Introduction
In the last decade, proton radiation therapy for brain tumours has become increasingly the treatment of choice [Citation1–7]. The characteristics of a proton beam have an advantage over a photon beam, because a proton beam disposes most of its energy (Bragg peak) at a certain depth and no residual radiation will exist beyond this point [Citation8,Citation9]. The Danish Centre for Particle Therapy (DCPT) equipped with a ProBeam spot scanning beam from Varian Medical Systems™ (Palo Alto, CA) started treating patients in January 2019. Prior to the opening of the centre, the Danish Neuro-Oncology Group (DNOG) decided to implement selection criteria for adult patients with primary brain tumours with a good or intermediate prognosis. The selection criteria have been based on comparison treatment plans from the referring regional departments. In 2018, they published the dosimetric criteria for adult patients with brain tumour for potential proton radiation treatment () [Citation10]. At a national video conference, it is decided that there is an advantage for proton therapy according to the DNOG criteria. However, treatment planning for the modality comparison is time and resource consuming and it is dependent on local treatment planning skills. To ease and to speed this up, a knowledge-based tool for proton treatment planning can be used and it may in addition homogenise the selection quality across the centres. RapidPlan™ (Varian Medical Systems™, Palo Alto, CA) is available at the majority of radiation centres in Denmark. This tool predicts outcome of the dose-volume histograms (DVHs) for different OARs and sets automatically the optimising parameters used for achieving this predicted dose distribution based on a tumour site specific model.
Table 1. DNOG’s OAR’s dose sparing criteria compared to photons for potential proton treatment.
In the present study, doses to OARs relevant for the selection of patients for proton therapy treatment according to the DNOG-criteria predicted by RapidPlan™ were compared to the corresponding doses from the original clinical proton treatment plans or from the comparison proton treatment plan with the aim to assess the performance of RapidPlan™ in selection of patients with brain tumours for proton therapy.
Materials and methods
Patient cohort and delineation
To train the RapidPlan™ brain model, the treatment plans of 90 patients with astrocytoma, IDH mutated WHO grade 2/3, oligodendroglioma, IDH mutated, 1p19q co-deleted WHO grade 2/3, and meningioma WHO grade 1–3, treated at our institution from 2019 to 2020, were included. The target coverage of the clinical target volume (CTV) was robustly optimised. The prescribed dose to the CTV was depending on the diagnosis and varied between 50.4 GyRBE and 59.4 GyRBE assuming a uniform RBE of 1.1. This brain model has been verified with positive results [Citation11], e.g., improvements up to 11% in mean dose have been seen for the chiasm when using RapidPlan™. The independent cohort of 30 patients for the present analysis of the prediction of the knowledge-based prediction tool consists of patients, that were part of the selection process from January 2020 to December 2022. The age interval of the patients was from 22 to 66 years old with a median of 46 years.
The GTV and CTV are delineated according to the DNOG Guidelines [Citation12], which are based on the ESTRO ACROP Guidelines [Citation13]. The OARs are delineated according to the EPTN atlas-based consensus [Citation14]. The present study was approved by the Quality Assurance Committee at the Aarhus University Hospital.
Treatment planning
The proton treatment plans were generated with Eclipse™ treatment planning system in combination with the Non-linear Universal Proton Optimizer (NUPO) version 16.1 (Varian Medical System, Palo Alto, CA). The plans of the test set of 30 patients consisted of at least three beams covering the target. The angles were chosen in a way that it will ensure the shortest path to the tumour avoiding unnecessary irradiation of healthy tissue, but considered that there will not be overlapping distal edge in critical organs [Citation15]. For superficial targets, the beams are equipped with range shifters of either 3 cm or 5 cm. The plans were robust optimised on the CTV and critical OARs with a set-up and range uncertainty of 2 mm and 3.5%, leading to a total of 12 combined uncertainty scenarios and two scenarios based on the range uncertainty only. The set-up uncertainty used for these model patients was 3 mm. The coverage criterion of the CTV of the worst-case scenario is set to be D98% = 95% conform to the DNOG radiotherapy guidelines. If the patient was enrolled to the proton selection process, the proton treatment plan was compared to the photon plan using the dose to the specific DNOG criteria organs at risk (OARs) ().
Analysis and evaluation
The RapidPlan™ brain model was executed on duplicates of the original proton plans. For each organ at risk, Pearson’s R2 regression correlation coefficient between the predicted and actual DVHmetrics was calculated. The regression of the data was analysed by calculating the slope and intercept, which is a measure for the accuracy of the prediction. If the slope is close to 1 and the intercept is close to 0, then the model can predict the dose to the OARs close to the ideal. To evaluate the degree of dispersion of the difference between the actual and predicted DVH metrics, the standard deviation and the mean difference were calculated. The studied OARs were the whole brain after subtraction of the CTV (brain-CTV), the hippocampus, the cochleas, and the pituitary gland.
Results
Correlation analysis revealed a strong correlation between the predicted and actual DVH metrics for brain-CTV, hippocampus and pituitary gland (). The intercept for all OARs, but the cochlea right shows a near perfect prediction with a very low systematic intercept. shows the graphical correlation between predicted and actual DVH metrics. For the hippocampus, cochlea, and pituitary gland, the prediction and standard deviation were less good.
Figure 1. The correlation between the predicted (x-axis) and actual (y-axis) for the DNOG specific DVHmetrics. The solid line represents the fit through the data points. The dashed lines represent the ±1 standard deviation of the fit. The standard deviations are specified in .
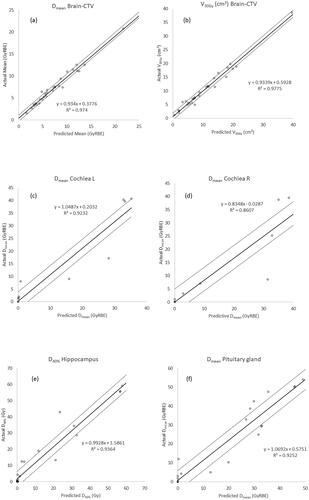
Table 2. Regression analysis data for the DNOG’s OARs.
Discussion
The result of this study shows a strong correlation between the predicted and actual/achieved DVH metrics of brain-CTV. There is a plausible correlation for hippocampus and poor correlation for the cochlea and pituitary gland. This is probably due to the small volume of the individual OARs and the placement of the tumour relative to it. In this study, the amount of data for the cochlea and pituitary gland is relatively sparse for a correlation analysis and the volume of the structures is small. On the other hand, the model predicts the DVH metrics for the whole brain when the CTV is subtracted (brain-CTV) very well. The standard deviation of the difference in metrics and the range are small. The use and verification of this knowledge based planning tool for both photon and proton treatment planning are described in the literature [Citation16–20]. In the literature, there is one Dutch study with positive results using the prediction of RapidPlan™ for selecting patients with head and neck cancer for proton radiation therapy [Citation21]. In another study, the photon model was used as a quality assurance tool for treatment plans that are included in the EORTC-1219-DAHANCA-29 trial [Citation22]. The anatomy of the head and neck and the use of integrated boost to the target with different dose levels is more complex compared to our brain tumour cases. Therefore, building a knowledge based model should be simpler. On the other hand, the beam configuration of a head and neck plan is quite standard compared to the configuration for brain tumours, depending on the localisation of the tumour and the OAR relative to it.
Moreover, proton beam therapy has its challenges, i.e., range uncertainty of the Bragg peak and the increased linear energy transfer at the end of range causing more biological damage compared to the same physical dose in other parts of the beam [Citation15,Citation23–30]. Until this effect is fully understood and implemented in a treatment planning system, treatment planning of proton beams must take this into account by choosing the right beam angles, excluding overlapping distal ends of the Bragg peak in an essential organ at risk. Still, decreased integral dose to brain and sparing OARs can be gained by using proton radiation therapy. The RapidPlan™ model makes a correlation between the dose to OAR and overlapping percentage with the target in the beam eye view. The model does not handle any knowledge about the placement of the distal edge of the beam. This could, in principle, lead to sub-optimal predictions. The model consists also of treatment plans with prescription doses of 54 and 59.4 GyRBE, where the field angles were chosen not to overlap in the critical OAR. Therefore, the model should, indirectly, take this issue in to account.
In the literature, there are only a couple of articles concerning RapidPlan™ for brain treatments that describe mainly whole brain radiation treatment with hippocampus sparing for metastases [Citation31–35]. There is only one study describing the use of RapidPlan™ in high grade glioma [Citation19]. In that study, only RapidPlan™ prediction of doses to cochlea and the pituitary gland was investigated in patients planned for photon radiotherapy. A Dutch study [Citation21] had overall good prediction of OAR doses using the RapidPlan™ model for patients with head and neck cancer. They go even further by using the prediction of the photon model to predict the dose reduction for critical organs used in the selection of patients for proton radiation treatment. In principle, there was no need to make treatment plans in full extend. In that study, they had also seen some large discrepancies between the predicted and the ultimately achieved dose to some OARs, which was explained by the small volume of the structures studied, like in our study. In brain tumour patients, the small OARs, like the cochleas could be either spared or receiving a high dose in the vicinity of the target, making the dose regression for these OARs more imprecise.
In our experience from discussing the comparative treatment plans, the selection of patients for proton therapy are rarely based on the dose reduction of the cochlea and pituitary gland. Most of these patients are selected, based on the criteria of the dose reduction of the structure brain-CTV. Using the RapidPlan™ model for comparative treatment planning in selection of patients will reduce the time and resources spent on the process considerably. Especially, based on the prediction of the dose metrics of brain-CTV, the process of fully optimising the treatment plan could be avoided. The time saved is approximately 1 h per proton treatment plan and depends on the hardware used and calculation settings. However, caution should be taken for the other OAR used as selection-based criteria for tumours close to critical OAR, such as the hippocampus, pituitary gland, and cochlea or results close to the selection criteria. False positive and false negative results of this prediction will be investigated in future work. This procedure of using the prediction of the DVHmetrics for the OARs could be further automated by using plan scripts leading to a potential larger throughput and faster pre-screening for patients eligible for proton radiation therapy at the referring departments.
Conclusions
In conclusion, the RapidPlan™ model predicts the DVH dose metrics for brain-CTV very accurately. The model has a plausible prediction for the hippocampus, but assessment of a larger group of patients is need to validate the model. The dose prediction for smaller structures like the cochlea and pituitary gland has a less good agreement and more data are needed. Using this model, potentially spares time and resources in selection and referral of brain tumour patients for proton therapy.
Disclosure statement
The authors have nothing to disclose.
Data availability statement
Corresponding author can be contacted for access to data and will provide data upon reasonable request. However, this requires that the ethical and data protection regulations allow sharing of the requested data.
Additional information
Funding
References
- Shih HA, Sherman JC, Nachtigall LB, et al. Proton therapy for low-grade gliomas: results from a prospective trial. Cancer. 2015;121(10):1712–1719. doi: 10.1002/cncr.29237.
- Noel G, Gondi V. Proton therapy for tumors of the base of the skull. Chin Clin Oncol. 2016;5:1–17.
- Noël G, Habrand JL, Mammar H, et al. Highly conformal therapy using proton component in the management of meningiomas. Preliminary experience of the Centre de Protonthérapie d’Orsay. Strahlenther Onkol. 2002;178:480–485.
- Sherman JC, Colvin MK, Mancuso SM, et al. Neurocognitive effects of proton radiation therapy in adults with low-grade glioma. J Neurooncol. 2016;126(1):157–164. doi: 10.1007/s11060-015-1952-5.
- Thurin E, Nyström PW, Smits A, et al. Proton therapy for low-grade gliomas in adults: a systematic review. Clin Neurol Neurosurg. 2018;174:233–238. doi: 10.1016/j.clineuro.2018.08.003.
- van der Weide HL, Kramer MCA, Scandurra D, et al. Proton therapy for selected low grade glioma patients in The Netherlands. Radiother Oncol. 2021;154:283–290. doi: 10.1016/j.radonc.2020.11.004.
- Grau C, Durante M, Georg D, et al. Particle therapy in Europe. Mol Oncol. 2020;14(7):1492–1499. doi: 10.1002/1878-0261.12677.
- Willson R. Radiological use of fast protons. Radiology. 1946;47:487–491.
- Durante M, Paganetti H. Nuclear physics in particle therapy: a review. Rep Prog Phys. 2016;79:096702.
- DNOG. DNOG 2018 Nationale retningslinjer for proton behandeling [National guidelines for proton therapy]; 2018. Available from: https://www.dnog.dk/assets/files/Retningslinier PDF/DNOG 2018 Proton retningslinjer.pdf
- Smulders B, Alshaikhi J, Stolarczyk L, et al. Proceedings to the 60th Annual Conference of the Particle Therapy Cooperative Group. Int J Part Ther. 2023;9(4):306–470.
- DNOG. Retningslinjer for stralebehandling [Guidelines for radiotherapy]; 2016. p. 1–16. Available from: https://dnog.dk/onewebmedia/files/Retningslinier PDF/DNOG 2016 Retningslinjer for straalebehandling final.pdf
- Combs SE, Baumert BG, Bendszus M, et al. ESTRO ACROP guideline for target volume delineation of skull base tumors. Radiother Oncol. 2021;156:80–94. doi: 10.1016/j.radonc.2020.11.014.
- Eekers DB, In 't Ven L, Roelofs E, et al. The EPTN consensus-based atlas for CT- and MR-based contouring in neuro-oncology. Radiother Oncol. 2018;128(1):37–43. doi: 10.1016/j.radonc.2017.12.013.
- Toma-Dasu I, Dasu A, Vestergaard A, et al. RBE for proton radiation therapy – a Nordic view in the international perspective. Acta Oncol. 2020;59(10):1151–1156. doi: 10.1080/0284186X.2020.1826573.
- Tol JP, Delaney AR, Dahele M, et al. Evaluation of a knowledge-based planning solution for head and neck cancer. Int J Radiat Oncol Biol Phys. 2015;91(3):612–620. doi: 10.1016/j.ijrobp.2014.11.014.
- Xu Y, Cyriac J, De Ornelas M, et al. Knowledge-based planning for robustly optimized intensity-modulated proton therapy of head and neck cancer patients. Front Oncol. 2021;11:737901. doi: 10.3389/fonc.2021.737901.
- Xu Y, Brovold N, Cyriac J, et al. Assessment of knowledge-based planning for prostate intensity modulated proton therapy. Int J Part Ther. 2021;8(2):62–72. doi: 10.14338/IJPT-20-00088.1.
- Chatterjee A, Serban M, Abdulkarim B, et al. Performance of knowledge-based radiation therapy planning for the glioblastoma disease site. Int J Radiat Oncol Biol Phys. 2017;99(4):1021–1028. doi: 10.1016/j.ijrobp.2017.07.012.
- Delaney AR, Verbakel WF, Lindberg J, et al. Evaluation of an automated proton planning solution. Cureus. 2018;10(12):e3696. doi: 10.7759/cureus.3696.
- Delaney AR, Dahele M, Tol JP, et al. Using a knowledge-based planning solution to select patients for proton therapy. Radiother Oncol. 2017;124(2):263–270. doi: 10.1016/j.radonc.2017.03.020.
- Tol JP, Dahele M, Gregoire V, et al. Analysis of EORTC-1219-DAHANCA-29 trial plans demonstrates the potential of knowledge-based planning to provide patient-specific treatment plan quality assurance. Radiother Oncol. 2019;130:75–81. doi: 10.1016/j.radonc.2018.10.005.
- Lühr A, von Neubeck C, Pawelke J, et al. “Radiobiology of proton therapy”: results of an International Expert Workshop. Radiother Oncol. 2018;128(1):56–67. doi: 10.1016/j.radonc.2018.05.018.
- Paganetti H. Relative biological effectiveness (RBE) values for proton beam therapy. Variations as a function of biological endpoint, dose, and linear energy transfer. Phys Med Biol. 2014;59(22):R419–R472. doi: 10.1088/0031-9155/59/22/R419.
- Giovannini G, Böhlen T, Cabal G, et al. Variable RBE in proton therapy: comparison of different model predictions and their influence on clinical-like scenarios. Radiat Oncol. 2016;11(1):68. doi: 10.1186/s13014-016-0642-6.
- Schreuder AN, Hedrick SG, Renegar JR, et al. Review of proton radiation therapy and the path to widespread clinical adoption. Med Phys Int J. 2016;4:35–46.
- Ödén J, Toma-Dasu I, Witt Nyström P, et al. Spatial correlation of linear energy transfer and relative biological effectiveness with suspected treatment-related toxicities following proton therapy for intracranial tumors. Med Phys. 2020;47(2):342–351. doi: 10.1002/mp.13911.
- Peeler CR, Mirkovic D, Titt U, et al. Clinical evidence of variable proton biological effectiveness in pediatric patients treated for ependymoma. Radiother Oncol. 2016;121(3):395–401. doi: 10.1016/j.radonc.2016.11.001.
- Jones B, McMahon SJ, Prise KM. The radiobiology of proton therapy: challenges and opportunities around relative biological effectiveness. Clin Oncol (R Coll Radiol). 2018;30(5):285–292. doi: 10.1016/j.clon.2018.01.010.
- Niemierko A, Schuemann J, Niyazi M, et al. Brain necrosis in adult patients after proton therapy: is there evidence for dependency on linear energy transfer? Int J Radiat Oncol Biol Phys. 2021;109(1):109–119. doi: 10.1016/j.ijrobp.2020.08.058.
- Feng CH, Cornell M, Moore KL, et al. Automated contouring and planning pipeline for hippocampal-avoidant whole-brain radiotherapy. Radiat Oncol. 2020;15(1):251. doi: 10.1186/s13014-020-01689-y.
- Liu H, Clark R, Magliari A, et al. RapidPlan hippocampal sparing whole brain model version 2—how far can we reduce the dose? Med Dosim. 2022;47(3):258–263. doi: 10.1016/j.meddos.2022.04.003.
- Rusu I, Roeske J, Solanki A, et al. Fully automated planning and delivery of hippocampal-sparing whole brain irradiation. Med Dosim. 2022;47(1):8–13. doi: 10.1016/j.meddos.2021.06.004.
- Sagawa T, Ueda Y, Tsuru H, et al. Dosimetric potential of knowledge-based planning model trained with HyperArc plans for brain metastases. J Appl Clin Med Phys. 2023;24:1–10.
- O'Toole J, Picton M, Perez M, et al. Improving efficiency in the radiation management of multiple brain metastases using a knowledge-based planning solution for single-isocentre volumetric modulated arc therapy (VMAT) technique. J Med Radiat Sci. 2021;68(4):364–370. doi: 10.1002/jmrs.526.