Abstract
Background
Exposure to different forms of ionizing radiation occurs in diverse occupational, medical, and environmental settings. Improving the accuracy of the estimated health risks associated with exposure is therefore, essential for protecting the public, particularly as it relates to chronic low dose exposures. A key aspect to understanding health risks is precise and accurate modeling of the dose-response relationship. Toward this vision, benchmark dose (BMD) modeling may be a suitable approach for consideration in the radiation field. BMD modeling is already extensively used for chemical hazard assessments and is considered statistically preferable to identifying low and no observed adverse effects levels. BMD modeling involves fitting mathematical models to dose-response data for a relevant biological endpoint and identifying a point of departure (the BMD, or its lower bound). Recent examples in chemical toxicology show that when applied to molecular endpoints (e.g. genotoxic and transcriptional endpoints), BMDs correlate to points of departure for more apical endpoints such as phenotypic changes (e.g. adverse effects) of interest to regulatory decisions. This use of BMD modeling may be valuable to explore in the radiation field, specifically in combination with adverse outcome pathways, and may facilitate better interpretation of relevant in vivo and in vitro dose-response data. To advance this application, a workshop was organized on June 3rd, 2022, in Ottawa, Ontario that brought together BMD experts in chemical toxicology and the radiation scientific community of researchers, regulators, and policy-makers. The workshop’s objective was to introduce radiation scientists to BMD modeling and its practical application using case examples from the chemical toxicity field and demonstrate the BMDExpress software using a radiation dataset. Discussions focused on the BMD approach, the importance of experimental design, regulatory applications, its use in supporting the development of adverse outcome pathways, and specific radiation-relevant examples.
Conclusions
Although further deliberations are needed to advance the use of BMD modeling in the radiation field, these initial discussions and partnerships highlight some key steps to guide future undertakings related to new experimental work.
Context
Integration of scientific knowledge into human health risk management requires the meaningful translation of wide-ranging research outputs, derived from a plethora of diverse data, to hazard and risk assessment strategies. Amongst the various data types generated in the radiation field, epidemiological studies have been important in informing risk models for hazard assessment. Specifically, the ongoing epidemiological studies of aging exposure cohorts (ICRP Citation2012) has helped to improve the understanding of health risks from particularly high acute dose exposure. However, the effects of low-dose exposures are still subject to some uncertainty. This has led to new research on the practicality of using the linear-no threshold (LNT) model as the basis of radiation protection (NCRP Citation2020).
The LNT model is largely derived from specific life span study data of exposure of the Japanese atomic bomb survivors, and medical therapy patients, and continues to be supported by nuclear plant worker studies (NCRP Citation2020). Over the years, it has been recognized that alongside human epidemiological studies, mechanistic data from in vitro and in vivo studies are also valuable in reducing controversies and uncertainties on the health impacts of low-dose and low-dose rate exposures. Heightened global interest on the LNT model has directed efforts toward exploring new technological developments and approaches that have emerged during the past decade.
Two analytical methods that consider causal reasoning to justify exposure and response relationships as used in the regulation of chemical toxicants have been suggested to help refine risk estimations for exposures in the radiation field. These approaches include Benchmark Dose (BMD) modeling (reviewed in Filipsson et al. Citation2003; Sand et al. Citation2008; Davis et al. Citation2011; EFSA Citation2017; Haber et al. Citation2018) and the Adverse Outcome Pathway (AOP) framework as launched by the Organisation for Economic and Co-operation and Development (OECD) (Gibb Citation2008; Krewski et al. Citation2010; OECD Citation2017, Citation2018; Wang et al. Citation2020). Both are applicable to biological changes observable soon after exposure, which may be correlated to the dynamics of latent disease progression (Boobis et al. Citation2006; Boobis et al. Citation2008).
Efforts are underway to raise awareness of the AOP approach among radiation researchers, regulators, and policy-makers (Preston Citation2015; Chauhan et al. Citation2016; Chauhan et al. Citation2021b; Chauhan et al. Citation2022a). As of June 2021 integrating AOPs into radiation research and regulation is an area being explored through the OECD Nuclear Energy Agency (NEA) High-Level Group on Low-Dose Research (HLG-LDR) Radiation/Chemical AOP joint topical group (Chauhan et al. Citation2021c; Chauhan et al. Citation2022b). A goal of the topical group is to demonstrate the use of new approach methods (e.g. transcriptional data and other OMICS technologies), review available data and analysis methods and advance the applicability of AOPs for ionizing radiation. Despite the adoption of BMD modeling for robust effect threshold characterization of chemicals, limited understanding and implementation of the BMD approach is currently hindering the applicability for AOP development for ionizing radiation.
Workshop goals
To bring awareness of BMD modeling as a robust effective modeling approach to the radiation community, a workshop was organized and held on June 3rd, 2022. The workshop brought together Canadian and American radiation scientists, regulators, and policy-makers to discuss the BMD approach with renowned international experts in the area of BMD modeling it as relates to the chemical field. The objectives of the BMD workshop were to:
Introduce the concept (what it is and how it is being used through shared experiences from experts in chemical toxicology, as well as key metrics for consideration in the design of experiments) to attendees involved in radiation research, regulation, and policy-making;
Demonstrate the BMDExpress software that is routinely used in BMD analysis of genomics data (Phillips et al. Citation2019);
Provide case examples demonstrating its comparability to existing applications in chemical regulation;
Discuss the relevance of BMD modeling in low-dose radiation health and ecological impacts.
The workshop (Supplementary Figure 1, agenda) started with opening remarks highlighting the context and key goals. Experts from Health Canada, the University of Ottawa (Canada), the University of Cincinnati (USA), and the National Institute of Environmental Health Sciences (NIEHS) (USA) provided an overview of phenotypical (apical) and transcriptional BMD effect modeling and how to effectively design experiments to support their use. The workshop speakers also presented a comprehensive overview of the BMD approach to new users and the types of studies that can be evaluated. In addition, a vision for use was presented on how the radiation field can harness BMD modeling to address regulatory questions and potential challenges that require focused discussions. The goals, content, and outcomes of the workshop are described here.
BMD modeling: considerations and advantages for use on apical endpoints
For the radiation field to begin the application of the BMD modeling approach, there is a need to understand the considerations for proper implementation. Lynne Haber, Associate Professor at the University of Cincinnati, explained that the BMD approach has been proposed as a substitute to the use of a No Observed Adverse Effect Level (NOAEL) or a Lowest Observed Adverse Effect Level (LOAEL) approach (Crump Citation1984). Normally, chemical risk is evaluated based on the adverse effects of a toxicant and understanding the dose-response relationship using animal models. In this hazard assessment, what is relevant to consider is the dependency on the dose or concentration (equivalency to dose rate) in the context of response using an endpoint of human or environmental health relevance (e.g. an observable effect such as the increase in genotoxicity or reduction in reproduction). BMD modeling fits a group of flexible mathematical models to dose-response data to identify a dose (the benchmark dose, or BMD) that corresponds to a specified change in response compared to the background (USEPA Citation2012; reviewed in Haber et al. Citation2018). The specified change in response is called the benchmark response (BMR), or critical effect size (CES). In parallel with the BMD, the lower bound (BMDL) and upper bound (BMDU), representing the 95% confidence limits on the BMD, are also calculated (). In chemical hazard assessment, the BMDL often is used to set safe dose limits.
There are several advantages that BMD modeling has over the traditional derivation of the No Observed Adverse Effect Level (NOAEL) and the Lowest Observed Adverse Effect Level (LOAEL). The NOAEL is limited to the specific doses tested, while the BMD, which is interpolated from the dose-response curve and therefore, can provide a more accurate point of departure for hazard characterization. Furthermore, a BMD can be calculated for studies that do not identify a NOAEL. The BMD is based on a defined response level, and thus is consistent across experimental studies, whereas the experimental response at a NOAEL can vary substantially depending on the study sensitivity (e.g. statistical power) and the number of doses used (e.g. spacing of treatment groups). Finally, the NOAEL is highly dependent on the sample size and variability within the control and treatment groups, while the BMD approach appropriately reflects the uncertainty in the data (when the BMDL is used). For example, a response that may be statistically different from controls (and thus a LOAEL) with 50 animals per dose, may not be statistically significant in a study with 10 animals per dose. However, the BMDL will be lower in the study with fewer animals, due to the resulting wider confidence limits, and thus more appropriately reflect the greater uncertainty in the smaller study.
Lynne Haber also described the importance of the choice in the mathematical model used to fit the data. Early BMD modeling work often resulted in the modeler choosing a single best-fit model. This sometimes meant that groups that modeled the same data could obtain different final BMDLs because they chose different models. More modern methods (e.g. Wheeler and Bailer Citation2009) use model averaging, where models are weighted by the Akaike information criterion (AIC), a measure of the model fit that also rewards parsimony in the number of model parameters (Wheeler and Bailer Citation2007, Citation2009). The use of model averaging improves the consistency of the final result and better reflects the uncertainty in the models.
A final discussion point was determining the appropriate BMR. Slob and colleagues developed an approach for identifying the BMR for genotoxicity data, based on the endpoint-specific maximum or its surrogate, the within-group standard deviation, (SD) (Slob Citation2017). Using this approach, the BMR for in vivo chromosome damage is 71–79%, but additional research is needed to identify other BMR for other genotoxicity endpoints. The EPA has recommended that the BMD corresponding to one standard deviation from the control mean response be reported for modeling of continuous (generally apical) endpoints, regardless of the method used for defining the BMR (USEPA Citation2012).
These considerations should, in general, be applicable to radiation datasets, however further evaluation on the technical aspects of optimal or minimum requirements of test designs and the use of data from different levels of biological organization for defining the PODs will be important. Specifically, on the appropriate degree of change and BMR for radiation cytotoxicity and the best use of appropriate dosimetry metrics (e.g. dose or dose rate), could be evaluated. An assessment of these will then ensure meaningful BMD values are derived as the basis for translation into protective radiation dose limits for human and environmental health risks.
Transcriptional BMDs: proof of concept and advances in use for chemical hazard and risk assessment
The workshop also discussed how BMD could be applied to transcriptomic datasets. This is relevant to the radiation field which has generated a considerable depth of transcriptional outputs. In the chemical field, mechanistic information is increasingly being used to improve the prediction of chemically-induced human health effects, specifically, with the use of toxicogenomics and other sources of high-content response data. Toxicogenomics is viewed as particularly valuable because it provides broad (genome-wide) coverage of the biological effects that are altered following exposure. However, one key challenge has been determining the best way to efficiently analyze and interpret these complex data sets to inform regulatory decision-making. This has hindered regulatory uptake for chemical hazard assessment. One solution works toward a long-term vision that applies transcriptomic biomarkers (e.g. single gene responses or combination of these in relevant gene sets, pathways, etc.) that can be used to predict hazards, complemented by dose-response modeling to identify a concerted molecular response and alignment with the AOP framework for predicting toxicity outcomes at different levels of biological organization. A key tool for advancing this area is the development and release of the BMDExpress software and similar tools (e.g. R-package, DRomics), which enable parallel BMD modeling of a high number of transcripts.
Overview of BMDExpress software
Scott Auerbach from the US National Toxicology Program at the NIEHS provided a demonstration of the BMDExpress Software (Yang et al. Citation2007; Zheng et al. Citation2014; Phillips et al. Citation2019; Ewald et al. Citation2021). An overview of the current guidance and best practice of performing genomic dose-response analysis using the BMDExpress software was initially reviewed. Subsequently, presented were recent updates to the software including their impact on the analysis of results, and the functionality the software demonstrated with a proposed workflow using a radiation dataset (). Scott Auerbach explained that following data normalization, quality control filtering is performed to identify dose-responsive features using a Williams trend test in combination with an effect size filter (e.g. fold change>|1.5|). Features passing the prefilter are then subjected to dose-response model fitting using 8 parametric models. A single best model is then selected based on the lowest AIC score. The best-fit models for each feature are then further filtered, removing those with a BMD/BMDL >20 and global goodness of fit p-value <0.1. The surviving features are collapsed into genes and then passed into a functional classification analysis where they are sorted into predefined gene sets (e.g. gene ontology biological processes). Finally, genes passing all the above criteria are identified to be ‘active’, i.e. responsive to radiation treatment. The gene set demonstrating the lowest BMD or BMDL is reported as the molecular POD for the test article. In addition, toxicokinetic modeling functionality has been integrated into recent versions of the software. This additional feature allows the user to estimate dose levels that correspond to internal and external doses.
Figure 2. A general work flow demonstrating the use of BMDExpress for genomic dose response analysis. The steps include study design, expression data (data quality control), pre-filtering for dose-response behavior, BMD analysis (selection, parameterization, and characterization of BMD), functional classification (pathway analysis), and biological interpretation (tPOO determination). QC: quality control; ANOVA: analysis of variance; FOR: false discovery rate; ORIOGEN: Order-restricted inference for ordered gene expression; BMD: benchmark dose; EPA: environmental protection agency; BMDS: benchmark dose software; MLE: maximum likelihood estimation; BEPOO: biological effect point of departure (lowest BMD and BMDL of the ‘active’ gene sets); tPOO: transcriptomic point of departure; GO: gene ontology; GSG: general signature genotoxicity.
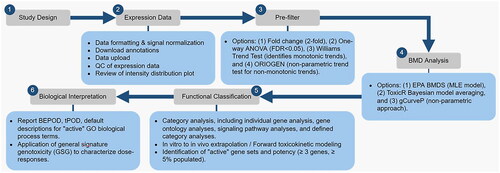
Apical and transcriptional BMD validation studies and technical challenges
Andrew Williams from Health Canada presented how the BMD is defined generally using the BMDExpress software. This included the various dose-response models and model assumptions as well as how BMD modeling was adapted for transcriptomics. He also discussed traditional PODs and reviewed different types of proposed summary statistics for defining transcriptomic POD (tPODs) (Webster et al. Citation2015; Farmahin et al. Citation2017). He highlighted that many validation studies have shown that tPODs tend to be within ten-fold lower of the apical PODs (Thomas et al. Citation2013b; Farmahin et al. Citation2017; Pagé-Larivière et al. Citation2019; Ramaiahgari et al. Citation2019; Gwinn et al. Citation2020; Johnson et al. Citation2020; Alcaraz et al. Citation2021; Crizer et al. Citation2021; Alcaraz et al. Citation2022; Mittal et al. Citation2022). This quantitative concordance supports the potential utility of short-term transcriptomic studies in chemical hazard assessment, and it has generally been argued that the use of tPODs would be protective for human health risk assessment purposes. For example, using rat transcriptome and apical data for 79 chemicals obtained from the Open Toxicogenomics Project-Genomics Assisted Toxicity Evaluation System (TG-GATES), Johnson et al. demonstrated that short-term exposure, transcriptome-based liver tPODs were predictive of longer-term (chronic) phenotypic PODs (Johnson et al. Citation2020). Apical endpoints considered in this study were body weight, clinical observation, kidney weight and histopathology and liver weight and histopathology. A strong positive correlation (Pearson R = 0.86) and predictive accuracy (root mean square difference = 0.41) were observed between the 29-d liver tPODs and the apical PODs. Johnson et al. concluded that apical PODs from liver and non-liver compartments derived from longer-term studies could be estimated using a liver tPOD estimated from an acute or a sub-acute exposures. Numerous other studies have been conducted to similarly support the utility of tPODs in predicting the dose at which adverse apical effects occur (Thomas et al. Citation2013a; Pagé-Larivière et al. 2019; Gwinn et al. Citation2020).
Andrew Williams explained some additional technical challenges concerning tPODs due to the high dimensionality of these genome-wide experiments. He presented results from a study using solely solvent controls that evaluated TempO-Seq (https://www.biospyder.com/) gene expression data to examine the empirical false discovery rate (FDR) (Ramaiahgari et al. Citation2019). This analysis randomly assigned solvent control samples to ‘dose groups’ and applied various BMD modeling pipelines. He showed that increasing the sample size and/or using a more conservative p-value cutoff for the Williams’ Trend Test, or increasing the fold change cutoff prior to BMD modeling, improved the control of the empirical false discovery rates, FDRs (i.e. FDRs < 0.05).
Andrew Williams also discussed the technical challenges of estimating confidence intervals for tPODs. With the complexity of many of the tPOD definitions, the bootstrap method is one technique that could be used to appropriately estimate tPOD confidence intervals. Confidence intervals for the 25th gene of the total distribution and the lowest pathway were examined. These analyses indicate that the confidence intervals for the 25th gene are not as wide as those for the lowest pathway.
Carole Yauk (University of Ottawa) discussed that despite the significant advances made in developing transcriptomic technologies and pipelines for these applications, there remain numerous challenges that must be addressed to facilitate its regulatory adoption in chemical toxicology. Some key questions include:
Will certain toxicological effects be missed?
Since gene expression changes are transient and not necessarily adverse, will decisions be based on adaptive versus adverse effects?
Since gene expression changes are among the first and most sensitive responses to a stressor, and how predictive and protective are such low doses for phenotypic events?
What is the uncertainty associated with these new approaches?
What is the optimal quantization method for transcriptomic POD (tPOD)?
Are different modeling approaches and methods (software) providing comparable results?
To address these questions, case studies that bring together the regulatory and research community are being used to facilitate knowledge exchange, obtain feedback on feasibility and potential barriers. These case studies provide opportunities for training, harmonization, and early integration that can build confidence for regulatory adoption.
Case studies
Carole Yauk described a few case studies exploring the use of tPODs in different decision-making contexts. She first described collaborative efforts on the chemical hexabromocyclododecane (HBCD) to demonstrate how transcriptomic BMD modeling of liver samples from short-term studies could be used within a tiered testing paradigm. This work showed high concordance in the PODs derived from conventional test methods relative to tPODs produced from rat livers (Farmahin et al. Citation2019). In a case study on a chemical grouping of 23 per- and poly-fluoroalkylated substances (PFAS), she discussed how tPODs produced from exposed human liver hepatocyte spheroids could be used to rank order the potency of chemicals within this group (Reardon et al. Citation2021; Rowan-Carroll et al. Citation2021). The data were consistent with the expected potency of these compounds based on the carbon chain length of each of the chemicals. In a case study on substitutes for the endocrine-disrupting chemical bisphenol A in MCF7 cells, she summarized how transcriptomic BMD modeling supports not only potency comparison but identification of inactive compounds in this model system (Matteo et al. Citation2022). Finally, the work also showed how transcriptomic BMD modeling can be used to examine mechanistic similarities by pathway and upstream regulator enrichment analysis of dose-responsive pathways (Matteo et al. Citation2022).
Overall, these case studies exploring the use of transcriptomics in decision-making for chemical hazard assessment have demonstrated that the dose at which transcriptional perturbations arise in short-term studies is consistent with the dose at which adverse phenotypic effects like cancer occur following longer-term exposures (Thomas et al. Citation2013b; Pagé-Larivière et al. 2019; Gwinn et al. Citation2020; Johnson et al. Citation2020). These studies also suggest that tPODs are not overly conservative and will not produce unachievable risk management levels for chemical exposures. One important challenge that remains is identifying the optimal approach to derive a tPOD (i.e. best practices and guidelines). However, the work suggests that the dose at which the transcriptome is robustly perturbed would also induce adverse health consequences following prolonged exposure. It also suggests that a variety of approaches can be used to identify tPODs and they lead to similar outcomes.
Carole Yauk concluded that these case studies have been very useful to inform regulatory applications and to build confidence in applications for chemical hazard assessment. She recommended that research experts in transcriptomics should continue to promote and support collaborations with the regulatory community to advance these applications. The establishment of best practices for deriving tPODs is necessary and should consider both the technical aspects (e.g. impacts of filtering, normalization, platform, study design, etc.) alongside the different contexts of use for the tPODs in decision-making. She noted that the OECD has developed a framework for reporting data and methods used for regulatory submissions of transcriptomic (and other OMICS) data that will ensure transparency and may facilitate the developing acceptable practices (Harrill et al. Citation2021b). She emphasized that it will be critical to demonstrate applicability across a broad chemical and biological space to support mainstream integration for decision-making.
Radiation BMD modeling and tPODs: current status and future perspective
Vinita Chauhan (Health Canada) described recent work using the BMD approach for radiation datasets. She provided a vision for how the BMD approach may be used in the field of radiation research and regulation. Ngoc Vuong (Health Canada) followed and discussed the importance of study design to achieve optimal BMD outputs.
Vinita Chauhan highlighted that transcriptional events may indeed provide a predicative understanding of the exposure-response to outcome relationship, especially if organized across levels of biological organization using the AOP framework (). However, currently in the radiation field, this type of data is minimally explored and efforts need to be directed to help advance this area. This is particularly timely as more efforts globally are being directed to understand the health effects from low dose and low dose-rate radiation exposures.
Radiation case studies
Vinita Chauhan’s group has published several case studies on the BMD approach using ‘in-house’ generated datasets (Chauhan et al. Citation2016; Qutob et al. Citation2018; Chauhan et al. Citation2019) to explore the relationship between transcriptomic BMDs and identifying dose limits. The undertakings focused on assessing BMD outputs related exposure of the lens of the eye to ionizing radiation, and UV exposure of skin leading to erythema. Human lens epithelial cells were exposed across a broad dose-range (0–5 Gy) to X-rays administered at a low dose-rate (LDR: 1.62 cGy/min) or high dose-rate (HDR: 38.2 cGy/min). BMD analysis of the transcriptional responses showed initiation of different pathways for the HDR exposure relative to the LDR exposure. The analysis highlighted that the lowest pathway BMDs (0.6 Gy) for the HDR exposure aligned well with the threshold of effect identified by the International Commission on Radiological Protection (ICRP) for the lens of the eye (0.5 Gy) (Ainsbury et al. Citation2009). The ICRP limits were derived from a review of epidemiological studies using lens opacities as the measurement endpoint (ICRP Citation2012). Furthermore, for the LDR exposures, transcriptional BMD analysis showed the lowest pathway BMD value to be 2.5 Gy, indicating a dose-rate effect that may or may not have repercussions for late effects such as cataracts. Similarly, a study assessing UV exposure and skin erythema using human epidermal keratinocytes demonstrated that a subset of transcriptional BMD values aligned with current dose estimates for skin reddening. Together, these case studies show observations of concordance between tPODs and apical PODs, similar to chemical studies.
Additionally, Vinita Chauhan discussed the need to validate the consistency and reproducibility of BMDs in the context of radiation datasets. For this, she presented a meta-analysis of 33 relevant transcriptomic studies deposited in the Gene Expression Omnibus (GEO: https://www.ncbi.nlm.nih.gov/geo/) (Chauhan et al. Citation2021a). The work highlighted how BMD values are reproducible across similarly designed studies and how radiation parameters (dose-rate and radiation type) can impact BMD outputs. This latter observation underscored the potential of using BMD values to compare exposure scenarios. For example, using results from a study conducted ‘in-house’, she showed that an alpha particle exposure to blood cells generally derived much lower gene and pathway BMD values compared to an X-ray or γ-ray exposure, implying the latter two are less effective in activating transcriptional responses (Chauhan et al. Citation2021a). Additionally, the meta-analysis also showed that lymphocytes generally have lower transcriptional BMD values relative to fibroblasts for the same pathways, indicating that the latter cell type may be more radioresistant.
An interesting finding that arose from this work was the bimodal distribution of BMD values, an observation not prevalent in the chemical toxicology field. Vinita Chauhan explained how these modes may translate to early transcriptional responses that may underlie key events in AOPs. Pathways with low BMDs values (DNA repair, cell signaling), representing mode 1, could be associated with early key events in an AOP and higher pathway BMD values could be associated with later key events (immune response, pathology). Further exploration of BMDs is required toward defining tissue weighting factors and relative biological effectiveness (). Together, these initial studies have provided some key findings that underscore areas where BMD modeling could be applicable in the radiation field.
Figure 4. Schematic showing how key events in an adverse outcome pathway can be the basis of experimental design and data interpretation using the BMD approach and provide information on relative biological effectiveness, tissue weighting factors and the protective dose. AOP: adverse outcome pathway; KE: key events; BMD: benchmark dose. Additional details on derived outputs can be found in Chauhan et al. (Citation2016).
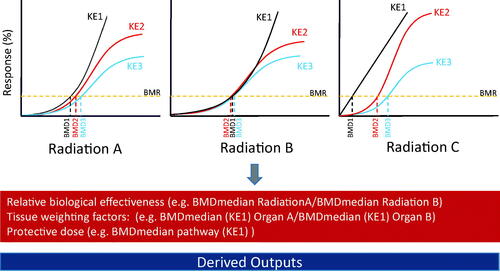
Importance of experimental design
Vinita Chauhan also highlighted the importance of identifying technical parameters (i.e. exposure doses and biological replicates) to develop guidance on how the BMD approach may effectively be applied in terms of optimal study design for radiation datasets. In this context, Ngoc Vuong discussed in depth the impact of the number of exposure doses and biological replicates required to produce meaningful BMD results (Stainforth et al. Citation2022). To illustrate these points, a microarray dataset (GSE52403) from mice irradiated with 137Cs γ rays was used (Lucas et al. Citation2014). In the study, animals were subjected to whole-body irradiation at 8 different absorbed doses (0, 1, 2, 3, 4.5, 6, 8, and 10.5 Gy), where 15 mice were used for the control and 5 mice were used for the non-zero doses. For the meta-analysis, systematic removal of dose(s) or biological replicates was undertaken and observed changes to the trend of their respective BMD outputs were assessed. Overall, the accuracy of BMD modeling was low when 1 Gy dose was removed or the number of doses was <4. The study also showed that BMD modeling accuracy and precision were reduced with decreasing number of replicates, particularly for the non-zero dose groups. Reducing biological replicates led to fewer genes identified, higher false discoveries, and/or lower accuracy in BMD value.
Ngoc Vuong also explained the potential application of BMD modeling to discern susceptibilities relating to biological variables such as sex and smoking status. To this end, he used a publicly available microarray dataset (GEO #GSE23515) that examined human peripheral blood exposed to 0, 0.1, 0.5 and 2 Gy of γ rays ex-vivo at 0.82 Gy/min, and assessed 6 h post-exposure (Adam et al. Citation2022). In this study, blood was donated by six female nonsmokers (F-NS), six male nonsmokers (M-NS), six female smokers (F-S), and six male smokers (M-S). The results showed BMD distribution was bimodal across the four groups. Unique genes were identified for each of the confounding groups F-NS (74), M-NS (41), F-S (62), and M-S (62). Pathway analysis identified common pathways in all 4 study groups. The majority of the common pathways centered on TP53-regulated pathways of cell death and cell cycle. There was no notable differential sensitivity of responses in these robust common genes and pathways for any specific group. However, unique pathways with associated BMDs could be identified for each group: F-NS (1), M-NS (7), F-S (1), and M-S (2). Clearly, to enhance confidence in the unique responses, future studies should use a larger cohort of individuals and a broader dose range to confirm the BMD modeling outputs. This initial work highlights promising aspects of the BMD approach, which warrant further exploration through well-designed experimental work and data assessment.
Discussions and key messages
There was a general consensus supporting the use of BMD modeling in AOP-informed radiation hazard and risk assessment applications. Participants agreed that the approaches and tools presented have matured to a stage where broader adoption is possible based on the extensive work done in chemical toxicology. Participants commented that the data integration in BMDExpress is impressive and the examples of data analyses, biomarker discovery, and their use for setting guidelines were informative. The comparison between the BMDs derived using transcript data and BMD using conventional toxicity tests provides support for focused efforts in the translation of this approach to human radiological risk assessment. The comparability of BMDs derived using transcriptomics data and BMDs from conventional toxicity testing is an important validation exercise that is needed for confident use in assessments of the health risks of exposure to ionizing radiation. Participants also indicated that the model averaging approach is intriguing, possibly requiring standardization/harmonization and assessment across different radiation parameters.
It was noted that in addition to transcriptomic data, the BMD approach has also been tested using other kinds of high-dimensional data, including proteomics, epigenomics, and metabolomics (which reflects a later endpoint more closely tied to health outcomes) in chemical toxicology studies (Miousse et al. Citation2017; Rager et al. Citation2017; Olesti et al. Citation2021). In fact, TOXcms, a recently published freely available software (http://pattilab.wustl.edu/software/toxcms), was developed to understand biochemical mechanisms and off-target drug effects for dose-response metabolomics (Yao et al. Citation2020).
Additionally, the multi-modal distribution of gene BMDs was of interest, as this had been observed when examining radiation datasets. It is evident that some genes respond in the low dose range, and there may be a 2nd (or a 3rd) surge in responses at a higher dose. With the BMD approach, it could be possible to distinguish between lower dose vs higher dose mechanisms when evaluating health impacts and identify the most relevant toxicity mechanism and pathways for a given adverse outcome. This can be pragmatically used to identify the most relevant AOPs in more complex AOP network using either BMD modeling or Bayesian approaches (Moe et al. Citation2021).
In the future, it will be worth exploring how phenomena such as hormesis and chronic low dose exposures translate to transcriptional BMD values. It may be that the peaks observed in a hormetic response as assessed by an exposure-response curve represent tipping points for different types of toxicity that lead to bursts in transcriptomic activity in specific pathways (Vaiserman et al. Citation2021). In the case of chronic low dose exposures, reported signature genes may show shifted BMD values as the duration of the exposure decreases. These types of questions and others may be the focus of future work.
Conclusion
The outcomes of the workshop have highlighted that the BMD approach has been well developed and validated over the past decades, the analytical pipelines are fully transparent and readily transferrable between labs (user-friendly), and the case examples with different radiation sources demonstrate proof of concept in their application for radiation risk assessment. Transcriptional BMDs can provide predictive mechanistic information for human health effects that can support hazard and risk assessment strategies (Harrill et al. Citation2021a; Krewski et al. Citation2020; Ramaiahgari et al. Citation2019; Thomas et al. Citation2007; Thomas et al. Citation2013a). Adoption of the approach for radiation protection could be a consideration, particularly with the many exposure scenarios defined by various radiation types, doses, and dose rates of delivery and associated health outcomes.
It is envisioned that continued partnerships with the chemical field will position the radiation field to produce similar correlative studies that promise to show how early relevant molecular events (transcriptional changes) could align with adverse effects observed in an animal or human studies (). Efforts in this direction have already been undertaken but more case studies are needed, particularly informed by AOPs. To progress efforts toward consideration in regulatory guidelines the following steps are needed:
Knowledge transfer to the international radiation community of work underway in the chemical field in the context of new approach methods and use in human health protection
International survey to gauge the perception of experts within the radiation field to use transcriptional data to inform hazard and risk assessment strategies
Development of case studies that identify PODs informed by AOPs that can be used as dose concordance data
As work in these area progresses, it is envisioned that BMD modeling and quantitative AOP development will identify more refined dose estimates for the risk of health effects from low-dose and low dose-rate ionizing radiation exposures when physical radiation dosimetry is unavailable.
Acknowledgments
This work was supported by the Genomics Research and Development Initiative and the Atomic Energy of Canada Limited’s Federal Nuclear Science & Technology Program. The authors are grateful to Dr. Holly Laakso and Dr. Lindsay Beaton and Dr. Kathleen Cooke for critical review of the manuscript. The contents of this manuscript neither constitute, nor necessarily reflect the views of the institutions of the authors. Mention of trade names or commercial products does not constitute endorsement or recommendation for use.
Disclosure statement
No potential conflict of interest was reported by the author(s).
Additional information
Funding
Notes on contributors
Vinita Chauhan
Vinita Chauhan is a senior research Scientist at the Consumer and Clinical Radiation Protection Bureau of Health Canada. Her research focus includes the application of new approach technologies to help reduce the uncertainty in health risks at low dose low dose-rate radiation exposures. She is a Canadian delegate of the Nuclear Energy Agency high-level group on low-dose research (HLG-LDR) and co-chairs an international topical group which is working to integrate the use of adverse outcome pathway in radiation research.
Jihang Yu
Jihang Yu is a biologist at Canadian Nuclear Laboratories. Her current research interest is radiation-induced biological effects and application in adverse outcome pathways.
Ngoc Vuong
Ngoc Q. Vuong is a Senior Research Assistant at the Radiation Protection Bureau (RPB) of Health Canada. He graduated from the University of Ottawa with a Ph.D degree in Biochemistry (specialized in environmental toxicology). He joined the RPB in 2018, and has expertise in the areas of computational biology, bioinformatics, proteomics and high throughput assays.
Lynne T. Haber
Lynne Haber received a Ph.D. in biology from the Massachusetts Institute of Technology and leads the Risk Science Center in the Department of Environmental and Public Health Sciences at the University of Cincinnati. She has authored chemical hazard and dose-response assessments for many different organizations and conducted research on many aspects of chemical risk assessment, including a 2018 review of issues related to benchmark dose modeling.
Andrew Williams
Andrew Williams received an MSc in statistics from Carleton University, Ottawa, Ontario. Mr. Williams joined the Biostatistics Unit in the Environmental Health Sciences and Research Bureau at Health Canada in 2001. Mr. Williams has a broad knowledge of statistical theory and methods, with 23 years of experience working on projects requiring expertise in the areas of statistical inference, experimental design, generalized linear and generalized mixed modeling, and predictive modeling including data mining or machine learning methodologies.
Scott S. Auerbach
Scott Auerbach received a Ph.D. in Pharmacology from the University of Washington. In 2009 he became a staff scientist at the National Toxicology Program and a Diplomat of the American Board of Toxicology. He is currently the Toxicoinformatics Group Leader in the Biomolecular Screening Branch of the National Toxicology Program. His research focuses on application of molecular and high dimensional data to toxicological assessment with the goal of increasing efficiency in toxicology testing.
Danielle Beaton
Danielle Beaton, is a Research Scientist at Canadian Nuclear Laboratories. Her current research focuses on the effects of low dose radiation on biological systems. She is on the NEA HLG-LDR committee and assists the co-chairs of the HLG-LDR Rad/Chem AOP Joint Topical Group.
Yi Wang
Yi Wang is a research scientist and biologist at Canadian Nuclear Laboratories and an adjunct professor at the University of Ottawa. He graduated with a PhD degree in cell biology from the University of Alberta and received his postdoc training at the University of Toronto.
Robert Stainforth
Robert Stainforth is a Physical Scientist at the National Radon Laboratory, Radiation Protection Bureau, Health Canada. After graduating from Durham University (UK) in Math and Physics in 2012 Robert completed a Ph.D. in experimental particle physics at Liverpool University (UK) in 2016. Prior to joining Health Canada in 2019 he was a postdoctoral researcher of dark matter and astroparticle physics at Carleton University and specialized in the characterization of background signals from radon.
Ruth C. Wilkins
Ruth Wilkins is a Research Scientist at the Consumer and Clinical Radiation Protection Bureau of Health Canada and the Chief of the Ionizing Radiation Health Sciences Division. She graduated with a Ph.D. in Medical Physics from Carleton University and has been employed at Health Canada since 1996. She is an Adjunct Professor and lecturer of Radiobiology in the Department of Physics at Carleton University and the alternative representative of Canada to the United Nations Scientific Committee on the Effects of Atomic Radiation (UNSCEAR).
Edouard I. Azzam
Edouard Azzam is a Radiation Biologist at Canadian Nuclear Laboratories. He is also Professor Emeritus at Rutgers New Jersey Medical School and member of the US National Council on Radiation Protection and Measurements. His research has focused on the role of intercellular communication and oxidative metabolism in expression of adaptive responses and bystander effects induced by low doses/low fluences of ionizing radiation from environmental, occupational, or medical sources.
Richard B. Richardson
Richard Richardson, is a principal research scientist in the Radiobiology and Health Branch of CNL. He is also an adjunct professor of the Medical Physics Unit at McGill University and PhD supervisor of a CNL employee. Currently, Dr. Richardson is the principal investigator for three major research projects at CNL including assessing the radiation quality of thermal and fast neutrons, radiation-induced cataracts and to identify radon biomarkers in human blood. Dr. Richardson has authored recent publications on multistage carcinogenesis and the role of oxygen levels on cataract development in astronauts.
Md Gulam Musawwir Khan
Md Gulam Musawwir Khan is a dynamic and passionate researcher in Cancer Biology with a strong background in biochemistry, molecular/cellular biology, cell signaling and genomics. He has over 10 years of research experience in cancer molecular biology, especially in the field of liver cancer. Current research focuses on low dose radiation and its differential impacts on normal cells, cancer cells, and stem cells. Besides exploring the beneficial biological effects of low-dose radiation, he is also involved in the research of targeted alpha therapy with the aim to develop advanced life-saving treatments for cancers. He has published many scientific articles/reviews (as first author and co-author) in reputed international journals defining new scientific concepts and has also been invited for poster and oral presentations at national and international conferences.
Ashok Jadhav
Ashok Jadhav, is working as a Veterinarian in the Canadian Nuclear Laboratories (CNL), Chalk River. He has completed his degrees BVSc and AH (DVM) and MVSc (Vet. Pathology) from the Bombay Veterinary College and further received PhD from the University of Saskatchewan, Saskatoon (2009). He has published several peer-reviewed publications in the area of cardiovascular, diabetes, and, hypertension areas. His area of research interests are pharmacology, toxicology, pathology, and laboratory animal medicine. In the CNL, he supports animal care and ethic program as well as low dose radiation research. He is also supporting Good Laboratory Practice program and pre-clinical toxicity testing research.
Julie J. Burtt
Julie J. Burtt is a radiation biologist with the Canadian Nuclear Safety Commission. Her research focuses on the potential health effects of exposure to low doses of ionizing radiation. She has experience as an advisor for Canada to the United Nations Scientific Committee on the Effects of Atomic Radiation (UNSCEAR) as well as a technical expert for the UNSCEAR Secretariat. She also serves as vice-chair of the National Energy Agency’s Expert Group on Non-radiological Public Health Aspects of Radiation Emergency Planning and Response. Julie is also a member of Committee 4 for the International Commission on Radiological Protection.
Julie Leblanc
Julie Leblanc, Ph.D. is a radiation biologist with the Canadian Nuclear Safety Commission. Her research focuses on the potential health effects of exposure to low doses of ionizing radiation. She is a Canadian Delegate on the High-Level Group on Low Dose Research and International Commission on Radiological Protection mentee and member of task group 111.
Kristi Randhawa
Kristi Randhawa is a Radiation and Health Sciences Officer (epidemiology) with the Canadian Nuclear Safety Commission. Her research involves studying the health effects of ionizing radiation exposure, particularly exposure to low doses of radiation or radiation delivered at low dose-rates. She works as a coordinator and advisor for various research projects and is a technical expert to the United Nations Scientific Committee on the Effects of Atomic Radiation (UNSCEAR) Secretariat.
Knut Erik Tollefsen
Knut Erik Tollefsen, Ph.D., is a Chief Scientist at the Norwegian Institute for Water Research (NIVA) and an Adjunct professor at the Norwegian University of Life Sciences (NMBU). He is a Norwegian delegate of the HLG-LDR and OECD EAGMST, co-chairs the HLG-LDR Rad/Chem AOP Joint Topical Group and co-ordinates NIVA’s Computational Toxicology Program, NCTP (www.niva.no/nctp).
Carole L. Yauk
Carole Yauk was the lead scientist of the Genomics Laboratory in the Environmental Health Science and Research Bureau at Health Canada for 18 years. She joined the University of Ottawa’s Department of Biology as a professor in September 2020, where she holds the Canada Research Chair in Genomics and the Environment. Her research focuses on the development and implementation of genomic tools for human health risk assessment of environmental exposures. She is actively involved in various international committees to advance these fields.
References
- Adam N, Vuong NQ, Adams H, Kuo B, Beheshti A, Yauk C, Wilkins R, Chauhan V. 2022. Evaluating the Influences of confounding variables on benchmark dose using a case study in the field of ionizing radiation. Int J Radiat Biol. 98(12):1845–1855.
- Ainsbury EA, Bouffler SD, Dörr W, Graw J, Muirhead CR, Edwards AA, Cooper J. 2009. Radiation cataractogenesis: a review of recent studies. Radiat Res. 172(1):1–9.
- Alcaraz AJG, Baraniuk S, Mikulášek K, Park B, Lane T, Burbridge C, Ewald J, Potěšil D, Xia J, Zdráhal Z, et al. 2022. Comparative analysis of transcriptomic points-of-departure (tPODs) and apical responses in embryo-larval fathead minnows exposed to fluoxetine. Environ Pollut. 295:118667.
- Alcaraz AJG, Mikulášek K, Potěšil D, Park B, Shekh K, Ewald J, Burbridge C, Zdráhal Z, Schneider D, Xia J, et al. 2021. Assessing the toxicity of 17α-ethinylestradiol in rainbow trout using a 4-day transcriptomics benchmark dose (BMD) embryo assay. Environ Sci Technol. 55(15):10608–10618.
- Boobis AR, Cohen SM, Dellarco V, McGregor D, Meek ME, Vickers C, Willcocks D, Farland W. 2006. Ipcs framework for analyzing the relevance of a cancer mode of action for humans. Crit Rev Toxicol. 36(10):781–792.
- Boobis AR, Doe JE, Heinrich-Hirsch B, Meek ME, Munn S, Ruchirawat M, Schlatter J, Seed J, Vickers C. 2008. IPCS framework for analyzing the relevance of a noncancer mode of action for humans. Crit Rev Toxicol. 38(2):87–96.
- Chauhan V, Kuo B, McNamee JP, Wilkins RC, Yauk CL. 2016. Transcriptional benchmark dose modeling: exploring how advances in chemical risk assessment may be applied to the radiation field. Environ Mol Mutagen. 57(8):589–604.
- Chauhan V, Rowan-Carroll A, Gagné R, Kuo B, Williams A, Yauk CL. 2019. The use of in vitro transcriptional data to identify thresholds of effects in a human lens epithelial cell-line exposed to ionizing radiation. Int J Radiat Biol. 95(2):156–169.
- Chauhan V, Adam N, Kuo B, Williams A, Yauk CL, Wilkins R, Stainforth R. 2021a. Meta-analysis of transcriptomic datasets using benchmark dose modeling shows value in supporting radiation risk assessment. Int J Radiat Biol. 97(1):31–49.
- Chauhan V, Stricklin D, Cool D. 2021b. The integration of the adverse outcome pathway framework to radiation risk assessment. Int J Radiat Biol. 97(1):60–67.
- Chauhan V, Wilkins RC, Beaton D, Sachana M, Delrue N, Yauk C, O’Brien J, Marchetti F, Halappanavar S, Boyd M, et al. 2021c. Bringing together scientific disciplines for collaborative undertakings: a vision for advancing the adverse outcome pathway framework. Int J Radiat Biol. 97(4):431–441.
- Chauhan V, Beaton D, Hamada N, Wilkins R, Burtt J, Leblanc J, Cool D, Garnier-Laplace J, Laurier D, Le Y, et al. 2022a. Adverse outcome pathway: a path toward better data consolidation and global co-ordination of radiation research. Int J Radiat Biol. 98(12):1694–1703.
- Chauhan V, Hamada N, Garnier-Laplace J, Laurier D, Beaton D, Tollefsen KE, Locke PA. 2022b. Establishing a communication and engagement strategy to facilitate the adoption of the adverse outcome pathways in radiation research and regulation. Int J Radiat Biol. 98(12):1714–1721.
- Crizer DM, Ramaiahgari SC, Ferguson SS, Rice JR, Dunlap PE, Sipes NS, Auerbach SS, Merrick BA, DeVito MJ. 2021. Benchmark concentrations for untargeted metabolomics versus transcriptomics for liver injury compounds in in vitro liver models. Toxicol Sci. 181(2):175–186.
- Crump KS. 1984. A new method for determining allowable daily intakes. Fundam Appl Toxicol. 4(5):854–871.
- Davis JA, Gift JS, Zhao QJ. 2011. Introduction to benchmark dose methods and U.S. EPA’s benchmark dose software (BMDS) version 2.1.1. Toxicol Appl Pharmacol. 254(2):181–191.
- EFSA. 2017. Update: use of the benchmark dose approach in risk assessment. EFSA J. 15(1):e04658.
- Ewald J, Soufan O, Xia J, Basu N. 2021. Fastbmd: an online tool for rapid benchmark dose-response analysis of transcriptomics data. Bioinformatics. 37(7):1035–1036.
- Farmahin R, Gannon AM, Gagné R, Rowan-Carroll A, Kuo B, Williams A, Curran I, Yauk CL. 2019. Hepatic transcriptional dose-response analysis of male and female fischer rats exposed to hexabromocyclododecane. Food Chem Toxicol. 133:110262.
- Farmahin R, Williams A, Kuo B, Chepelev NL, Thomas RS, Barton-Maclaren TS, Curran IH, Nong A, Wade MG, Yauk CL. 2017. Recommended approaches in the application of toxicogenomics to derive points of departure for chemical risk assessment. Arch Toxicol. 91(5):2045–2065.
- Filipsson AF, Sand S, Nilsson J, Victorin K. 2003. The benchmark dose method–review of available models, and recommendations for application in health risk assessment. Crit Rev Toxicol. 33(5):505–542.
- Gibb S. 2008. Toxicity testing in the 21st century: a vision and a strategy. Reprod Toxicol. 25(1):136–138.
- Gwinn WM, Auerbach SS, Parham F, Stout MD, Waidyanatha S, Mutlu E, Collins B, Paules RS, Merrick BA, Ferguson S, et al. 2020. Evaluation of 5-day in vivo rat liver and kidney with high-throughput transcriptomics for estimating benchmark doses of apical outcomes. Toxicol Sci. 176(2):343–354.
- Haber LT, Dourson ML, Allen BC, Hertzberg RC, Parker A, Vincent MJ, Maier A, Boobis AR. 2018. Benchmark dose (BMD) modeling: current practice, issues, and challenges. Crit Rev Toxicol. 48(5):387–415.
- Harrill JA, Everett LJ, Haggard DE, Sheffield T, Bundy JL, Willis CM, Thomas RS, Shah I, Judson RS. 2021a. High-throughput transcriptomics platform for screening environmental chemicals. Toxicol Sci. 181(1):68–89.
- Harrill JA, Viant MR, Yauk CL, Sachana M, Gant TW, Auerbach SS, Beger RD, Bouhifd M, O'Brien J, Burgoon L, et al. 2021b. Progress towards an OECD reporting framework for transcriptomics and metabolomics in regulatory toxicology. Regul Toxicol Pharmacol. 125:105020.
- ICRP. 2012. ICRP publication 118: ICRP statement on tissue reactions and early and late effects of radiation in normal tissues and organs — threshold doses for tissue reactions in a radiation protection context. Ann ICRP. 41(1–2):1–322.
- Johnson KJ, Auerbach SS, Costa E. 2020. A rat liver transcriptomic point of departure predicts a prospective liver or non-liver apical point of departure. Toxicol Sci. 176(1):86–102.
- Krewski D, Acosta D, Andersen M, Anderson H, Bailar JC, Boekelheide K, Brent R, Charnley G, Cheung VG, Green S, et al. 2010. Toxicity testing in the 21st century: a vision and a strategy. J Toxicol Environ Health B. 13(2–4):51–138.
- Krewski D, Andersen ME, Tyshenko MG, Krishnan K, Hartung T, Boekelheide K, Wambaugh JF, Jones D, Whelan M, Thomas R, et al. 2020. Toxicity testing in the 21st century: progress in the past decade and future perspectives. Arch Toxicol. 94(1):1–58.
- Lucas J, Dressman HK, Suchindran S, Nakamura M, Chao NJ, Himburg H, Minor K, Phillips G, Ross J, Abedi M, et al. 2014. A translatable predictor of human radiation exposure. PLOS One. 9(9):e107897.
- Matteo G, Leingartner K, Rowan-Carroll A, Meier M, Williams A, Beal MA, Gagné M, Farmahin F, Wickramasuriya S, Reardon AJF, et al. 2022. In vitro transcriptomic analyses reveal pathway perturbations, estrogenic activities, and potencies of data-poor BPA alternative chemicals. Toxicol Sci. 191(2):266–275.
- Miousse IR, Murphy LA, Lin H, Schisler MR, Sun J, Chalbot MG, Sura R, Johnson K, LeBaron MJ, Kavouras IG, et al. 2017. Dose-response analysis of epigenetic, metabolic, and apical endpoints after short-term exposure to experimental hepatotoxicants. Food Chem Toxicol. 109(Pt 1):690–702.
- Mittal K, Ewald J, Basu N. 2022. Transcriptomic points of departure calculated from rainbow trout gill, liver, and gut cell lines exposed to methylmercury and fluoxetine. Environ Toxicol Chem. 41(8):1982–1992.
- Moe SJ, Wolf R, Xie L, Landis WG, Kotamäki N, Tollefsen KE. 2021. Quantification of an adverse outcome pathway network by bayesian regression and bayesian network modeling. Integr Environ Assess Manag. 17(1):147–164.
- NCRP. 2020. Approaches for Integrating Information from radiation biology and epidemiology to enhance low-dose health risk assessment. Bethesda (MD): National Council on Radiation Protection and Measurements. NCRP Report No.: 186.
- OECD. 2017. Guidance document for the use of adverse outcome pathways in developing integrated approaches to testing and assessment (IATA). Series on testing and assessment no. 260. Paris France: Environment, Health and Safety, Environment Directorate, OECD.
- OECD. 2018. Users’ handbook supplement to the guidance document for developing and assessing adverse outcome pathways. OECD Series on adverse outcome pathways, no 1. Paris France: Environment, Health and Safety, Environment Directorate, OECD.
- Olesti E, González-Ruiz V, Wilks MF, Boccard J, Rudaz S. 2021. Approaches in metabolomics for regulatory toxicology applications. Analyst. 146(6):1820–1834.
- Pagé-Larivière F, Crump D, O'Brien JM. 2019. Transcriptomic points-of-departure from short-term exposure studies are protective of chronic effects for fish exposed to estrogenic chemicals. Toxicol Appl Pharmacol. 378:114634.
- Phillips JR, Svoboda DL, Tandon A, Patel S, Sedykh A, Mav D, Kuo B, Yauk CL, Yang L, Thomas RS, et al. 2019. Bmdexpress 2: enhanced transcriptomic dose-response analysis workflow. Bioinformatics. 35(10):1780–1782.
- Preston RJ. 2015. Integrating basic radiobiological science and epidemiological studies: why and how. Health Phys. 108(2):125–130.
- Qutob SS, Chauhan V, Kuo B, Williams A, Yauk CL, McNamee JP, Gollapudi B. 2018. The application of transcriptional benchmark dose modeling for deriving thresholds of effects associated with solar-simulated ultraviolet radiation exposure. Environ Mol Mutagen. 59(6):502–515.
- Rager JE, Auerbach SS, Chappell GA, Martin E, Thompson CM, Fry RC. 2017. Benchmark dose modeling estimates of the concentrations of inorganic arsenic that induce changes to the neonatal transcriptome, proteome, and epigenome in a pregnancy cohort. Chem Res Toxicol. 30(10):1911–1920.
- Ramaiahgari SC, Auerbach SS, Saddler TO, Rice JR, Dunlap PE, Sipes NS, DeVito MJ, Shah RR, Bushel PR, Merrick BA, et al. 2019. The power of resolution: contextualized understanding of biological responses to liver injury chemicals using high-throughput transcriptomics and benchmark concentration modeling. Toxicol Sci. 169(2):553–566.
- Reardon AJF, Rowan-Carroll A, Ferguson SS, Leingartner K, Gagne R, Kuo B, Williams A, Lorusso L, Bourdon-Lacombe JA, Carrier R, et al. 2021. Potency ranking of per- and polyfluoroalkyl substances using high-throughput transcriptomic analysis of human liver spheroids. Toxicol Sci. 184(1):154–169.
- Rowan-Carroll A, Reardon A, Leingartner K, Gagné R, Williams A, Meier MJ, Kuo B, Bourdon-Lacombe J, Moffat I, Carrier R, et al. 2021. High-throughput transcriptomic analysis of human primary hepatocyte spheroids exposed to per- and polyfluoroalkyl substances as a platform for relative potency characterization. Toxicol Sci. 181(2):199–214.
- Sand S, Victorin K, Filipsson AF. 2008. The current state of knowledge on the use of the benchmark dose concept in risk assessment. J Appl Toxicol. 28(4):405–421.
- Slob W. 2017. A general theory of effect size, and its consequences for defining the benchmark response (BMR) for continuous endpoints. Crit Rev Toxicol. 47(4):342–351.
- Stainforth R, Vuong N, Adam N, Kuo B, Wilkins RC, Yauk C, Beheshti A, Chauhan V. 2022. Benchmark dose modeling of transcriptional data: a systematic approach to identify best practices for study designs used in radiation research. Int J Radiat Biol. 98(12):1832–1844.
- Thomas RS, Allen BC, Nong A, Yang L, Bermudez E, Clewell HJ III, Andersen ME. 2007. A method to integrate benchmark dose estimates with genomic data to assess the functional effects of chemical exposure. Toxicol Sci. 98(1):240–248.
- Thomas RS, Philbert MA, Auerbach SS, Wetmore BA, Devito MJ, Cote I, Rowlands JC, Whelan MP, Hays SM, Andersen ME, et al. 2013a. Incorporating new technologies into toxicity testing and risk assessment: moving from 21st century vision to a data-driven framework. Toxicol Sci. 136(1):4–18.
- Thomas RS, Wesselkamper SC, Wang NC, Zhao QJ, Petersen DD, Lambert JC, Cote I, Yang L, Healy E, Black MB, et al. 2013b. Temporal concordance between apical and transcriptional points of departure for chemical risk assessment. Toxicol Sci. 134(1):180–194.
- USEPA. 2012. Benchmark dose technical guidance, EPA/100/R-12/001. Washington (DC): Risk Assessment Forum US Environmental Protection Agency.
- Vaiserman A, Cuttler JM, Socol Y. 2021. Low-dose ionizing radiation as a hormetin: experimental observations and therapeutic perspective for age-related disorders. Biogerontology. 22(2):145–164.
- Wang Z, Walker GW, Muir DCG, Nagatani-Yoshida K. 2020. Toward a global understanding of chemical pollution: a first comprehensive analysis of national and regional chemical inventories. Environ Sci Technol. 54(5):2575–2584.
- Webster AF, Chepelev N, Gagné R, Kuo B, Recio L, Williams A, Yauk CL. 2015. Impact of genomics platform and statistical filtering on transcriptional benchmark doses (BMD) and multiple approaches for selection of chemical point of departure (POD). PLOS One. 10(8):e0136764.
- Wheeler MW, Bailer AJ. 2007. Properties of model-averaged BMDLs: a study of model averaging in dichotomous response risk estimation. Risk Anal. 27(3):659–670.
- Wheeler MW, Bailer AJ. 2009. Benchmark dose estimation incorporating multiple data sources. Risk Anal. 29(2):249–256.
- Yang L, Allen BC, Thomas RS. 2007. Bmdexpress: a software tool for the benchmark dose analyses of genomic data. BMC Genomics. 8(1):387.
- Yao CH, Wang L, Stancliffe E, Sindelar M, Cho K, Yin W, Wang Y, Patti GJ. 2020. Dose-response metabolomics to understand biochemical mechanisms and off-target drug effects with the TOXcms software. Anal Chem. 92(2):1856–1864.
- Zheng L, Yan X, Suchindran S, Dressman H, Chute JP, Lucas J. 2014. Biological pathway selection through bayesian integrative modeling. Stat Appl Genet Mol Biol. 13(4):435–457.