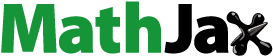
Abstract
Aims
Six Delta is a six-dimensional independent platform for outcome-based pricing/contracting. The second dimension (δ2) estimates prices on the basis of four willingness-to-pay (WTP) thresholds. We describe this dimension’s methodology and present a proof-of-concept application to the treatment of non-small cell lung cancer (NSCLC) with EGFR mutation with osimertinib.
Materials and methods
Eight WTP scenarios based on four levels of real gross domestic product per capita (<1GDP/capita, 1 × GDP/capita, 3 × GDP/capita, and >3 × GDP/capita) and two market conditions (monopolistic versus competitive) were assumed. The incremental cost-utility ratio (ICUR) was applied to differently to both markets. In the monopolistic market, assuming no competitors, the cost/QALY ratio for a drug was used; whereas in the competitive market, assuming competitors, the incremental cost-utility ratio (ICUR) was applied. One-way sensitivity analyses were performed and predictive equations were specified to estimate the prices of treatment for the resulting eight WTP scenarios; for which subsequently the average and standard deviation were calculated. A gamma distribution was specified and Monte Carlo Simulation (MCS) was applied to estimate the dimension-specific price based on WTP (DSPWTP). A proof-of-concept exercise with osimertinib in NSCLC was performed for two hypothetical outcome-based contracts: 1-year (2019–2020) and 2-year (2019–2021). The 2018 wholesale acquisition cost (WAC) of $14,616 (30-day prescription) was used to estimate the DSPWTP for each contract.
Results
The 1-year estimates averaged $4,654 (SD=$6,462) and the MCS yielded a DSPWTP of $4,547 or −68.89% of the 2018 WAC for a 30-day prescription. The 2-year estimates averaged $4,7667 (SD=$6,480) with the MCS generating a DSPWTP of $4,704 or −67.82% of the WAC.
Conclusions
We demonstrated that WTP-based methods that include various WTP thresholds and market conditions generate price estimates across these thresholds and market conditions that can be integrated into our proposed Six Delta platform for outcome-based pricing/contracting.
Introduction
In our previous articleCitation1 on δ1, we used cost-effectiveness analysis (CEA) and cost-utility analysis (CUA) to estimate a dimensional-specific price (DSPCEA/CUA) for osimertinib when probabilistic sensitivity analysis was considered for pricing. In this second dimension of the Six Delta platform, we use the base case analysis, moreover limited to CUA, to estimate DSPWTP, which is based on different scenarios for willingness-to-pay (WTP) thresholds per quality-adjusted life year (QALY) gained.
Estimating a price for a treatment at a given WTP/QALY gained, using base case analyses, is a conventional method in settings in which a specific WTP is specified; for instance, in single-payer systems. In the US, an omnibus WTP has not been set, mainly because the US health system is a mixed payer-system and applying a specific WTP to estimate a price for a drug is challenging and may not be generalizable to all payers. More generally, to enable drug pricing in resource-constrained countries and markets, the World Health Organization (WHO) Commission on Macroeconomics and Health has proposed a WTP/QALY gained threshold range of 1× to 3× the gross-domestic product (GDP) per capitaCitation2,Citation3, with the 1 × GDP/capita applying to low-income and the 3 × GDP/capita applying to high-income countries. The WHO recommended thresholds have been used empiricallyCitation2. We propose to use the range of 1–3 × GDP to set prices in our Six Delta platform. However, we also believe that some countries’ resources are so constrained that the lower bound of the WHO’s suggested range may still be too high and unaffordable. Hence we suggest that <1 × GDP/QALY gained should be considered as a scenario along the WHO’s prespecified 1–3 × GDPCitation2,Citation3. In addition, we recognize that the pricing freedom in the US has significantly increased prices for drugs and other health care services and takes the WTP/QALY gained threshold beyond the 3 × GDP upper bound proposed by the WHO. Here too we proposed to add a > 3 × GDP/QALY gained scenario along the WHO’s pre-specified 1–3 × GDPCitation2,Citation3.
In this study, we aim to optimize pricing information derived from CUA base case results for outcome-based pricing. In the first dimension of our platformCitation1, we developed a pricing method based on the cost-effectiveness acceptability curve (CEAC) generated by probabilistic sensitivity analyses for the incremental cost-effectiveness ratio (ICER) and incremental cost-utility ratio (ICUR). In the second dimension presented herein the focus is on optimizing the pricing information gained from the CUA base case results to estimate eight price scenarios resulting from crossing four scenarios for WTP with two market conditions: a monopolistic market (i.e. the drug has no competition, has patent protection, and/or has market exclusivity) and a competitive market (i.e. the drug has in-class or standard of care competition and/or no longer has patent protection or market exclusivity). Subsequently, the eight estimates are pooled, averaged, and simulated using the method of MCS to derive the DSPWTP. Additional market conditions can be integrated to generate mor pricing scenarios in this dimension.
Readers are encouraged to review the form of model inputs selection (Supplemental Material) in which outcome-based contractors can choose model scenarios, market conditions, and other inputs of interest.
Model
Overview
Pricing based on WTP requires a CUA be performed, which are reported in the δ1 paper in this seriesCitation1. In the WTP-based pricing algorithm, the base case results of the CUA are used to examine a set of WTP scenarios. Using the above referenced WHO WTP thresholds and the monopolistic and competitive market conditions. In the monopolistic market analysis, where a treatment is the only available, no treatment comparator is assumed and the base case cost/QALY gained was used for pricing the treatment of interest. On the other hand, in the competitive market analysis, a treatment comparator is assumed in the base case ICUR results were used for pricing the treatment of interest. Given these WTP scenarios and market conditions, we specified mathematical equations to generate prices for each of the eight scenarios. Subsequently, the eight prices were averaged and a gamma distribution was specified, and MCS was used to derive the DSPWTP. MCS is a technique for estimating summary statistics for a population by sampling a dataset with replacement. In a large number of iterations within a specified distribution. The summary estimate is calculated by averaging the results of iterationsCitation3. In our study, gamma distribution was specified for the pooled price scenarios because monetary inputs like price can range in value from 0 to +∞Citation4.
A proof-of-concept analysis performed using osimertinib in treating NSCLC with EGFR mutation. The aim of this proof-of-concept was to assess the price of a 30-day prescription of osimertinib by using the 2018 wholesale acquisition cost (WAC). In the proof-of-concept analysis, two hypothetical outcome-based contracts were generated for 1-year contract (2019–2020) and a 2-year contract (2019–2021).
WTP-based pricing methodology ()
Base case analysis ICUR
We used the base case results of cost/QALY gained (without a comparator) for the monopolistic market condition and the ICUR (with comparator) for the competitive market condition from our δ1 analysisCitation1 and matched these base case results with four GDP scenarios to estimate prices: <1 × GDP per capita, 1 × GDP per capita, 3 × GDP per capita, >3 × GDP per capita.
One-Way sensitivity analyses and predictive equations
More generally, in the δ2 dimension conventional one-way sensitivity analyses are performed to assess how changes in the base case cost/QALY gained and ICUR affect price estimates. These analyses can be performed with downward or upward changes in the price of the treatment of interest and examining how this affects the estimated cost/QALY gained (monopolistic market) and ICUR (competitive market). For each upward or downward adjustment, the base case cost/QALY gained and ICUR are recalculated. Because the one-way sensitivity analyses are performed on a single estimate (i.e. price of the treatment of interest), two linear predictive equations are applied to estimate the base case cost/QALY gained and ICUR. The common form these two equations is
(1)
(1)
where y is the base case cost/QALY gained (monopolistic market) or base case ICUR (competitive market), x represents the percentage of linear reduction or increase in price of treatment, m is the slope, and b is the intercept. Notably, y as the base case cost/QALY gained or ICUR equals b if there is no change in the price of the treatment of interest (x is zero).
Estimation of percentage (%) of price adjustment
Using the assumed GDP per capita as WTP scenarios in the EquationEquation (1)(1)
(1) , once with base case cost/QALY gained (monopolistic market) and once with base case ICUR (competitive market), the resulting predictive equations are used to predict the percentage (%) of price adjustment for a treatment:
(2)
(2)
where GDP per capita scenario is whether <1×, 1×, 3×, >3 × GDP per capita, b is the intercept, and m is the slope.
Price adjustment
Next, the marketed price (e.g. WAC) of the drug of interest is recalculated using the % of price adjustment and applying the percentage of medical inflation rate that is represented by medical consumer price index (mCPI) for prescription medication for a given year. This yields the adjusted price of the drug in a specific year.
(3)
(3)
where Year Z marketed price of drug is the price of drug set by the manufacturer in year Z, mCPI is the medical consumer price index. The mCPI is retrieved and projected from the US Bureau of Labor StatisticsCitation5.
Resulting adjusted prices
By replacing the four WTP thresholds (<1 × GDP, 1 × GDP, 3 × GDP, >GDP per capita) with the respective base case cost/QALY gained (monopolistic market) and ICUR (competitive market), four different price estimates are generated for each of market environment. The total number of prices generated is eight.
MCS to estimate price in a random pattern of variation
Because these price estimates are based on four different WTP values and for two market conditions, the variance between these estimates may be significant. MCS can be applied to refer this variance in prices to a random pattern of price variations. Thus, MCS can derive a single estimate after averaging the eight prices and specifying a gamma distribution for price variations. MCS is a parametric method that empirically involves large numbers of iterations (e.g. 1000 or 2000) to estimate the shape of the statistical distribution of varying drug pricesCitation4. The following equations are used to specify the parameters for gamma distributionCitation4:
(4)
(4)
(5)
(5)
where ɑ is alpha; β is beta; X̄ is average price; SD is standard deviation
Proof-of-concept: application to osimertinib in NSCLC with EGFR mutation
Background
As an application of the suggested WTP-based pricing dimension, we aimed to perform price assessment for osimertinib, a third generation, irreversible EGFR tyrosine kinase inhibitor (TKI) in NSCLC. Osimertinib was approved in 2018, based on the results of the FLAURA clinical trialCitation6 and priced at approximately two times the first- and second-generation EGFR-TKIs gefitinib, erlotinib, and afatinibCitation7. In our price assessment for osimertinib, we used the Alkhatib et al.Citation1 reported base case results of cost/QALY gained and ICUR for osimertinibCitation1. In the Alkhatib et al.Citation1 analysis, the 2018 WAC price of osimertinib was used at $14,616 the base case cost/QALY gained was $2,45,644/QALY gained, and the ICUR was $2,02,574/QALY gainedCitation1. In addition to the proof-of-concept, two hypothetical outcome-based contracts were assumed for, respectivey, a 1-year contract (2019–2020) and a 2-year contract (2019–2021).
Model inputs
As shown in , base case cost/QALY gained and ICUR estimates were retrieved from Alkhatib et al.Citation1. The osimertinib price was $14,616 based on 2018 WAC. The base case CUA applied to the price assessment of osimertinib for NSCLC with EGFR mutation () showed that osimertinib resulted in a gain of 2.91 QALYs at a lifetime cost of $7,14,826 and SOC resulted in a gain of 1.68 QALYs at a lifetime cost of $4,65,660. The resultant base case cost/QALY gained, assuming a monopolistic market, was $2,45,644/QALY gained. The resultant base case ICUR, assuming a competitive market, was $2,02,574/QALY gained. A range of WTP per QALY was assumed at <1×, 1×, 3×, >3× the GDP per capita. The 2018 GDP was extracted from the World Bank (<1 × GDP per capita at <$56,455, 1 × GDP per capita at $56,455; 3 × GDP per capita at $1,69,365; >3GDP per capita at >$169,365)Citation8 and subjected to a projected growth rate of 2.9% as per the World Bank to estimate GDP in 2019. No inflation adjustment was to the GDPs from 2019 to 2020 for the 1-year contract and from 2019 to 2021 for the 2-year contractCitation9. The seasonally adjusted mCPI specified for prescription drugs was extracted from the US Bureau of Labor StatisticsCitation5 and was projected by averaging the 2014–2018 mCPI estimates to increase by 0.44% during the 1-year contract in 2019–2020 and by 0.72% during the 2-year contract in 2019–2021 ().
Table 1. Model inputs.
Analysis
The base case cost/QALY gained (for monopolistic market) and ICUR (for competitive market) were used to generate eight possible prices for osimertinib under consideration of WTP thresholds of <1 × GDP per capita, 1 × GDP per capita, 3 × GDP per capita and >3 × GDP per capita. One-way sensitivity analyses were performed by assessing the effect of a 10% reduction in the price of osimertinib on the base case cost/QALY gained and ICUR estimates. Then, two predictive linear equations to estimate three prices at the three suggested GDP scenarios were generated for both the monopolistic and competitive market conditions. The eight generated prices were then inflated as needed for the 1-year contract in 2019–2020 and the 2-year contract in 2019–2021. This yielded a pool of eight prices resulting. The average and standard deviation (SD) were calculated and used to find the ɑ and β gamma distributional functions for the pooled prices. Subsequently, MCS with 2000 iterations was performed to generate a random price at the dimensional level (i.e. DSPWTP) (See Supplemental Materials for a description of our approach in selecting the number of iterations. Analyses were performed in Microsoft Excel 365Footnotei with MSO supported coding of Visual Basic for applications.
Results
WTP-Projections
As shown in , 1.96% growth rate on GDP per capita was applied to the 2018 GDP per capita estimates to estimate the 2019 GDP per capita. For both hypothetical contracts, <1 × GDP was <$58,122 per capita, 1 × GDP was $58,122 per capita, 3 × GDP was $1,74,366 per capita, >3 × GDP was >$174,366 per capita.
WTP-based pricing
The one-way sensitivity analyses performed by estimating the base case cost/QALY gained and ICUR at every additional 10% price reduction of the marketed price of osimertinib generated two linear equations as shown in :
Figure 1. Flowchart for the δ2 price analysis. Abbreviations. ICUR, incremental-cost effectiveness ratio; GDP per capita, gross domestic product per capita; WTP, willingness-to-pay; QALY, quality-adjusted life year gained; DSPWTP, dimension-specific price based on willingness-to-pay

Figure 2. One-way sensitivity analyses and predictive equations: (A) monopolistic market; (B) competitive market. Abbreviations. QALY, quality-adjusted life year gained; ICUR, incremental-cost effectiveness ratio.

For the monopolistic market price, shows the linear reduction in cost/QALY gained estimates for every 10% reduction of the marketed price of osimertinib. According to the predictive equation, the % of price adjustment for osimertinib was generated as follows:
For the competitive market, shows the linear reduction in ICUR estimates for every 10% reduction of the marketed price of osimertinib. According to the predictive equation, the % of price adjustment for osimertinib was generated as follows:
In each hypothetical contract, eight prices were generated and inflated with mCPIs that were projected for 1-year contract (2019–2020) and was 0.44%; for 2-year contract (2019–2021) and was 0.72%. these projections were performed by averaging the mCPI estimates from 2014–2018 as per the US Bureau of Labor StatisticsCitation5.
As shown in , for the 1-year contract, the inflated WAC of osimertinib was $14,680 and the eight price estimates for osimertinib were: $0 in both monopolistic and competitive markets at <1 × GDP per capita of <$58,122; $0 in both monopolistic and competitive markets at 1 × GDP per capita of $58,122; $2,592 in monopolistic and $5,281 in competitive market at 3 × GDP per capita of $1,74,366; $14,680 in both markets at >3 × GDP per capita (>$174,366).
Table 2. Estimated prices of osimertinib for two hypothetical contracts (2019–2020 and 2019–2021) at osimertinib 2018 WAC price of $14,616 for a 30-day prescription.
For the 2-year contract, the inflated WAC of osimertinib was $14,721 and the eight price estimates for osimertinib were: $0 in both monopolistic and competitive markets at 1 × GDP per capita of <$58,122; $0 in both monopolistic and competitive markets at 1 × GDP per capita of $58,122; $2,599 in monopolistic and $5,296 in competitive market at 3 × GDP per capita of $1,74,366; $14,721 in both markets at >3 × GDP per capita (>$174,366).
The 1-year contract price estimates were averaged at $4,654 (SD=$6,462), and the DSPWTP was at $4,547 or −68.89% the 2018 US osimertinib price. The 2-year contract price estimates were averaged at $4,667 (SD=$6,480), and the DSPWTP was $4,704 or −67.82% of the 2018 US osimertinib price.
Discussion
Building upon the CEA and CUA performed as part of dimension δ1, we presented here dimension δ2 that applies the CEA and CUA findings, aligns them with WTP thresholds, and provides decision-making support. Specifically, we provided price assessments under four possible WTP scenarios (<1 × GDP per capita, 1 × GDP per capita, 3 × GDP per capita, >3 × GDP per capita) and under two market conditions (monopolistic, and competitive). For the monopolistic market, the metric of interest was the case base cost/QALY gained estimate for the treatment of interest and without comparator(s); for the competitive market, the metric was the base case ICUR estimate. Eight price scenarios were generated and integrated, and their average and SD were calculated to specify a gamma distribution in which MCS was used to derive the DSPWTP.
Statistically, the metrics (base case cost/QALY gained and ICUR estimates) were subjected to one-way sensitivity analyses in which the marketed price of treatment was arbitrarily changed to assess the change in these metrics. Because these one-way sensitivity analyses were performed by manipulating a single model input (i.e. marketed price of the treatment of interest), changes in the metrics of interest were linear and generated two linear predictive equations, one for each of the metrics (See also Supplemental Material). These two equations were subsequently used to estimate the % of price adjustment at the four WTP levels in the two market conditions. This, in turn, yielded eight adjusted prices, which were then adjusted to consider future inflation. As noted, mean and SD of these prices were calculated to specify a gamma distribution and MCS was performed to estimate the dimensional price DSPWTP. The proof-of-concept analysis initiated in the δ1 was confirmed in δ2 and used to model 1-year (2019–2020) and 2-year (2019–2021) outcome-based contracts.
In the 1-year contract, the price of osimertinib was $0 at <1 × GDP per capita for both market conditions; $0 at 1 × GDP per capita for both market conditions; $2,592 assuming a monopolistic market and $5,281 assuming a competitive market at 3 × GDP per capita; and $14,680 for both conditions at >3 × GDP per capita. In the 2-year contract, the price of osimertinib was $0 at <1 × GDP per capita for both market conditions; 0$for both conditions at 1 × GDP per capita; $2,599 assuming a monopolistic market and $5,296 assuming a competitive market at 3× GDP per capita; $14,721 for both conditions at >3 × GDP per capita. These results show some interesting dynamics. First, the price of osimertinib was reduced to $0 at <1GDP per capita and 1 × GDP per capita as long as the base case cost/QALY gained and ICUR remained above 1 × GDP per capita. At these WTP thresholds, osimertinib is not affordable even if its acquisition is zero and after adjustment for inflation because of the other costs’ association with treating NSCLC. The cost of treating the NSCLC itself already exceeded the 1 × GDP per capita before the approval of osimertinib. Second, at 3 × GDP per capita, a competitive market required less price reduction than a monopolistic market for osimertinib to meet the WTP threshold of 3 × GDP per capita. This supports the general real-world observation that pricing strategies require higher prices in a competitive market to salvage profits lost to competing products and declining market share.
After the MCS, the 1-year contract yielded a decrease of −68.89% of the 2018 price of osimertinib, thus suggesting the DSPWTP of $4,547 for a 30-day prescription; the 2-year contract yielded a decrease of −67.82% of the 2018 price of osimertinib, thus suggesting the DSPWTP of $4,704 for a 30-day prescription. The 1.07% high prices in the 2-year price compared to the 1-year price is due to a random effect of inflation rate between the price scenarios.
Our proposed method for WTP-based pricing, by itself but specifically as one of the six dimensions of our platform, contributes significantly to outcome-based pricing. The transparency of the methods enables payers to consider varying WTP thresholds and to match estimates of drug prices with these WTP thresholds. We assumed WTP scenarios that go below and beyond the WHO-recommended WTP scale of 1–3 × GDP per capitaCitation2,Citation3, but also covers unbound thresholds <1 × GDP per capita and >3GDP per capita. The latter might be better aligned with pharmaceutical free markets like the US and other high-income countriesCitation2,Citation3.
Further, the inclusion of monopolistic and competitive market conditions improves the generalizability of methods and results by emulating and balancing first-in-class and later-in-class pharmaceutical products. Statistically, the results of one-way sensitivity analyses were not the end but instead a means for developing predictive linear equations to estimate the price for a drug at any WTP. Using clinical trial data in the proof-of-concept exercise, while awaiting real-word data and reconciling these with the trial evidence, provides a transitional buffer against opportunistic pricing.
Of note, we aimed to provide greater price dispersion by integrating eight price scenarios and simulating this higher level of dispersion using MCS under a gamma distribution to achieve more plausible price adjustments for a given treatment. Conceptually, the higher level of dispersion in this study was justifiable because of the imperfect information about specific WTP thresholds in the US market.
Our proposed δ2 method has some limitations. Our MCS was limited to 2000 iterations. This may be considered low by some, however no significant fluctuation in results was observed when iterations exceeded 2000. Our study used clinical trial data to provide a price assessment in the proof-of-concept exercise. The price elasticity shown in this exercise may not fully reflect treatment effects in real-world oncology practice. Relatedly, we relied on the theory of random pattern of price variation because of the imperfect information inherent to clinical trial data, the absence of real-world data, yet also the appropriateness for estimating short term contractsCitation10,Citation11. In our study, we used the real GDP in our proof-of-concept exercise which might be debatable. However, our aim was to provide feasibility testing to the dimensional technicalities. Other indicators than the real GDP can be added to this dimension. We encourage contractors to set this discussion upfront and use the preferred indicator (See Supplemental Material for Form of Model Inputs Selection). Future pricing efforts are encouraged to involve higher price dispersion based on different attributes, assumptions, and scenarios; all of which may reflect the real market dynamics and interests of patients, payers and drug manufacturers.
Conclusion
Our methods for WTP-based pricing are include various WTP thresholds and market conditions. Results can be further improved by integrating the price scenarios in this second dimension of the Six Delta platform with other dimensions that reflect more assumptions and interests, as we will show in other papers in this series. In the interim, we demonstrated that the generate price estimates in this dimension can be integrated into our proposed six-dimensional platform for outcome-based contracting.
Transparency
Declaration of funding
The work reported herein was performed without sponsorship or grant funding.
Disclosure of financial/other interests
The authors have no financial relationships to declare.
JME peer reviewers on this manuscript have no relevant financial or other relationships to disclose.
Author contributions
All named authors meet the International Committee of Medical Journal Editors (ICMJE) criteria for authorship of this manuscript.
JME-2020-0094-RT.R1_SUPPLEMENT_CATS.docx
Download MS Word (22.7 KB)Acknowledgements
No assistance was received in the preparation of this article. Sandipan Bhattacharjee is now at the University of Texas at Austin.
Notes
i Microsoft Excel 365 is a registered trademark of Microsoft Research Lab, Redmond, Washington, DC, USA.
References
- Alkhatib NS, Erstad B, Ramos K, et al. Pricing methods in outcome-based contracting-δ1: cost-effectiveness analysis and cost utility analysis-based pricing. J Med Econ. 2020;23(11):1215–1222.
- Woods B, Revill P, Sculpher M, et al. Country-level cost-effectiveness thresholds: initial estimates and the need for further research. Value Health. 2016;19(8):929–935.
- World Health Organization. The world health report 2002: reducing risks, promoting healthy life. Geneva. 2002; [cited 2020 Jul 27]. Available from: https://www.who.int/whr/2002/en/.
- Briggs A, Claxton K, Sculpher M. Decision modelling for health economic evaluation. New York (NY): Oxford University Press; 2011.
- U.S. Bureau of Labor Statistics. Prescription drugs in U.S. city average, all urban consumers, seasonally adjusted; [cited 2019 Dec 27]. Available from: https://data.bls.gov/timeseries/CUSR0000SEMF01?output_view=pct_3mths.
- Soria J, Ohe Y, Vansteenkiste J, et al. Osimertinib in untreated EGFR-mutated advanced non–small-cell lung cancer. N Engl J Med. 2018;378(2):113–125.
- RedBook Online [subscription database online]. Greenwood Village, CO: Truven Health Analytics, Inc. updated periodically; [cited 2019 Dec 27]. Available from: http://micromedex.com/products/product-suites/clinical-knowledge/redbook.
- The World Bank. World bank national accounts data, and OECD national accounts data files; [cited 2019 Dec 27]. Available from: https://data.worldbank.org/indicator/NY.GDP.PCAP.CD?locations=US.
- Thokala P, Ochalek J, Leech AA, et al. Cost-effectiveness thresholds: the past, the present and the future. Pharmacoeconomics. 2018;36(5):509–522.
- Griffith D. Four types of price variation. Presented in March 2013; Laramie, WY; [cited 2019 Dec 27]. Available from: http://www.uwagec.org/rightrisk/presentations/2013_03_26_DGriffithMarketing/1_Introduction/1_FourTypesPriceVariation.pdf.
- Bodoh-Creed A, Boehnke J, Hickman B. Using machine learning to predict price dispersion. Scholars at HARVARD; [cited 2019 Dec 27]. Available from: https://scholar.harvard.edu/files/boehnke/files/bcbh_machine_learning_price_dispersion.pdf.