Abstract
Classifying behavior by tracking acceleration has received increased interest lately. Here, we evaluated the performance of three commercial activity trackers in differentiating seven dog behaviors. Adult companion dogs (N = 70) performed still (lying, sitting, standing) and dynamic (walking, sniffing, trotting, playing) tasks, while wearing ActiGraph GT9X Link, Kaunila and FitBark devices placed on the neck collar and ActiGraph GT9X Link placed on the back. Each task was performed for 3 min within a session and repeated in two sessions; the behaviors were confirmed from video recordings. Activity scores of devices were calculated as median values for behavioral differentiation, and as minute-based values for inter-device correlations and cutoff analysis. Measurements of all devices correlated with each other, and median activity scores of all devices − unaffected by dog age, weight or sex − differentiated the still from dynamic behaviors. Dynamic behaviors were also differentiated from each other, with exception of walking vs. sniffing by back-placed ActiGraph GT9X and Kaunila. The definition of cutoffs between behaviors varied from moderate to high accuracy; defined cutoffs for standing and walking were the least accurate. The classification performance of the cutoffs had an accuracy of 80% in all the devices; thus, they performed reasonably well in classifying these behaviors.
1. Introduction
Behavior quantification based on large databases and the utilization of artificial intelligence are becoming more popular also in the field of veterinary medicine − increasing, for example, the standardization of methods [Citation1]. Behavior-related databases can be compiled in many ways, and tracking the activity of animals by their motion and acceleration in certain situations is one way of collecting such information [Citation2–4]. For dog owners, commercially available activity trackers can provide motivational information related to dog state and exercise [Citation5,Citation6]. However, activity trackers can encompass a wide variety of devices with different properties and components. They vary from devices containing the simplest uniaxial accelerometers to more complex devices with triaxial accelerometers, triaxial gyroscopes, triaxial magnetometers and thermometers, among other components.
In animal research, activity trackers are used for estimating energy expenditure and assessing behaviors in wildlife studies (e.g. [Citation7–9]), animal welfare research [Citation10] and in veterinary medicine (e.g. [Citation11–13]). There are several devices for research purposes, from which raw data can be extracted, such as Hobo [Citation14], VetSens [Citation15], ActiGraph GT3X/GT3X + [Citation16,Citation17] and Actical [Citation18,Citation19]. The latter two are the most commonly used in canine studies (e.g. [Citation11,Citation13,Citation17]). On the other hand, commercial dog activity trackers have become popular in everyday life activity monitoring of pet dogs. These devices are small, inexpensive and easy to use; they are targeted at dog owners and also have the potential for clinical use [Citation20] but only limited information about their accuracy and validity is offered. Of the commercially available dog activity trackers, Whistle [Citation21], and initially also PetPace [Citation22] have been validated with or close to scientific accuracy; additionally, PetDialog+ and FitBark have been examined with observational methods [Citation20,Citation23].
The most useful feature of the activity trackers, both scientific and for everyday use, is differentiating various dog behaviors from the tracker data. There have been multiple attempts and approaches to extracting behavioral classes from activity measurements. The potentially more precise approach, but also more technologically complex, is using different algorithm classifiers and machine learning techniques (e.g. [Citation24–26]). The behavior classification approach has reached 69% of accuracy for differentiating 16 separate behaviors, showing better performance for small and medium dogs than for large dogs [Citation26]. More recent studies have succeeded in detecting resting and itching-related (i.e. head shaking and scratching) behaviors with high accuracies (86% and >99%, respectively) [Citation27,Citation28]. Another potentially less accurate, but easier-to-apply, approach is the determination of thresholds (i.e. cutoff values) for each behavior using ROC (Receiver Operating Characteristic) curves. Typically, cutoffs have been used to define up to three activity levels in dogs [Citation16,Citation17]. In this study, we used this latter approach.
In addition to the activity of the dog itself, other factors might also affect the activity readings, such as the placement of the tracker [Citation29,Citation30] and dog signalment (e.g. [Citation31–33]). In the current study, we focused on medium- to large-sized dogs and compared activity tracker placement on the ventral neck versus on the back, between scapulae. Furthermore, links between activity, age and weight were considered, as younger dogs may have higher activity scores [Citation31–34] and heavier or overweight dogs might have lower activity scores [Citation17,Citation31,Citation35,Citation36].
Thus, dog activity trackers are multifunctional and promising tools for both scientists and dog owners to detect changes in the health and behavior of dogs, but they often lack scientifically precise and openly communicated reliability measures. Therefore, we compared two commercial activity trackers targeted for consumer usage and aimed for tracking dog activity (Kaunila and FitBark) with a validated research device earlier utilized in many different kinds of acceleration measurements (ActiGraph GT9X Link) to assess their reliability in canine activity measurement. We utilized simultaneously four separate accelerometer measurement devices, as one ActiGraph device was attached to the neck (collar) and one at the back (harness) of the dog; one Kaunila and one FitBark device were attached to the neck (collar). We also clarified whether activity scores differ statistically among behaviors; how many behaviors they allow to differentiate; how accurately the differentiation can be conducted; and, by comparing the ActiGraph devices in the neck and the back of the dog, what is the best placement for the devices to obtain the previous goals.
2. Materials and methods
2.1. Animals
The experiments were conducted in the Faculty of Veterinary Medicine at the University of Helsinki. The procedures were approved by the Viikki Campus Research Ethics Committee at the University of Helsinki (minutes 5/2017) and all dog owners completed an informed written consent before participating in the study.
A total of 70 healthy pet dogs (19 intact females, 19 neutered females, 25 intact males, 7 neutered males) from 29 breeds and 5 crossbred dogs participated in the study. Their mean age was 4.8 years (range 1–9 years) and their mean weight was 24.5 kg (range 13–41 kg) (Table S1). Participants, with the inclusion criteria of 10–50 kg weight and 1–10 years of age, were recruited through the internet (the project website) and social media (Facebook).
2.2. Equipment
During the tests, dogs wore four separate commercial activity tracker devices, three of which were different from one another (Table ). Kaunila, FitBark and one ActiGraph GT9X Link were taped tightly on the ventral side of the neck collar in this order (exchanging randomly the position of Kaunila and FitBark between dogs), and another ActiGraph GT9X Link was placed inside a tight pocket made of neoprene on the back belt of dog harness.
Table 1. Information about the activity trackers used in the experiment and their placement in this study.
2.3. Procedure
The measurements were conducted in a dog sporting hall with artificial turf. The size of the testing arena was 10 m × 18 m. There were two testing sessions (Test 1 and Test 2) each of them consisting of seven 3-minute lasting tasks: three still (lying, sitting, standing) and four dynamic (walking, sniffing, trotting, playing) behaviors (Figure ). The tasks were performed sequentially in a semi-randomized order per dog and session, alternating between still and dynamic tasks and ending always with a sniffing task. In walking and trotting tasks, the dog ran around the arena in a clockwise direction in Test 1 and in a counterclockwise direction in Test 2. The sniffing task consisted of placing small pieces of dog treats on the floor (spread over an area of 4 m × 4 m, adjacent to the testing arena) and allowing the dog to search them by sniffing. Playing with the dog in free-style aimed at promoting intense activity, such as running after a ball and playing a tug-of-war game. Except in playing and sniffing tasks, dogs were on leash and led by their owner or experimenter. The leash (1.5 m) was attached to a separate collar than the one to which the devices were attached to; the device collar was placed cranially. Owners were free to give food rewards and give cues to their dogs as considered necessary during the test. A resting period for a mean of 34 ± 21 s minutes (range 29−46 min) was kept between the two testing sessions.
2.4. Behavioral recording
Dogs’ movements and behaviors were video-recorded during the test with Panasonic HDC-SD600 and Sony HDR-CX450 cameras positioned in opposite lateral walls toward the testing arena. The behavior of each dog was annotated from video recordings post-hoc with Observer XT 10.5 (Noldus, The Netherlands); see Table . Only one behavior at a time, lasting ≥ 1 s, was annotated. Criteria for still behaviors were that limbs were not moving and that there was no physical contact between handler and dog, except if a treat was given. For walking and trotting, the behavior was annotated if the gait pattern was clear and continuous without leaning toward the handler or pulling the leash, so that it would affect the gait pattern.
Table 2. Ethogram for annotation of dogs’ behaviors from video recordings.
2.5. Data collection
Activity data were extracted from the FitBark and Kaunila activity trackers as minute-by-minute total activity scores, hereafter referred to as ‘activity scores’. These were derived from triaxial accelerometer data with manufacturers’ built-in algorithms. The data of these devices were sent to the cloud server via their respective mobile phone applications. FitBark activity scores were extracted from the web dashboard and Kaunila activity scores were obtained via the manufacturer; the exact algorithm used by the devices for calculating the activity scores from the accelerometer sensors was not open for the authors as these are commercial devices targeted for consumer usage. As the ActiGraph GT9X Link is a more research-directed device that is used for many kinds of acceleration measurement, the minute-by-minute activity value (vector magnitude; the square root of the sum of the squares of each of the three accelerometer axes) was extracted from 100 Hz triaxial accelerometer data using ActiLife software (ActiGraph LLC, USA). Due to the individual algorithms, these three different devices utilized in our study have also differing scales for activity scores. In our study including still and dynamic canine tasks, ActiGraph GT9X Link activity score values were approximately 0–25 000 (|v|); Kaunila activity scale values were approx. 0–2500 (a.u.) and FitBark activity scores were approx. 0–300 (a.u.). All of these values are represented henceforth as counts per minute (cpm), unless otherwise stated.
All the measurements were aligned up to a minute precision for each dog by the maximum significant value in their cross-correlation. Behaviors obtained from video recordings were aligned with the activity scores of the devices by syncing the time of the laptop and phone associated with the activity trackers with one-second accuracy and showing it to the camera at the beginning of the test. The total duration of each behavior was calculated in seconds per each minute. In other words, all the seconds within a minute performing a behavior according to the ethogram were summed up and divided by 60 to obtain a percentage of the minute performing that behavior that was later used as criteria for including and excluding data points (see Statistical analyses).
2.6. Statistical analyses
All statistical analyses were carried out with IBM SPSS Statistics software version 24 (IBM Corp, USA) including Jon Peck (2013) and David Nichols (2015) Stats weighted Kappa.spe extension. The significance level for all tests was p < 0.05. The automatization of the analyses, the data splitting, the sensitivity and specificity calculation, and the calculation of the Youden index were performed using Python 3.
The statistical analyses included three parts: differentiating behaviors; determining cutoffs for the statistically different behaviors of each device; and comparing between minute-based activity scores of each device directly and investigating the effect of age, sex and weight on the mean activity scores. The analyses of the two former parts included the data of the minutes in which the dog performed the target behavior according to the ethogram ≥92% of that minute (i.e. ≥ 55 s of the 60 s). For statistical analysis, still behaviors were also combined together as an additional static category. This category consisted of the behaviors of lying, sitting and standing when one and only one of those behaviors fulfilled the time requirement (≥ 92% of the minute); in other words, those minutes got double label: lying, sitting or standing, as well as ‘static’.
Minute-based activity scores were not normally distributed; thus a nonparametric approach was selected. A Friedman test followed by Wilcoxon Signed Ranks tests were used for behavioral differentiation analyses of median activity scores of the behaviors within devices and multiple comparisons were corrected by the False Discovery Rate (FDR) setting the q-value at 0.05. Results are reported as medians with their respective first and third quartiles (Q1 and Q3). Those behaviors whose median activity scores differed from each other regarding Wilcoxon Signed Ranks tests were further used to analyze the cutoffs between different behaviors. For the usage in ROC curves, the behaviors or categories, between which the activity scores did not differ according to Wilcoxon Signed Ranks tests, were regrouped to form new behavior categories and reanalyzed as one behavior (i.e. a new activity score median was calculated including the data from the behaviors forming it) until clearly distinct behaviors or categories could be established.
Next, a 10-fold cross-validation was performed, using the training subsets of data to determine the optimal cutoff values of activity scores and the testing subsets to evaluate its performance. Following the procedure of Morrison et al. [Citation37], ROC curves were calculated with SPSS software, assigning the positive value (1) to the behavior for which the cutoff was calculated and the negative value (0) to the others. Those scores with the maximum generalized Youden value (J) were selected in each subset and the average among the scores with the highest generalized Youden value was considered as optimal [Citation38]. Reported results are area under the curve (AUC), sensitivity (Se) and specificity (Sp) of cutoffs. Criteria for AUC accuracy defined by Greiner et al. [Citation39] were used: highly accurate (AUC > 0.9), moderately accurate (0.7 < AUC ≤ 0.9), less accurate (0.5 < AUC ≤ 0.7) or noninformative (AUC ≤ 0.5). Contingency tables and two quadratic weighted kappa values (κ) were used to evaluate the cutoff classification performance. Both κ included all the behaviors whose cutoffs were calculated, but one (κstill) did not include static and the other (κstatic) included none of the respective still behaviors but the static category, unless otherwise stated in the text. Altman [Citation40] criteria were used for rating κ values: ‘poor’ (≤0.20); ‘fair’ (0.21–0.40); ‘moderate’ (0.41–0.60); ‘good’ (0.61–0.8) and ‘very good’ (0.81–1.00).
Furthermore, Spearman’s correlation coefficients between minute-based test activity scores of each device were calculated. Pearson’s correlation coefficients for dog age and weight and mean activity scores per dog and device were also calculated. The differences between sexes and their interaction with neutering status in mean activity scores were also tested by using one-way ANOVA. Pearson’s correlation and ANOVA results are reported as supporting information (Table S2 and Table S3, respectively).
3. Results
3.1. Activity score agreement between devices and with dog signalment
Data were collected for a mean of 57 min per dog and device (range of 41–68 min). However, at least part of the Kaunila data (in seven dogs) and ActiGraph GT9X Link data (in six dogs) were lost due to technical problems, affecting a total of 25 testing sessions. Therefore, a total of 3964 min were collected from ActiGraph GT9X Link placed on the back, 3733 min from the ActiGraph GT9X Link placed on the neck, 4010 min from FitBark and 3644 min from Kaunila. A total of 3073 min from 58 dogs were obtained simultaneously from all devices and used to calculate the comparisons in Figures and . From those, a total of 988, 907, 996 and 922 min (respectively, 4–30 min per dog) fulfilled the time criteria of a behavior lasting ≥92% of the minute (i.e. 55 s of the minute) to be included in differentiation and classification analyses.
Figure 2. Correlation of the activity scores obtained by different devices (panels A-C) and locations (panel D), given in counts per minute (cpm).

Figure 3. Medians (with Q1 and Q3) of minute-based total activity score (in counts per minute; cpm) for the analyzed behaviors (in the horizontal axis), measured by the four devices.
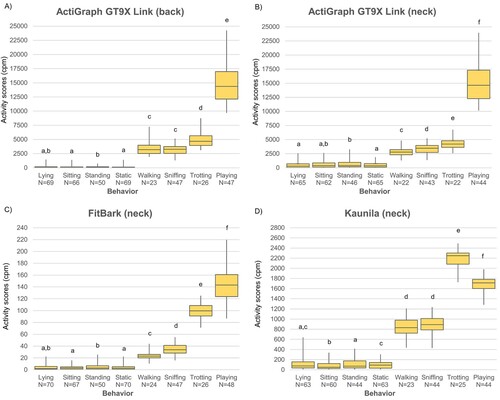
Activity scores of the three activity trackers were correlated statistically significantly strongly or very strongly with each other, despite utilizing different scales (Figure ). Neither age nor weight correlated with the mean activity scores of any device (Table S2) and neither sex nor its interaction with neutering status had an effect on activity scores (Table S3).
3.2. Behavioral differentiation
Median activity scores of dynamic behaviors for each device except Kaunila were, from the lowest to the highest scores, as follows: walking, sniffing, trotting and playing. For Kaunila, the median activity scores per dog were higher for trotting than for playing (see Figure ). The median activity scores between walking vs. sniffing by Kaunila and the back-placed ActiGraph GT9X Link did not differ significantly (Wilcoxon, p > 0.05). Furthermore, the median activity scores did not differentiate between the still behaviors (lying, sitting and standing) in any of the devices (see Figure ). Median activity scores differed significantly between all the other behaviors in all devices (Wilcoxon, p < 0.05).
3.3. Determination of activity cutoffs for the behaviors
Statistical difference between behaviors is needed to be able to correctly determine thresholds between categories using ROC curves. Thus, the behaviors that did not differ from each other in terms of the median activity scores were combined into new categories, selecting those combinations of behaviors that maximized the total number of categories. Following this procedure, the new behavioral categories (statistically differing from the other categories, all p < 0.05, Wilcoxon) were the following: lying-sitting for all devices except from Kaunila, Lying-Standing for Kaunila and walking-sniffing for the ActiGraph GT9X Link placed on the back and Kaunila.
ROC curves were calculated based on the statistically different behaviors and new behavioral categories (Table ). Behavior classification accuracy was moderate to high for all behaviors in all devices (Table ), except for standing in the ActiGraph GT9X Link (both locations) and walking in the ActiGraph GT9X Link placed on the neck and FitBark, which were less accurate.
Table 3. Accelerometer cutoffs and their accuracy for the different behaviors (lying, sitting, standing, walking, sniffing, trotting and playing) and devices (ActiGraph GT9X Link placed on the back and on the neck, FitBark and Kaunila). Cutoff = mean optimal cutoff calculated among the 10 training subsets; AUC (95% CI) = mean area under the curve with its 95% confidence interval among the 10 training subsets; Se = mean sensitivity among the 10 training subsets and Sp = mean specificity among the 10 training subsets.
When these cutoffs were applied to the testing subsets, the cutoffs showed very good agreement, classified according to Altman [Citation40] between the observed behavior and that classified using the activity scores for all devices (mean κstill ≥ 0.88 for all devices). The agreement was further improved when still behaviors were regrouped as a static category (mean κstatic ≥ 0.93). Tables show the confusion matrices of the classification accuracies of the ActiGraph GT9X Link placed on the back (Table ); the ActiGraph GT9X Link placed on the neck (Table ); FitBark (Table ) and Kaunila (Table ), for the behaviors classified using activity scores. In all these tables, zeros have been omitted for readability purposes.
Table 4. Confusion matrix showing the classification accuracy of ActiGraph GT9X Link placed on the back for the behaviors classified using activity scores. Classification accuracy is reported as the percentage (%) of the average amount of minutes fulfilling the time requirement and belonging to each category among the 10 testing subsets.
Table 5. Confusion matrix showing the classification accuracy of ActiGraph GT9X Link placed on the neck for the behaviors classified using activity scores. Classification accuracy is reported as the percentage (%) of the average amount of minutes fulfilling the time requirement and belonging to each category among the 10 testing subsets.
Table 6. Confusion matrix showing the classification accuracy of FitBark placed on the neck for the behaviors classified using activity scores. Classification accuracy is reported as the percentage (%) of the average amount of minutes fulfilling the time requirement and belonging to each category among the 10 testing subsets.
Table 7. Confusion matrix showing the classification accuracy of Kaunila placed on the neck for the behaviors classified using activity scores. Classification accuracy is reported as the percentage (%) of the average amount of minutes fulfilling the time requirement and belonging to each category among the 10 testing subsets.
The κstill and κstatic calculations included those the behavior categories that showed a statistically significant difference (as shown in Figure ), and for clarity, the categories are given in brackets. For the back-placed ActiGraph GT9X Link, the measurement of agreement between recorded and all possible classified behaviors (lying-sitting, standing, walking-sniffing, trotting, playing) κstill = (95% CI): 0.922 (0.889–0.955); and the measurement of agreement between recorded and classified behaviors, with the alternatively combined static category (static, walking-sniffing, trotting, playing) κstatic = (95% CI): 0.975 (0.953–0.995). For the neck-placed ActiGraph GT9X Link, the measurement of agreement between recorded and classified behaviors (lying-sitting, standing, walking, sniffing, trotting, playing) κstill = (95% CI): 0.880 (0.826–0.935); and the measurement of agreement between recorded and classified behaviors, with the alternatively combined static category (static, standing, walking, sniffing, trotting, playing) κstatic = (95% CI): 0.937 (0.895–0.980). For FitBark (only placed on the neck), the measurement of agreement between recorded and all possible classified behaviors (lying-sitting, standing, walking, sniffing, trotting, playing) κstill = (95% CI): 0.901 (0.858–0.943); and the measurement of agreement between recorded and classified behaviors, with the alternatively combined static category (static, standing, walking, sniffing, trotting, playing) κstatic (95% CI): 0.961 (0.935–0.988). For Kaunila (only placed on the neck), the measurement of agreement between recorded and classified behaviors (sitting, lying-standing, walking-sniffing, playing, trotting) κstill = (95% CI): 0.883 (0.837–0.929); and measurement of agreement between recorded and classified behaviors with the static category included (static, walking-sniffing, playing, trotting) κstatic = (95% CI): 0.985 (0.969–1).
4. Discussion
Today, commercially available activity trackers are rather affordable and easy to use, and they can provide information of dog exercise and behavior that dog owners may find interesting and useful in their daily lives [Citation5,Citation6]. Here, we clarified the accuracy of three commercially available activity trackers, two of which were especially targeted for pet owners to be used with dogs (Kaunila and FitBark). We compared the recordings of the devices during a semi-controlled test in seven different tasks, in which the dog behavior and motion were confirmed from a video, and found that the activity scores (i.e. activity points per a minute) of all three devices were strongly correlated. Activity scores allowed differentiation of four to six behavioral categories out of the initial seven. Nevertheless, none of the devices completely differentiated between the still postures (lying, sitting and standing) from each other. Higher agreement between the video-annotated and the classified behaviors was achieved when less categories were classified, i.e. when walking and sniffing were combined; or when the still tasks were regrouped as one static category.
Interestingly, the behaviors that could be differentiated depended on the device. Activity scores of the back-placed ActiGraph GT9X Link or neck-placed Kaunila did not differentiate walking from sniffing, but the activity scores of both the neck-placed ActiGraph GT9X Link and neck-placed FitBark differentiated these dynamic behaviors from each other, although they presented less accurate classification together with standing compared to the other behaviors. It is possible that the back placed ActiGraph GT9X Link did not differentiate between walking vs. sniffing because of its position: dogs mainly walked in sniffing, stopping a few times with minor head movements, which might be more difficult to detect by the device placed on the back. However, the placement does not explain why Kaunila activity scores did not differ between walking vs. sniffing, as Kaunila was placed on the neck together with the other Actigraph GT9X Link and FitBark. Also, the Kaunila device had some other particularities related to activity scores. First, Kaunila activity scores grouped lying and standing together and separated sitting from these, while the other devices grouped lying and sitting together and separated standing from these two. Second, the activity scores of Kaunila were significantly higher for trotting than for playing, as opposed to the other devices, in which the activity scores were higher for playing than for trotting.
The device-related differences in grouping the behaviors might be related to a different G threshold utilized in the accelerometer of these devices and the algorithms of each company. Previously, the G threshold value was determined to have the possibility to detect different types of movements in dogs, such as head movements, position transitions and whole-body movements (i.e. dynamic behaviors) keeping the device in the same placement [Citation41]. Therefore, probably due to its G threshold, the Kaunila device may detect accelerations of behaviors associated with wider body translocations, to which higher scores were assigned. Another possible or complementary explanation is that it may filter out or assign lower scores to the smaller accelerations that imply only partial body translocations (e.g. fast turning, tugging) or smaller position transitions. This could also be related to the fact that the classification performance of Kaunila activity scores that implied different activity levels (static, walking-sniffing, playing and trotting) was higher than the performance of the other devices. However, Kaunila activity scores did not differentiate more subtle behaviors, such as walking from sniffing, when the other devices placed on the neck did differentiate these. Nevertheless, the G thresholds or any other parameters of the algorithms for signal processing were not available for all the studied devices, thus we cannot confirm the reasons behind the difference.
The results of our study regarding the ActiGraph GT9X Link used in dogs are in line with previous literature: the obtained cutoff values of static category for the ActiGraph GT9X Link placed on the neck were similar as previously found with ActiGraph GT3X in the dorsal neck (<1352 cpm; Se = 95%) [Citation16,Citation17]. The small differences between the cutoffs could be due to multiple factors, such as the different version of the device, the slightly different placement and the small posture readjustment that was allowed only in this study and the up to 5 s in each minute doing another behavior. However, light–moderate (1352–5696 cpm) and vigorous (>5696 cpm; Se = 92.5%) behaviors of the previous studies [Citation16,Citation17] were not directly comparable to ours. In this study, playing behavior was defined and intended to be really intense and according to its cutoff, it clearly belongs to the previously established category of vigorous dog behavior [Citation16,Citation17]. On the other hand, trotting included a range of speeds, so probably the faster ones fitted into the definition of vigorous activity [Citation16], but the slower speeds belonged to the light–moderate category instead. Therefore, the lower cutoff obtained for trotting in this study was smaller than the lower cutoff for the previous studies [Citation16,Citation17]. Nevertheless, the obtained differences were minor, and generally, the activity scores of all examined devices allowed for behavioral differentiation, especially when behaviors clearly differed in their intensity.
The classification of the FitBark device agreed with those of the ActiGraph GT9X Link placed on the neck collar. Recently, the activity data produced by FitBark were been compared to the dog step counted from a recorded video where step counts and FitBark activity were highly correlated when the dogs were exploring a room off-leash and when they were interacting with their owner [Citation23]. When the dogs were being walked on a leash, the correlation between step counts and FitBark activity counts was somewhat smaller but statistically significant. In our study, the dogs were mostly on leash. The dynamic tasks of walking and trotting were performed on leash, whereas sniffing and playing were performed mostly off-leash due their nature. For the static tasks, we expect dog being on-leash or off-leash not to have a significant effect, as there are no steps that could be considered. We found that FitBark differentiated between all other tasks except lying from sitting − in other words, all dynamic tasks could be differentiated, whether the dog was on or off leash. However, in our study, we did not examine the different steps performed by dogs but we adopted a more holistic approach, categorizing dog behavior through ethograms.
We also sought to compare the effect of the device placement to the accuracy of activity tracking. Activity scores of the ActiGraph GT9X Link on the neck were higher than those of that on the back, similarly as found by in, e.g. goats [Citation29]. Furthermore, both placements allowed the same behavioral differentiation, except for walking and sniffing, for which the activity scores did not differ if the device was placed on the back. Generally, the classification accuracy was similar or slightly lower for the neck than for the back. Besides, the agreement between predicted and observed behaviors was also lower for the neck placement. Altogether, our results indicate a slightly poorer behavioral differentiation performance of the ActiGraph GT9X Link when placed on the neck compared to the back. However, keeping the three devices in the same collar and using different attachment methods for both placements might have affected the reliability; even if the devices, the collar and the harness were tightened as much as possible and a second collar was used for the leash, following the recommendations of Martin et al. [Citation42].
Previous findings show that dog’s individual signalment might have an effect on the activity scores [Citation11,Citation17,Citation31–36]; therefore, we also tested whether the dogs’ age, weight or sex affected the obtained activity scores. In our study, the results of the devices were comparable between dogs regardless of dogs’ signalment, as in previous literature [Citation31] for most behaviors. Here, the dogs performed semi-controlled tasks for the same amount of time, whereas the studies that found links between signalment and activity scores measured dogs’ activity in less controlled setups, such as daily life physical activity [Citation11,Citation17,Citation32–36] or trotting up and down stairs [Citation31]. In the conditions of the previous studies, older dogs were not as active as young adults [Citation31–34] and heavier or overweight dogs were less active [Citation17,Citation31,Citation35,Citation36]. Generally, all the devices included in this study measured the dog movements similarly, so the possible activity differences found in previous studies [Citation11,Citation17,Citation31–36] likely reflect individual differences in the dogs’ general activity levels. The lack of signalment effect in our study may be due to the experiment setup: tight human control that the dogs actually performed the tasks as intended. Additionally, we only had medium- to large-sized dogs in the current study, thus it may be that the inclusion of small dogs might have affected the signalment results.
Our study has several limitations that should be taken into account. Here, we wished to obtain comparable information from middle- to large-sized, adult dogs. Thus, the full range of the possible sizes of dogs is not well represented, and as dog weight may affect the activity scores [Citation17,Citation31,Citation35,Citation36], the current data may not fit to dogs under 13 kg or over 41 kg. Likewise, puppies or elderly dogs are not included in our sample, as the ages of our participant dogs varied from 1 to 9 years. Here, we did not find the effect of age, weight, sex or neutering status on the short-term activity scores, possibly due to the sample limitation as intended. Nevertheless, as activity scores may vary with age [Citation31–34], the correspondence of the current results should be confirmed separately for dogs outside our study sample. The possible effect of the differences in dog age, weight and sex on the activity scores of different devices could be further studied with a more representative sample with a larger variation. Furthermore, this evaluation only concerned seven different tasks that could be performed for a prolonged period of time; of course, natural dog behavior is more variable, but our evaluation cannot be extended for other tasks or behaviors on the basis of the current work. Additionally, other tasks except for playing and sniffing were conducted while the dogs were on leash and led by a human handler. This was due to our aim to obtain seven clearly different kinds of tasks that were behaviorally verified, and this is quite difficult to obtain for a sufficient time and for video-verified behavior without human control. Although the leash was not attached to the same collar as the measurement devices, we cannot rule out the effect of human control to the dog behavior. The human handler may at least affect the dog performance, movement and speed, and consequently also the activity scores obtained, thus obtaining similar, behavior-verified data from freely moving dogs would be important in the future.
To conclude, the measurements of ActiGraph GT9X placed on the back and on the neck, FitBark and Kaunila correlated statistically significantly with each other, showing reliability of the devices. In general, median activity scores of the devices differentiated between the still and dynamic behaviors and allowed a classification of four to six behavioral categories depending on the placement and the device used. Mean activity scores were unrelated to age, weight and sex of dogs in this study. Thus, the results provide evidence for the devices being comparable between dogs in controlled conditions and the possibilities of these devices to classify the behaviors performed with a moderate reliability.
Acknowledgements
We would like to thank Heli Hyytiäinen for her kind advice in planning the study setup and Kyllikki Aakko for offering the sewing machine for preparing dog’s harnesses. Additionally, we are especially grateful for the other members of Turre ja Toivoset 2.0 project for their valuable suggestions for this experiment.
Disclosure statement
No potential conflict of interest was reported by the author(s).
Author contributions
Conceptualization: MVK, AVC, SS, HT, HV, PI, PM, VS, OV, AV; Data curation: AVC, SS, AV; Formal Analysis: AVC, SS, AV; Funding acquisition: MVK, PI, PM, VS, OV, AV; Investigation: AVC, SS, HT, LI, AK, AM; Methodology: AVC, SS, AV; Project administration: MVK, SS; Resources: AV, OV; Visualization: MVK, AVC, SS; Writing – original draft: MVK, AVC, SS and Writing – review and editing: MVK, AVC, SS, HT, LI, AK, AM, HV, PI, PM, VS, OV, AV.
Additional information
Funding
Notes on contributors
Miiamaaria V. Kujala
Miiamaaria V. Kujala is an Academy Researcher at the Jyväskylä Centre for Interdisciplinary Brain Research and Department of Psychology, Faculty of Education and Psychology, University of Jyväskylä; and Adjunct professor (docent) of Comparative Cognitive Neuroscience in the Faculty of Veterinary Medicine, University of Helsinki. She is interested in the physiological and neuroscientific basis of social interaction in both human and nonhuman animals, and the methodological development in the field comparative cognition.
Anna Valldeoriola Cardó
Anna Valldeoriola Cardó is a veterinarian and a computer scientist. She is currently working on a Finnish health technology company specialized in cardiological software solutions.
Sanni Somppi
Sanni Somppi is a project researcher at the Department of Psychology, Faculty of Education and Psychology, University of Jyväskylä. She has pioneered in canine cognition research combining behavioral studies with biometric methods such as eye tracking, heart rate variability, activity tracking and electroencephalography at the University of Helsinki.
Heini Törnqvist
Heini Törnqvist is a post-doctoral researcher at the Department of Psychology, Faculty of Education and Psychology, University of Jyväskylä. She received a PhD degree in 2020 in the comparative perspective on canine object perception, studied with noninvasive electroencephalography and eye gaze tracking.
Leena Inkilä
Leena Inkilä is a Licentiate of Veterinary Medicine and a doctoral researcher in the Doctoral Programme in Clinical Veterinary Medicine, University of Helsinki. Her current interests are in the health care of the competition-level agility dogs, e.g. training, management and risk factors for injuries.
Aija Koskela
Aija Koskela is a biologist and a doctoral researcher in the Doctoral Programme in Clinical Veterinary Medicine, University of Helsinki; and a project researcher at the Department of Psychology, Faculty of Education and Psychology, University of Jyväskylä.
Anne Myller
Anne Myller is a veterinarian, holding double academic degrees: Licentiate of Veterinary Medicine from the Faculty of Veterinary Medicine, University of Helsinki, and Master of Science in Technology from the Aalto University School of Science. She is particularly focused in the management of challenging behaviors and chronic pain in small animals, and also has a professional qualification as an animal trainer.
Heli Väätäjä
Heli Väätäjä is a Principal Lecturer at Lapland University of Applied Sciences, Finland. In addition to human−technology interaction related research, she studies animal−computer interaction as well as technological solutions for welfare and emotions of animals.
Poika Isokoski
Poika Isokoski is a CHI researcher at Tampere University in Finland. He defended his doctoral thesis in 2004 on text entry methods. Later he had worked on eye tracking as an input method, scents in virtual reality and also on dog technology. His approach is to build software and hardware prototypes to evaluate different human−machine or animal−machine interaction designs.
Päivi Majaranta
Päivi Majaranta is a Senior Research Fellow at the Faculty of Information Technology and Communication Sciences, Tampere University. She received her PhD in Interactive Technology in 2009. She is interested in human−technology interaction, with special expertise on the applied use of eye tracking in interfaces.
Veikko Surakka
Veikko Surakka is a Professor of Interactive Technology at the Faculty of Information Technology and Communication Sciences, Tampere University. He is the head of the Research Group for Emotions, Sociality, and Computing https://research.tuni.fi/esc/. The group develops and studies new methods for human−technology interaction.
Outi Vainio
Outi Vainio is a Professor Emerita of Veterinary Pharmacology at the Department of Equine and Small Animal Medicine, Faculty of Veterinary Medicine, University of Helsinki. She is specializes in pharmacological alleviation of animal pain, and during her whole career, she has conducted widely acknowledged work in promoting animal welfare.
Antti Vehkaoja
Antti Vehkaoja is an Associate Professor of Sensor Technology and Biomeasurements at the Faculty of Medicine and Health Technology, Tampere University. He received his D.Sc. (Tech.) degree in automation science and engineering and obtained a title of docent in methods for physiological monitoring from Tampere University of Technology, Tampere, Finland in 2015 and 2017, respectively. His research interests include the development of photoplethysmography sensors and other wearable embedded measurement technologies for physiological measurements and related signal processing and data analysis methods.
References
- Owens A, Vinkemeier D, Elsheikha H. A review of applications of artificial intelligence in veterinary medicine. Companion Anim. 2023;28:78–85. doi:10.12968/coan.2022.0028a
- Zanello G, Srinivasan CS, Nkegbe P. Piloting the use of accelerometry devices to capture energy expenditure in agricultural and rural livelihoods: protocols and findings from northern Ghana. Dev Eng. 2017;2:114–131. doi:10.1016/j.deveng.2017.10.001
- Nathan R, Spiegel O, Fortmann-Roe S, et al. Using tri-axial acceleration data to identify behavioral modes of free-ranging animals: general concepts and tools illustrated for griffon vultures. J Exp Biol. 2012;215:986–996. doi:10.1242/jeb.058602
- Dalton AJ, Rosen DA, Trites AW. Season and time of day affect the ability of accelerometry and the doubly labeled water methods to measure energy expenditure in northern fur seals (Callorhinus ursinus). J Exp Mar Biol Ecol. 2014;452:125–136. doi:10.1016/j.jembe.2013.12.014
- Zamansky A, van der Linden D, Hadar I, et al. Log my dog: perceived impact of dog activity tracking. Computer. 2019;52:35–43. doi:10.1109/MC.2018.2889637
- Väätäjä H, Majaranta P, Isokoski P, et al. Happy dogs and happy owners: using dog activity monitoring technology in everyday life. Proceedings of the Fifth International Conference on Animal-Computer Interaction [Internet]. New York, NY, USA: Association for Computing Machinery; 2018. doi:10.1145/3295598.3295607.
- Elliott KH. Measurement of flying and diving metabolic rate in wild animals: review and recommendations. Comp Biochem Physiol A Mol Integr Physiol. 2016;202:63–77. doi:10.1016/j.cbpa.2016.05.025
- Halsey LG, Shepard EL, Wilson RP. Assessing the development and application of the accelerometry technique for estimating energy expenditure. Comp Biochem Physiol A Mol Integr Physiol. 2011;158:305–314. doi:10.1016/j.cbpa.2010.09.002
- Campbell HA, Gao L, Bidder OR, et al. Creating a behavioural classification module for acceleration data: using a captive surrogate for difficult to observe species. J Exp Biol. 2013;216:4501–4506.
- Chapa JM, Maschat K, Iwersen M, et al. Accelerometer systems as tools for health and welfare assessment in cattle and pigs – a review. Behav Processes. 2020;181:104262. doi:10.1016/j.beproc.2020.104262
- Brown DC, Boston RC, Farrar JT. Use of an activity monitor to detect response to treatment in dogs with osteoarthritis. J Am Vet Med Assoc. 2010;237:66–70. doi:10.2460/javma.237.1.66
- Rhodin M, Bergh A, Gustås P, et al. Inertial sensor-based system for lameness detection in trotting dogs with induced lameness. Vet J. 2017;222:54–59. doi:10.1016/j.tvjl.2017.02.004
- Helm J, McBrearty A, Fontaine S, et al. Use of accelerometry to investigate physical activity in dogs receiving chemotherapy. J Small Anim Pract. 2016;57:600–609. doi:10.1111/jsap.12587
- Clarke N, Fraser D. Automated monitoring of resting in dogs. Appl Anim Behav Sci. 2016;174:99–102. doi:10.1016/j.applanim.2015.11.019
- Westgarth C, Ladha C. Evaluation of an open source method for calculating physical activity in dogs from harness and collar based sensors. BMC Vet Res. 2017;13:1–7. doi:10.1186/s12917-017-1228-8
- Yam P, Penpraze V, Young D, et al. Validity, practical utility and reliability of actigraph accelerometry for the measurement of habitual physical activity in dogs. J Small Anim Pract. 2011;52:86–91. doi:10.1111/j.1748-5827.2010.01025.x
- Morrison R, Penpraze V, Beber A, et al. Associations between obesity and physical activity in dogs: a preliminary investigation. J Small Anim Pract. 2013;54:570–574. doi:10.1111/jsap.12142
- Hansen BD, Lascelles BDX, Keene BW, et al. Evaluation of an accelerometer for at-home monitoring of spontaneous activity in dogs. Am J Vet Res. 2007;68:468–475. doi:10.2460/ajvr.68.5.468
- Olsen AM, Evans RB, Duerr FM. Evaluation of accelerometer inter-device variability and collar placement in dogs. Vet Evid. 2016;1:1–9. doi:10.18849/ve.v1i2.40
- den Uijl I, Gómez Álvarez CB, Bartram D, et al. External validation of a collar-mounted triaxial accelerometer for second-by-second monitoring of eight behavioural states in dogs. Plos One. 2017;12:e0188481. doi:10.1371/journal.pone.0188481
- Yashari JM, Duncan CG, Duerr FM. Evaluation of a novel canine activity monitor for at-home physical activity analysis. BMC Vet Res. 2015;11:1–7. doi:10.1186/s12917-015-0457-y
- Belda B, Enomoto M, Case B, et al. Initial evaluation of PetPace activity monitor. Vet J. 2018;237:63–68. doi:10.1016/j.tvjl.2018.05.011
- Colpoys J, DeCock D. Evaluation of the FitBark activity monitor for measuring physical activity in dogs. Animals (Basel). 2021;11:781. doi:10.3390/ani11030781
- Venkatraman S, Long JD, Pister KSJ, et al. Wireless inertial sensors for monitoring animal behavior. 29th Annual International Conference of the IEEE Engineering in Medicine and Biology Society, Lyon, France; 2007. doi:10.1109/IEMBS.2007.4352303
- Brugarolas R, Loftin RT, Yang P, et al. Behavior recognition based on machine learning algorithms for a wireless canine machine interface. Silver Spring (MD): IEEE; 2013. p. 1–5.
- Ladha C, Hammerla N, Hughes E, et al. Dog's life: wearable activity recognition for dogs. UbiComp '13: Proceedings of the 2013 ACM international joint conference on Pervasive and ubiquitous computing. 2013: 415–418. doi: 10.1145/2493432.2493519
- Griffies JD, Zutty J, Sarzen M, et al. Wearable sensor shown to specifically quantify pruritic behaviors in dogs. BMC Vet Res. 2018;14:124. doi:10.1186/s12917-018-1428-x
- Ladha C, Hoffman CL. A combined approach to predicting rest in dogs using accelerometers. Sensors. 2018;18:2649. doi:10.3390/s18082649
- Moreau M, Siebert S, Buerkert A, et al. Use of a tri-axial accelerometer for automated recording and classification of goats’ grazing behaviour. Appl Anim Behav Sci. 2009;119:158–170. doi:10.1016/j.applanim.2009.04.008
- Boerema ST, Van Velsen L, Schaake L, et al. Optimal sensor placement for measuring physical activity with a 3D accelerometer. Sensors. 2014;14:3188–3206. doi:10.3390/s140203188
- Brown DC, Michel KE, Love M, et al. Evaluation of the effect of signalment and body conformation on activity monitoring in companion dogs. Am J Vet Res. 2010;71:322–325. doi:10.2460/ajvr.71.3.322
- Michel KE, Brown DC. Association of signalment parameters with activity of pet dogs. J Nutr Sci. 2014;3:e28. doi:10.1017/jns.2014.49
- Morrison R, Penpraze V, Greening R, et al. Correlates of objectively measured physical activity in dogs. Vet J. 2014;199:263–267. doi:10.1016/j.tvjl.2013.11.023
- Michel KE, Brown DC. Determination and application of cut points for accelerometer-based activity counts of activities with differing intensity in pet dogs. Am J Vet Res. 2011;72:866–870. doi:10.2460/ajvr.72.7.866
- Jones S, Dowling-Guyer S, Patronek GJ, et al. Use of accelerometers to measure stress levels in shelter dogs. J Appl Anim Welf Sci. 2014;17:18–28. doi:10.1080/10888705.2014.856241
- Knazovicky D, Tomas A, Motsinger-Reif A, et al. Initial evaluation of nighttime restlessness in a naturally occurring canine model of osteoarthritis pain. PeerJ. 2015;3:e772. doi:10.7717/peerj.772
- Morrison R, Sutton D, Ramsoy C, et al. Validity and practical utility of accelerometry for the measurement of in-hand physical activity in horses. BMC Vet Res. 2015;11:1–8. doi:10.1186/s12917-015-0550-2
- Nakas CT, Dalrymple-Alford JC, Anderson TJ, et al. Generalization of Youden index for multiple-class classification problems applied to the assessment of externally validated cognition in Parkinson disease screening. Stat Med. 2013;32:995–1003. doi:10.1002/sim.5592
- Greiner M, Pfeiffer D, Smith RD. Principles and practical application of the receiver-operating characteristic analysis for diagnostic tests. Prev Vet Med. 2000;45:23–41. doi:10.1016/S0167-5877(00)00115-X
- Altman DG. Practical statistics for medical research. New York: CRC press; 1990.
- Yamada M, Tokuriki M. Spontaneous activities measured continuously by an accelerometer in beagle dogs housed in a cage. J Vet Med Sci. 2000;62:443–447. doi:10.1292/jvms.62.443
- Martin KW, Olsen AM, Duncan CG, et al. The method of attachment influences accelerometer-based activity data in dogs. BMC Vet Res. 2016;13:1–6. doi:10.1186/s12917-017-0971-1