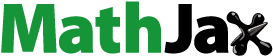
Abstract
Background
In the Danish Head and Neck Cancer Group (DAHANCA) 35 trial, patients are selected for proton treatment based on simulated reductions of Normal Tissue Complication Probability (NTCP) for proton compared to photon treatment at the referring departments. After inclusion in the trial, immobilization, scanning, contouring and planning are repeated at the national proton centre. The new contours could result in reduced expected NTCP gain of the proton plan, resulting in a loss of validity in the selection process. The present study evaluates if contour consistency can be improved by having access to AI (Artificial Intelligence) based contours.
Materials and Methods
The 63 patients in the DAHANCA 35 pilot trial had a CT from the local DAHANCA centre and one from the proton centre. A nationally validated convolutional neural network, based on nnU-Net, was used to contour OARs on both scans for each patient. Using deformable image registration, local AI and oncologist contours were transferred to the proton centre scans for comparison. Consistency was calculated with the Dice Similarity Coefficient (DSC) and Mean Surface Distance (MSD), comparing contours from AI to AI and oncologist to oncologist, respectively. Two NTCP models were applied to calculate NTCP for xerostomia and dysphagia.
Results
The AI contours showed significantly better consistency than the contours by oncologists. The median and interquartile range of DSC was 0.85 [0.78 − 0.90] and 0.68 [0.51 − 0.80] for AI and oncologist contours, respectively. The median and interquartile range of MSD was 0.9 mm [0.7 − 1.1] mm and 1.9 mm [1.5 − 2.6] mm for AI and oncologist contours, respectively. There was no significant difference in NTCP.
Conclusions
The study showed that OAR contours made by the AI algorithm were more consistent than those made by oncologists. No significant impact on the NTCP calculations could be discerned.
Background
Modern radiation treatment is complex and involves many manual and time-consuming procedures. Even with national and international guidelines, contouring of cancer targets and organs at risk (OARs) in head and neck (H&N) cancer patients varies between treatment centres and clinical experts [Citation1–3]. Variability in the contouring of OARs has a significant dosimetric impact on patient treatment [Citation2,Citation4] and may influence the results of clinical trials [Citation5].
In the Danish Head and Neck Cancer Group (DAHANCA) 35 trial (NCT04607694), H&N cancer patients are selected for proton treatment based on the simulated benefit in terms of Normal Tissue Complication Probability (NTCP) for xerostomia and dysphagia [Citation6]. Contouring of OARs is part of the basis for treatment planning and, consequently, for the NTCP estimations. Thus, the accuracy and consistency of the OAR contours potentially affect the patient selection process for the DAHANCA 35 trial.
To test the feasibility and safety of proton treatment, the DAHANCA 35 pilot trial (NCT05423704) was conducted [Citation7]. Patients selected for the DAHANCA 35 pilot trial received proton treatment, whereas the patients selected for the DAHANCA 35 trial will be randomised for proton or photon treatment [Citation6]. Results from the DAHANCA 35 pilot trial showed that the NTCP estimates, based on contours and treatment plans from different treatment centres, had variations that could be related to target contouring. There were also indications that the variation in OAR contours could play an important role in the robustness of patient selection [Citation7].
Artificial Intelligence (AI) has been investigated as a useful tool in radiotherapy [Citation8,Citation9]. Previous studies have showed improved efficiency and standardisation of treatment by implementing AI algorithms for auto-segmentation of OARs on computed tomography (CT) scans in H&N cancer treatment [Citation10,Citation11]. AI has also shown good performance in contouring OARs compared to oncologist contours as ground truth [Citation12,Citation13]. It is therefore hypothesised that AI segmentation of OARs could improve the patient selection robustness for the DAHANCA 35 trial.
This study aimed to quantify the consistency and variation in the contouring by oncologists of relevant OARs on two different CT scans for the same patient. This was compared to the consistency in contours performed by an AI segmentation algorithm on the same CT scans. Secondly, the impact on estimated NTCP using AI contours of OARs compared to contours made by oncologists was assessed to evaluate the clinical relevance of the difference in contouring consistency between AI and oncologists.
Materials and methods
From May 2019 to March 2021, 63 patients were included in the DAHANCA 35 pilot trial [Citation6,Citation7]. Each patient was diagnosed with squamous cell carcinoma of the pharynx or larynx at a local DAHANCA centre. Before potential inclusion in the trial, the following was performed at the local centre: a CT scan (local CT scan), contouring of the target volumes and OARs by radiation oncologists (local oncologist contours), as well as treatment planning for both photon treatment (local photon plan) and proton treatment (local proton plan).
Two NTCP models, one for xerostomia grade 2+ and one for dysphagia grade 2+, validated in a Danish cohort [Citation14], were used to estimate the NTCP [Citation15] for the local photon and proton treatment plans. If the difference in estimated NTCP (NTCP) for the local photon plan compared to the local proton plan was larger than 5%-point for either xerostomia, dysphagia or both, the patient was offered inclusion in the trial and, on informed consent, referred to the national proton centre for proton treatment.
At the national proton centre, a new proton therapy compatible immobilisation mask and a new CT scan were made (clinical CT scan), as well as new contours (clinical oncologist contours) and a new proton treatment plan (clinical proton plan).
The present study is a retrospective analysis of contouring consistency and NTCP, and thus does not influence the course of treatment of the patients included in the DAHANCA 35 pilot trial.
Artificial intelligence segmentation model
The AI model used for segmentation was a Convolutional Neural Network (CNN) based on the nnU-Net model presented by Isensee et al. [Citation16]. The AI model was trained on CT scans from a national trial [Citation14] and relevant contours of OARs in H&N cancer recontoured by radiation oncologists following international standards [Citation17]. The model performance was validated on OARs contoured by H&N oncology specialists from a Danish national workshop [Citation18]. The model was used to retrospectively contour 12 OARs relevant for H&N cancer on both local and clinical CT scans for all patients from the DAHANCA 35 pilot trial (local AI contours and clinical AI contours, respectively). The OARs available from the model were: extended oral cavity, upper-, middle-, and lower constrictor muscles, glottic larynx, supraglottic larynx, left and right parotid, left and right submandibular, thyroid, and oesophagus.
Data analyses
The data analyses were performed on data from the 63 patients in the pilot trial. Local AI contours on local CT scans were compared to clinical AI contours on clinical CT scans, and local oncologist contours on local CT scans were compared to clinical oncologist contours on clinical CT scans.
Data pre-processing and statistical analyses were performed in MATLAB R2022b.
Data pre-processing
One patient was missing the contour of the left submandibular on one of the CT scans, and another patient was missing contours of both the left and right submandibular. Thus, contours from 61 patients were used for the statistical comparison of the left submandibular and 62 for the right submandibular. Contours from all 63 patients were used for the remaining 10 OARs.
Using MIM software, Deformable Image Registration (DIR) was performed [Citation19], transferring local AI and oncologist contours to the clinical CT scan, transferring both sets of contours using the same DIR for each patient. The DIR process in MIM first uses a rigid registration, then a coarse-to-fine multi-resolution approach, and finally, a custom-modified gradient descent for optimisation [Citation20]. The result of the DIR was accepted based on visual inspection of the deform fusion alignment in MIM.
Contour overlap
Contouring consistency was measured by contour overlap in terms of Dice Similarity Coefficient (DSC) [Citation21,Citation22] and Mean Surface Distance (MSD) [Citation23]. The higher the DSC and the lower the MSD for a contour comparison, the better the consistency.
The oncologists contour oesophagus in the caudal direction until it is no longer deemed clinically relevant, concerning the radiotherapy treatment plan. However, as the dose tolerance is the mean dose, it can influence the plan. The oesophagus contours were analysed as directly contoured and after correcting the contours to have the same caudal length, i.e., when comparing two contours of oesophagus, the contour with the caudal part, most cranial determined the caudal length, and slices below that point were removed for the other contour.
Normal tissue complication probability
NTCP is the foundation for patient selection in the DAHANCA 35 trial, and preliminary results from the pilot trial showed a disparity in NTCP when comparing the local
NTCP (
) to the clinical
NTCP (
) [Citation7]. NTCP for xerostomia grade 2+ and dysphagia grade 2+ was estimated using the Dutch models for selection [Citation15]. The model for xerostomia included baseline xerostomia and mean dose to the contralateral parotid [Citation24], the model for dysphagia included baseline dysphagia and mean dose to the upper pharyngeal constrictor muscle and extended oral cavity (regression coefficients in Supplementary Table 1).
In the present study, NTCP was calculated based on the original contours made by oncologists and the corresponding photon and proton plans, as described by Hansen et al. [Citation7]. Additionally, NTCP was calculated based on the AI contours on the original photon and proton plans. The treatment plans were not optimised for the AI contours, as these were delineated retrospectively after patient treatment.
The disparity in local and clinical NTCP was visualized using a scatterplot and the variation using a Bland-Altman plot.
Statistical analyses
Wilcoxon Signed Rank test for non-parametric data was used to compare the consistency and NTCP between AI and oncologist contours for each patient, using a significance level of 5%.
Results
Contour overlap
In terms of DSC and MSD, the AI contours showed significantly better consistency than the contours by the oncologists. The median and interquartile range of DSC across all 12 OARs were 0.85 [0.78 − 0.90] and 0.68 [0.51 − 0.80] for AI and oncologist contours, respectively. The median and interquartile range of MSD for all OARs were 0.9 mm [0.7 − 1.1] mm and 1.9 mm [1.5 − 2.6] mm for AI and oncologist contours, respectively. The DSC and MSD for the individual OARs are collected in . Comparing the DSC between AI and oncologists for each OAR, the DSC was significantly larger for AI contours. All p-values were significant (p). The MSD for all OARS was significantly lower for AI contours compared to oncologist contours. All p-values were significant (p
). As seen in , the AI contours of oesophagus were still significantly more consistent than oncologist contours after correction to have the same caudal length (p
).
Table 1. DSC and MSD for 12 OARs contoured by AI oncologists, respectively.
shows the DSC represented as box plots with raw data points overlaid for all 12 OARs, and shows the MSD. Supplementary Figures 1 and 2 show the DSC and MSD, respectively, for the oesophagus with and without correction to have the same caudal length. When correcting for the same length, the median DSC and MSD match the other OARs.
Figure 1. Box plot with individual samples overlaid showing the DSC for the 12 OARs. Green boxes and samples show the DSC for the AI contours, and blue boxes and samples are results comparing oncologist contours. The raw data points are shown to visualise the distribution.
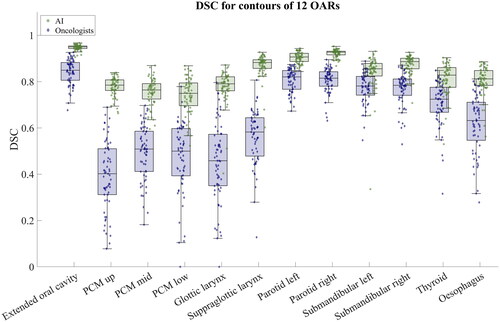
Figure 2. Box plot with individual samples overlaid showing the MSD for the 12 OARs. Green boxes and samples show the MSD for the AI contours, and blue boxes and samples are results comparing oncologist contours. The raw data points are shown to visualise the distribution. For visualisation, the plot has been scaled, omitting two outliers from oesophagus and one from glottic larynx for oncologist contours.
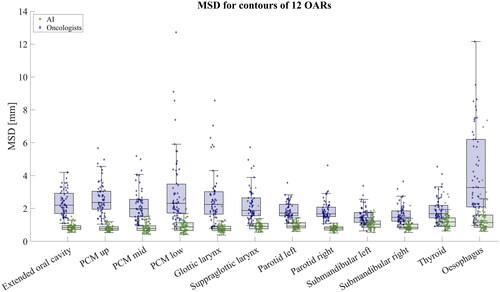
Normal Tissue Complication Probability
Considering the local NTCP compared to the clinical
NTCP, no significant difference was found between the ΔNTCP calculated based on AI contours and ΔNTCP calculated on oncologist contours.
shows a scatter plot with the local NTCP on the x-axis and the clinical
NTCP on the y-axis. The
NTCP is shown in %-point. The dotted black line shows the identity line. If there was no difference between the local and clinical
NTCP, all samples would be on the dashed identity line.
Figure 3. Scatter plot of the local ΔNTCP () and clinical ΔNTCP (
) based on AI (green data points) and oncologist (blue data points) contours, respectively, for xerostomia and dysphagia.
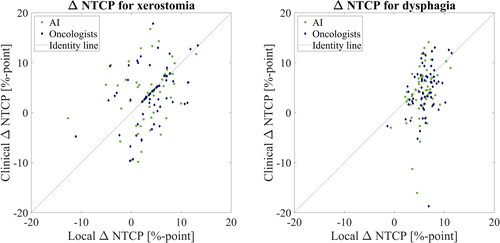
shows a Bland-Altman plot of the mean between the local and clinical NTCP on the x-axis and the difference between the local and clinical
NTCP on the y-axis. The
NTCP is shown in %-point.
Figure 4. Bland-Altman plot showing the mean and difference between the local and clinical NTCP for xerostomia and dysphagia. The green data points represent the
NTCP based on AI contours, and the blue data points represent the
NTCP calculated based on oncologist contours.
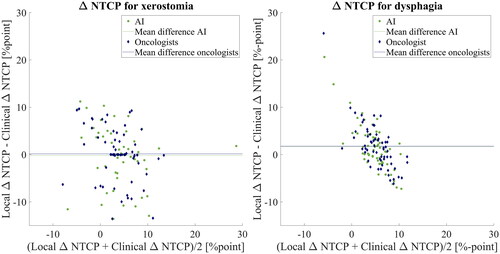
For the model used in the DAHANCA 35 pilot trial, the median difference and interquartile range in NTCP for xerostomia were 0%-point [−2–3] %-points for AI and 0%-point [−1–4] %-points for oncologists. The p-value was not significant (p
). For dysphagia, the median difference in
NTCP was 1%-point [−1–4] for AI and 1%-point [−1–4] for oncologists. The p-value was not significant (p
).
Discussion
The results of the present study showed that contours generated by the AI segmentation algorithm were significantly more consistent than contours made by oncologists. The AI contours investigated in the present study are not adjusted by oncologists, which they would be if used for patient treatment. Furthermore, different centres might have slightly different procedures when working with AI contours. This could alter the consistency between contours, but it would presumably still be more harmonised, as all oncologists would use a more consistent starting point. Implementing an AI segmentation algorithm, with consistency as shown in this study, would therefore introduce less inter-observer variability in a clinical trial, assuming that the post-correction done by oncologists would be limited.
The contouring consistency was lower for oncologists than for AI across all OARs; however, the consistency for oncologists was highest in the OARs that have the longest history of guidelines and where the oncologist interpretation has been discussed over the years (i.e., extended oral cavity, left and right parotid, left and right submandibular, and thyroid) [Citation25–27]. This is especially evident in , showing the DSC. The consistency for contours by oncologists was lower for OARs implemented in the guidelines most recently, like the glottic larynx, supraglottic larynx, and constrictor muscles.
The variation in consistency in the oesophagus contouring mainly depends on the length of the contoured organ as determined by the oncologist. The AI contours were still significantly more consistent after correcting the oesophagus contours to have the same caudal length. The corrected contours might give a more fair comparison, as the length of oesophagus outside of what is clinically relevant is less important. The AI contours of oesophagus without correction were also significantly more consistent than the oncologist contours after correction; thus, using these would give a more representative mean dose.
The contrast on CT scans differs for different OARs, requiring an individual oncologist’s specific interpretation of anatomy. This could explain some of the lack of consistency for oncologists, as some OARs might be more difficult to distinguish. Here the AI algorithm places a typical segmentation that matches the patient in shape and size. The algorithm works in 3D, whereas the oncologist works in 2D in the three different planes, which again could explain why the AI contours are more consistent.
The lower consistency in contours made by oncologists supports the statement that even with national and international guidelines [Citation17,Citation27], there is a gap between what has been generally accepted and what is practically performed at different treatment centres [Citation28]. In Denmark, every treatment centre adheres to the same guidelines [Citation27], but even then, this study indicates that the interpretation and execution differ across the country. Implementing AI for contouring could reduce the gap between guidelines, interpretation, and execution. Contouring at the national proton centre is always conducted using an MR scan performed in the treatment position in addition to the planning CT scan. Although it is recommended to use MR [Citation29], it is not always acquired at the local centres, and the use of MR for contouring OARs is not always used. This difference may explain some of the inconsistencies in contours between oncologists.
The results on consistency in this study are a combination of contouring consistency, DIR, and differences in procedures between local and clinical centres. The OARs were investigated in terms of volume change before and after DIR; for most OARs, the volume changed by approximately 10%. This could be because of the DIR process, but also differences in scanning procedures between local centres and the proton centre, where the local CT scans are always performed with contrast and the proton centre CT scans are without. However, each set of local oncologists and AI contours was transferred using the same DIR process, which means that the potential change due to the DIR process was applied to both. Therefore it was assumed not to alter the overall conclusion of the study.
A change in OAR contouring will affect the treatment planning, which in turn can affect estimations of NTCP [Citation2]. Brouwer et al. investigated the effect of differences in delineation on resulting NTCP estimations. They found little NTCP differences in the majority of patients and large NTCP differences of in a few patients [Citation2]. For a clinical trial like DAHANCA 35, utilising patient selection based on NTCP estimates, consistency in NTCP is important. Results from the DAHANCA 35 pilot trial, showed that for patients selected for specific toxicity, the mean
NTCP for xerostomia and dysphagia was significantly reduced from the local centre to the national proton centre [Citation7]. The mean local
NTCP for xerostomia was 7.3%-point, and the mean clinical
NTCP was 4.9%-point. For dysphagia, the mean local
NTCP was 6.9%-point, and the mean clinical
NTCP was 5.3%-point [Citation7]. The present study did not show significant differences in
NTCP between contours by oncologists and AI, potentially because the treatment plans were not optimised according to the AI contours. It would be expected that improving the contour consistency of OARs and target as well as optimising treatment plan quality, would result in more consistent NTCP estimates. The consistency of OAR contours could be improved by using AI, as suggested in this study. Even though DAHANCA guidelines have already improved the contouring consistency of clinical target volumes [Citation3], it could be further enhanced by implementing AI for segmentation of target volumes as a starting point for oncologists [Citation30]. Furthermore, optimising treatment plan quality to spare OARs could be done using automated and knowledge-based treatment planning tools [Citation31–33]. The field of AI continues to develop, and better segmentation models will likely be developed for contouring for both OARs and cancer targets, thus improving consistency between treatment centres. Similarly, dose prediction AI algorithms [Citation34–36] will potentially help improve NTCP estimates. The dose distribution can be predicted without simulating the full complex photon or proton plan, presumably increasing the consistency.
The results of this study do not indicate whether contours made by AI or oncologists are more correct, only that AI contours are more consistent for the same patient. Before implementing an AI model for the segmentation of OARs, it should be investigated if the AI model performs to a clinically acceptable standard. This was not investigated in this study; however, the current AI model performance was investigated in a study by Lorenzen et al. [Citation37], who found that it performed as well as, or better than, the expert oncologists for almost all OARs investigated here. An exception was the upper pharyngeal constrictor muscle, where the model was trained on segmentation from a vague definition of the upper pharyngeal constrictor muscle. For this reason, the AI model is being updated.
Higher consistency in contouring would contribute to increasing the chance of more consistent treatment planning across treatment centres, influencing NTCP estimates. In combination with improved target contouring, it would thus potentially result in improved patient selection for the trial, potentially improving the overall outcome of the trial. In general, AI OAR segmentation could provide a common starting point which, in the long run, could lead to harmonised treatment procedures and improve the local selection of patients for appropriate treatment, independent of local expertise and workload, and hence improve equality in health care.
This study investigated the consistency in contouring when using AI, but AI may also be useful for quality assurance of clinical trials and clinical practice [Citation36]. Quality assurance could be implemented like in this study, where AI constitutes a second opinion to consider, or as a tool for decision support to form the basis for oncologist contouring. It could also be used directly for quality assurance of the AI-generated contours [Citation38].
In conclusion, AI is more consistent for segmentation of OARs in H&N cancer patients compared to oncologist contours. However, the more consistent contours did not translate into more consistent ΔNTCP estimates.
Supplemental Material
Download MS Word (122.7 KB)Disclosure statement
No potential conflict of interest was reported by the author(s).
Data availability statement
The data used in this study are part of a clinical trial and are not available.
Additional information
Funding
References
- Brouwer CL, Steenbakkers RJHM, van den Heuvel E, et al. 3D variation in delineation of head and neck organs at risk. Radiat Oncol. 2012;7(1):32. doi: 10.1186/1748-717X-7-32.
- Brouwer CL, Steenbakkers RJ, Gort E, et al. Differences in delineation guidelines for head and neck cancer result in inconsistent reported dose and corresponding NTCP. Radiother Oncol. 2014;111(1):148–152. doi: 10.1016/j.radonc.2014.01.019.
- Hansen CR, Johansen J, Samsøe E, et al. Consequences of introducing geometric GTV to CTV margin expansion in DAHANCA contouring guidelines for head and neck radiotherapy. Radiother Oncol. 2018;126(1):43–47. doi: 10.1016/j.radonc.2017.09.019.
- Voet PW, Dirkx ML, Teguh DN, et al. Does atlas-based autosegmentation of neck levels require subsequent manual contour editing to avoid risk of severe target underdosage? A dosimetric analysis. Radiother Oncol. 2011;98(3):373–377. doi: 10.1016/j.radonc.2010.11.017.
- Peters LJ, O'Sullivan B, Giralt J, et al. Critical impact of radiotherapy protocol compliance and quality in the treatment of advanced head and neck cancer: results from TROG 02.02. J Clin Oncol. 2010;28(18):2996–3001. doi: 10.1200/JCO.2009.27.4498.
- Friborg J, Jensen K, Eriksen JG, et al. Considerations for study design in the DAHANCA 35 trial of protons versus photons for head and neck cancer. Radiother Oncol. 2023; Under review.
- Hansen CR, Jensen K, Smulders B, et al. Evaluation of decentralised model-based selection of head and neck cancer patients for a proton treatment study. DAHANCA 35. Radiother Oncol. 2023;109812. doi: 10.1016/j.radonc.2023.109812.
- Chufal KS, Ahmad I, Chowdhary RL. Artificial intelligence in radiation oncology: how far have we reached? IJMIO. 2023;8:9–14. doi: 10.25259/IJMIO_32_2022.
- Lim JY, Leech M. Use of auto-segmentation in the delineation of target volumes and organs at risk in head and neck. Acta Oncol. 2016;55(7):799–806. doi: 10.3109/0284186X.2016.1173723.
- van der Veen J, Willems S, Deschuymer S, et al. Benefits of deep learning for delineation of organs at risk in head and neck cancer. Radiother Oncol. 2019;138:68–74. doi: 10.1016/j.radonc.2019.05.010.
- Kosmin M, Ledsam J, Romera-Paredes B, et al. Rapid advances in auto-segmentation of organs at risk and target volumes in head and neck cancer. Radiother Oncol. 2019;135:130–140. doi: 10.1016/j.radonc.2019.03.004.
- Sartor H, Minarik D, Enqvist O, et al. Auto-segmentations by convolutional neural network in cervical and anorectal cancer with clinical structure sets as the ground truth. Clin Transl Radiat Oncol. 2020;25:37–45. doi: 10.1016/j.ctro.2020.09.004.
- Wong J, Fong A, McVicar N, et al. Comparing deep learning-based auto-segmentation of organs at risk and clinical target volumes to expert inter-observer variability in radiotherapy planning. Radiother Oncol. 2020;144:152–158. doi: 10.1016/j.radonc.2019.10.019.
- Hansen CR, Friborg J, Jensen K, et al. NTCP model validation method for DAHANCA patient selection of protons versus photons in head and neck cancer radiotherapy. Acta Oncol. 2019;58(10):1410–1415. doi: 10.1080/0284186X.2019.1654129.
- Langendijk JA, Lambin P, De Ruysscher D, et al. Selection of patients for radiotherapy with protons aiming at reduction of side effects: the model-based approach. Radiother Oncol. 2013;107(3):267–273. doi: 10.1016/j.radonc.2013.05.007.
- Isensee F, Jaeger PF, Kohl SAA, et al. nnU-Net: a self-configuring method for deep learning-based biomedical image segmentation. Nat Methods. 2021;18(2):203–211. doi: 10.1038/s41592-020-01008-z.
- Brouwer CL, Steenbakkers RJ, Bourhis J, et al. CT-based delineation of organs at risk in the head and neck region: DAHANCA, EORTC, GORTEC, HKNPCSG, NCIC CTG, NCRI, NRG oncology and TROG consensus guidelines. Radiother Oncol. 2015;117(1):83–90. doi: 10.1016/j.radonc.2015.07.041.
- Jensen K, Lorentzen E, Eriksen JG, et al. MO-0713 inter-expert observer variance of organs at risk according to the DAHANCA guidelines. ESTRO 2023 - Abstract Book 2023. 2023:596–597.
- Kristensen MH, Hansen CR, Zukauskaite R, et al. Co-registration of radiotherapy planning and recurrence scans with different imaging modalities in head and neck cancer. Phys Imaging Radiat Oncol. 2022;23:80–84. doi: 10.1016/j.phro.2022.06.012.
- Piper J, Nelson A, Harper J. Deformable image registration in MIM maestro® evaluation and description. MIM Software Inc. 2018 (White Paper).
- Dice LR. Measures of the amount of ecologic association between species. Ecology. 1945;26(3):297–302. doi: 10.2307/1932409.
- Sørensen T. A method of establishing groups of equal amplitude in plant sociology based on similarity of species and its application to analyses of the vegetation on Danish commons. Det Kongelige Danske Videnskabernes Selskab. 1948;5(4):1–34.
- Lorenzen EL, Kallehauge JF, Byskov CS, et al. A national study on the inter-observer variability in the delineation of organs at risk in the brain. Acta Oncol. 2021;60(11):1548–1554. doi: 10.1080/0284186X.2021.1975813.
- Beetz I, Schilstra C, van der Schaaf A, et al. NTCP models for patient-rated xerostomia and sticky saliva after treatment with intensity modulated radiotherapy for head and neck cancer: the role of dosimetric and clinical factors. Radiother Oncol. 2012;105(1):101–106. doi: 10.1016/j.radonc.2012.03.004.
- Hansen CR, Johansen J, Kristensen CA, et al. Quality assurance of radiation therapy for head and neck cancer patients treated in DAHANCA 10 randomized trial. Acta Oncol. 2015;54(9):1669–1673. doi: 10.3109/0284186X.2015.1063780.
- Overgaard J, Hoff CM, Hansen HS, et al. DAHANCA 10 - Effect of darbepoetin alfa and radiotherapy in the treatment of squamous cell carcinoma of the head and neck. A multicenter, open-label, randomized, phase 3 trial by the Danish head and neck cancer group. Radiother Oncol. 2018;127(1):12–19. doi: 10.1016/j.radonc.2018.02.018.
- Jensen K, Friborg J, Hansen CR, et al. The Danish head and neck cancer group (DAHANCA) 2020 radiotherapy guidelines. Radiother Oncol. 2020;151:149–151. doi: 10.1016/j.radonc.2020.07.037.
- van der Veen J, Gulyban A, Willems S, et al. Interobserver variability in organ at risk delineation in head and neck cancer. Radiat Oncol. 2021; 16(1):120. doi: 10.1186/s13014-020-01677-2.
- Jensen K, Al-Farra G, Dejanovic D, et al. Imaging for target delineation in head and neck cancer radiotherapy. Semin Nucl Med. 2021;51(1):59–67. doi: 10.1053/j.semnuclmed.2020.07.010.
- Wei Z, Ren J, Korreman SS, et al. Towards interactive deep-learning for tumour segmentation in head and neck cancer radiotherapy. Phys Imaging Radiat Oncol. 2023; 25:100408. 1/01/doi: 10.1016/j.phro.2022.12.005.
- Tol JP, Delaney AR, Dahele M, et al. Evaluation of a knowledge-based planning solution for head and neck cancer. Int J Radiat Oncol Biol Phys. 2015;91(3):612–620. doi: 10.1016/j.ijrobp.2014.11.014.
- Hansen CR, Bertelsen A, Hazell I, et al. Automatic treatment planning improves the clinical quality of head and neck cancer treatment plans. Clin Transl Radiat Oncol. 2016;1:2–8. doi: 10.1016/j.ctro.2016.08.001.
- Hussein M, Heijmen BJM, Verellen D, et al. Automation in intensity modulated radiotherapy treatment planning-a review of recent innovations. Br J Radiol. 2018;91(1092):20180270. doi: 10.1259/bjr.20180270.
- Gronberg MP, Beadle BM, Garden AS, et al. Deep learning-based dose prediction for automated, individualized quality assurance of head and neck radiation therapy plans. Pract Radiat Oncol. 2023;13(3):e282–e291. doi: 10.1016/j.prro.2022.12.003.
- Baroudi H, Brock KK, Cao W, et al. Automated contouring and planning in radiation therapy: what is 'clinically acceptable’? Diagnostics. 2023;13(4):667. doi: 10.3390/diagnostics13040667.
- Vandewinckele L, Claessens M, Dinkla A, et al. Overview of artificial intelligence-based applications in radiotherapy: recommendations for implementation and quality assurance. Radiother Oncol. 2020;153:55–66. doi: 10.1016/j.radonc.2020.09.008.
- Lorenzen EL, Zukauskaite R, Kyndt M, et al. OC-0118 first results on DAHANCA automatic segmentation algorithms of organs at risk. ESTRO 2023 - Abstract Book 2023. 2023:92–93.
- Luan S, Xue X, Wei C, et al. Machine learning-based quality assurance for automatic segmentation of head-and-neck organs-at-risk in radiotherapy. Technol Cancer Res Treat. 2023;22:15330338231157936–15330338231157936. doi: 10.1177/15330338231157936.