Abstract
Background: Previous investigations of time-to-pregnancy recognition have analysed data from national surveys and clinics, but this has not been investigated in the context of digital fertility applications. Timely pregnancy recognition can help individuals in health and pregnancy management, reducing maternal and foetal risk and costs, whilst increasing treatment options, availability, and cost. Methods: This dataset contained 23,728 pregnancies (conceived between June 2018 and December 2022) from 20,429 participants using a Food and Drug Administration (FDA) cleared fertility app in the United States. Most participants (with non-missing information) identified as Non-Hispanic White, and one-third reported obtaining a university degree. We used two-tailed Welch’s t-test, Mann–Whitney U-test, and two-tailed Z-tests to compare time to pregnancy recognition between those using the app to conceive or contracept. Results: Participants using an app to conceive recognised pregnancy on average at 31.3 days from last menstrual period (LMP) compared to 35.9 days among those using the app to prevent pregnancy. Conclusion: Generalisability is limited, as all participants were using a fertility app and had relatively homogenous sociodemographic characteristics.
PLAIN LANGUAGE SUMMARY
People who recognise pregnancy early may benefit, as earlier recognition can reduce costs and risks, and make more treatment options available. In the past, researchers have studied the time it takes for an individual to recognise that they are pregnant by asking them in national surveys or when they attend a clinic. However, with the advent of digital fertility tracking apps, we investigated the time it takes to recognise pregnancy when using such an app. We analysed data from 23,728 pregnancies from 20,429 users of the Natural Cycles app between June 2018 and December 2022. We found that participants using the app to try to get pregnant recognised pregnancy an average of 4.6 days earlier than those using the app to prevent pregnancy.
Introduction
Scholars have called for research on pregnancy recognition trajectories across different contexts (Strong et al. Citation2023). Studies (with variable methodological approaches and definitions of pregnancy recognition) show inconsistent results concerning time to pregnancy recognition (TTPR) (), but generally, later recognition was more common among youth (Finer et al. Citation2006), those with lower education attainment, lower socioeconomic status, unintended pregnancy (Dott et al. Citation2010, Swanson et al. Citation2014, Ayoola Citation2015), or identifying as Black and Latino (Branum and Ahrens Citation2017). A 2021 study found that among women seeking second-trimester abortions in the United States (US), most recognised pregnancy more than 8 weeks after LMP; more than one in five recognised pregnancy after 20 weeks (Foster et al. Citation2021).
Table 1. Summary of the literature investigating time to pregnancy detection.
Timely recognition of pregnancy can facilitate health and pregnancy management. Those who will seek an abortion may benefit from earlier pregnancy detection (Ralph et al. Citation2022) which can broaden method options to include medication abortion, reduce cost of care, and reduce risk of complications (Jones et al. Citation2017; Upadhyay et al. Citation2022, Citation2015). Early pregnancy detection and first-trimester prenatal care may decrease the risk of adverse pregnancy outcomes (American College of Obstetricians and Gynecologists,Citation2005 Ayoola et al. Citation2009, Floyd et al. Citation2013). Attempts to predict pregnancy go back decades, often using Bayesian methods (Lum et al. Citation2016). Other women’s health tracking mobile applications have been used to predict pregnancy (Liu et al. Citation2019). Many of the hundred of fertility apps on the market are heterogeneous in their underlying methods of predicting fertile days and the benefit of using them to reduce time to pregnancy needs to be evaluated (Ali et al. Citation2020). Fecundability in relation to use of mobile computing apps to track the menstrual cycle has also been investigated, finding that they can increase fecundability from 12–20% (Stanford et al. Citation2020). Overall, individuals using fertility apps want the apps to be accurate, evidence based regardless of whether they are using them as contraceptives or for pregnancy planning (Earle et al. Citation2021). Better understanding of TTPR in the United States, as well as its variation according to multiple physiological or social variables, is needed to guide clinical counselling and legal guidelines (Watson and Angelotta Citation2022).
We aimed to investigate self-reported gestational age at pregnancy detection in a cohort of Natural Cycles (NC°) users, and to understand factors associated with TTPR.
Methods
We conducted a retrospective cohort analysis of prospectively collected data on pregnancy recognition, defined as the date participants reported the first positive pregnancy test result in the app. Natural Cycles users track menstrual cycle and basal body temperatures (BBT), and an algorithm predicts the most fertile days of their menstrual cycle, either for contraception or to aid conception. We focused this analysis on users residing in the United States who registered on the application after June 1, 2018 and were 18 to 45 years old. We studied all pregnancies (as defined below) indicated on the application between June 1, 2018 and January 1, 2023.
FDA-cleared fertility application
Natural Cycles is a mobile-based application for fertility monitoring and contraception cleared by the Food and Drug Administration (FDA) in the United States and marked by the Conformité Européenne (CE) in the European Union. Users must record BBT and menstruation dates and can, optionally, add results from home-based urine luteinizing hormone tests. The proprietary algorithm then estimates and shows the user’s most likely upcoming ovulation date, fertile window, and upcoming menstruation dates. Participants can indicate pregnancy by entering a positive urine pregnancy test result, in real time or retroactively.
The user indicates their intent to either plan or avoid a pregnancy by selecting between two modes: NC° Plan Pregnancy or NC° Birth Control. The selected mode does not influence estimates for ovulation and menstruation dates but affects the application’s urine pregnancy test recommendations encouraging NC° Plan Pregnancy users to test for pregnancy 1–5 days after their predicted menstruation, depending on their cycle regularity.
As a proxy for participant’s intention towards pregnancy, we relied on the NC° mode being used by participants at conception (e.g. NC° Plan Pregnancy or NC° Birth Control). The number of unintended pregnancies in this analysis was aligned with the published 13-cycle cumulative typical use pregnancy probability of 7.2% (Pearson et al. Citation2021).
Quantitative variables
We took the self-reported age for each conception cycle as their age on the first day of the cycle and classified the sample into five categories (18–24, 25–29, 30–34, 35–39, or 40–45 years). We computed the body mass index (BMI) from self-reported height and weight at the time of registration and classified the sample into four categories (<18.5, underweight; 18.5–25, normal weight; >25–30, overweight; >30, obese).
Menstrual cycle length was based on cycles recorded in the app from registration until the cycle prior to conception. We calculated average cycle length and classified these into six categories (0–20, >20–25, >25–30, >30–35, >35–40, >40 days). We defined two categories for the standard deviation in cycle length: <5 and ≥5 days. If no cycles were recorded on the application prior to conception, these variables were reported as missing data.
The application periodically requests sociodemographic information on race/ethnicity, parity, education level, relationship status, and the number of young children living with the participant but answering these questions in the application is voluntary. Sociodemographic variables that were never given by the participant are reported as No information. Furthermore, we only included information on the time-varying socio-demographic variables (i.e. parity, relationship status, and the number of young children living with the participant) if this information was updated within the year prior to the start of the conception cycle; otherwise we classified this information as No information. Socio-demographic information that was time-invariant or reasonably stable over time (race/ethnicity, education level) was not filtered according to the time it was indicated by the participant.
Outcomes
Our primary outcome was the number of days between the first day of the last recorded menstrual period (LMP) and the first positive self-reported pregnancy urine test result. Our secondary outcomes were the proportion of pregnancies in which recognition time since LMP was = > 6 and = >7 weeks.
Sensitivity analyses
We did not restrict the analysis according to the frequency of temperature measurement in the main analysis. Computation of TTPR relies on the correct tracking of the LMP and the first positive pregnancy result.
We performed a sensitivity analysis restricted to participants who added at least 10 BBT measurements between the 1st and 30th day of the conception cycle as a proxy for those most likely to accurately record menstruation or pregnancy test data in a timely manner.
Statistical analysis
We stratified the analytical population into two cohorts according to mode used in the application (NC° Plan Pregnancy vs. NC° Birth Control). For each cohort, we computed the sample mean and 95% confidence interval for TTPR.
We used two-tailed Welch’s t-tests to compare average TTPR between cohorts, Mann-Whitney U-tests to compare the probability distributions of TTPR between cohorts, and two-tailed Z-tests to compare proportions of late recognition time between cohorts.
We compared TTPR stratifying for one covariate at a time among sociodemographic variables and menstrual characteristics prior to pregnancy. We computed Kruskal–Wallis H-tests to compare mean TTPR without the assumption of equal variances among categories.
We conducted data extraction and analysis using Kotlin and Python, and we considered values of P < 0.01 as statistically significant.
Data privacy
All participants included in this analysis authorised use of their pseudonymized data for research purposes in the application. At any time, participants could remove this consent (including for retrospective data). Researchers only had access to pseudonymized copies of data and were unable to derive the identity of any participant. Natural Cycles is compliant with General Data Protection Regulation (GDPR) standards. We obtained an ethics waiver for analysis of pseudonymized data (Reading Independent Ethics Committee, 10022023).
Results
The dataset contains 23,728 pregnancies from 20,429 participants (). Beforehand, we excluded 34 pregnancies for being logged over 40 weeks after last logged menstruation, which would exceed the expected biological length of pregnancy. Participants originated from 50 states, the District of Columbia, and the four territories of the United States.
Overall, two-thirds (75.6%) of pregnancies occurred in individuals aged 25–35 (). Among the 27% who indicated race/ethnicity, 82.0% identified as non-Hispanic White. A total of 45.0% reported obtaining a university or doctoral degree, whereas 47.5% did not receive or answer the educational level question. We had substantial fractions of missing data for all variables except age (), for example, BMI remains unknown for 41.1% of participants.
Table 2. Demographic characteristics: distribution of the pregnancies (n = 23728) and stratified mean time from the last menstrual period to the first positive pregnancy test (NC° Plan Pregnancy vs NC° Birth Control).
NC° Plan Pregnancy users had significantly higher temperature measurement frequencies versus NC° Birth control users (56.2% of days during first 30 days of the conception cycle, versus 48.8% of days (p-value <0.001, data not shown)). Additionally, the mean number of urine LH tests within the studied conception cycle was 3.0 for NC° Plan Pregnancy and 1.2 for NC° Birth control (p-value <0.001, data not shown).
Time to pregnancy recognition
Pregnancies occurring on NC° Plan Pregnancy were recognised an average of 4.6 days earlier than those on NC° Birth Control; respectively, 31.3 days versus 35.9 days from LMP (, , p-value <0.001).
Figure 2. Mean time from last menstrual period to first positive pregnancy test: NC° Plan Pregnancy vs NC° Birth Control.
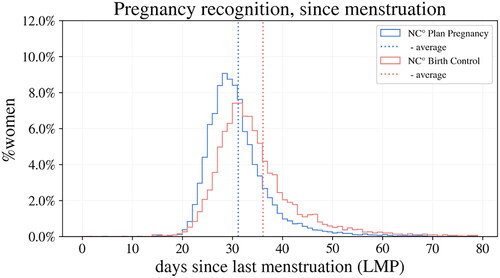
Table 3. Time to pregnancy confirmation (days from the first day of the last menstrual period to the first positive pregnancy test), average and right tail of the distribution: NC° Plan Pregnancy vs NC° Birth Control.
A significantly smaller proportion of NC° Plan Pregnancy users (6.5%) than NC° Birth Control users (15.7%) recognised the pregnancy later than six weeks after LMP (, p-value <0.001). Similarly, 3.0% of NC° Plan Pregnancy users and 7.6% of NC° Birth Control users recognised pregnancy later than seven weeks after LMP (p-value <0.001). We compared TTPR with observations from prior studies (Table S1).
Sensitivity analyses
We observed faster recognition times among participants with at least ten BBTs within the first 30 days of the conception cycle (), 0.8 and 1.7 days for participants on NC° Plan Pregnancy and NC° Birth Control, (p-value <0.001). In this subgroup, we also found a smaller proportion with later pregnancy recognition, especially for participants on NC° Birth Control: there was an absolute decrease of 2.6% and 2.0% in the proportion of participants recording a first positive pregnancy test at 6 and 7 weeks, respectively (p-value <0.001). We performed a second sensitivity analysis to evaluate the impact of in-application communication about late menstruation to participants on NC° Birth Control but found no significant difference in mean pregnancy recognition time between NC° Birth Control users receiving or not receiving warning messages on late menstruation (p-value = 0.53), see supplementary material (Table S2).
Effect of demographic and menstrual characteristics on pregnancy recognition
BMI, parity, and the presence of young children at home was associated with TTPR (). Among participants on NC° Plan Pregnancy, we observed longer pregnancy recognition time among NC° Plan Pregnancy users who registered a BMI above 30.0 (obese) or below 18.5 (underweight), (p-value = 0.004). On the contrary, parity (versus nulliparity) was associated with shorter mean recognition time and lower likelihood of late pregnancy recognition (p-value <0.001). Maternal age (p-value = 0.06) and living with young children (p-value = 0.06) were not significantly correlated to pregnancy recognition time. No significant differences across other demographic categories (race/ethnicity, relationship status, and education level) were observed (p > 0.05).
Both average cycle length and cycle length regularity had a significant influence on TTPR. Participants with irregular cycles (cycle-length standard deviation in previous cycles ≥ 5 days) or longer cycles took longer, on average, to recognise pregnancy (p-value <0.001 for both analyses).
Discussion
In this cohort of nearly thirty thousand individuals, pregnancies logged on NC° Plan Pregnancy and on NC° Birth Control were recognised at 31.3 and 35.9 days from the LMP, respectively. A significantly smaller proportion of NC° Plan Pregnancy users (6.5%) versus NC° Birth Control users (15.7%) recognised the pregnancy later than six weeks after the LMP.
This cohort of digital fertility application users (including both those trying to conceive and prevent pregnancy) appears to detect pregnancies earlier on average than populations studied in previous publications (Table S1). However, sociodemographic characteristics of our analytic population and those of previous studies differ substantially. For example, in Ralph et al. (Citation2022), study participants identified mostly as Latina (42%) or Non-Latina Black (22%), largely reported a high school diploma as their highest education level (74%), and nearly half received public assistance (42%). In comparison with the Ralph cohort, the Natural Cycles participants (the majority of whom, where data were provided, identified as non-Hispanic White and holding a university degree) recognised pregnancy on average 12 days sooner when trying to avoid it. Furthermore, the proportion of individuals recognising pregnancy over six or seven weeks after LMP in our study was less than one-third of that reported in previous studies (Ayoola,Citation2015 Branum and Ahrens Citation2017, McCarthy et al. Citation2018, Ralph et al. Citation2022).
Several factors may have contributed to the shorter pregnancy recognition time in our study. Natural Cycles relies on daily tracking of menstrual data; hence, users may be more alert to missed menstruation than the general population. In addition, the application provides estimations of the participant’s ovulation date and fertile window; therefore, participants may be comparatively more conscious of the likelihood of conception from unprotected intercourse on a day indicated by the app as fertile. Furthermore, in addition to the unique sociodemographic characteristics of our sample, our cohort may differ in other unmeasured ways from those who do not use an app for fertility tracking. For example, the application of Natural Cycles offers regular in-application content on sexual and reproductive health, which may improve knowledge of the signs and symptoms of pregnancy, which in turn may accelerate timely pregnancy recognition (Watson and Angelotta Citation2022).
The user-selected fertility mode had a significant impact on the TTPR, with participants who conceived in the NC° Plan Pregnancy mode logging a first positive pregnancy test on average five days earlier than those on NC° Birth Control. This result correlates closely with previous studies in which unintended or unwanted pregnancies were suspected, recognised, or confirmed later (Ayoola Citation2015, Branum and Ahrens Citation2017, Ralph et al. Citation2022). Participants actively planning a pregnancy are more prone to testing before or on the first day of missed menstruation, whereas those trying to avoid pregnancy suspect pregnancy only after late menstruation.
Sensitivity analyses suggest that more active app users recognised pregnancy earlier, particularly those using NC° Birth Control. Participants who use the application more regularly may have a higher interest in tracking their fertility, may receive more accurate menstruation predictions from the application, may have more knowledge on fertility and pregnancy, and are prompted earlier to test for pregnancy. Those using the application more sporadically may be less likely to correctly log their first positive pregnancy test on the actual date of testing, which would bias the pregnancy recognition time of this cohort towards longer durations.
The relationship between pregnancy recognition time and various demographic variables in our study showed some similarities and differences with previous studies. While age was not correlated with TTPR in our study, older age was associated with earlier pregnancy recognition elsewhere (Ayoola Citation2015, Branum and Ahrens Citation2017, Ralph et al. Citation2022). We also identified a relationship between parity and the presence of young children at home with faster pregnancy recognition, as in some (Foster et al. Citation2021) but not all other studies (Ayoola Citation2015, Branum and Ahrens Citation2017, Ralph et al. Citation2022). We observed no significant differences in pregnancy recognition time by relationship status, whereas other studies found that pregnancies were identified later if individuals were not married or argued more with their partners (Ayoola,Citation2015 Ralph et al. Citation2022). We observed no significant relationship between race/ethnicity and TTPR in our cohort. Previous cohorts among lower income, lower educated populations have shown an increase in TTPR among Hispanic and Non-Hispanic Black compared to Non-Hispanic White individuals. Since race is a sociologic and not a biologic construct, these differences, if present, may be due to systemic inequities in healthcare access literacy and body literacy. (Ayoola Citation2015, Branum and Ahrens Citation2017, Ralph et al. Citation2022). Our findings may be different because this group had fewer other adverse social determinants (e.g. education, health insurance) than previous cohorts. Additionally, our sample was relatively homogenous, and had substantial missing data for several socio-demographic variables, thus limiting our ability to detect potential differences.
We also observed later pregnancy recognition among participants with longer or irregular menstrual cycles, which can be easily explained by physiological and behavioural factors. A longer follicular phase delays fertilisation, implantation, and hCG increase. Consequently, pregnancy recognition is naturally farther away from the LMP. An individual with a more irregular cycle may take longer to suspect pregnancy as delayed menses are ‘normal’ for them. The average cycle length, average follicular phase length, and cycle length variation did not vary significantly between the pregnancies on NC° Plan Pregnancy and NC° Birth Control, and hence did not contribute to the observed gap in pregnancy recognition time between the two cohorts.
Our study had several limitations, including substantial missing data for several sociodemographic characteristics. This is due to the fact that this is a pragmatic study conducted amongst a real-world population of contraceptive app users not in the context of a clinical trial. Users were not required to input all data into the app in order to use it. The benefit to this approach is studying how real-world fertility awareness app users interact with pregnancy testing.
We also did not collect data on all social determinants of health which have been observed to be correlated with the timing of pregnancy recognition, such as food insecurity, (Ralph et al. Citation2022). However, the sociodemographic information collected shows the cohort to be a relatively homogeneous sample, not representative of the general population in the US. In our cohort, participants predominantly identified as Non-Hispanic White (82.0% compared to 59.3% in the general population (United States Census Bureau Citation2023)) and highly educated (85.8% of those who answered the question had obtained a university degree). Our dataset, representing only women who use a fertility awareness-based method, is likely different from the general population in ways that would impact TTPR. Some misclassification of pregnancy intentions is also possible in our data. We cannot draw meaningful conclusions for the cohort with the shortest cycles with an average cycle length below 20 days due to the small size of the sample. Finally, this study focused solely on the recognition of pregnancy through a positive pregnancy urine test result. Pregnancy suspicion based on physical symptoms and pregnancy confirmation by a healthcare professional were not captured.
Furthermore, this study could only compare the TTPR in cycles where a positive urine pregnancy test was logged into the application. However, there could be a difference in the frequency of unreported pregnancy tests between participants planning to conceive and those intending to avoid pregnancy, which could influence the observed difference in TTPR between those cohorts.
Timely recognition of pregnancy can aid individuals in their health and pregnancy management. Not all fertility-tracking apps are regulated, and some may not have robust data privacy policies in place for the benefit of the user (Mozilla Citation2022). In such cases, any gains in the speed of pregnancy awareness may need to be balanced against the potential risks of uploading pregnancy-related information, particularly in places where abortions are criminalised.
Author contributions
AvL and EB designed, performed and interpreted the analysis and wrote the manuscript, JTP, RU, KGD, HKK, CF, AN, EB and RS interpreted the analysis, wrote and reviewed the manuscript.
Supplemental Material
Download MS Word (33 KB)Acknowledgements
We would like to express our sincere gratitude to the participants, who generously consented to sharing their anonymized menstrual and fertility data on the Natural Cycles application for research. A special thank you to Chelsea Polis who provided substantial input into prior versions of this manuscript; she has not received any financial support from Natural Cycles.
Disclosure statement
AvL, JP, EB, and CF are employees of Natural Cycles with shares or stock warrants in the company. EBS and RS are the CEO’s and Co-founders of Natural Cycles and have shares in the company. KGD received honorarium for the support of manuscript writing and as a speaker at meetings for Natural Cycles. HKK received a past honorarium from Natural Cycles. AN had previously attended an advisory board for Natural Cycles. CBP and RU did not receive any financial support from Natural Cycles.
Data availability statement
Data is available upon reasonable request to the corresponding author.
Additional information
Funding
References
- Ali, R., Gürtin, Z. B. and Harper, J. C., 2020. Do fertility tracking applications offer women useful information about their fertile window? Reproductive Biomedicine Online. doi: 10.1016/j.rbmo.2020.09.005.
- American College of Obstetricians and Gynecologists. 2005. ACOG Committee Opinion Number 313, September 2005. The importance of preconception care in the continuum of women’s health care. Obstetrics and Gynecology, 106 (3), 1–9.
- Ayoola, A. B., 2015. Late recognition of unintended pregnancies. Public Health Nursing (Boston, Mass.), 32 (5), 462–470.
- Ayoola, A. B., Stommel, M. and Nettleman, M. D., 2009. Late recognition of pregnancy as a predictor of adverse birth outcomes. American Journal of Obstetrics and Gynecology, 201 (2), 156.e1–6–156.e6.
- Branum, A. M. and Ahrens, K. A., 2017. Trends in timing of pregnancy awareness among US women. Maternal and Child Health Journal, 21 (4), 715–726.
- Dott, M., National Birth Defects Prevention Study., et al., 2010.Association between pregnancy intention and reproductive-health related behaviors before and after pregnancy recognition, national birth defects prevention study, 1997-2002. Maternal and Child Health Journal, 14 (3), 373–381
- Earle, S., et al., 2021. Use of menstruation and fertility app trackers: a scoping review of the evidence. BMJ Sexual and Reproductive Health, 47 (2), 90–101.
- Finer, L. B., et al., 2006. Timing of steps and reasons for delays in obtaining abortions in the United States. Contraception, 74 (4), 334–344.
- Floyd, R. L., et al., 2013. A national action plan for promoting preconception health and health care in the United States (2012-2014). Journal of Women’s Health (2002), 22 (10), 797–802.
- Foster, D. G., Gould, H. and Biggs, M. A., 2021. Timing of pregnancy discovery among women seeking abortion. Contraception, 104 (6), 642–647.
- Jones, H. E., et al., 2017. First trimester medication abortion practice in the United States and Canada. PLoS One, 12 (10), e0186487.
- Liu, B., et al., 2019. Predicting pregnancy using large-scale data from a women’s health tracking mobile application. Proceedings of the. International World-Wide Web Conference. International WWW Conference, 2019, 2999–3005.
- Lum, K. J., et al., 2016. A Bayesian joint model of menstrual cycle length and fecundity. Biometrics, 72 (1), 193–203.
- McCarthy, M., et al., 2018. Predictors of timing of pregnancy discovery. Contraception, 97 (4), 303–308.
- Mozilla 2022. In Post Roe v. Wade Era, Mozilla Labels 18 of 25 Popular Period and Pregnancy Tracking Tech With *Privacy Not Included Warning. Accessed 27th April 2023, https://foundation.mozilla.org/en/blog/in-post-roe-v-wade-era-mozilla-labels-18-of-25-popular-period-and-pregnancy-tracking-tech-with-privacy-not-included-warning/.
- Pearson, J. T., et al., 2021. Contraceptive effectiveness of an FDA-cleared birth control app: results from the natural cycles U.S. Cohort. Journal of Women’s Health (2002), 30 (6), 782–788.
- Ralph, L. J., et al., 2022. Home pregnancy test use and timing of pregnancy confirmation among people seeking health care. Contraception, 107, 10–16.
- Stanford, J. B., et al., 2020. Fecundability in relation to use of mobile computing apps to track the menstrual cycle. Human Reproduction (Oxford, England), 35 (10), 2245–2252.
- Strong, J., et al., 2023. Pregnancy recognition trajectories: a needed framework. Sexual and Reproductive Health Matters, 31 (1), 2167552.
- Swanson, M., et al., 2014. Delayed pregnancy testing and second-trimester abortion: can public health interventions assist with earlier detection of unintended pregnancy? Contraception, 89 (5), 400–406.
- United States Census Bureau 2023. Quick facts, United states. Available at: https://www.census.gov/quickfacts/fact/table/US/RHI825221#RHI825221
- Upadhyay, U. D., et al., 2015. Incidence of emergency department visits and complications after abortion. Obstetrics and Gynecology, 125 (1), 175–183.
- Upadhyay, U. D., et al., 2022. Trends in self-pay charges and insurance acceptance for abortion in the United States, 2017-20. Health Affairs (Millwood), 41 (4), 507–515.
- Watson, K. and Angelotta, C., 2022. The frequency of pregnancy recognition across the gestational spectrum and its consequences in the United States. Perspectives on Sexual and Reproductive Health, 54 (2), 32–37.