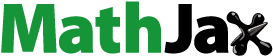
Abstract
Aims
Six Delta is a six-dimensional independent platform for outcome-based pricing/contracting. The fourth dimension (δ4) estimates prices on the basis of assessments of the safety of the drug using an ex ante analysis based on clinical trial data. We describe this dimension’s methodology and present a proof-of-concept application to the treatment of non-small cell lung cancer (NSCLC) with EGFR mutation with osimertinib.
Materials and methods
The safety-based pricing dimension utilizes a four-step method: 1) pooling adverse events (AE), standardizing, estimating 95%Cis, and adjusting for time; 2) estimating correction factors and corrected probabilities of AEs; 3) estimating the probability of at least one adverse event (AE) occurring and leading to treatment discontinuation; and 4) estimating ranges for payback percentages and performing Monte Carlo Simulation to estimate a DSPSafety. A proof-of-concept exercise with osimertinib in NSCLC was performed for two hypothetical outcome-based contracts: 1-year (2019–2020) and 2-year (2019–2021). We estimated the DSPSafety based on the grade 3/4 AEs observed for osimertinib and standard of care. The 2018 wholesale acquisition cost (WAC) of osimertinib at $14,616 for a 30-day prescription was used.
Results
AEs3/4 were retrieved from the FLAURA trial. In the 1-year contract, the DSPSafety of osimertinib was estimated at $14,627 (or +0.08% the 2018 WAC) for a 30-day prescription. In the 2-year contract, the DSPSafety of osimertinib was estimated at $14,516 (or −0.68% the 2018 WAC) for a 30-day prescription.
Conclusions
We demonstrated that ex ante pricing methods-based paybacks for safety issues leading to treatment discontinuation can be integrated into our proposed Six Delta platform for outcome-based pricing/contracting.
Introduction
Previously, we provided methods to estimate dimension-specific price (DSP) for three dimensions: δ1. Cost-effectiveness analysis and cost-utility analysis-based pricingCitation1; δ2. Willingness-to-pay-based pricingCitation2; δ3. Reference-based pricingCitation3. In this study, we aim to develop methods to estimate safety-based DSP (DSPSafety).
Systematic methods for contracting that consider safety outcomes are lacking even though some current outcome-based contracts are relating percentages of payback to safety measuresCitation4 but do so in a seemingly nonsystematic way and without transparency and the ability to verify and validate. In these contracts, manufacturers claim to provide paybacks to payers when the rate of adverse events (AE) associated with their treatment exceeds what was reported in clinical trials or observed in real-world settings. However, how this is done is a matter of private negotiation. Further, focusing on observed rates ignores the fact that these are sampled data, may be biased, and are not conditional for use in probabilistic models that can be generalized across settings, providers, patient population, and payers. Thus, methods to probabilistically model the risk of adverse events to exceed the evidence-based threshold are needed. In addition, these methods should be reproducible, inclusive of safety outcomes of treatment alternatives, and adaptable to use either clinical trial data or real-world data (RWD).
Specifically, in this study, we aimed to guide price assessment by using safety data from clinical trials; though our proposed methods can also rely on RWD to support pricing methods. We propose a method for safety-based pricing wherein a percentage of the treatment price is paid back because a magnitude of safety risk is captured that exceeds a pre-specified criterion or threshold. In our proposed methods, we define safety risk as any serious AE resulting from treatments as reported from clinical trials or from routine clinical practice studies. We propose a 4-step method for safety-based pricing assessment that can be integrated into our six-dimensional platform for outcome-based contracting. Additionally, we apply this method in a proof-of-concept application in cancer; specifically, treatment with osimertinib versus standard of care (SOC) in patients with NSCLC and EGFR mutation. The analyses are from the perspective of both payer and manufacturer.
Model
Overview
Clinical trials inform about AEs observed over the course of trial in the treatment arms. The frequencies (and percentages) with these AEs that are reported provide absolute thresholds that could serve as acceptable limits if a manufacturer and a payer agree to negotiate an outcome-based contract based on the safety of a given treatment especially if it leads to treatment discontinuation and therefore likely to affect efficacy. The AE crude rates from one or more trials serve as thresholds that trigger a payback guarantee by the manufacturer to the payer when the rate of AE-related treatment discontinuation exceeds the rate reported in trials. The percentage of payback can be estimated using our 4-step method for safety assessment ().
Safety-based pricing methodology
Step 1. Pooling AEs, standardization, estimating 95%Cis, and adjusting for time
In the first step, probabilities of AEs are pooled for each of the treatment arms in a clinical trial, standardized to one sample size, analyzed to estimate confidence intervals (95%CI), and adjusted to a specific time period (i.e. time of contract) (). Generally, AEs associated with treatments in a clinical trial are standardized to an equal sample size of patients in each arm. This process of standardization involves using the sample size of one treatment and estimating the relative AE proportions for the comparator treatment. This can be achieved by the following stepsCitation5: (1) a 2 × 2 table between treatments A and B for each AE is set up; (2) odds ratios (OR) are calculated for treatment A relative to B using EquationEquation (1)(1)
(1) below; and (3) the OR calculation is used to estimate the standardized probability of AEs from treatment B after adjusting for sample size using EquationEquation (2)
(2)
(2) below.
(1)
(1)
(2)
(2)
where ORA→B is the odds ratio of an AE occurring for treatment A relative to treatment B; number of AEA is the number patients who developed an AE from treatment A; number of AEB is the number patients who developed an AE from treatment B; number not having AEA is the number of patients who did not develop an AE from treatment A; number not having AEB is the number of patients who did not develop an AE from treatment B.
Next, the 95%CIs are estimated. By applying EquationEquations (1)(1)
(1) and Equation(2)
(2)
(2) to all AEs, the probability of an AE is estimated for the treatment and comparator using the standardized single sample size. While theoretically each probability of a reported AE has a 95%CI, clinical trial reports tend to focus on frequencies and percentages. If so, the 95%CI can be estimated using common statistical proceduresCitation6.
To adjust for time, the probability of AEs for both trial arms is measured over the follow-up time of the clinical trial. For a time-specific contract, mathematical equations that convert the probability measured in a clinical trial (PCT) to a rate in that clinical trial (RCT) and then to a probability over the time of contract T (PT). The following conversion equations are usedCitation7:
(3)
(3)
(4)
(4)
where RCT is the rate of AE during the clinical trial; PCT is the probability of AE reported in clinical trial over time t; t is the duration of the clinical trial (i.e. follow-up duration); PT is the probability of AE over the time of contract T; and T is the duration of contract. These steps are performed for all AEs for both treatments A and B.
Step 2. Estimating correction factors and corrected probabilities of aEs
In the second step, over the period of the contract, the risk difference (RD) is calculated for the probabilities of an AE from treatment comparators and reported in clinical trial(s). As shown in , the RD is known to follow a normal distribution bounded from −∞ to +∞Citation8; thus, the absolute risk difference (ARD) follows the normal distribution but is bounded from 0 to +∞. We recognize two regions around the estimated ARD: the desirable and undesirable probability density functions of the ARD as shown in . The desirable probability density function (Dpdf) is defined here as the area under the normal distribution curve where any value for ARD is favorable; patients in this region have a lower risk of developing an AE than the reported risk in a clinical trial. Conversely, the undesirable probability density function (Updf) – also called the “correction factor” – is defined as the area under the normal distribution where any value of the ARD is not favorable; patients in this region have a higher risk of developing an AE than the reported risk in a clinical trial. This correction factor is used to adjust the probability of an AE in the clinical trial by multiplying the correction factor, adjusted to the duration of the contract, with the probability of AE estimated over that period. This metric is referred to as the “corrected probability of AE”. As confirmatory procedure, we perform β distribution-based MCS to simulate the safety performance of treatment comparators in a hypothetical cohort (e.g. 2,000 iterations) and to estimate the Updf (i.e. the correction factor) for each AE. We provide additional technical details about how the number of iterations was set as Supplemental Material.
Figure 2. Risk difference and location of desirable and undesirable regions for absolute risk difference. Abbreviations. RD, risk difference; ARR, absolute risk reduction; ARI, absolute risk increase.

As shown in , the ARD has two namesCitation8: (1) if treatment A is associated with lower probability of AE than with treatment B, the ARD is called the “absolute risk reduction” (ARR)Citation8, as shown in ; (2) if treatment A is associated with a higher probability of AE than treatment B, the ARD is called the “absolute risk increase” (ARI)Citation8, as shown in .
(5)
(5)
where ARDT is the absolute risk difference over time T, ARRT is the absolute risk reduction over time T, ARIT is the absolute risk increase over time T, PT of AE from treatment A is the probability of AE from treatment A overtime T as calculated from EquationEquation (4)
(4)
(4) , PT of AE from treatment B is the probability of AE from treatment B over time T as calculated from EquationEquation (4)
(4)
(4) , and T is the duration of the contract.
As mentioned, and based on this definition for ARD and its derivatives, we introduce the term “undesirable probability density function” for ARD (Updf). This means that any calculated ARD (called expected ARD herein) is distributed normally from 0 to +∞ and splitting the probability density function of the normal distribution into two halves: 50% equal or below the expected ARD (0 to ARD), and 50% above the ARD (ARD to +∞). This is because the probability of any random value for ARD being the same as the expected ARD is approximately zero.
As shown in , in case of ARR, the undesirable region is left of the expected ARR, where any possible value of ARR is below the expected ARR, whether the risk of AE from treatment A is increased (the safety value of A decreased) or the risk of AE from treatment B is decreased (safety value of B increased). Both cases are not favorable to the payer if treatment A is of interest. Quantitatively, the risk of both cases is approximately 50%. This suggests that the probability of AE from treatment A will be corrected by approximately – and naturally – 50% risk, indicating that 50% of patients who had AE from treatment A would have had a chance to benefit more with treatment B. In this example, 50% is the correction factor and conditioned by the occurrence of AE.
As shown in , in case of ARI, the undesirable region is right of the expected ARI, where any possible value of ARI is above the expected ARI, whether the risk of AE from treatment A is increased (safety value of A decreased) or the risk of AE from treatment B is decreased (safety value of B increased). Both cases are not favorable by the payer if treatment A is of interest. Quantitatively, the risk of both cases is approximately 50%. This suggests that the probability of AE from treatment A will be corrected by approximately and naturally – 50% risk, indicating that 50% of patients who had AE from treatment A would have had a chance to benefit more with treatment B. In this example, the 50% is the correction factor and conditioned by occurrence of the AE.
To confirm the 50% assumed as a correction factor, we used beta distribution-based MCS, in which the probability of AE from treatments A and B and their estimated 95%CIs over the specified period are simulated on a hypothetical cohort of, for example, 1,000 or 2,000 iterations or more, using EquationEquations (6)(6)
(6) and Equation(7)
(7)
(7) to specify the beta distribution for each AE from treatment A and treatment BCitation7:
(6)
(6)
(7)
(7)
where ɑ and β are shape functions and always >0, X̄ is the average of probabilities, and SD is the standard deviation.
The MCS generates random probabilities of AE from both treatments that are limited by 0 and 1 specified for the beta distribution. Also, a skilled analyst could use coding to enable simultaneous calculation of simulated ARD and estimation of correction factors in case of ARR and ARI.
The correction factor estimated by MCS over a specified time is then multiplied by the probability of AE from treatment A expected over the same time [as generated from EquationEquation (4)(4)
(4) ]. This yields the corrected probability of AE from treatment A. The corrected probability is defined as the probability of an AE to occur undesirably beyond the expected probability reported in the clinical trial. The corrected probability can be estimated by using EquationEquation (8)
(8)
(8) :
(8)
(8)
where corrected PT is the corrected probability of AE from treatment A over T, PT is the probability of AE from treatment A over T, and T is the duration of contract.
Step 3. Estimating the probability of at least one AE occurring and leading to treatment discontinuation
In the third step, considering that the calculations above were replicated for all other AEs, the probability of at least one undesirable AE occurring and leading to treatment discontinuation over the specified time period.
During a course of a treatment, it is possible and, therefore, there is a given probability that at least one undesirable AE could occur and lead to treatment discontinuation. In safety-based pricing, payback is likely to be guaranteed if at least one AE exceeds the reported probability of that AE and, critically, leads to treatment discontinuation and therefore may impair treatment efficacy. This probability is calculated by using EquationEquation (9)(9)
(9) :
(9)
(9)
As shown above, the probability of at least one undesirable AE leading to discontinuation can be calculated; further, a range for that probability can be derived by first estimating the 95%CI for the probability of treatment discontinuation and replicating the equation above using the lower and upper bounds of the 95%CI estimated for the probability of discontinuation over time T.
Step 4. Estimating ranges for payback percentages and performing MCS to estimate the DSPSafety
Fourth, the estimated probability of at least one undesirable AE leading to discontinuation and its range are considered discounting estimates (i.e. percentages for price adjustment) and should be deducted from the marketed price of the evaluated treatment. This yields a price after adjustment and its range. With that application, the average and standard deviation for the adjusted price and its range are estimated to specify a gamma distribution, followed by MCS over an arbitrarily selected number of iterations (e.g. 2,000). This yields the DSPSafety. The gamma distribution uses functions as in EquationEquations (10)(10)
(10) and Equation(11)7
(11)
(11) :
(10)
(10)
(11)
(11)
where ɑ represents shape, β represents scale, X̄ is average price, and SD is standard deviation.
Assumptions
First, we assumed that the rate of any AE is fixed over time; thus, we applied the conversion equations mentioned earlier – EquationEquations (3)(3)
(3) and Equation(4)
(4)
(4) . Second, the expected ARD for any AE is the median, following a normal distribution, and splitting that distribution yields equal halves – 50% for each. Third, payback is guaranteed if treatment discontinuation is conditioned by occurrence of undesirable AE(s). This means that payback is only guaranteed if the probability of AE(s) is beyond the expected probability(s) and treatment discontinuation occurred. Fourth, we assumed that our methods provide an ex ante assessment and price adjustment should be warranted at the time of contracting. An additional assumption made in the proof-of-concept exercise was that the safety-based price assessment was limited to grade 3 and 4 AEs (AE3/4).
Proof-of-concept: application to osimertinib in NSCLC with EGFR mutation
Background
As mentioned, the AEs were retrieved for osimertinib and SOC from FLAURA trialCitation9. The 2018 wholesale acquisition cost (WAC) of osimertinib for a 30-day prescription was the price used for assessment in the proof-of-concept exercise ($14,616)Citation10. Also, the proof-of-concept provided two outcome contracts: a 1-year contract (2019–2020) and a 2-year contract (2019–2021). We averaged the medical consumer price index (mCPI) over 5 years (2014–2018) and resulted in 0.44% over the 1-year contract and 0.72 over the 2-year contractCitation11.
Analysis
In this proof-of-concept, the analyses were performed in Microsoft Excel 365Footnotei with MSO supported coding of visual basic for applications, and R for statistics/version i386 3.5.2 (Boston, MA, USA).
Results
Step 1. Pooling AEs, standardization, estimating 95%CIs, and adjusting for time
shows the pooled AE3/4 (n = 12) as reported in the FLAURA clinical trialCitation9. It also shows the pooled probabilities of treatment discontinuation that were used later in step 3 for osimertinib only. As shown in , the results of sample size standardization did not change the probability of AEs because the sample sizes between the arm of osimertinib and SOC were comparable (279 versus 277) as per FLAURACitation9. The OR calculations used for standardizations estimated per EquationEquations (1)(1)
(1) and Equation(2)
(2)
(2) and included in Supplemental Material (S1). As shown in , the 95%CIs were estimated using R, assuming the exact method for estimation. The code is available in Supplemental Material (S2).
Table 1. Probabilities (%) of adverse events of grades 3 and 4 (AEs3/4)Table Footnotea and estimated 95% confidence intervals (95%CIs).
shows the time-adjusted probability of the reported grade 3/4 AEs. The conversions used EquationEquations (3)(3)
(3) and Equation(4)
(4)
(4) to project the probabilities of grade 3/4 AEs over the period of the hypothetical 1-year and 2-year contracts. Note that the probability of any AE over 2 years is not two times that over 1 year.
Table 2. Probabilities (%) and 95% confidence intervals (95%CIs) for adverse events of grades 3 and 4 (AEs3/4) standardizedTable Footnotea and adjusted for 1-year and 2-year contracts.
Step 2. Estimating correction factors and corrected probabilities of AEs
shows the RD calculated for each AE3/4 probability of osimertinib and SOC over 1-year and 2-year contracts as per EquationEquation (5)(5)
(5) . As noted, the sign of RD indicates whether osimertinib was expected to have equal risk of an AE3/4 with SOC (if no risk difference was expected, zero with no sign), whether osimertinib was expected to have lower risk of an AE3/4 than SOC (negative RD or ARR) or whether osimertinib was expected to have higher risk of an AE3/4 than SOC (positive RD or ARI). As shown in for the 1-year contract, osimertinib was expected to have equal risk with SOC in fatigue and stomatitis; a lower risk than SOC in diarrhea, rash or acne, anemia, dyspnea, dry skin, paronychia, aspartate aminotransferase (AST) and alanine aminotransferase (ALT) elevations; and a higher risk than SOC in decreased appetite and prolonged QT. For the 2-year contract, osimertinib was expected to have equal risk with SOC in fatigue; a lower risk than SOC in diarrhea, rash or acne, stomatitis, anemia, dyspnea, dry skin, paronychia, and AST and ALT elevation; and a higher risk than SOC in decreased appetite and prolonged QT. The undesirable regions were specified as follows: in case of equal risk, the Updf is under the area where any ARI is greater than zero; in ARR, the Updf is under the area where any ARR is lower than the expected ARR; in ARI, the Updf is under the area where any ARI is greater than the expected ARI.
Table 3. Risk differences (RD) for 1-year and 2-year contracts between osimertinib and SOC.
shows the Updf (correction factors) for all undesirable ARDs estimated over the 1-year and 2-year contracts. These correction factors represent probabilities that any possible value of any ARD is located under the undesirable region. As per EquationEquations (6)(6)
(6) and Equation(7)
(7)
(7) , we performed a total of 2,000 iterations to simulate treatment safety for osimertinib and SOC, and to provide the correction value, which was approximately 50%.
Table 4. Correction factor (%) from MCS, corrected probability (%), and ranges for price adjustments.
Applying EquationEquation (8)(8)
(8) , the correction factor is multiplied by the probability of AE3/4 estimated over the 1-year and 2-year contracts to produce the corrected probability of each AE3/4. shows the corrected probability of occurrence of each AE3/4, specified over the 1-year and 2-year contracts.
Step 3. Estimating the probability of at least one AE occurring and leading to treatment discontinuation
First, EquationEquation (9)(9)
(9) was applied and then the complement of each corrected probability was calculated (1 − the corrected probability) of each AE3/4. shows that, over the 1-year contract, the probability of at least one undesirable AE3/4 occurring was 4.15% and was conditioned by treatment discontinuation that is specified for the 1-year contract at 8.87% (95%CI = 6.23%–11.98%). Thus, multiplying 4.15% by the probability of discontinuation and its 95%CI gives a probability of 0.37% (range = 0.26–0.50%). shows that, over the 2-year contract, the probability of at least one undesirable AE3/4 occurring was 8.14% and was conditioned by treatment discontinuation specified for the 2-year contract at 16.95% (95%CI = 12.08%–22.52%). Thus, multiplying 8.14% by the probability of treatment discontinuation and its 95%CI gives a probability of 1.38% (range = 0.98–1.83%).
Step 4. Estimating ranges for payback percentages and performing MCS to estimate the DSPSafety
The probability of at least one AE3/4 occurring and leading to discontinuation is considered as a percentage for price adjustment. shows that for the 1-year contract, multiplying 4.15% by the probability and 95%CI of treatment discontinuation suggested a safety-based adjustment of −0.37% (range = −0.26% to −0.50%). In the 2-year contract, multiplying 8.14% by the probability and 95%CI of treatment discontinuation suggested a safety-based adjustment of −1.38% (range = −0.98% to −1.83%).
Table 5. Prices of osimertinib for two hypothetical contracts (2019–2020 and 2019–2021) at osimertinib 2018 WAC price of $14,616 for a 30-day prescription.
As shown in , assuming the 1-year contract was during 2019–2020, with the inflation rate projected for mCPI was at +0.44%Citation11, the safety-based price assessment for osimertinib mandated adjustment by +0.07% (range = −0.06–0.18%). The resulting price for osimertinib is $14,627 (range = $14,608–$14,642), averaged at $14,626 (SD = $17). Upon gamma MCS, the DSPSafety was averaged for the 2,000 iterations performed at $14,627 (or +0.08% the 2018 WAC).
Also, as shown in , assuming the 2-year contract was from 2019 to 2021, with the inflation rate projected for mCPI was at +0.72%Citation11, the safety-based price assessment for osimertinib mandated adjustment by −0.66% (range = −1.11% to −0.26%). The resulting price for osimertinib is $14,520 (range = $14,453–$14,578), averaged at $14,517 (SD = $62). Upon gamma MCS, the DSPSafety averaged $14,516 for the 2,000 iterations performed (or −0.68% the 2018 WAC).
Discussion
While our efforts to develop an inclusive method for outcome-based pricing as proposed by the initiative are ongoing, we aimed in this study to focus on the attribute of safety value in treatment pricing over a short-term assessment. Thus, cautions should be considered at the beginning of this discussion. First, the methodology in this study has focused only on safety data adjusted for the short term; second, we have used data from clinical trials. Thus, it is important to integrate the suggested prices in this study with those generated from the other dimensions that provide short-term and long-term assessments for treatment price; further, it is recommended to replicate the methods in this study using RWD to reflect the reality of clinical practice and performance of treatments on treatment pricing.
Relevant to the concept of risk sharingCitation12, we aimed to provide a method for safety-based pricing based on the fact that in any outcome-based contract, payback is guaranteed for any AE that could occur at unexpected rate (undesirable risk) and lead to treatment discontinuation. That is, among a list of possible AEs, payback can be quantified by the probability of at least one AE occurring and leading to discontinuation; that probability is considered as the percentage of payback, which then can be discounted prospectively, ex ante, from the marketed price of the evaluated treatment. In our assessment, we quantified the undesirable risk of AE occurrence and discontinuation, with that magnitude of risk, the price of treatment was adjusted. Thus, the payer accepts the risk in exchange for price reduction, and the treatment manufacturer is cleared from any safety risk that may be associated with taking their treatment.
We estimated the average and standard deviation for treatment prices and simulated by MCS within the gamma distribution [EquationEquations (10)(10)
(10) and Equation(11)
(11)
(11) ], resulting in DSPSafety for the given two contracts. In the proof-of-concept exercise, given that the 2018 WAC price of osimertinib was $14,616: for the 1-year contract (2019–2020), the price was estimated at $14,627 ($14,608–$14,642), averaged at $14,626 (SD = $17), and MCS was performed to estimate the DSPSafety at $14,627 (+0.08%). For the 2-year contract (2019–2021), the price was estimated at $14,520 ($14,453–$14,578), averaged at $14,517 (SD = 62.14), and MCS was performed to estimate the DSPSafety at $14,516 (−0.68%).
Importantly, three observations can be made from our methods and the proof-of-concept exercise. First, the correction factor among all estimated ARDs was close to 50%. This followed naturally from the assumption that the expected ARD, whether it is ARR or ARI, is the median and follows a normal distribution. The reason behind this assumption is that AEs reported in clinical trials are priori and have no 95%CIs. Particularly in the proof-of-concept exercise, the 95%CIs around the reported probabilities were estimated by a software that assume the exact method with equal variances for estimating the 95%CI. The amount of the correction factor may vary if the probabilities of AEs reported in the clinical trial are with 95%CI.
Second, outcome-based contracts using only safety data will suggest price reductions. As shown in the proof-of-concept, the model suggests a price reduction: 1-year contract at −0.37% (range = −0.26% to −0.50%) and 2-year contract at −1.38% (range = −0.98% to −1.83%); however, when applying projected inflation rates of mCPI, the treatment adjustment varied between positive and negative percentages. For the 1-year contract, the inflation rate was higher than the safety-based adjustment, which explains the increase in the price of osimertinib by +0.08%; for the 2-year contract, inflation was lower than the safety-based adjustment at that time, which explains the reduction in osimertinib price by −0.68%.
Third, contracting treatments for a longer term using the relative value of safety (osimertinib versus SOC) can yield more reductions than contracting for the short term. This is because the probability of at least one AE occurring and leading to discontinuation increases over time at a fixed rate. As demonstrated in the proof-of-concept exercise, the price of osimertinib was reduced more in the 2-year contract (−0.68%) than in in the 1-year contract (+0.08%).
Our study shows several strengths. Probabilistic methods for contracting cancer treatments based solely on safety value have not yet been proposed. Our methods depend on the relative safety value of a treatment versus a comparator. This is more valuable than using only the safety data of treatment of interest. Thus, we used risk difference as opposed to the risk itself for estimating the payback. We did not use the price of comparators to set a price for the treatment of interest; and this is importantly considering pricing freedom in the US. The suggested methods are statistically plausible and transparent in handling probabilities, estimating 95%CIs, and converting probabilities over a specific time; all validated statistical proceduresCitation6,Citation7,Citation13. The correction factor and its use in correcting probability of an event have been used previously in pharmacoeconomic modellingCitation7. Our suggested four-step method can be considered a collection of already existing statistical methods combined with new technology; for example, where we incorporated MCS to simulate a hypothetical cohort to confirm the correction values estimated mathematically. This collection of tools adds value to our study; the random nature of MCS was confirmed by the mathematical constructions we applied. The method of creating price dispersion by suggesting a price and its ranges are important at the approval stage of a treatment when no RWD are available to support prices. This represents the randomness of pricing after considering all possible scenarios. Economically, price variation under randomness is justifiable when information about the value of treatments is not available yetCitation14.
Our study also has some limitations. In the methods and the proof-of-concept exercise, we were limited to data from a clinical trial. Trials are not the ideal source for safety performance of treatments and RWD are more informative on how a treatment performs from a safety point of view. However, we included MCS to randomly sample the probability of AEs with estimated 95%CIs, and suggested DSPSafety with ranges.
The method of pricing based on safety information may be affected by selection bias in the AEs considered. We suggest establishing inclusion and exclusion criteria in which expert opinion is considered (Supplemental Material). In our proof-of-concept, we only included reported AE3/4 in the clinical trial that were submitted to the Food and Treatment Administration (FDA) in the approval processCitation9.
Our method assumed a fixed rate over time. This may be a conservative and exploratory assumption in which the rate of AE depends on different factors: aging, co-morbidities, polypharmacy, and so on. With greater availability of safety information, our method could be less conservative, and a flexible rate can be estimated.
The effect of safety information on the accuracy of price adjustment is critical. The 50% correction factor can be adjusted based on the assumption made by the analyst. No 95%CIs were estimated in the FLAURA trial used for the proof-of-concept exercise. The 95%CIs for AEs were estimated using statistical software that assumed equal variance. In the proof-of-concept exercise, the probability of discontinuation may have included reasons other than AE3/4. The clinical trial report did not specify that probability or which events that probability included. Future studies will require collection of additional safety data beyond those from clinical trials, including RWD.
Conclusion
Ex ante safety-based price assessments are important for payers and treatment manufacturers. This assessment should be integrated with the other dimensions in the six-dimensional platform for outcome-based contracting being developed. Safety-based pricing yield price reductions, and the amount of those reductions depends mainly on the amount of safety information available, the assumptions used, and the number of AE included in the assessment, as well as being affected by inflation rate.
Transparency
Declaration of funding
The work reported herein was performed without sponsorship or grant funding.
Declaration of financial/other relationships
The authors have no financial relationships to declare.
JME peer reviewers on this manuscript have no relevant financial or other relationships to disclose.
Author contributions
All named authors meet the International Committee of Medical Journal Editors (ICMJE) criteria for authorship of this manuscript.
JME-2020-0096-RT.R1_SUPPLEMENT_CATS.docx
Download MS Word (30 KB)Acknowledgements
The authors thank Amber Koslucher and Rayan Maldonado from the College of Public Health, University of Arizona, for validating the mathematical equations and for performing independent revisions of the assumptions, equations, and calculations.
Notes
i Microsoft Excel 365 is a registered trademark of Microsoft Research Lab, Redmond, Washington, DC, USA.
References
- Alkhatib NS, Erstad B, Ramos K, et al. Pricing methods in outcome-based contracting-δ1: cost-effectiveness analysis and cost utility analysis-based pricing. J Med Econ. 2020;23(11):1215–1222.
- Alkhatib NS, Ramos K, Erstad B, et al. Pricing methods in outcome-based contracting-δ2: willingness-to-pay-based pricing. J Med Econ. 2020;23(11):1223–1229.
- Alkhatib NS, Erstad B, Ramos K, et al. Pricing methods in outcome-based contracting-δ3: reference-based pricing. J Med Econ. 2020;23(11):1230–1236.
- PhRMA. Value-based contracts: 2009-Q1 2018. USA; 2018 [accessed December 2019]. Available from: http://phrma-docs.phrma.org/files/dmfile/PhRMA_ValueBasedContracts_V9.pdf
- Martin J. Probability given odds. [accessed December 2019]. Available from: https://www.ctspedia.org/do/view/CTSpedia/OddsTerm#Calculating_Probability_Given_Od
- Ho AM, Dion PW, Karmakar MK, et al. Estimating with confidence the risk of rare adverse events, including those with observed rates of zero. Reg Anesth Pain Med. 2002;27:207–210.
- Briggs A, Claxton K, Sculpher M. Decision modelling for health economic evaluation. New York (NY): Oxford University Press; 2011.
- Kenneth RJ. Epidemiology: an introduction. 2nd ed. New York (NY): Oxford University Press; 2012. p. 66–167.
- Soria J, Ohe Y, Vansteenkiste J, et al. Osimertinib in untreated EGFR-mutated advanced non–small-cell lung cancer. N Engl J Med. 2018;378:113–125.
- RedBook Online [subscription database onlie]. Updated periodically. Greenwood Village (CO): Truven Health Analytics. [Accessed December 2019]. Available from: http://micromedex.com/products/product-suites/clinical-knowledge/redbook
- U.S. Bureau of Labor Statistics. Prescription drugs in U.S. city average, all urban consumers, seasonally adjusted. [accessed December 2019]. Available from: https://data.bls.gov/timeseries/CUSR0000SEMF01?output_view=pct_3mths
- Garrison LP, Towse A, Briggs A, et al. Performance-based risk-sharing arrangements-good practices for design, implementation, and evaluation: report of the ISPOR good practices for performance-based risk-sharing arrangements task force. Value Health. 2013;16:703–719.
- Zikmund-Fisher BJ, Fagerlin A, Roberts TR, et al. Alternate methods of framing information about medication side effects: incremental risk versus total risk of occurrence. J Health Commun. 2008;13:107–124.
- Griffith D. Four types of price variation. Laramie (WY); 2013 [accessed December 2019]. Available from: http://www.uwagec.org/rightrisk/presentations/2013_03_26_DGriffithMarketing/1_Introduction/1_FourTypesPriceVariation.pdf