Figures & data
Table 1. Primers used for the qRT-PCR
Table 2. Characteristics of datasets included in the integrated analysis
Figure 1. Differentially expressed genes and common differentially expressed genes in two datasets. (a and b) The volcano plots visualize the differentially expressed genes in GSE12452 and GSE53819. (c and d) Common differentially expressed genes in two datasets
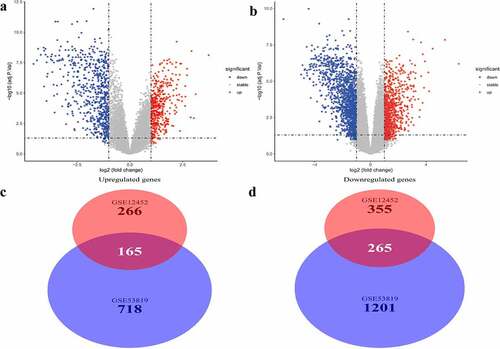
Figure 2. Determination of soft-thresholding power in the weighted gene co-expression network analysis (WGCNA). (a) Analysis of the scale-free fit index for various soft-thresholding powers (β). The red line indicates where the correlation coefficient is 0.9, and the corresponding soft-thresholding power is 5. (b) Analysis of the mean connectivity for various soft-thresholding powers. The red line indicates where the correlation coefficient is 0.9, and the corresponding soft-thresholding power is 5. (c) Histogram of connectivity distribution when β = 5. (d) Checking the scale-free topology when β = 5
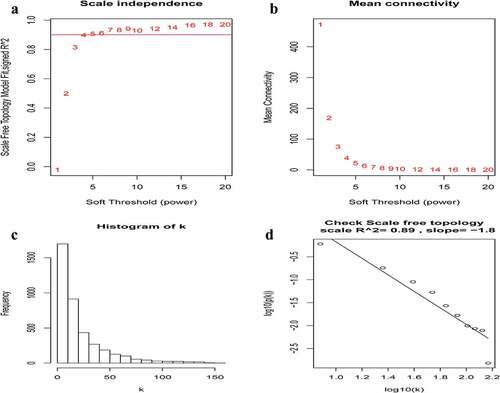
Figure 3. Construction of WGCNA modules. (a) The cluster dendrogram of module eigengenes. (b) The cluster dendrogram of the genes with median absolute deviation in the top 25% in the GSE12452. Each branch in the figure represents one gene, and every color below represents one co-expression module
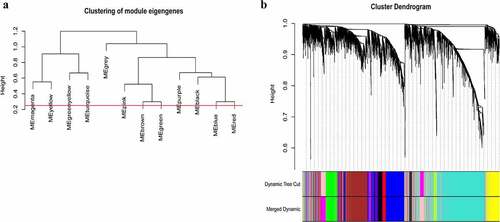
Figure 4. Relationship between modules and clinical traits. (a) Module eigengene dendrogram and eigengene network heatmap summarize the modules yielded in the clustering analysis. (b) Heatmap of the module-trait relationships. The green module was significantly associated with N stage
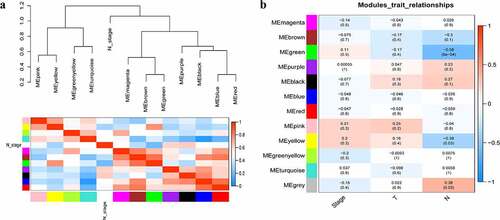
Figure 5. Functional enrichment analysis of hub module genes. (a) GO terms and KEGG pathway were presented, and each band represents one enriched term or pathway colored according to the -log10(p). (b) Network of the enriched terms and pathways. Nodes represent enriched terms or pathway with node size indicating the number of hub module genes involved in. Nodes sharing the same cluster are typically close to each other, and the thicker the edge displayed
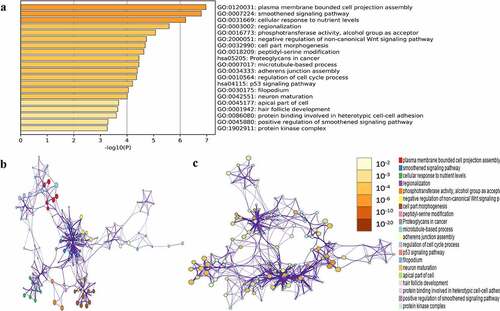
Figure 6. Identification of hub module and candidate hub gene. (a) Distribution of average gene significance and errors in the modules associated with the NPC. (b) Scatter plot for correlation between gene module membership in the green module and gene significance
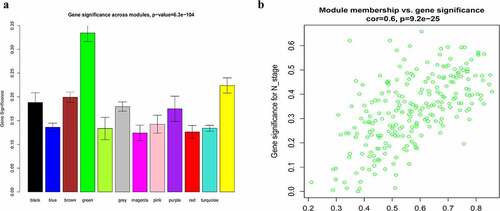
Figure 7. Validation of hub genes in the gene expression level. (a-d) Validation of hub genes in the GSE12452. PGAP1, MARK1, and KITLG were significantly higher expression in NPC compared with normal tissues, while CRIP1 was significantly lower expression in NPC compared with normal tissues. (e-h) Validation of hub genes in the GSE53819 and the results were the same as earlier
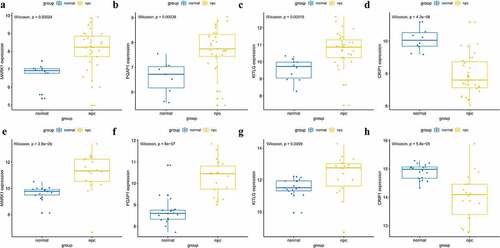
Figure 8. Validation of hub genes in the diagnostic value. (a-d) Validation of hub genes in the GSE12452. Receiver operating characteristic (ROC) curves and area under the curve (AUC) statistics are used to evaluate the capacity to discriminate NPC from normal controls with excellent sensitivity and specificity. (e-h) Validation of hub genes in the GSE53819 and the results were the similar as earlier. These findings indicated these four hub genes have excellent diagnostic efficiency in NPC
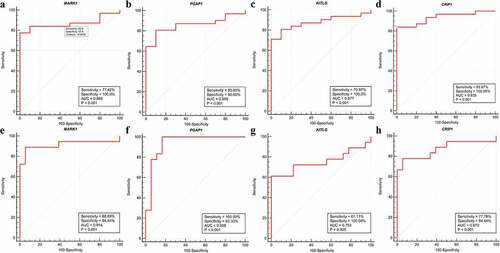
Figure 9. Validation the expression levels of four hub genes in the combined microarray datasets. (a-d) PGAP1, MARK1, and KITLG were significantly higher expression in NPC compared with normal tissues, while CRIP1 was significantly lower expression in NPC compared with normal tissues
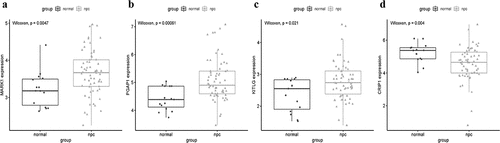
Table 3. Top 10 most significant small molecule drugs based on CMAP
Figure 10. Relative expression of MARK1, PGAP1, KITLG and CRIP1 mRNA in clinical nasopharyngeal carcinoma tissues and cell lines. (a-d) The relative expression of MARK1, PGAP1, KITLG and CRIP1 mRNA in 20 paired nasopharyngeal carcinoma tissues and adjacent tissues were detected by qRT-PCR. (e-h) The relative expression of MARK1, PGAP1, KITLG and CRIP1 mRNA in 5 cell lines (NP69, CNE1, HNE3, 5–8 F and HK-1) were detected by q-RT-PCR. *P < 0.05, **P < 0.01, ***P < 0.001
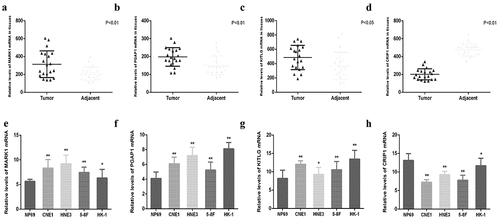