the error in the derived streamflow records can be estimated using the law of propagation of uncertainty as described in documents such as ANSI/NCSL Z540-2-1997 (American National Standards Institute/American National Conference of Standards Laboratories, Citation1997). Each component is addressed below.
The uncertainty in staff gauge readings can be quantified by the resolution of the instrument. Resolution of the instrument is the precision to which the instrument can be read and is less than or equal to the smallest division that is marked on the instrument. Standard metric (SI) gauge plates are typically marked in 5 mm increments and can be read to the nearest millimetre, so the resolution of the instrument for a standard metric gauge plate is 0.001 m. Imperial (SAE) gauge plates are usually marked in increments that can be read to 0.01 ft. Hence, the instrument limit of error of an imperial gauge plate is about 3 times that of a metric gauge plate (0.01 ft 0.003 m). However, the true error of a staff gauge reading may have less to do with the resolution of the instrument and more to do with environmental conditions. Poor lighting, very clear water and wave action have all been cited as reasons for inaccurate staff gauge readings (Sauer and Turnipseed, Citation2010). Whereas measurement uncertainty could be evaluated by making repeated measurements of the same true value, time is of the essence during a typical field visit. As a result there is typically a trade-off to be made between measurement duration and uncertainty. A nominal uncertainty (say 0.003 m) is typically adopted for an instantaneous reading rather than having a protocol of making replicate readings over some duration of time that produces both an observation and an estimate of observational uncertainty.
In principle, a continuous measure of gauge height uncertainty could be obtained by installing redundant sensors at each station. However, cost effectiveness is of paramount importance in hydrometric monitoring. In practice, therefore, any additional monitoring resources are in far greater demand to be deployed elsewhere in ungauged basins. As a result, the uncertainty of recorded stage data is almost never known.
Several research studies have quantified uncertainty in discharge measurements using both replication of measurements and propagation of errors (e.g., Pelletier, Citation1988). Measurement of uncertainty by replication of observations is impractical in most operational settings and is not even possible when field conditions are severe and discharge is changing rapidly. Application of the uncertainty equation to standard uncertainties (Herschy, Citation2009) is based largely on the notion of resolution of the instrument. The Interpolated Variance Estimation (IVE) method (Kiang et al., Citation2009) is being investigated as a potential method for quantifying the uncertainty of discharge measurements as a function of the spatial auto-correlation of individual observations in a cross-section. Some agencies, such as the USGS, qualify all discharge measurements with a subjective estimate of uncertainty. Other agencies such as WSC do not keep such records. All three methods of uncertainty estimation systematically show negative bias with respect to uncertainties determined by replicate measurements for uncertainties greater than about 4% (Kiang et al., Citation2011).
Uncertainty in the fitting of a rating curve can result in significant uncertainty in the derived discharge record (Clarke, Citation1999; Jonsson et al., Citation2002). Sources of uncertainty result from: (1) the choice of functional form (e.g., single power-law versus multiple segments), (2) the uncertainties in the stage and discharge measurements used to fit the relation, (3) uncertainties associated with extrapolating the curve outside the range of gauged flows, and (4) shifts in the stage-discharge relation associated with in-stream vegetation growth or channel change (Schmidt, Citation2004). At sites with stable rating curves, the scatter of points around the fitted relation will reflect the effects of random errors in discharge and stage measurements.
At locations where the rating curve can be considered stable, measurement conditions also tend to be favourable, leading to relatively low uncertainty. It is the locations that have both high measurement uncertainty and an unstable rating that are of greater concern. Aquatic vegetation, for example, presents a condition that will result in both high measurement error, as a result of indefinite resolution of the effective flow boundary, and an unstable rating as the annual life cycle of the plants impact on cross-sectional area available for flow as well as introducing changes in flow resistance.
A decision threshold of 5% is commonly used to evaluate whether a departure from the base curve is due to measurement error (less than 5%) or model error (greater than 5%). If the control feature is known to be unstable (e.g., in streams with a high rate of sediment transport), then deviations greater than 5% will generally trigger a shift in the rating. If the control feature is known to be stable (e.g., bedrock control) then some deviations greater than 5% may be tolerated and assumed to be measurement error until there is an accumulation of evidence that a rating shift has occurred. In other words, deviation from an established rating curve is used as a de facto measure of uncertainty. Unfortunately, it can be difficult to ascertain whether a deviation from a rating curve is a result of measurement uncertainty or a result of a change in the control conditions. The decision to shift the rating curve to every measurement with a deviation greater than 5% would introduce a systematic change in discharge derivation as a result of what may only be random measurement error.
It is possible to estimate the uncertainty of a rating curve provided there are enough rating measurements. Herschy (Citation2009) recommended at least 20 measurements per rating curve segment for a statistically acceptable estimate of rating curve uncertainty. In practice, a shift correction to a rating curve can be defined by as little as a single measurement, which does not allow for a robust estimate of uncertainty for discharge values derived while the shift is in effect. Consequently, the uncertainty of the shifted curve should be greater than the uncertainty of the base curve, so a gauging station record that involves frequent curve shifts should have higher uncertainty in the derived discharge than a location with a stable base curve. Unfortunately, metadata about rating curve stability is relatively inaccessible to the general public for evaluation of discharge records. Such information would be valuable for differentiating systematic departures from the base rating that are well supported by successive measurements from departures for which no confirmation is available.
The origin of the 5% guideline for discriminating between measurement and model error pre-dates readily available reference sources but it is thought this value may be based on sampling theory for a standard, 20-panel, discharge measurement. Error in the derived discharge time-series is sensitive to the agreement of the decision threshold with true measurement uncertainty. Where true measurement uncertainty is greater than 5% some shifts will be erroneously applied to the rating curve, whereas if true measurement uncertainty is less than 5% then there is a risk that some changes in the actual control conditions will not be correctly identified, should the changes in conditions be relatively minor.
The use of a decision threshold that is constant through time for updating rating curves may be problematic in the face of changes in measurement methodology. Oberg and Mueller (Citation2007) investigated Acoustic Doppler Current Profiler (ADCP) measurements and found differences in variance with respect to the reference measurements used. These differences in variance will have the potential to change the frequency with which measurements plot outside of the 5% decision threshold coincident with a change from current meter to ADCP technology.
Another area of concern is in the extrapolation of rating curves. Opportunities to measure peak flows arise infrequently and are typically of short duration. Measurement conditions are often particularly unfavourable during either low or high events. These measurements have high leverage on the shape of the rating curve where it extends outside the range of measured discharge. It is not unusual to see a rating curve fit exactly through the most extreme measurement (as if it were without error). It may take years, or even decades, to get another measurement point for validation of the rating curve extrapolation by which time a shift in the rating curve may have occurred. The random uncertainty of these measurements will translate into systematic errors in the derived extreme values until such a time as there are additional measurements to reduce the bias. These systematic errors will persist in the record unless revision to the rating curve is sufficiently large to trigger the organization's data revision criteria (e.g., WSC will revise data if a data review indicates a change of 50% or more in daily discharge, 10% in monthly mean discharge, or 15% in an annual extreme value). Unfortunately, metadata about the measurements used to define rating curves are relatively inaccessible to the general public for evaluation of discharge records.
Faults in the stage sensing and recording system create data gaps that must be filled by estimation. Discharge estimation is frequently required even when there are valid stage data, such as during episodes when the stage variability is dominated by ice in the channel or by beaver dam activity. A wide variety of flow estimation methods are used, each of which is subject to assumptions about the relations between predictor variables and discharge. Unfortunately, most hydrometric data providers do not publish metadata about the flow estimation methods used or of any change in assumptions implicit in those methods.
The uncertainty in the observations made over a period of time can be aggregated into uncertainty for a time-averaged value. Hydrometric data are typically published at daily resolution. The uncertainty of the daily aggregate is a function of the effective coverage of the day with observations. Days with substantial gaps in coverage will have greater uncertainty than days that have been regularly sampled throughout the day. Historically, the period of record for many locations includes extended periods of time when the gauge was read once per day by an observer. Information about the method of observation can usually be discovered within the location metadata but the impact on data uncertainty will vary from location to location and with time of year, depending on the amount of variability in stage or discharge that can occur in one day (e.g., a slow response stream versus a flashy stream, which can be related to the size of the watershed).
Case Studies from the Historic Record in Canada
In this section, we draw upon a set of case studies to illustrate the nature of uncertainties inherent in streamflow records, which are not clearly documented in readily available metadata.
Evolution of Rating Curves
The early stages of the evolution of a stage-discharge rating at a WSC gauging station established in 1998 is used as an example of the disproportionate leverage that peak flow measurements have on the shape of a rating curve. The two rating curves shown in Citation Figure 1 diverge at the top end of the rating. The dashed line shows rating curve 2, which was in effect during the 1999 calendar year, and the solid line shows rating curve 3, which was in effect for the first six months of the year 2000. Rating curve 2 is highly influenced by the highest measurement available at the time (40.9 m3s1) whereas rating curve 3 was influenced by an even higher discharge measurement (88.8 m3s1).
Figure 1. Illustration of the effect of rating curve evolution for Carbon Creek near the mouth (07EF004). The dotted line shows rating curve 2 and the dashed line shows rating curve 3. The solid dots indicate the measurements used to define curve 2; the open circles indicate additional measurements available to define curve 3.
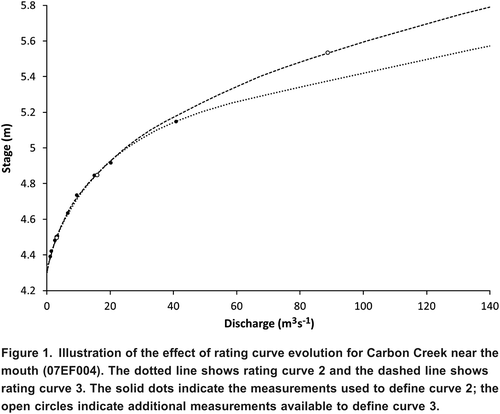
Rating curve 2 was extrapolated to the stage of the peak flow event with an estimated discharge (181 m3s1) about four times as high as the highest measurement (40.9 m3s1). Had rating curve 3 been applied to the highest stage in 1999, then the peak discharge would have been estimated as 25% lower than what has been published. The 1999 peak flow is graded as estimated so revision criteria do not apply and the originally published value stands, even though the true value is almost certainly smaller in magnitude than the published value.
Additional measurements obtained over a longer period of time, as shown in Citation Figure 2 , indicate that initial judgements about the shape of the rating curve may have been mis-informed by inherent measurement uncertainty. The solid line shows the most recent rating curve and the dashed and dot-dash lines show rating curves 2 and 3 for comparison.
Figure 2. Illustration of the effect of rating curve evolution for Carbon Creek near the mouth (07EF004). The solid line shows curve 12. The dotted line shows curve 2 and the dashed line shows curve 3. Solid dots indicate measurements used to define curve 2; open circles indicate additional measurements used to define curve 3; open triangles indicate additional measurements used for curve 12.
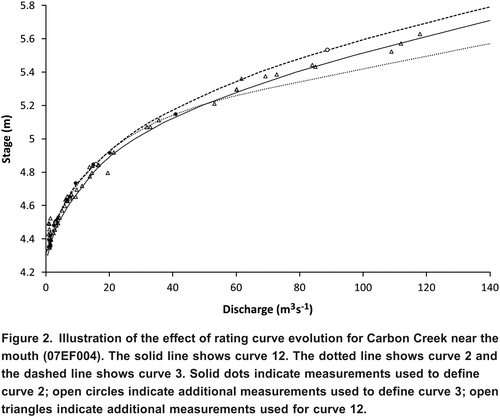
The plot of rating measurements for Thompson River near Spences Bridge (Citation Figure 3 ) is illustrative of the relevance of measurement variance in the data production process. In this case, 197 measurements were acquired over a period of 60 years. With the benefit of hindsight, a single segment rating curve can be fit through all of the measurements with just 10 deviations greater than 7.5%, consistent with estimates of measurement uncertainty at the 95% confidence interval (e.g., Pelletier, Citation1988). In fact, nine different rating curves have been used to produce discharge at this station. The 5% decision threshold contributes to the number of times the curve has been either shifted or modified. Sometimes the threshold is exceeded by accumulation of new information (e.g., curve extension), sometimes the change may be a result of actual change in the control conditions, but sometimes the threshold exceedence may simply reflect random measurement error. There is a risk that if the decision threshold is not well calibrated to the actual uncertainty of the measurement method then spurious modifications to the rating curve are possible.
In contrast to the Thompson River, where there are sufficient measurements for resolution of the rating curve, there are many streams for which the stage-discharge relation is largely notional. The rating measurements and shift curves, as shown in Citation Figure 4 for Ekapo Creek, illustrate the magnitude of variability that can be attributed to beaver activity. These shift curves are defined by as few as one measurement so the quality of the record at a location like this is largely a function of the measurement frequency. Single point shift corrections are uninformative about both measurement and model uncertainty. Confidence in the measurement and in the form of the shift curve can only be based on close inspection and interpretation of field notes.
Figure 4. Ekapo Creek near Marieval (05JM010) rating curve and shift curves used for the 2007 data year. The solid line indicates the base rating curve; the open circles indicate the rating measurements; the dashed lines indicate the shifts applied over the summer of 2007 (identified by mm-dd). The shifts are due to beaver activity.
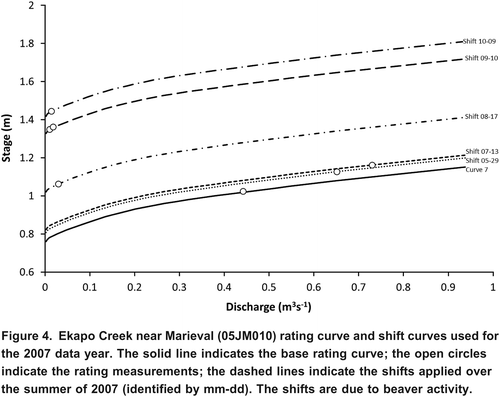
Estimation of Streamflow Under Ice
The methods used to estimate winter streamflow vary widely. Melcher and Walker (Citation1992) investigated 17 different methods in use by the USGS for winter streamflow estimation and noted significant differences in results depending on method. The method most frequently used in Canada is subjective interpolation. Two examples are provided to illustrate the impact of change in subjective assumptions on the resultant hydrographs.
Chin (Citation1966) inferred that temperature has an effect on winter streamflow variability based on high frequency measurements conducted during the winter of 19641965 on the Takhini River. The winter hydrographs shown in Citation Figure 5 illustrate the impact of this study on winter streamflow estimates. The first set of hydrographs (19541963) pre-date the Chin study and a smoothly varying winter hydrograph is evident in some years, with step changes in others. Following Chin's (1966) study, hydrographs for 1965 to 1982 exhibit synoptic scale variations around the general recession trend, which were subjectively interpreted by hydrometric technicians in the Whitehorse office based on an assumed positive correlation between air temperature and streamflow. From 1983 on, winter hydrographs dominantly reflect a reversion to the original assumption of a smoothly varying winter recession.
Figure 5. Illustration of the effect of changes in procedures for subjective estimation of winter streamflow for Takhini River near Whitehorse (09AC001).
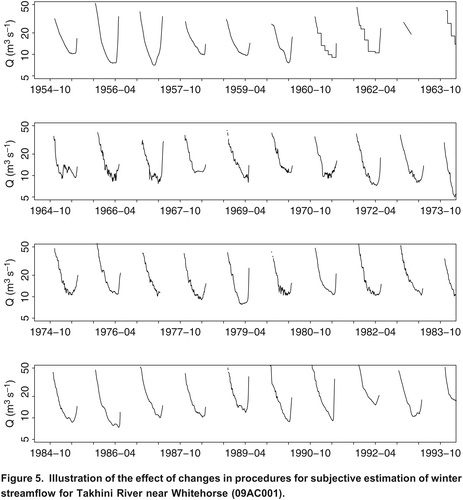
The concept of storage-related decreases in flow was described by Gray and Prowse (Citation1993) using examples from the Liard and Mackenzie Rivers and shown to occur more generally by Moore et al. (Citation2002). The magnitude of these abstractions is influenced by the rate of formation and type of ice, presence of lakes, and the extent of stream-groundwater interaction (Gerard, Citation1989). Sometimes the reduction in flow is sufficiently high that the annual low flow event occurs during freeze-up rather than at the end of the winter baseflow period (Beltaos et al., Citation1993). These concepts, once published, have been sporadically adopted in the method used for winter streamflow estimation as illustrated in the hydrographs for Liard River at Upper Crossing (Citation Figure 6 ). The winter hydrographs for the years 1992 through 1996 (Citation Figure 6 , second from the top) indicate substantial discharge depressions at the start of each winter. None of the hydrographs prior to 1992 shows evidence of storage-related reductions in flow. Only a few of the hydrographs since 1996 indicate any discharge depression and those are of shorter duration and lesser magnitude than during the period from 1992 to 1996.
Figure 6. Illustration of the effect of changes in procedures for subjective estimation of winter streamflow for Liard River at Upper Crossing (10AA001). Grey line indicates open-water record; black line segments show the ice-cover period.
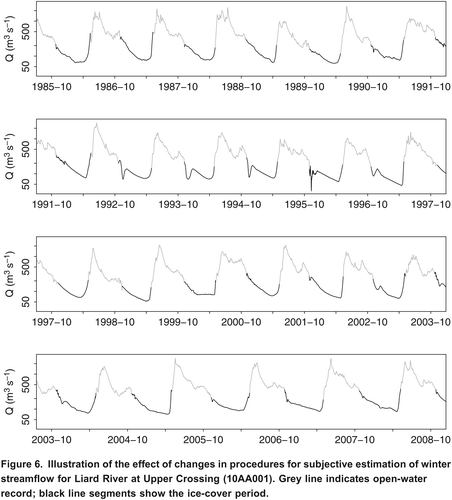
Whether the discharge depressions actually occurred from 1992 to 1996 and whether they did not occur in each of the other years of record could be confirmed only if field measurements of streamflow are available. Events of this type typically occur in the early winter before the ice is generally considered safe to work on so there is insufficient evidence one way or the other to draw conclusions. An evaluation of the uncertainty of these winter records would require consideration of the method used, assumptions made, and of all supporting evidence available.
Change from Manual to Recorded Stage
The gauge on the Lillooet River near Pemberton (WSC station number 08MG005) was established in 1914 and has one of the longest streamflow records in British Columbia. Glaciers cover about 15 to 20% of the catchment area, and the streamflow regime is nivo-glacial, with the most extreme peak flows occurring during autumn-winter rain and rain-on-snow events (Eaton and Moore, Citation2010). The published discharge record was based on manual stage observations from 1914 to 1947 and again from 1956 to 1959, when a continuous gauge was installed. To simulate the effect of this change in stage observation, data for the period from 1997 to 2011 are used to compare streamflow values based on the discharge at 9:00 a.m. each day to those computed from the continuous record of discharge values.
Citation Figure 7 compares flows computed from the manual observation scenario and recorded stage. During the warm season (April to October), there is a tendency for the manual stage measurements to overestimate monthly discharge by a few percent. There are occasional months when the deviation exceeds 5% and even 10%. At a daily resolution, errors occasionally exceed 10% and range up to about 50%.
Figure 7. Simulation of the difference between discharge based on once-per-day manual observations and recorded stage for Lillooet River near Pemberton (08MG005) for the period 1997 to 2011. Qm represents discharge based on stage observed at 09:00 and Qc represents discharge computed from continuously recorded stage. a) Monthly mean streamflow, b) daily mean streamflow. Horizontal lines indicate 5% deviation from a ratio of unity.
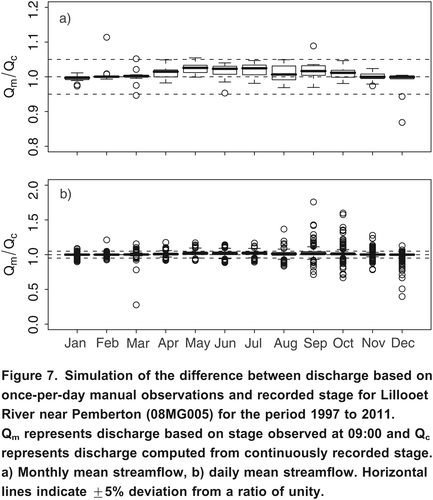
Effects of Technological and Methodological Change on Data Uncertainty
Hydrometric technology is undergoing a period of rapid change. Applications of ADCP and acoustic velocity meters show great promise for addressing some of the short-comings of traditional current meters. Pressure transducers and digital dataloggers have almost completely replaced analog water level recording systems. Real-time data transmission and advanced data management systems are changing not only the way that hydrometric data are produced but also the way in which they are used.
Change in hydrometric methods may be motivated by efficiency gains or by a desire to improve the data. The two examples given of change in methods used to estimate winter streamflow (Takhini and Liard) were inspired by advances in understanding of winter streamflow processes with the intent of using this knowledge to better represent reality in the discharge record. The change in method altered fundamental characteristics of the data. In most instances of methodological change, the resultant change in data characteristics goes unnoticed. This may be because the change in the data is too subtle to notice, but a more likely explanation is that there is little, or no, effort to identify the change and too few metadata about the change to establish a causal relationship.
Acoustic technologies for discharge measurement are capable of producing highly accurate results when used in ideal conditions in the hands of a skilled operator. However, given adverse conditions and a poorly trained operator, the results can be highly unreliable, as is the case for conventional current meter measurements. Most measurement results will fall somewhere in the range from very good to very bad. Paradoxically, it is often at times and locations where the gauging conditions are unfavourable that we place the highest confidence in our measurements. For example, a single measurement in the presence of in-channel vegetation is often used to define a curve shift, or an outlying peak flow measurement or a single extreme low flow measurement are used to define the upper or lower ends of a rating curve, as if the measurements had zero error. It is only when we have a stable, well-defined, rating curve that we accommodate a nominal measurement uncertainty.
A change in measurement variance as a result of change in measurement methodology would not normally be expected to have too much effect for stations with reliable rating curves. However, the example of the Thompson River rating curve illustrates that, even when conditions are nearly ideal, the 5% decision threshold can have an impact on interpretation of the measurements and therefore on interpretation of the rating curve.
Evaluation of the impacts of a change in measurement methodology must consider the transformation of measurement uncertainty into derived discharge uncertainty. The law of propagation of uncertainty applies to straightforward mathematical transformations, such as a rating curve. Propagation of uncertainty gets more complicated when decision thresholds are invoked, especially if those decision thresholds are poorly calibrated to actual measurement uncertainty. If there is a systematic change in measurement uncertainty then there will be a systematic change in the decisions that are made in the context of an unchanging decision threshold.
The example of the Carbon Creek rating curve illustrates the risk that random measurement errors can be transformed into systematic errors that persist in the data record. If the control conditions in the channel are constantly changing, as illustrated in the Ekapo Creek example, then there is currently no way of accumulating an improved understanding of the magnitude of errors in the measurements. These measurement errors are transformed into systematic errors in the derived discharge time-series. Any change in measurement uncertainty as a result of change of methods will therefore propagate directly to the derived discharge time-series and be almost completely untraceable. Currently published metadata are insufficient to distinguish between derived estimates based on a shift to a single measurement from those derived using a stable rating curve.
For shift corrections and high leverage measurements, the random error of the measurements is typically transformed into bias error in the derived discharge time-series. These effects are, for the most part, invisible to the end-user. Changes in measurement methodology have the potential to alter the magnitude and distribution of these errors.
Almost 20% of all Canadian hydrometric data are affected by ice conditions. This includes almost 40% of all annual low flow records and almost 10% of all annual high flow records. Whether the recorded annual low flow occurs in the fall or the spring can be a function of the method used to produce the data, as illustrated by the Liard River example. Interpretation and analysis of the winter streamflow data are therefore complicated by the need for consideration of the method(s) used to produce the data.
Estimated data in Canada account for a little over 5% of all historic records. This has improved to a little over 3% of records since 1990. Most of this improvement in reliability can probably be attributed to the adoption of solid state electronic systems for data logging and water level sensing. The error in data estimates is inherently unknowable. The requirement for estimation of peak flow events is most common in the early years of station operation, as illustrated in the Carbon Creek example. Estimated data do not trigger revision criteria in the WSC dataset. The persistence of these values in the dataset has the potential to introduce a trend in the uncertainty of the peak flow time-series.
One, potentially insidious, impact of technological change may be a change in the role of the field hydrographer. The improved reliability of monitoring equipment means that the hydrographer does not need to be in the field as often to wind the recorder clock, replace the chart, top up the ink in the pen, or flush the intakes. Improved data communication systems mean the field hydrographer does not need to be in the field as frequently to download the data. Reducing the frequency of field visits may be of little consequence for locations producing data with stable, well-defined, rating curves. However, the uncertainty of any data produced by shift curves or by any of the flow estimation methods is highly sensitive to the timing and frequency of field measurements. Increased attention to field safety considerations reduces the likelihood that storage-related flow reductions during freeze-up will be measured while river ice is still consolidating. This may, at least in part, explain why recent discharge records no longer show evidence of these events.
There is constant pressure on monitoring agencies to do more with less. Technological advances that reduce operational costs are readily adopted. The assumption that these changes in technology are benign is largely based on the notion that there is a stable and well-defined rating curve for deriving discharge. For locations and time periods where the rating curve is unreliable, then any change in measurement timing and frequency will influence the uncertainty of the data. In the absence of systematic evaluation of the uncertainty in the derived discharge time-series, it is highly likely that there have been, and will continue to be, methodological artefacts in the data of which we are completely unaware.
Identifying Uncertainty in the Production of Hydrometric Data
The true uncertainty of a water level time-series is with reference to gauge datum. The raw water level error includes errors from reference benchmark instability, errors in transferring datum elevation from bench marks to a reference gauge, errors due to the instability of the reference gauge, errors in reading the reference gauge, errors due to sensor inaccuracy, errors caused by equipment malfunction, errors introduced by data recording and/or communication and human error. Most of these errors are discoverable and correctable within the context of a quality management system. The challenge is how to quantify the uncertainty that remains at the end of the data correction process.
A nave approach is that the corrected water level uncertainty is equal to sensor accuracy specifications, which are a readily identifiable but uncorrectable source of uncertainty. The true uncertainty will almost always be greater, particularly with respect to locations with unstable benchmarks, substantial disturbances (e.g., sediments, debris, ice) or other adverse conditions.
The uncertainty due to benchmark instability is potentially identifiable because a best practice is to maintain at least three independent benchmarks. The uncertainty due to transference of benchmark elevation to the reference gauge is identifiable from the level circuit closure error (i.e., the difference between the sum of foresights and the sum of backsights). The uncertainty due to reference gauge instability is potentially identifiable from the history of reference gauge adjustments. The uncertainty of reference gauge readings is potentially identifiable by replicate sampling. Aggregation of these component uncertainties yields the error of a gauge reading used to correct the time-series of recorded stage. This uncertainty is the minimum possible uncertainty after time-series corrections have been made. The uncertainty within the time-series will increase with time and change in stage from these correction landmarks. Uncertainty will also increase with separation from these correction points as a function of the reliability of sensor calibration and as a function of malfunctions and adverse environmental conditions. The uncertainty due to these factors may be inferred from the data corrections history. Logically, if frequent, high magnitude corrections are required (e.g., as a result of orifice movements or silting of the orifice), then the uncertainty will likely be greater than if infrequent, low magnitude corrections are required.
Rating measurements have two error dimensions: one for discharge, usually expressed as a relative error, and another for mean gauge height, usually expressed as an absolute error. Both dimensions are relevant to evaluating the uncertainty of the rating curve.
The uncertainty of the mean gauge height is the water level uncertainty combined with the uncertainty from the averaging method used. If the change in stage over the duration of the measurement is greater that about 0.03 m, then a weighted calculation of mean gauge height is recommended. The partial-discharge weighting method tends to over-estimate and the time-weighting method tends to under-estimate the mean, so the USGS recommends the use of an average of the time-weighted and discharge-weighted mean (Turnipseed and Sauer, Citation2010).
The application of the standard equation for error propagation which combines the standard uncertainties for sampling efficiency, and width, depth, and velocity observations (Herschy, Citation2009) to calculate discharge measurement uncertainty would lead one to believe that the majority of variance in discharge measurements can be explained by the number of panels in the cross-section. From field experience, it is evident that measurements in narrow streams, with otherwise favourable conditions, can be highly repeatable even if there is room for only a few panels in the cross-section. At the other end of the spectrum, increasing the number of panels will not substantially improve the reproducibility of measurements made under adverse conditions. The Interpolated Variance Estimator (IVE) method attempts to estimate measurement uncertainty from a step-wise evaluation of sampling adequacy. The IVE method does not penalize the favourable but panel-limited measurement, nor does it reward the unfavourable but highly sampled measurement as much as the standard uncertainty method.
The true error of an area-velocity discharge measurement is a function not only of adequate sampling of the variability of depth and velocity but also of the assumptions of local fitness-for-purpose of the measurement equipment in the context of measurement conditions. For example, during high velocity events, zeroing the current meter at the turbulent upper surface can be subject to error very much higher than the limit of error of the sounding reel. Establishing the panel depth requires feeling the streambed sounding, which is compromised by the tug of the high velocity flow on the sounding line, by the requirement to walk the sounding weight upstream to a minimum sounding once it is in the relatively slow flowing boundary layer near the bed, and by the softness of a moving bed. These factors result in additional compounding error on the zero error. There can be a bias error if the sounding weight is inadequate for the combination of depth and velocity. The wetline/dryline bias error can be mitigated by the use of tags on the sounding line or compensated by vertical angle corrections.
Many discharge measurements are compromised by at least some degree of mis-match between the measurement technology and the measurement conditions. The limitations may be too little depth or too much; too little velocity or too much; too little width or too much; too much turbulence; too much of the channel occupied by weeds; too much anchor ice, frazil ice, surface ice or flowing ice; too much debris or sediment transport; or flow that is unsteady or non-uniform. The design of conventional stream gauging equipment is remarkable for its versatility; however, even within a cross-section there can often be combinations of conditions that require difficult choices in the selection of equipment. This is even more critical for emerging technologies for which the consequences of divergence from optimal conditions are currently poorly understood.
Uncertainty estimation using either the standard error propagation equation or the IVE method will be an underestimate of true uncertainty because of the potential for measurement equipment mis-match and because the potential for equipment calibration error is undetectable with either method. These objective estimates of uncertainty are, at least, highly repeatable. Subjective uncertainty estimation by the stream hydrographer reflects the confidence in the equipment used in the context of the measurement conditions. The repeatability of subjective estimates is a function of the training and experience of the hydrographer. Training will vary from agency to agency, and experience varies within agencies, compromising the potential for repeatability of any given subjective estimate.
Deviation from the rating curve is often considered a useful approximation of rating measurement error. A complementary approach would be to consider the deviations of width, depth and velocity from hydraulic geometry relations determined from historic measurements obtained at a common cross-section. The discharge-width, discharge-depth and discharge-velocity relations can expose random uncertainty as well as systematic errors (e.g., blunders such as setting the wrong distance for the height above the meter weight).
Systematic over-prediction of velocity corresponding with under-prediction of depth is evident in the hydraulic geometry relations in Citation Figure 8 . The ovals in plots b) and c) indicate the non-conforming data. The double headed arrows connect measurements with a similar error of the opposite sign. In this case, these deviations cancel out so that there is no evidence in the stage-discharge relation that these measurements are somehow different from the rest. The deviations may indicate a change in measurement method or measurement location or may reflect a threshold in channel control conditions. However, if the deviations are due to systematic sounding error then the corresponding positional error in the vertical could explain the error in velocity with the opposite sign. Compensating errors would not, necessarily, result in obvious deviations in the discharge-width or discharge-stage relation. An error in velocity (e.g., inaccurate meter equation) would not directly cause error in either of the other two dimensions of discharge. The expected result of a non-compensated error would be deviations in both of the other dimensions of the hydraulic geometry.
Figure 8. Hydraulic geometry plots for Thompson River near Spences Bridge (08LF051). The ovals in plots b) and c) indicate a region of systematic deviation between predicted and observed depth and velocity. The double headed arrows connect examples of individual measurements that have may have compensating errors.
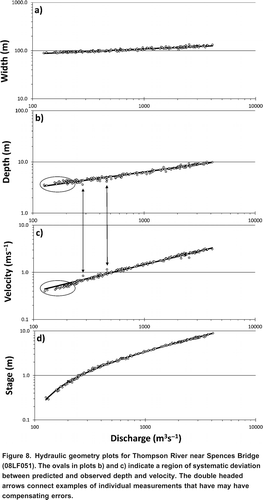
The uncertainty of a rating curve can be evaluated using a standard error of the mean relation approach (Herschy, Citation2009). This will provide a good estimate of rating curve uncertainty for locations like the Thompson River, where there are dozens of measurements spanning the entire range of stage with no breakpoints. This is not the typical case in hydrometric monitoring, where the rating may be defined by as few as one measurement (in the case of a shift correction) or where the shape of the curve must be determined by inference and expert judgement.
Where a curve is fit to a single measurement, the uncertainty of the curve cannot be less than the uncertainty of the measurement used to define the curve. This minimum will be valid for the time of the measurement, but it is expected that the uncertainty increases with separation in units of stage away from the stage of the measurement (because there are insufficient degrees of freedom to constrain the potential shape of the curve) and the uncertainty will increase with separation in units of time from the measurement (because whatever phenomena caused the shift are unlikely to be constant).
The relevance of the measurement for the purpose of defining the curve will decrease with time, particularly for extreme low measurements. Consider a record low-flow measurement that is influential in determining the point of zero flow and hence has high leverage on the shape of the curve. For the purpose of evaluating the uncertainty of the rating curve, the uncertainty could not be less than the uncertainty of the measurement at the time of the measurement. However, as time goes on and there is increasing opportunity for channel change, there should be less confidence that the measured discharge would continue to occur at the gauge height observed at the time of measurement. Hence the mean gauge height at the time of discharge measurement only has a limited but often unknown period of relevance for defining the rating curve. More recent measurements should have a higher weight for evaluation of rating curve uncertainty than measurements for which the relevance of the gauge height is uncertain.
In addition to the case of measurements used to define shift corrections, there are high leverage measurements that define the shape of curve extensions, as in the Carbon Creek example. The uncertainty of the rating curve at the stage of these high leverage measurements cannot be less than the uncertainty of these measurements. Typically, the curve will be drawn right through these measurements so a standard error of the mean relation method would not resolve the true rating curve uncertainty. The uncertainty of the curve will further increase as a function of stage, becoming larger as the curve is extrapolated.
The estimation of uncertainty for a derived discharge time-series must take into consideration transitions from one rating curve to the next. Rating curves have a period of applicability and a simplistic solution would result in an abrupt transition from the uncertainty of one curve to the next curve at the boundary of the period of applicability. This transition is frequently timed to occur at a peak flow event, but the actual hydraulic change probably occurs over a longer duration as sediments are mobilized, transported and deposited.
In most discharge time-series there are periods of time when there are either no valid stage data or no valid rating curve. In some cases discharge estimates can be made with high confidence, well supported by alternative data sources and field visits. In other cases there is little supporting evidence for estimates that may span a substantial period of time. In cases in which the estimate is based on a regression model, the uncertainty can be quantified by the standard error of prediction. However, in cases where estimates are based on ad hoc or highly subjective approaches, the estimate of uncertainty must be similarly subjective.
Recommendations: From Principles to Practice
Fortunately, the development of techniques for quantification of hydrometric uncertainty is advancing on many fronts. The USGS, WMO, International Standards Organization and the North American Stream Hydrographers are committed to advancing the state of the art as quickly as possible. In an ideal world, data that are discoverable and accessible (i.e., using internet based technology) and thus available for advancing our understanding of the distribution of water in time and space will have reliable estimates of uncertainty that are also discoverable and accessible, thus enabling seamless use of data from a variety of sources.
Given the critical role of the rating curve in the production of streamflow data, more effort needs to focus on quantifying the uncertainties associated with extension of the rating curve, shift corrections based on few or even a single measurement, and the possibility for erroneously generating new curves triggered by measurements that exceed some threshold deviation from the existing curve. The use of static decision thresholds should be re-considered in the context of rating measurements that include a variety of technology-specific error variances. Alternative decision rules should be considered to reduce the opportunity for translation of random errors into systematic errors.
The quantification of uncertainty in rating curves should be based on the uncertainties in both the stage and discharge dimensions of the rating measurements. The method for calculation of uncertainty should be robust to the case of shift measurements and to high leverage measurements; this means that the method should incorporate the concept of uncertainty as a function of both time and stage. The estimation of rating curve uncertainty should also be informed by external sources of information such as cross-sections of the control feature, particularly for estimates of the extrapolated portion of the curve. Further research may yield useful nominal rates of change in uncertainty as a function of separation (in time, and in stage magnitude) from calibration measurements, but ultimately these functions may be discoverable from consideration of patterns in rating curve residuals.
One approach to assessing the effect of shift corrections would be to conduct high-frequency measurements at sites such as Ekapo Creek for comparison with streamflow data produced on the basis of the typically less frequent operational schedule. The effect of rating curve evolution and curve extension could be simulated by producing streamflow on the basis of measurements sampled from the total set (e.g., by withholding the highest measurements to simulate the effect of rating curve extension) for sites with high numbers of discharge measurements.
In cases where flows are estimated (including B and E) values, a narrative should be added to the station metadata providing an explanation of approach used, including any assumptions underlying subjective interpretation and the data used to support the estimation procedure. Standards need to be developed for estimation of uncertainty of estimated periods. This is particularly crucial for estimates of ice-affected flow, which can be a large part of the annual record and include both annual extremes.
The effect of shifting from manual observations to recorded stage could be examined by repeating the exercise conducted for the Lillooet River for a broader range of catchments. By referring to weather data and the general sequencing of flows, it may be possible to identify conditions under which streamflow based on manual measurements deviates greatly from estimates based on recorded stage, and thus to assign a quantitative uncertainty estimate to the historic record.
To address the effect of a change in measurement technology, the best approach is to run the earlier and the new technology in parallel for some period of time. While this approach will involve greater cost, we believe that the cost would be justified by the benefits of improvements in our ability to estimate the resulting changes in uncertainty. All changes in methodology used to produce the data should be captured in location metadata to support an improved understanding of causal relations between methods and uncertainty.
A method to estimate the uncertainty between calibrated correction points in the water level time-series is required. The corrections history is a good source of information. If corrections are infrequently needed and of low magnitude, then it may be reasonable to assume that the uncertainty is dominated by sensor accuracy (discoverable from sensor specifications). However, if corrections are frequently needed or are of high magnitude, then it may be assumed that uncertainty in the time-series is dominated by an adverse monitoring environment, resulting in an increase in uncertainty with increase in separation from points where the time-series has been corrected to agree with field observations.
The development of robust methods for quantification of uncertainty is hindered by hydrometric field practices that do not return sufficient information needed as inputs to an uncertainty management framework. It is clear that uncertainty propagation has to begin with robust estimates of the uncertainty of the field observations used to correct the time-series of stage and to derive rating curves.
Discharge measurement uncertainty is difficult to evaluate objectively. Evaluation of residuals from hydraulic geometry relationships (where applicable) may be useful as an input for evaluation of uncertainty. Methods such as the use of the standard error propagation equation and IVE should be further developed as part of the solution, but subjective estimation of uncertainty will likely be required for the foreseeable future.
The primary weakness of subjective estimation is repeatability. This means that training in field measurement uncertainty estimation should be rigorous and, ideally, standardized and perhaps even accredited. This training should include participation in field campaigns of replicate measurements in conditions at the limits of applicability of the technology being used. Experience gained in these field campaigns will translate into improved skill (hence, repeatability) in estimating uncertainty and improved judgement in the selection of methods and technologies, and the results of these campaigns could be fed back to researchers to improve methods for quantification of uncertainty.
The development and implementation of methods for measurement and publication of hydrometric uncertainty will involve some cost. It currently appears unlikely that new money will be made available to hydrometric agencies to fund this activity. However, it needs to be recognized that the credibility and defensibility of hydrometric data are extremely valuable attributes, and the standard is inevitably evolving to include estimation of uncertainty. Data providers who choose not to adapt may find that their data will not be trusted at the same level as are those from data providers who quantify and publish uncertainty estimates.
In the past, there was only one published version of the data a version that had undergone many checks and balances. Now, many versions of the data may be publicly available, as the data are published in near-real-time and then progressively improved on. Measurement of uncertainty provides a way of binding these versions with information needed for interpretation of the data. Analysts will be better able to appreciate the value added by quality management systems, as uncertainty is reduced with each successive version of the data, and in comparison between data providers who incorporate best practices to systematically resolve data uncertainties and those who don't. The cost of development and implementation of methods for uncertainty measurement should be evaluated in the context of the benefits achieved through improved availability of data.
Acknowledgements
We thank Dave Hutchinson and other staff at the Water Survey of Canada for consultation, help, advice and access to the data for the case study investigations, and Mike Church and two anonymous reviewers for thoughtful, detailed, and constructive comments that led to significant and substantive improvements in this manuscript.
References
- American National Standards Institute /American National Conference of Standards Laboratories ANSI / NCSL. 1997. American national standard for calibration: U.S. guide to the expression of uncertainty in measurement. ANSI/NCSL Z540-2-1997. American National Standard Institute, American National Conference of Standards Laboratories, 101 pp.
- Beltaos, S., D. J. Calkins, L. W. Gatto, T. D. Prowse, S. Reedyk, G. J. Scrimgeour, S. P. Wilkins. 1993. Physical effects of river ice. In Environmental aspects of river ice, ed. T. D. Prowse and N. C. Gridley. National Hydrology Research Institute (NHRI) Science Report No. 5. Saskatoon, Saskatchewan: NHRI, pp. 372.
- Beven , K. and Westerberg , I. 2011 . On red herrings and real herrings: disinformation and information in hydrological inference . Hydrological Processes , 25 : 1676 – 1680 .
- Chin, W. Q. 1966. Hydrology of the Takhini River Basin, Y.T. with special reference to accuracy of winter streamflow records and factors affecting winter streamflow. Water Resources Branch, Dept. of Energy Mines and Petroleum Resources, Internal Report No. 2, 65 pp.
- Clarke , R. T. 1999 . Uncertainty in the estimation of mean annual flood due to rating-curve indefinition . Journal of Hydrology , 222 : 185 – 190 .
- Eaton, B. C., and R. D. Moore. 2010. Regional hydrology. Chap. 4 in Compendium of forest hydrology and geomorphology in British Columbia, ed. R. G. Pike, T. E. Redding, R. D. Moore, R. D. Winkler, and K. D. Bladon. Land Management Handbook 66. BC Ministry of Forests and Range, Forest Science Program, Victoria, BC and FORREX Forum for Research and Extension in Natural Resources, Kamloops, BC, pp. 85110.
- Gerard, R. 1989. Ice jam floods. Chap. 10 in Hydrology of floods in Canada, ed. W. E. Watt. Ottawa, Ontario: National Research Council of Canada, pp. 169184.
- Gray, D. M., and T. D. Prowse. 1993. Snow and floating ice. In Handbook of hydrology, ed. D. R. Maidment. Toronto: McGraw Hill, pp. 7.17.58.
- Herschy, R. W. 2009. Streamflow measurement. 3rd edition. Boca Raton, FL: CRC Press, 510 pp.
- Jonsson, P., A. Petersen-Overleir, E. Nilsson, M. Edstrom, H. L. Iversen, and H. Sirvio. 2002. Methodological and personal uncertainties in the establishment of rating curves. In Proceedings of XXII Nordic Hydrological Conference, Roros Norway, August 47, 2002, ed. A. Killingtveit. Nordic Hydrological Programme, NHP Report No. 47. Norwegian Water Resources and Energy Directorate, pp. 3544.
- Kiang, J. E., T. A. Cohn, and R. R. Mason. 2009. Quantifying uncertainty in discharge measurements: a new approach. In Proceedings of World Environmental and Water Resources Congress 2009, ed. S. Starrett, May 1721, 2009, Kansas City, Missouri: American Society of Civil Engineers. doi:10.1061/41036(342)599.
- Kiang, J. E., T. A. Cohn, R. R. Mason, J. Fulford, and S. Hamilton. 2011. A comparison of methods for estimating uncertainty in discharge measurements (abstract). In Proceedings of Canadian Water Resources Association 64th Annual Conference, St. John's, Newfoundland, June 2730, 2011. St. John's, NFLD: Canadian Water Resources Association.
- Melcher, N. B., and J. F. Walker. 1992. Evaluation of selected methods for determining streamflow during periods of ice effect. United States Geological Survey (USGS) Water Supply Paper 2378. Washington, D.C.: USGS, 47 pp.
- Moore , R. D. , Hamilton , A. S. and Scibek , J. 2002 . Winter streamflow variability, Yukon Territory, Canada . Hydrological Processes , 16 : 763 – 778 .
- Oberg , K. and Mueller , D. S. 2007 . Validation of streamflow measurements made with acoustic doppler current profilers . Journal of Hydraulic Engineering , 133 ( 12 ) : 1421 – 1432 .
- Pelletier , P. M. 1988 . Uncertainties in the single determination of river discharge: a literature review . Canadian Journal of Civil Engineering , 15 ( 5 ) : 834 – 850 .
- Sauer, V. B., and D. P. Turnipseed, 2010. Stage measurement at gaging stations. U.S. Geological Survey Techniques and Methods Book 3, Chap. A7, 45 pp. (available at http://pubs.usgs.gov/tm/tm3-a7/).
- Schmidt, A. R. 2004. Uncertainties in discharges from stations where rating shifts are utilized. In Proceedings of American Society of Civil Engineers (ASCE) World Water Congress, ed. G. Sehlke, D.F. Hayes and D.K. Stevens, June 27 July 1, 2004, Salt Lake City, Utah, 9 pp.
- Turnipseed, D. P., and V. B. Sauer. 2010. Discharge measurements at gauging stations. United States Geological Survey (USGS) Techniques and Methods 3-A8. Washington, D.C.: USGS, 87 pp.