Abstract
Background: Virtual patients (VPs) are narrative-based educational activities to train clinical reasoning in a safe environment. Our aim was to explore the influence of the design of the narrative and level of difficulty on the clinical reasoning process, diagnostic accuracy and time-on-task.
Methods: In a randomized controlled trial, we analyzed the clinical reasoning process of 46 medical students with six VPs in three different variations: (1) patients showing a friendly behavior, (2) patients showing a disruptive behavior and (3) a version without a patient story.
Results: For easy VPs, we did not see a significant difference in diagnostic accuracy. For difficult VPs, the diagnostic accuracy was significantly higher for participants who worked on the friendly VPs compared to the other two groups. Independent from VP difficulty, participants identified significantly more problems and tests for disruptive than for friendly VPs; time on task was comparable for these two groups. The extrinsic motivation of participants working on the VPs without a patient story was significantly lower than for the students working on the friendly VPs.
Conclusions: Our results indicate that the measured VP difficulty has a higher influence on the clinical reasoning process and diagnostic accuracy than the variations in the narratives.
Introduction
Virtual patients (VPs) provide a safe environment in which students can practice clinical reasoning skills in their own learning pace without harming patients.
Research has identified design aspects of VPs, that support clinical reasoning skills training. For example, Huwendiek et al. (Citation2009) elaborated in a focus group study that aspects such as feedback on learners’ decision, appropriate media use, or authenticity of learner tasks are relevant VP design principles to foster clinical reasoning training.
VPs are based on narratives, telling a patient's story with textual information, dialogs and media elements in a scenario in which the learner typically takes the role of the responsible healthcare professional (Ellaway and Topps Citation2009). Narratives to engage learners are not only applied in virtual patients, but in almost every part of healthcare education, such as case-based learning or problem-based learning scenarios (Ellaway and Topps Citation2009).
Another important aspect that has to be considered when creating and providing VPs to learners is an appropriate level of difficulty for the target group (Posel et al. Citation2009).
In VPs, the influence of the narrative and the level of difficulty on the clinical reasoning process has not yet been investigated in detail. For example, it is not clear how varying contextual information, such as the presentation of the patient in a VP scenario, influences the clinical reasoning process.
Outside the world of VPs, it has been shown that contextual factors in learning cases influence the clinical reasoning process of healthcare professionals. For example, McBee et al. (Citation2015) showed that residents experienced difficulties with closure of a video-taped patient encounter when patients showed an emotional volatile behavior.
Schmidt et al. (Citation2017) implemented a study comparing paper cases depicting patients with disruptive versus neutral behavior. They discovered that the description of a disruptive behavior led to lower diagnostic accuracy while the time spent with the cases remained the same. A recent study compared a written case presentation, which had to be solved in individual study, with a video presentation of a patient, which had to be solved in a group discussion. The two groups did not differ in diagnostic accuracy, but the video group was more efficient (Linsen et al. Citation2017).
These studies show that the design of case narratives influences the clinical reasoning process. However, the interaction of learners with paper-based cases is different from VP scenarios, which are nowadays an important element of medical curricula.
Therefore, our aims were to investigate the influence of the narrative, that is, the representation of a patient in VP scenarios, and the measured level of difficulty on (1) diagnostic accuracy, (2) time on task and (3) the clinical reasoning process of medical students.
Methods
Participants
All final-year medical students at Ludwig–Maximilians– Universität München were invited via the faculty's learning management system and with an email to take part in this study. Participants received a monetary compensation of 50 Euros after completing six virtual patients and filling out two questionnaires. We obtained ethical approval for this study from the Ethical Committee of the University of Munich (No 17-122).
Design of virtual patients
We created six virtual patients in the VP system CASUS (CASUS Citation2017) covering a variety of topics (). The VPs consisted of 7–8 screen cards with an introduction of the patient, history taking, physical exam, performed laboratory or technical examinations, elaboration of the final diagnosis and treatment.
Table 1. Overview of the six virtual patients created for the study.
We designed each VP in three different versions concerning the patient representation in the narrative:
Group FRIENDLY – A patient showing a neutral/friendly behavior
Group DISRUPTIVE – A patient showing a disruptive behavior ()
Group NOSTORY – No patient story is told.
In each version of the VP, we provided the same information. In the groups FRIENDLY and DISRUPTIVE, the communication between the physician and the patient was presented in a dialog format with a comparable amount of text.
In the group NOSTORY the patient’s problems were described in bullet points, without using any dialog format, which led to a shorter text than for group 1 and 2.
The following example taken from the VP Benedikt Vogt illustrates the differences between the three versions:
DISRUPTIVE:
Physician: “Do you take any medication?”
Mr. Vogt: “I take tablets for my high cholesterol, although I am not convinced that tablets really help. Sometimes I forget to take them and I don't feel worse. I only take them because my wife gets angry with me if I don't.”
FRIENDLY:
Physician: “Do you take any medication?”
“I take tablets for my high cholesterol. I take them regularly as you told me to and I've also been trying to follow your nutritional advice.”
Medication: Cholesterol lowering tablets.
On the last card of each VP participants were asked on a Likert scale from 1 to 5 (1 = not at all, 5 = very much) to rate how likeable they found the patient. The VPs are available under an Open Source license and can be obtained from the authors.
Concept mapping tool
To document the clinical reasoning process, we combined the VPs with a concept mapping tool (Hege et al. Citation2017a, Citation2017b). Participants were prompted to select problems/findings, differential diagnoses, relevant tests and treatment options from a type-ahead list based on the Medical Subject Heading list (MeSH Citation2017), to add them as nodes to the concept map, and to draw connections between these nodes if appropriate (). Towards the end of the VP scenario, participants had to submit a final diagnosis in order to conclude the scenario, but submission was also possible at an earlier stage. Participants were allowed to re-submit a final diagnosis as long as it did not match with the expert's diagnosis; after one unsuccessful try they could also choose to be provided with the correct diagnosis. The system was able to consider synonyms and similar entries in all categories. When submitting a final diagnosis participants were asked to indicate their confidence with the decision on a slider from 0% to 100%.
Figure 1. Screenshot of the virtual patient Miray Günal with the concept mapping tool on the right side.
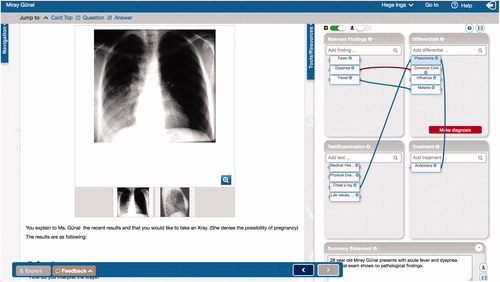
On the last card of the VP scenario participants could access an expert's concept map and compare it with their solution. The tool can be accessed at http://crt.casus.net (guest access).
The VPs and the expert concept maps were reviewed by experienced clinical educators (IK, JS) and were piloted with seven medical students, who did not participate in the main study.
In addition to this basic estimation of difficulty, we developed a binary coding for the evaluation of the VP difficulty. If participants identified the correct final diagnosis in the first try, it was coded as 1 (solved); if no final diagnosis was made or the participant needed more than one attempt to correctly solve the VP, it was coded as 0 (not solved). We then calculated the mean for each VP over all three groups (). Thus, we identified four easy VPs (Michael Bauer, Miray Günal, Tim Wagemann, Frank Reiter), and two difficult VPs (Benedikt Vogt, Nina Sanders).
Questionnaires
Participants had to complete two online questionnaires, which were created and distributed with SurveyMonkey (Citation2017). Questionnaire 1 contained seven questions about demographic data, such as age, sex, exam grades, and clinical elective.
Questionnaire 2 (Supplementary Appendix 1) contained 28 questions from a usability questionnaire (System Usability Scale Citation1986), a motivational questionnaire (Prenzel et al. Citation1993), and a questionnaire designed to evaluate VPs (Huwendiek et al. Citation2015).
Study design
After agreeing to participate, students received a unique 8-digit pin, which was randomly assigned to one of the three study groups and were asked to fill out questionnaire 1 before accessing the VP course (). Participants were given two weeks’ time to complete the tasks and could work from home. If necessary, a short reminder was sent two days before the deadline.
As an introduction, participants were provided with a worked example and a short video (YouTube Citation2017) about how to use the concept mapping tool. Then, the VPs were accessible one after the other. After having completed the six VPs, participants received the link to questionnaire 2.
Data analysis
All interactions of the participants with the virtual patient system and the concept mapping tool were recorded with exact timestamps in a relational database, anonymized and exported into SPSS (Version 24, IBM Inc.) for further analysis.
After calculating the level of difficulty of the VPs (), we compared for both levels of difficulty the three experimental groups of participants (FRIENDLY, DISRUPTIVE, and NOSTORY) with the dependent variables:
mean number of tries until correct final diagnosis (= diagnostic accuracy),
mean number of nodes (problems, differential diagnoses, tests, and treatment options) and connections between these nodes,
mean time on task (measured from opening a VP until closing it),
mean number of requests for the correct final diagnosis to be revealed by the system, and,
mean level of confidence with the final diagnosis decision.
All anonymized data with a description can be provided on request.
We used a MANOVA to test all dependent variables at once. Alpha error was set to p < 0.05. If the MANOVA revealed significant differences between the groups we used Least significant difference (LSD) test for post hoc comparison.
Funding
The project receives funding from the European Union’s Horizon 2020 research and innovation program under the Marie Skłodowska-Curie grant agreement No 654857. The study is part of the doctoral thesis of AD.
Results
In the following, we will describe the results for all dependent variables for both levels of VP difficulty.
Participants
A total of 46 medical students completed the study. We could not detect any significant differences concerning the participants’ characteristics in the three groups; they were comparable in aspects such as age, sex and examination grades ().
Table 2. Participant characteristics and exemplary answers to questionnaire 2 for the three groups.
For the items in questionnaire 2, we found a significantly higher self-estimated performance satisfaction for group NOSTORY than for group FRIENDLY and a significantly higher rating of the tool complexity in group FRIENDLY, compared to group DISRUPTIVE. Additionally, the extrinsic motivation was significantly higher for the group FRIENDLY compared to group NOSTORY. Answers to all other questions concerning intrinsic motivation, estimation of competency and self-determination were comparable.
The means of the most relevant questions are included in . All questions and clustered means can be found in Supplementary Appendix 2.
Diagnostic accuracy and level of confidence
For the four easy VPs (), participants of all groups took about the same mean number of tries until they found the correct final diagnosis. For the two difficult VPs, the participants in the DISRUPTIVE and NOSTORY group needed significantly more tries to find out the correct final diagnosis than the FRIENDLY group (). A MANOVA showed that this difference is significant F(2;256) = 3.396; p = 0.20; eta2 = .030) with post hoc tests confirming both differences to be significant.
Figure 3. Mean number of tries to submit the correct final diagnosis for the two levels of difficulty and the three groups. * significant difference to group FRIENDLY (p < .05).
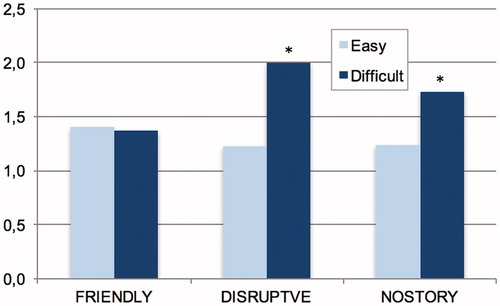
For the four easy VPs, the FRIENDLY (MFRIENDLY = .14; SD = .353) and the NOSTORY group (MNONARR = .17; SD = .383) significantly more often gave up and requested the system to reveal the correct final diagnosis than the DISRUPTIVE group (M = .00; SD = .00), F(2.258) = 3.909; p < 0.021; eta2 = .029). Post hoc test confirmed that both differences are significant.
For the easy VPs, the FRIENDLY group was significantly less confident with their final diagnosis decision (M = 60.12%; SD = 27.48) than both, the DISRUPTIVE (M = 72.85%; SD = 25.76) and the NOSTORY group (M = 74.11%; SD = 22.34). For the difficult VPs, the confidence of participants in the FRIENDLY group was higher (M = 68.07%; SD = 24.59) and comparable to the DISRUPTIVE (M = 71.43%; SD = 25.71) and NOSTORY group (M = 71.88%; SD = 28.04), so that there was no significant difference of confidence in difficult VPs.
Number of nodes and connections
When looking into the details of the concept maps, we found significant differences in the number of added nodes (problems, differential diagnoses, tests, treatment options) between the three groups and level of difficulty (). For easy VPs, group DISRUPTIVE added significantly more problems, differential diagnoses, tests and treatment options than group FRIENDLY; Group NOSTORY added less differential diagnoses and treatment options than the group DISRUPTIVE, but more tests than group FRIENDLY. There was no significant difference between the three groups for the number of connections added to the concept maps.
Table 3. Mean number of the different nodes and connections for the three groups and VP difficulty.
Time on task
The time on task was significantly lower for the NOSTORY group (M = 1038.10 sec, SD = 458.915) than for the DISRUPTIVE group (M = 1375.75; SD = 715.49), but not significantly lower than the FRIENDLY group (M = 1211.43 sec; SD = 808.09), (F(1;261) = 5,733, eta2 = .042) with a medium effect size.
Likeability of patients
On a Likert scale from 1 (not at all) to 5 (very much), participants in group FRIENDLY rated the likeability of the patient significantly (p < 0.001) higher (M = 4.32; SD = 0.81) compared to participants in the group NOSTORY (M = 3.54; SD = 0.96), and DISRUPTIVE (M = 2.40; SD = 1.14).
Discussion
Our study showed that diagnostic accuracy depends on both, the patient representation in the VP narrative and the level of difficulty of the VP. Additionally, we found significant differences in the structure of the concept maps, which were more distinct in easy VPs. In the following we will discuss the results in detail.
Level of difficulty
The level of difficulty is an important aspect of virtual patients influencing aspects such as learners’ engagement (Mallott et al. Citation2005). Reviewers, pilot testers and participants () found the level of difficulty and covered topics of all six VPs appropriate for final year medical students. However, we found a significant difference in the actual level of difficulty based on the number of attempts needed to provide a correct final diagnosis.
VP difficulty for education or research is often assessed as the perceived level of difficulty of students, case authors, or educators (Botezatu et al. Citation2010; Georg and Zary Citation2014). However, based on our findings, we see a discrepancy between estimated and measured level of VP difficulty; therefore, we suggest considering the actual and individual VP difficulty as a more accurate measurement before providing VPs to learners.
Diagnostic accuracy and confidence
In difficult VPs, diagnostic accuracy was significantly lower in the DISRUPTIVE and NOSTORY groups compared to the FRIENDLY group (), but we did not see any significant differences in diagnostic accuracy for the four easy VPs. Independent of the case difficulty, Schmidt et al. (Citation2017) found in residents a lower diagnostic accuracy for disruptive paper-based cases than for neutral cases. The results of our web-based study indicate that for final year medical students the case difficulty has a higher influence on diagnostic accuracy than the design of the narrative. For easy VPs, the variation in the narrative does not influence diagnostic accuracy, whereas in difficult VPs, diagnostic accuracy is significantly lower when confronted with disruptive patients. An explanation could be that in easy VPs medical students can compensate the higher context complexity of being confronted with a disruptive patient, whereas for difficult VPs they are overwhelmed. Interestingly, confidence of participants in the FRIENDLY group was higher for difficult than for easy VPs, which could indicate a potential overconfidence bias in difficult VPs.
Real-world challenging encounters are much more complex and emotionally distressing for both, patients and healthcare professionals, than our virtual encounters, in which the learner could not actively communicate with the patient. Apart from patient-related factors (e.g. showing a certain behavior), physician-related factors (e.g. insecurity) and situational factors influence the situation (Lorenzetti et al. Citation2013). But, even our simplified approach varying solely patient-related factors with relatively small differences between the friendly and disruptive behavior descriptions led to medium size effects. Follow-up studies could simulate also physician-related and situational factors and for example explore effects of a disruptive behavior of the healthcare professional.
Number of nodes and connections
Independent from the VP difficulty participants added significantly more problems and tests for disruptive than for friendly VPs. Steinmetz and Tabenkin (Citation2001) described 12 coping strategies of family practitioners when confronted with difficult patients; ordering tests and referring patients is one of the strategies. Interestingly, our findings indicate that this coping strategy seems to be also present in medical students. With our study design it is not possible to find out whether the participants also applied other coping strategies identified by Steinmetz et al., such as empathy, non-judgmental listening, patience or direct approach.
We can only speculate why participants added significantly more problems for disruptive patients. It could be that they were anxious to miss something and therefore regarded more problems described by the patient as relevant and added them to their map.
Further research, for example a combination of the VPs with the long interview approach applied by Steinmetz et al., is needed to find out more about the application of coping strategies and the impact on the clinical reasoning process.
Likeability of patients and learner motivation
As expected, participants rated the likeability of the virtual patients higher in the friendly compared to the disruptive version. This result is comparable with the study by Schmidt et al. (Citation2017) who found that average likability ratings were higher for neutral than for difficult paper-based patient cases. Likeability of the VPs without a story was neutral. This indicates that our modifications of the narratives were successful and as intended.
Surprisingly, learners in all groups equally appreciated the virtual patients as a worthwhile learning experience (), even if there was no patient story told and only facts were presented. While intrinsic motivation was comparable for all three groups, extrinsic motivation was significantly lower for the NONSTORY group compared to the FRIENDLY group. This finding supports current research on narratives as gamification elements (Rowe et al. Citation2007; Lister et al. Citation2014) and indicates that telling a story does not influence diagnostic accuracy or the clinical reasoning process, but can increase student’s extrinsic motivation.
Time on task
As expected, time on task was lower for the group, who worked on the VPs without a patient story (group NOSTORY). These VPs contained significantly less text and were focused on facts, thus, participants needed less time for reading. Time on task was not significantly different between the group DISRUPTIVE and FRIENDLY, which replicates a finding of Schmidt et al. (Citation2017).
Limitations
We are aware that our study has some limitations. First, since participants received a monetary compensation, there is a potential motivational bias. However, we can assume that this bias is comparable for the three groups. Second, our study involved only final-year medical students at one institution. Therefore, it is unclear to which extent our findings apply to less experienced students or students from other medical schools. Third, similar to the study by Schmidt et al. (Citation2017) we cannot exclude that the difficult behavior may have been interpreted as symptoms associated with other diagnoses. Although we paid special attention to this aspect when creating and testing the VPs, a further in-depth qualitative analysis of the concept maps is needed to explore this effect.
Conclusions
Our study shows that telling a story is important to enhance extrinsic motivation of learners.
When creating cases or VPs for healthcare education or research studies, the actual level of difficulty needs to be carefully considered since it influences the clinical reasoning process of medical students more than the design of the VP narrative. Moreover, the patient presentation (friendly vs disruptive) influences the clinical reasoning process and needs to be carefully designed depending on the case difficulty, intended learning objectives and level of learners’ expertise. Patients showing a disruptive behavior will most likely be part of students' future workplace and carefully designed and integrated VPs can help to prepare students for such situations and raise awareness of the potential effects on their clinical reasoning.
Glossary
Concept mapping: Is a tool for knowledge organization and representation and is an approach applied in healthcare education. Concept maps are graphical representations with which learners visualize their understanding of a concept. Elements of a concept map are so-called nodes (i.e. the concepts) and connections (i.e. relations) between these nodes.
Torre DM, Durning SJ, Daley BJ. Twelve tips for teaching with concept maps in medical education. Med Teach. 2013;35(3):201-8. PMID: 23464896
Supplementary_appendix.pdf
Download PDF (57.2 KB)Acknowledgments
We would like to thank all students who participated in the study or helped to pilot test the VPs. We also would like to thank all physicians and students for reviewing the VPs giving important suggestions for improvement. We would also like to thank Prof. Silvia Mamede and Prof. Henk Schmidt, who inspired us to implement this study and allowed us to access to the cases they used in their research.
Disclosure statement
The authors report no conflicts of interest. The authors alone are responsible for the content and writing of this article.
Additional information
Notes on contributors
Inga Hege
Inga Hege, MD, MCompSc, is Associate Professor for Medical Education. In an EC funded Marie-Curie Fellowship she researched how clinical reasoning training can be improved in virtual patients.
Anita Dietl
Anita Dietl, is a final year medical student at the Medical School of LMU Munich. This study is part of her MD thesis.
Jan Kiesewetter
Jan Kiesewetter, PhD, is psychologist and Associate Professor for Medical Education at the Institute for Medical Education at LMU Munich, Germany. His main research focus is fostering clinical competence in medical students.
Jörg Schelling
Prof. Jörg Schelling, MD, is a general practitioner with a long experience in medical education and especially case-based learning and virtual patients.
Isabel Kiesewetter
Isabel Kiesewetter, MD, MSc, is a clinical specialist in anaesthesiology. In medical education she is highly engaged in patient safety aspects and how the training for medical students can be improved.
References
- CASUS. 2017. Virtual Patient System. 1999-2017. [Accessed 2017 November 6]. http://crt.casus.net
- Botezatu M, Hult H, Tessma MK, Fors UGH. 2010. Virtual patient simulation for learning and assessment: Superior results in comparison with regular course exams. Med Teach. 32:845–850.
- Ellaway RH, Topps D. 2009. Rethinking fidelity, cognition and strategy: medical simulation as gaming narratives. Stud Health Technol Inform. 142:82–87.
- Hege I, Kononowicz AA, Adler M. 2017a. A Clinical Reasoning Tool for Virtual Patients: Design-Based Research Study. JMIR Med Educ. 3:e21.
- Hege I, Kononowicz AA, Berman NB, Lenzer B, Kiesewetter J. 2017b. Advancing clinical reasoning in virtual patients - development and application of a conceptual framework. Forthcoming 2018 Feb. GMS J Med Educ.
- Georg C, Zary N. 2014. Web-based virtual patients in nursing education: development and validation of theory-anchored design and activity models. JMIR. 16:10e105.
- Huwendiek S, Reichert F, Bosse HM, de Leng BA, van der Vleuten CPM, Haag M, Hofmann GF, Tönshoff B. 2009. Design principles for virtual patients: a focus group study among students. Med Educ. 43:580–588.
- Huwendiek S, De Leng BA, Kononowicz AA, Kunzmann R, Muijtjens AMM, Van Der Vleuten CPM, Hoffmann GF, Tönshoff B, Dolmans D. 2015. Exploring the validity and reliability of a questionnaire for evaluating virtual patient design with a special emphasis on fostering clinical reasoning. Med Teach. 37:775–782.
- Linsen A, Elshout G, Pols D, Zwaan L, Mamede S. 2017. Education in Clinical Reasoning: An Experimental Study on Strategies to Foster Novice Medical Students’ Engagement in Learning Activities. In Health Professions Education. ISSN 2452-3011. [Accessed 2017 November 6]. http://www.sciencedirect.com/science/article/pii/S2452301117300299?via%3Dihub
- Lister C, West JH, Cannon B, Sax T, Brodegard D. 2014. Just a fad? Gamification in Health and Fitness Apps. JMIR Serious Games. 2:e9.
- Lorenzetti RC, Jacques CM, Donovan C, Cottrell S, Buck J. 2013. Managing difficult encounters: understanding physician, patient, and situational factors. Am Family Phys. 87:419–425.
- Mallott D, Raczek J, Skinner C, Jarrell K, Shimko M, Jarrell B. 2005. A basis for electronic cognitive simulation: the heuristic patient. Surg Innov. 12:43–49.
- McBee E, Ratcliffe T, Picho K, Artino AR, Schuwirth L, Kelly W, Masel J, van der Vleuten C, Durning S. 2015. Consequences of contextual factors on clinical reasoning in resident physicians. Adv in Health Sci Educ. 20:1225–1236.
- Medical Subject Headings (MeSH). 2017. [Accessed 2017 November 6] https://www.nlm.nih.gov/mesh/
- Posel N, Fleiszer D, Shore BM. 2009. 12 Tips: Guidelines for authoring virtual patient cases. Med Teach. 31:701–708.
- Prenzel M, Eitel F, Holzbach R, Schoenhein RJ, Schweiberer L. 1993. Lernmotivation im studentischen Unterricht in der Chirurgie. [Learner Motivation in Teaching Students Surgery]. Zeitschrift Für Pädagogische Psychologie. 7:125–137.
- Rowe JP, Mcquiggan SW, Mott BW, Lester JC. 2007. Motivation in narrative-centered learning environments. In: Proceedings of the workshop on narrative learning environments, AIED:40–9.
- Schmidt HG, van Gog T,CE, Schuit S, Van den Berge K,LA, Van Daele P, Bueving H, Van der Zee W, Van Saase J, Mamede S. 2017. Do patients’ disruptive behaviours influence the accuracy of a doctor’s diagnosis? A randomised experiment. BMJ Qual Saf. 26:19–23.
- Steinmetz D, Tabenkin H. 2001. The “‘difficult patient’ as perceived by family physicians”. Fam Pract. 18:495–500.
- Surveymonkey. 2017. [Accessed 2017 October 2]. http://www.surveymonkey.com.
- System Usability Scale (SUS). 1986. [Accessed 2017 November 6]. https://www.usability.gov/how-to-and-tools/methods/system-usability-scale.html
- YouTube. 2017. Video about how to work with the concept mapping tool. [Accessed 2017 Nov 6]. https://www.youtube.com/watch?v=WgQxinhiw24