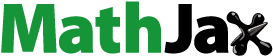
ABSTRACT
Introduction
Histological images contain phenotypic information predictive of patient outcomes. Due to the heavy workload of pathologists, the time-consuming nature of quantitatively assessing histological features, and human eye limitations to recognize spatial patterns, manually extracting prognostic information in routine pathological workflows remains challenging. Digital pathology has facilitated the mining and quantification of these features utilizing whole-slide image (WSI) scanners and artificial intelligence (AI) algorithms. AI algorithms to identify image-based biomarkers from the tumor microenvironment (TME) have the potential to revolutionize the field of oncology, reducing delays between diagnosis and prognosis determination, allowing for rapid stratification of patients and prescription of optimal treatment regimes, thereby improving patient outcomes.
Areas Covered
In this review, the authors discuss how AI algorithms and digital pathology can predict breast cancer patient prognosis and treatment outcomes using image-based biomarkers, along with the challenges of adopting this technology in clinical settings.
Expert Opinion
The integration of AI and digital pathology presents significant potential for analyzing the TME and its diagnostic, prognostic, and predictive value in breast cancer patients. Widespread clinical adoption of AI faces ethical, regulatory, and technical challenges, although prospective trials may offer reassurance and promote uptake, ultimately improving patient outcomes by reducing diagnosis-to-prognosis delivery delays.
1. Introduction
1.1. Background
Tumor histology uncovers phenotypic information that is indicative of not only disease progression, but also often patient outcome [Citation1]. Developments in artificial intelligence (AI) technology have allowed the mining of previously unexploited features from routine histology images of cancer, providing potential diagnostic, prognostic, predictive, and other clinically relevant information [Citation1]. Human assessments of histology are subjective and are prone to difficulties in terms of reproducibility due to inter-observer variability [Citation2].
The inherent uncertainty in traditional pathology analysis may result in inappropriate treatment and secondary complications, adversely affecting patient quality of life [Citation3]. Therefore, computational analysis of histology imaging has received significant attention since the timely and accurate assessment of tumor histomorphology and identifying pertinent prognostic/predictive markers are crucial to personalized cancer care. The utility of AI in detecting image-based prognostic/predictive biomarkers has been demonstrated to be particularly promising for breast cancer [Citation4–6].
Breast cancer is the most common female cancer, accounting for over a quarter of all cancers in women, with more than 2.3 million women diagnosed worldwide in 2020 [Citation7]. Breast cancer is a heterogeneous disease that still presents challenges for clinicians in predicting the likelihood of disease progression, particularly in patients where the disease is detected in the early stages [Citation8]. To manage the increasing volume of breast cancer cases, meaningful interpretation of breast cancer progression to establish prognostic factors and limit the number of patients undergoing unnecessary treatments is paramount.
Approximately 30% of patients initially presenting with early-stage disease develop a recurrence of the disease within 10 years and, therefore, require necessary, sometimes aggressive chemotherapy [Citation9]. However, differentiating between early-stage breast cancer patients whose disease will or will not recur remains a clinical challenge to avoid unnecessary application of aggressive treatment strategies. Traditionally, most early-stage patients with breast cancer were treated with chemotherapy to prevent recurrence, despite many not benefiting from such treatment, thereby exposing these individuals to severe chemotherapy-related side effects and often additional financial burden [Citation10]. Current approaches to treatment stratification and prognostic prediction of survival for breast cancer patients are based on molecular tests that are not readily available in many jurisdictions due to cost and other barriers. Thus, identifying an alternative, cost-effective patient stratification approach using AI-based digital pathology analysis of histopathology images has great potential for global patient benefit. This review aims to summarize the status of published work on prognostic/predictive image biomarkers for breast cancer that have been developed using AI and digital pathology.
1.2. What is AI, machine learning, and deep learning?
AI is a broadly encompassing term referring to the branch of computer science that attempts to emulate human intelligence. These specialized systems are developed and programmed to perform complex cognitive functions such as learning, problem-solving, and decision-making, tasks originally reserved for humans [Citation11]. Machine learning (ML), a subfield of AI, is defined as a computational system based on a set of algorithms that attempts to examine vast amounts of data, by using multiple layers of analysis, to ‘learn’ from the data to make predictions on future data () [Citation12,Citation13]. Over time, the computational algorithm performance improves with experience in respect to the instructed task, such as classification, regression, and clustering [Citation14,Citation15]. Deep learning (DL) is a ML technique comprising multiple layers of artificial neural networks (ANNs), which were devised in the 1980s as artificial representations of the human neural architecture [Citation13]. ANNs incorporate three functional layers of artificial neurons or ‘nodes,’ which include an input layer, multiple hidden layers, and an output layer () [Citation11]. Each node is connected to the immediately earlier and deeper layers via edges, with the strength of their connection being referred to as their ‘weight’ [Citation16]. The input layer nodes typically consist of the desired variables being quantified in the dataset of interest – for example, each node might represent the intensity value of a specific pixel in an image. The hidden layers of the ANN perform feature construction for the layers prior, with deeper layers refining earlier layers [Citation16]. For this reason, these algorithms can automatically learn feature representations without feature engineering, avoiding bias and achieving end-to-end result output [Citation17]. Improvements in computational processing power have led to an increased interest in using AI for digital pathology over the past decade.
1.3. Digital pathology
Digital pathology is essentially the process of generating high-resolution digital images from tissue sections on glass slides that are typically examined under a microscope, using complex, novel imaging systems and whole slide image (WSI) scanners [Citation18–20]. WSI scanners capture multiple images of entire tissue sections within minutes, allowing the complex spatial relationships within the tumor microenvironment (TME) to be assessed digitally. Additionally, digital images can be shared between hospitals and research labs for real-time analysis (telepathology), significantly improving workflow efficiency [Citation3]. In order to implement digital pathology successfully in the clinic, several critical elements need to be acquired namely (1) hardware, which is composed of a digital slide scanner, (2) software, which facilitates image creation, visualization, management, and analysis, and (3) network connectivity facilitated by central storage/cloud-based systems () [Citation21,Citation22]. Furthermore, ML and DL algorithms have been successfully incorporated into digital pathology workflows to computationally evaluate images, limiting the subjective nature of visual perception, human errors in data integration, and opinion between interobservers () [Citation15]. Moreover, the application of AI in digital pathology allows for the integration of clinical parameters, which presents an opportunity to develop image-based biomarkers for predicting breast cancer patient prognosis and treatment efficacy, a focal point of this review paper.
Figure 2. Artificial intelligence (AI) and Digital Pathology Workflow. DIA: data-independent acquisition. Initially, tissue samples are stained to highlight key histological features, followed by the digitization of the samples into high-resolution digital images using scanners. AI-based algorithms are subsequently applied to identify and quantify relevant features (ex-TIL count, stromal, and tumor area) within the images. The final step involves evaluating the association between the identified features and patient survival outcomes through statistical analysis.
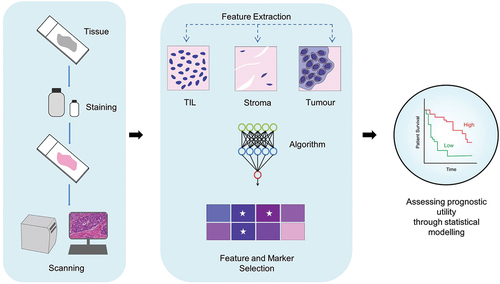
2. Application of AI in digital histopathology in breast cancer (predictive and prognostic biomarkers)
Both ML and DL algorithms have been demonstrated to successfully stratify breast cancer patients for prediction of prognosis and response to treatment. This review discusses their application in investigating the prognostic and predictive potential of histopathological features (Section 2.1), along with how DL algorithms can bypass conventional feature selection for prognosis prediction (Section 2.2).
2.1. Histopathological annotations
2.1.1. Tumor infiltrating lymphocytes
2.1.1.1. Prognostic
A successful response to immunotherapies is dependent on the immune contexture of the TME, with the presence of certain cell types, such as tumor infiltrating lymphocytes (TILs), being associated with enhanced survival in patients with various forms of cancer [Citation23,Citation24]. Traditionally, TILs are scored semi-quantitatively on standard H&E-stained sections as absent, non-brisk, or brisk, and some authors have suggested utilizing a four-tier grading system that considers TIL distribution and density [Citation25]. However, despite these well-established scoring methods and the body of evidence supporting a prognostic role for this biomarker, TIL quantification is yet to be broadly adopted for clinical decision-making due to a lack of standardization between institutes and apprehension regarding reproducibility between pathologists [Citation25]. However, the International Immuno-oncology Biomarkers Working Group has aimed to standardize TIL scoring through a guideline designed for the visual evaluation of TILs in Hematoxylin and Eosin (H&E) sections, with its application in breast cancer leading to an acceptable interobserver agreement between multiple pathologists [Citation26–28]. Moreover, AI and digital pathology can further aid in this standardization by analyzing complex spatial patterns and providing metrics for vigorous validation to allow for reliable patient stratification [Citation25,Citation29–31]. As such, the prediction of patient prognosis using AI and digital pathology was demonstrated in a cohort of triple-negative breast cancer (TNBC) patients using the open-source software QuPath [Citation32]. Interestingly, multivariate Cox regression analysis adjusting for stage, age, and histological grade demonstrated that measurement of the density of TILs over stromal area: easTIL% (100 * [sum of TIL Area (mm2)/Stroma Area (mm2)]), a marker which mimics the International TIL Working Group variable used by pathologist, was prognostically significant for overall survival (OS) in the validation cohort (p = 0.0003). Moreover, Heindl et al., demonstrated similar results in estrogen receptor positive (ER+) breast cancer using an AI image analysis pipeline exploiting the morphological differences between cancer cells, lymphocytes, and stromal cells in H&E-stained tissue sections obtained from 1,178 patients enrolled in the Arimidex or Tamoxifen Alone or Combined Trial (ATAC), 141 of which were also human epidermal growth factor receptor 2 positive (HER+) [Citation33]. Accuracy rates for identifying the three cell types were 93.8% for cancer cells, 87.9% for lymphocytes, and 84.2% for stromal cells. The immune abundance metrics, such as overall lymphocyte infiltration and intratumor lymphocyte ratio, did not provide significant prognostic information for recurrence [Citation33]. In contrast, high immune spatial scores, indicating increased cancer and lymphocyte spatial clustering, were significantly associated with poor 10-year recurrence-free survival. Moreover, the prognostic value for spatial scores for late recurrence (5–10 years) was similar to that of IHC 4 and Recurrence Score (RS; Oncotype Dx) in both the overall population and HER2+ population. Additionally, Immune-Cancer Hotspot, Immune Hotspot, and Cancer Hotspot scores provided statistically significant prognostic value when added to IHC4 and RS for 0–10 years, 5–10 years, and in both time windows, respectively, for the overall patient population. However, none of the combined scores outperformed the Clinical Treatment Score, a risk prediction model calculated from clinicopathological variables, and the PAM50 Risk of Recurrence Score [Citation33]. Furthermore, in accordance with these findings, Thagaard et al., 2021 demonstrated that stromal tumor-infiltrating lymphocyte (sTILs) could be utilized as a prognostic image biomarker for TNBC using a fully automated digital image analysis pipeline [Citation34]. The multistep process, developed and performed on the Visiopharm platform, involved 1) training a convolutional neural network (CNN) to differentiate between tissue and slide background, 2) a second level CNN that segmented areas of tumor, necrosis, and noninvasive epithelium (normal, pre-invasive lesions), 3) an object-based density analyzer which outlined tumor and tumor-associated stroma regions, 4) a third level CNN which detected TILs (as mononuclear immune cells), and 5) the generation of tumor heatmaps [Citation34]. The algorithm was tested on 257 patients, with univariate analysis demonstrating that high sTIL density was associated with significantly prolonged OS (every 10% or 300 sTILs/mm2 increase in the biomarker score resulted in a ~ 20% decrease in risk of death). Moreover, Thagaard et al., 2021 demonstrated that sTIL density was prognostic for OS, independent of age, tumor size, nodal status, and tumor type (HR: 0.81, p = 0.001) [Citation34]. Furthermore, Makhlouf et al., 2023 utilized a supervised DL model to analyze TILs from H&E-stained WSI from a cohort of 2,231 luminal early-stage breast cancer patients [Citation35]. The study elucidated that high sTILs and intatumoral (t)TILs counts were associated with significantly shorter survival than tumors with low sTILs and tTIL counts; HR = 2.5, 95% CI = 1.3–4.5, p = 0.004 and HR = 2.0, 95% CI = 1.06–3.7, p = 0.03, respectively [Citation35]. Additionally, the proximity of TILs to stromal and tumor cells (co-occurrence) were associated with poor clinical outcomes and clinicopathological parameters including younger age at diagnosis, high tumor grade, large tumor size, and lymph node metastasis, signifying the effective use of AI and digital pathology to elucidate the prognostic utility of TILs in luminal breast cancer [Citation35]. In summary, these studies illustrate the potential for AI algorithms to predict OS for breast cancer patients using TILs as an image-based biomarker in the clinic.
2.1.1.2. Predictive
Breast cancer patients are often administered neoadjuvant therapy, that is, pre-operative systemic treatment in the form of chemotherapy, radiotherapy, and endocrine therapy [Citation36]. Patients with tumors that demonstrate a pathological complete response (pCR), a surrogate endpoint for event-free survival (EFS), have a reduced risk of recurrence compared to those achieving a partial response [Citation37,Citation38]. However, only 20–30% of patients achieve a pCR, with this being dependent on the tumor biology; accordingly, identifying image biomarkers using AI and digital pathology may aid in the stratification of these patients [Citation39,Citation40]. Lee et al., 2022 demonstrated that AI-powered spatial TIL algorithms could successfully evaluate TIL densities in the tumor area and surrounding stroma in TNBC patients [Citation41]. Mean intratumoral (i) TIL scores (5.8% vs 4.5%, p < 0.001) and surrounding stromal (s)TIL scores (12.1% vs 9.4%, p < 0.001) were significantly higher in patients who achieved a pCR after neoadjuvant chemotherapy, with iTIL scores remaining an independent predictor of pCR along with CT stage and Ki-67 after multivariate analysis [Citation41].
Recently, Fanucci et al., 2023 assessed the predictive value of TILs for pCR and event-free survival in patients with stage IIB-IIIC HER2 breast cancer randomized to neoadjuvant chemotherapy (NAC) ± bevacizumab [Citation42]. In these 113 pretreatment patient samples, the authors demonstrated that a ML-derived digital measure of TILs (easTILs, described above) was predictive of pCR in breast cancer where the mean pretreatment easTILs% was significantly higher in cases with pCR compared to residual disease (median 36.1 vs 14.8%, p < 0.001). Quantification of easTILs had more effective outcome discrimination compared to the sTILs score interpreted by a pathologist [Citation42].
Further, Choi et al., 2023 established a DL-based algorithm to evaluate sTIL expression in breast cancer WSIs [Citation43]. Following manual assessment by a pathologist, WSIs from TNBC and HER2+ patients were reevaluated by the pathologist with the assistance of the DL model. Firstly, DL-assistance increased the concordance correlation co-efficient among pairs of pathologists. Further, compared to review by pathologists alone, DL-assistance further increased the difference between the average sTIL scores allocated to responders vs non-responders to NAC (26.8 ± 19.6 vs. 19.0 ± 16.4; p = 0.003), further demonstrating the ability of AI to heighten the predictive power of TIL scoring [Citation43].
In line with these findings, TILs have been demonstrated to predict breast cancer patient response to immune-checkpoint inhibition in a number of clinical trials, including Keynote-086 [Citation44]. Various studies have demonstrated the utility of AI to investigate TIL-based prediction of immunotherapy response. Park et al., 2022 categorized the TME of advanced non-small cell lung cancer (NSCLC) patients by immune phenotype, as either inflamed, immune-excluded, or immune-desert, based on TIL density using an AI-powered algorithm on H&E-stained WSIs [Citation45]. Patients with tumors exhibiting an inflamed immune phenotype demonstrated higher response rates to immune-checkpoint inhibitors, an increase in cytolytic activity and had prolonged progression-free survival (4.1 months), compared to patients whose tumors were differentiated as having an immune-excluded (2.2 months) or an immune-desert phenotype (2.4 months) [Citation45]. Additionally, there was a significant correlation between the AI model (Lunit SCOPE IO) assessment of TILs and pathologists’ manual evaluation of TILs (p < 0.001), indicating the potential for AI-based models to predict patient response to treatment in a reliable, accurate, and time-effective manner [Citation45]. These results demonstrate the potential use of TIL scores in predicting treatment response in breast and other cancer patients.
In 2023, Li and colleagues developed a novel 25 ML algorithm-based immune infiltrating cell (MLIIC) signature to assess TNBC prognosis, mutation, biological function, drug responsiveness, immune infiltration, and immunotherapy responsiveness [Citation46]. In a similar study, Tang et al., 2023 utilized ML algorithms to develop an immunophenotype-based risk assessment model for TNBC patients. The model demonstrated a significant improvement in its ability to predict prognosis and drug sensitivity for TNBC patients, with a high level of accuracy [Citation47].
2.2. Mitoses/large-scale DNA organization
2.2.1. Prognostic
In recent years, several genomic tests that predict cancer patient prognosis have been incorporated into the clinic [Citation48]. The effects of genetic and epigenetic changes are reflected in the nuclei of cancer cells via their morphological and chromatin organization [Citation49]. Advances in image processing algorithms, data science, optics, and microscopy have enabled the quantification of these nuclear features during tissue assessments of sectioned material by pathologists [Citation49]. In particular, the quantification of Large-Scale DNA Organization (LDO), referring to the measurement of different characteristics of the nuclear DNA organization such as the size, shape of the nucleus, and the intensity of the staining, can be correlated with disease states to predict cancer patient prognosis [Citation49–51]. Guillaud et al., 2018 demonstrated that LDO was an independent prognostic marker for breast cancer survival [Citation49]. A forward stepwise linear discriminant analysis determined 6 nuclear features that classified patients into ‘survival’ and “deceased’’ groups. A total of 76.3% of cells (of 5493 total detected cells) were correctly classified (into ‘survival’ and ‘deceased’ groups) with a sensitivity and specificity of 81.2% and 73.5%, respectively. Moreover, when a core LDO value of 60% was used as a cutoff point to differentiate between LDO negative (LDO score lower or equal to 60%) or LDO positive (LDO score higher than 60%) cores, the sensitivity and specificity of this prognostic test increased to 84.4 and 81.6%, respectively [Citation49]. In line with these findings, LDO scores were demonstrated to be predictive of prognosis in other solid tumor cancer types such as prostate [Citation52] and lung [Citation53], indicating the potential efficacy of this marker to be utilized in the clinic in the future.
The Nottingham Grading System has been widely used to determine the grade of breast cancer, a significant indicator of patient prognosis [Citation54]. The system focuses on three nuclear biomarkers, including nuclear atypia, tubule formation, and mitotic cell count [Citation55]. Among these biomarkers, mitotic cell count is considered the most important in terms of grading due to its direct relationship with tumor prognosis. However, manually counting mitotic features is time-consuming (typically taking a pathologist 5–10 min to perform), subjective, and suffers from low reproducibility [Citation55,Citation56]. Additionally, it may be difficult to differentiate between a mitotic figure and a cell undergoing degeneration, apoptosis, or necrosis [Citation57]. AI coupled with digital pathology may offer a potential solution to the aforementioned problem. Although studies investigating the prognostic utility of AI-based histological analysis of mitotic figures are sparse, Pantanowitz et al., 2020 demonstrated that pathology end-users were more accurate and efficient (overall timesaving of 27.8%) at quantifying mitotic figures in digital images of invasive breast carcinoma with the aid of AI [Citation57]. In 2023, van Bergeijk and colleagues conducted a study on AI-assisted approaches to mitotic counting (MC) in breast cancer biopsies/resections using WSI and light-microscopy (LM) [Citation58]. The study found that WSI-MC, with the help of AI aligns well with the LM-MC. As a result, WSI-MC is considered a promising alternative to LM-MC [Citation58]. More recently, Ibrahim et al., 2024, investigated the optimal method of using AI-based mitotic figure scoring in breast cancer [Citation59]. This study demonstrated that mitotic activity index (MAI), defined as the number of mitotic figures in 3 mm2 area within the mitotic hotspot, positively correlated with the gold standard visual scoring method used in the Nottingham Grading System (R = 0.8) and Ki67 scores (R = 0.69).
Regarding the ability of such an AI model to infer patient prognosis utilizing mitotic count, Ibrahim et al., 2024 also demonstrated that mitotic activity index (MAI) was an independent predictor of survival (p < 0.05) in multivariate Cox regression analysis [Citation59]. Moreover, Jaroensri and colleagues utilized DL approaches to develop an AI-based Nottingham grading system [Citation60]. The system was used to grade the three Nottingham grading system components; nuclear pleomorphism, tubule formation and mitotic count, in invasive breast carcinoma WSIs, with comparison to pathologist standards [Citation60]. Regarding the prognostic ability of mitotic count, utilizing progression-free interval as the endpoint, the AI-determined MC achieved a C-index of 0.58 (95% CI: 0.53, 0.64) vs that obtained by the pathologist-given MC of 0.54 (95% CI: 0.48, 0.59) [Citation60]. Further providing evidence of its prognostic potential, the AI-based model provided a MC score that demonstrated better correlation with Ki-67 gene expression with a correlation co-efficient of 0.47 (95% CI: 0.41, 0.52) vs a correlation coefficient of 0.37 (95% CI: 0.32, 0.43) between pathologist-based MC and Ki-67 gene expression, which may suggest that the AI-based findings could potentially be more indicative of the biologically relevant ground truth i.e. cell proliferation [Citation60]. These studies represent a potential future opportunity to utilize AI algorithms for predicting treatment outcomes in breast cancer patients based on mitotic analysis.
2.3. Nuclear features
2.3.1. Prognostic
As the field of digital pathology advances, nuclear architecture has arisen as a potential prognostic and predictive morphological feature in breast cancer. Not only could variations in nuclear shape reflect genetic instability but they may also influence the ability of cancer cells to migrate through tissues and form metastatic deposits that contribute to recurrence [Citation61,Citation62]. Beck et al., 2011 developed a ML-based model (C-Path) for automatically analyzing nuclear and cytoplasmic features from H&E-stained images to predict 5-year survival in two independent cohorts of breast cancer patients (Netherlands Cancer Institute (NKI): n = 248; Vancouver General Hospital (VGH): n = 328) [Citation63]. It was demonstrated that C-Path scores were significantly associated with OS (log-rank p = 0.001) in the test VGH dataset, in comparison to the standard manual pathological grading score assigned to WSIs from the same cohort of patients (log-rank p = 0.29) [Citation63]. Furthermore, Bootstrap analysis on the NKI dataset revealed that the measure of the relative border between spindled stromal nuclei to round stromal nuclei robustly contributed to the C-Path model, with an increased relative border of spindled stromal nuclei to round stromal nuclei being associated with worse prognostic outcomes [Citation63]. Although the biological significance of this morphological feature remains to be elucidated, this observation highlights a potential prognostic relationship between different stromal cell populations in breast cancer.
2.3.2. Predictive
Previous studies have also demonstrated that nuclear features, such as the modified Bloom Richardson (mBR) scale [Citation64], nuclear grade [Citation65], mitotic count [Citation66], and tubule nuclei density [Citation67], highly correlated with Oncotype DX risk category. Whitney et al., 2018 used supervised ML classifiers to catalog digitalized H&E ER+ breast cancer images into Oncotype DX risk categories through nuclear morphological feature evaluation [Citation62]. Interestingly, histomorphometrically, low Oncotype DX and intermediate Oncotype DX cases appeared more similar compared to the high Oncotype DX cases [Citation62]. Moreover, nuclear architecture and the shape of stromal nuclei were identified as the most discriminating epithelial and stromal features, respectively, when stratifying the different risk categories. These morphological features were also assessed by Lu et al., 2018, who investigated whether a computer-extracted measurement of nuclear orientation combined with features relating to nuclear shape and texture were predictive of aggressiveness and prognosis in H&E-stained images from a cohort of 276 early-stage ER+, lymph node negative (LN) breast cancer patients [Citation68]. The image classifier was constructed on an initial modeling group (n = 177) using 12 nuclear orientation features, a measure of the disorder in the orientation of adjacent nuclei, with increased heterogenicity of nuclear orientation being associated with worse survival outcomes reflecting the poorly differentiated, disorganized cell growth observed in aggressive tumors (AUC = 0.67 ± 0.03) [Citation68]. In an independent validation cohort (n = 99), the predictive labels generated by the image classifier correlated with worse survival independent of other variables (HR 3.17, 95% CI: 0.33–30.46, p = 0.01039) [Citation68]. These results highlight a potential prognostic impact of nuclear features when stratifying ER+, LN
breast cancer patients into low and high recurrence risk categories.
The evaluation of tumor nuclear features using AI has also been demonstrated to predict response to treatment in breast cancer. Doddington et al., 2021 developed multiple deep CNNs to automate tumor detection and nuclear segmentation in a cohort of 58 HER2+ and TNBC patients (pCR; n = 37, pathological partial response (pPR); n = 21) to predict response to NAC [Citation69]. Features such as nuclear count, area, circularity, and image-based first- and second-order features including mean pixel intensity and correlation of the Gray-Level Co-Occurrence Matrix (GLCM-COR) were assessed. Multivariate analysis showed that higher nuclear intensity (OR = 1.23, p = 0.018), fewer multifocal/multicentric tumors (OR = 0.14, p = 0.012), and lower GLCM-COR (OR = 0.96, p = 0.043) were each independently associated with the likelihood of achieving a pCR, with a model combining all three features successfully predicting treatment response in 79% of cases (62% for pPR and 89% for pCR) [Citation69]. Saednia et al., 2022 demonstrated similar findings in respect to the predictive efficacy of tumor nuclear features, with their best performing ML algorithm, incorporating GLCM and graph-based features extracted from tumor nuclear masks, achieving a test accuracy of 84%, a sensitivity of 85%, a specificity of 82% and an AUC of 0.9 [Citation70]. More recently, Shen et al., 2023, developed a novel AI pipeline to investigate cancer atypia that utilizes three independent models, including support vector machine (SVM) and random forest models that learn nuclear atypia from fine-grained nuclear features extracted by image analysis method [Citation71]. The CNN model successfully predicted response to NAC in a cohort of 103 breast cancer patients with an accuracy of 95.2% [Citation71]. These studies indicate the potentially predictive significance of tumor nuclear features when combined with digital pathology and AI algorithms in breast cancer.
2.4. Tumor-Stroma ratio
2.4.1. Prognostic
The TME is well known to play a pivotal role in the evolution of malignancies, particularly in the context of solid tumors. The complex organization of numerous cell types (such as adipose, immune, neuroendocrine, and fibroblast cells), blood and lymph vessels, and the extracellular matrix, makes for an environment that can widely influence the hallmarks of cancer [Citation72]. In particular, the tumor-stroma ratio (TSR) has come to light as a prognostic biomarker for solid tumors, with high stromal content (comprising cancer-associated fibroblasts) being associated with poorer outcomes [Citation73]. In fact, TSR was demonstrated to have prognostic impact in 16 solid tumor types (2,732 cancer patients) as shown by Micke et al., 2021, who calculated the ratio using immunofluorescent staining for epithelial markers and ML image analysis algorithms [Citation74]. In the context of breast cancer, Yan et al., 2022 developed a modified TSR assessment algorithm using the open-sourced image-analysis platform QuPath to assess the prognostic utility of TSR in 240 breast cancer patients [Citation75]. Tissue microarrays (TMAs) were stained with cytokeratin immunohistochemistry (IHC) to distinguish between the stromal and tumor areas prior to the application of automated quantitative image-analysis algorithms [Citation75]. Statistical analysis revealed that increased stromal content (low TSR) was associated with worse 5-year disease-free survival (DFS) in the complete breast cancer cohort (p < 0.0001), and when breast cancer was stratified into HER2+ (p < 0.05), TNBC (p < 0.05), and luminal HER2 (p < 0.05) subtypes [Citation75]. Interestingly, TSR was also significantly associated with ER (p = 0.005) and progesterone receptor (PR) status, (p = 0.035), and strongly associated with tumor size (p = 0.051) [Citation75]. These results demonstrate the prognostic impact of TSR in different subtypes of breast cancer [Citation75]. Similar findings were highlighted by Millar et al., 2020, who quantified TSR in digital images of H&E-stained TMAs in a cohort of 647 luminal and TNBC patients [Citation76]. The study demonstrated that in TNBC, low TSR (i.e. high stroma) was associated with OS (OS; HR 1.90, CI 1.10–3.29, p = 0.021) and breast cancer-specific survival (BCSS; HR 2.64, CI 1.31–5.35, p = 0.007) following multivariate analysis after consideration of age, size, grade, sTILs, lymph nodal status and chemotherapy [Citation76]. Additionally, when TSR was combined with the degree of TIL (Low ≤30% ≥ High) improved prognostic performance was demonstrated, with the high TSR/high TILs cohort having a 69% reduction in the risk of death compared to the low TSR/low TILs cohort for TNBC. In contrast, in luminal breast cancer patients, low TSR (i.e. high stroma) predicted favorable outcomes for OS in multivariate analysis independent of age, size, grade, sTILs, lymph nodal status, endocrine therapy and chemotherapy. In terms of BCSS, low TSR was a significant predictor of survival in univariate analysis but not multivariate analysis in luminal tumors [Citation76]. These results not only reflect the potential clinical significance of TSR in predicting breast cancer patient prognosis, but also highlight the inherent differences between the stroma of different subtypes of breast cancer. Although the biological significance of this morphological feature is yet to be elucidated, the findings from this study highlight the prognostic significance of spatial relationships between different stromal cell types [Citation63].
Furthermore, the spatial relationships within the TME were investigated by Abubakar et al., 2021 who applied ML algorithms to an ethnically diverse collection of digitalized H&E sections, comprising 2,084 breast cancer patients from Poland, the United States (US), and China, to determine the prognostic impact of tumor-associated stromal-cellular density (SCD) [Citation77]. SCD, defined as the percentage of tumor-stroma that is occupied by nucleated cells, was demonstrated to be an independent predictor of poor clinical outcomes in luminal cancers patients from all three study populations following multivariate analysis, with high SCD being associated with more aggressive tumors. Furthermore, SCD demonstrated prognostic power beyond standard clinical parameters such as tumor grade, size, subtype, and lymph node status [Citation77]. The prognostic impact of the parameter may be related to the extent of cancer-associated fibroblast (CAF), TIL, and macrophage infiltration which act as a proxy for inflammation [Citation78].
2.4.2. Predictive
Along with demonstrating prognostic utility, increasing evidence suggests that tumor–stroma interactions significantly influence treatment resistance, and therefore patient outcomes, in several cancer types [Citation79,Citation80]. Although studies investigating the predictive value of the tumor–stroma relationship using ML in the context of breast cancer are sparse, Li et al., 2022 developed and applied DL algorithms to predict pCR to NAC in a multicenter dataset comprising of 1,035 patients [Citation81]. The algorithm generated a tumor-associated stroma (TS) score which predicted pCR with an AUC of 0.745, 0.673, and 0.725 in the three validation cohorts. Furthermore, WSI with high TS-scores were associated with greater lymphocyte infiltration, while WSI with lower TS scores typically had a higher abundance of collagen and fibroblasts [Citation82]. In line with these findings, pCR rates for collagen-dominant type stroma, fibroblast-dominant type stroma, and lymphocyte-dominant type stroma were 14.2%, 20.6%, and 42.1%, respectively. These results suggest that information contained within the tumor-associated stroma can be utilized to predict response to NAC, and that stroma with high collagen and fibroblast dominance is associated with a poor response to treatment [Citation82,Citation83].
2.5. Automatically extracted histopathological features using DL
2.5.1. Prognostic
Unlike ML algorithms which require manual feature extraction, DL algorithms possess the ability to automatically extract histopathological features for prognosis prediction. Xu et al., 2021 developed and validated a DL-based primary tumor biopsy signature that successfully predicted axillary lymph node (ALN) metastasis preoperatively in early-stage breast cancer patients who are ALN negative (AUC 0.816) [Citation84]. A DL model to predict breast cancer recurrence was also developed by Phan et al., 2021, who adopted a transfer learning approach that circumvented the need for region of interest labeling (AUC 0.87) [Citation85]. Yang et al., 2022 created a deep CNN that evaluated the risk of relapse and metastasis in HER2+ breast cancer patients using H&E images [Citation86]. The model achieved an AUC of 0.72 when independently tested on 123 HER2+ breast cancer patients from The Cancer Genome Atlas, a cohort with ethnic diversity [Citation86]. Lazard et al., 2022 utilized DL algorithms to predict homologous recombination (HR) status, a marker of platinum salt and polyADP-ribose polymerase inhibitor chemotherapies, in luminal breast cancers from WSIs [Citation87]. More recently, Wahab et al., 2023, developed a DL prognostic algorithm for early-stage luminal HER2 BReAst CanEr, termed BRACE marker [Citation88]. The BRACE marker successfully stratified luminal breast cancer patients for both distant metastasis-free survival (p = 0.001, C-index: 0.73) and breast cancer-specific survival (p < 0.0001, C-index: 0.84), demonstrating prediction accuracy comparable to Nottingham Prognostic Index and Magee scores, which are both derived from manual histopathological assessment [Citation88]. Additionally, DL models have been demonstrated to predict breast cancer recurrence and metastasis by integrating histological H&E images with clinical information and gene expression data (AUC 0.75), highlighting the potential for AI algorithms to not only predict prognosis through histological images but incorporate prognostic information for other modalities which may have additive prognostic power [Citation89].
2.5.2. Predictive
DL algorithms can also be utilized to predict patient response to treatment. Li et al., 2021 developed a DL model that successfully predicted pCR to NAC from H&E images in a cohort of 540 breast cancer patients, with the model achieving an AUC of 0.822, an accuracy of 0.853, and an F1 score of 0.503 [Citation82]. Moreover, when sTILs and breast cancer subtype were incorporated into the model, an AUC of 0.890 was attained, surpassing the performance of the sTILs-subtype model by 0.051 (0.839, p = 0.001) [Citation82]. In line with these findings, Farahmand et al., 2022 developed a DL-CNN that predicted Trastuzumab response in HER2+ breast cancer patients with an AUC of 0.8 [Citation90]. Furthermore, a recent study by Hoang et al., 2022 demonstrated that Deep Pathology for Treatment (DeepPT), a DL model that predicts gene expression profiles directly from histopathological images, could be used in combination with ELIGHT, a platform that predicts patient response to treatment based on their tumor transcriptomics, to predict therapy response in breast and lung cancer patients [Citation91]. Moreover, Huang et al., 2023 utilized the DeepLabV3 model to predict NAC responses in a cohort of 62 HER2+ and 64 TNBC patients from H&E and multiplex IHC (PD-L1, CD8+, and CD163+) images [Citation92]. These results demonstrate, not only that DL algorithms can be applied to H&E images in a prognostic and predictive manner, but that genomic and proteomic data can be inferred from these images to provide a deeper understanding of the relationships within the TME.
3. Challenges of using AI in digital histopathology for cancer research
3.1. Algorithm
Although AI holds the potential to revolutionize the digital pathology field by overcoming subjective visual assessments, reducing the burdensome and time-consuming workflow of Pathologists, thereby improving time to diagnosis/prognosis delivery, there are several challenges that must be considered prior to its implementation ().
Table 1. Digital pathology related artificial intelligence models in oncology.
Table 2. Overview of the possible opportunities and challenges associated with the implementation of artificial intelligence in digital pathology.
3.1.1. Lack of annotated data for algorithm training (small data sets, shortage of pathologists, image quality)
In order to train an AI algorithm, a large sample of annotated (i.e. ‘labelled’) high quality images must be available. These labels delineating the region of interest (ROI) in a tissue section should ideally be curated by expert histopathologists whom may be challenged, not only by time constraints, but also by low resolution images, ambiguity of features, and slow network connectivity [Citation93]. Crowdsourcing is an alternative option to speed up the acquisition of labeled data, however, it possesses the ability to introduce interobserver variability. Furthermore, the curation of labeled data can also be limited by small sample size. In this case, there are several computational approaches that can be employed to maximize the utility of the available samples, such as data augmentation and active learning.
3.1.2. Algorithm validation for integration into the real world
For wide-spread integration of AI into digital pathology workflows, ML, and DL algorithms need to be extensively validated using multi-institutional data to ensure reproducibility of predictions [Citation13]. Variations in slide preparation, scanner models, and image format and analysis all highlight the need for standardized protocols and data normalization techniques to prevent false positive and negative predictions [Citation11,Citation13]. Moreover, different AI models are trained with different datasets of varying cohort sizes, with larger datasets generating more accurate predictions. Therefore, standardizing data format and normalizing data analysis can not only increase generalizability but also can permit the creation of a merged universal dataset which can be used to retrain models to account for the natural variation that exists between different populations [Citation11]. However, no official guidelines are currently available on the number of annotations, images, and laboratories required to capture this natural variation [Citation18]. However, a protocol for the development of a reporting guideline (TRIPOD-AI) and risk of bias tool (PROBAST-AI) has been published [Citation94]. Standardized practices have been developed by the Digital Imaging and Communications in Medicine (DCOM) for other digital images such as X-rays, CTs, ultrasounds, MRIs, with supportive guidelines being outlined for WSIs [Citation95].
3.1.3. Lack of transparency and interpretability (‘Black Box’)
The majority of AI algorithms in the context of digital pathology classify samples in a binary manner, that is, the model output is either a ‘yes’ or a ‘no,’ ‘malignant’ or ‘benign,’ ‘recurrence’ or ‘non-recurrence’ etc [Citation93]. In contrast, real world prognosis delivery by a pathologist encompasses first-hand experience, cognition, clinical context, rational, and descriptive language whereby complex or rare cases are flagged and discussed at multidisciplinary team meetings [Citation93]. These factors highlight the need for the inclusion of a plethora of heterogenous samples in the training set and incorporation of clinical data to improve model decision making. Additionally, the rationale behind sample classification is ambiguous in the context of DL, with algorithms often being referred to as ‘black boxes,’ leading to problems with transparency. This may hinder adoption of AI in the medical setting as clinicians typically justify clinical decisions to patients, which may ultimately affect the patients’ trust.
3.2. Software
3.2.1. Affordability of required computational expenses
A major obstacle to widespread adoption of AI-based digital pathology in clinical settings is the cost of the associated hardware, software, and storage [Citation18,Citation96,Citation97]. The application of deep-learning in AI-based digital pathology relies on the use of specialized hardware. Graphical Processing Units (GPUs), which describe intricate electronic circuits that work to process digital images in AI algorithm training [Citation98]. This often requires the use of specialized computers, as accomplishing AI-based activities on ordinary computers with Central Processing Units often proves slow and impractical. However, the installation and maintenance of a GPU server cluster solely to support the AI activities in a pathology laboratory is extremely costly [Citation98,Citation99].
The cost of long-term data storage of digital images for the development of AI algorithms also poses a significant challenge to the implementation of AI-based procedures [Citation97,Citation100]. WSIs account for hundreds of gigabytes of space and the training data alone must include the many variants of a diagnostic feature as well the varying depths of staining shades noted across different datasets incorporating thousands of WSIs [Citation101,Citation102]. While there is an availability of cloud-based storage solutions, many hospitals depend on internal data storage, and so can affect the cost of long-term storage [Citation102]. Further compounding the financial burden is the lack of standardized reimbursement procedures [Citation96]. While reimbursement codes are accessible for some computational analyses’ costs in countries such as the United States, they are not widely utilized and are regularly rejected [Citation103,Citation104]. Reimbursement models must be developed to incentivize pathology laboratories to incorporate AI into their clinical practice [Citation104].
4. Expert opinion
4.1. Scientific and regulatory hurdles for wide-spread adoption
A lack of value determination and infrastructural support pertaining to AI and digital pathology renders individual laboratories responsible for value interpretation, investment, and cost savings considerations, which may hinder widespread adoption [Citation18]. Furthermore, the sharing of sensitive patient data between clinics, laboratories, digital biobanks, and data scientists can enhance precision medicine while simultaneously increasing data vulnerability [Citation11]. Therefore, it is paramount that data governance, including ethics policies, be created with guidelines on providing informed consent for patients, particularly in relation to the digital data being used to train ML and DL models [Citation102]. Additionally, in order for the wide-spread adoption of AI and digital pathology into the clinic, pathologists must be upskilled in the area of computational pathology, particularly in relation to statistical analysis and data mining, so that if new image-based biomarkers are identified pathologists possess the skills required to fine-tune algorithms accordingly [Citation11].
4.2. Long-term goals for AI in digital pathology
With the rapid development of technological innovations in health care, areas such as digital pathology are being increasingly utilized in numerous specialties such as oncology. The successful incorporation of AI platforms, models, and systems into routine pathology practice requires a multifaceted approach encompassing technological, regulatory, and educational considerations [Citation105]. Robust infrastructure and computational resources are essential for processing and analyzing large volumes of pathology data efficiently. Moreover, interoperability standards and seamless integration with existing laboratory information management systems (LIMS) are crucial to ensure smooth workflow integration. From a regulatory perspective, compliance with data privacy and security regulations such as the General Data Protection Regulation (GDPR) in Europe or the Health Insurance Portability and Accountability Act (HIPAA) in the United States is paramount to safeguard patient confidentiality and data integrity. Furthermore, validation and standardization of AI algorithms is essential to ensure their reliability and reproducibility in the clinical setting, requiring collaboration between regulatory bodies, industry stakeholders, and academic institutions. In addition, clinicians, pathologists, and laboratory staff require comprehensive education and training tools to become familiar with AI tools, understand their capabilities and limitations, and effectively interpret AI outputs. As the field of AI and digital pathology develops, continuous professional development initiatives and interdisciplinary collaboration between pathologists, computer scientists, and data analysis is warranted to foster the innovative environment required for successful adoption of AI into the clinic. It is likely that clinical adoption of AI in digital pathology will begin as a tool to aid pathologists in diagnosing disease by assessing the characteristics of cancer cells, analyzing structural changes in lesions, and examining the expression pattern of biomarkers, leading to more accurate diagnoses, and faster time to prognosis prediction [Citation106]. In line with this, an AI model (PAIGE Prostate) which detects and localizes prostate cancer in needle biopsy specimens has been approved for clinical use by the U.S Food and Drug Administration (FDA) [Citation107]. Nonetheless, as the digital database expands and algorithm accuracy increases, AI may be utilized for more complex tasks such as predicting disease aggressiveness, patient prognosis, and treatment response [Citation106]. ML and DL algorithms may even be used to identify the original site of cancers of unknown primary (CUP) origin [Citation108]. Over time, genomic and proteomic data may be integrated with whole-slide imaging data to lead to the rapid expansion of AI-based computational pathology. Despite the many challenges, AI and digital pathology have extraordinary potential to significantly improve clinical workflows, increasing efficiency of clinical decision making, ultimately benefiting patients.
The adoption of digital pathology into the clinic and generation of large digital databases offers tremendous opportunity for computational analysis using AI. AI and digital pathology possess the capability to further elucidate the complex spatial relationships present in the TME and determine their diagnostic, prognostic, and predictive value. This review highlights the utility of AI to predict both breast cancer patient prognosis, and response to treatment based on several image-based biomarkers. Despite its potential, many ethical, regulatory, structural, and technical challenges remain prior to wide-spread clinical adoption of AI into pathology workflows. Prospective trials investigating the clinical benefit of AI and digital pathology may provide reassurance to both clinicians and patients, increasing trust and promoting its uptake in clinical practice, thereby reducing the delay between diagnosis and prognosis delivery and subsequentially improving patient outcomes.
A single H&E WSI generated from tumor biopsies withholds a plethora of prognostic and predictive information in the form of image-based biomarkers, some of which are discussed in the body of this review. Without the incorporation of AI algorithms to digital pathology workflows, the pragmatic approach would be to omit these biomarkers from clinical decision-making as pathologists’ workloads are too burdensome and their time too limited for manual assessment. Currently, there are several commercially available assays and standardized laboratory tests that predict breast cancer patient prognosis, such as Oncotype DX Recurrence Score, MammaPrint, Prosigna, EndoPredict, IHC4+ C-Score, and Breast Cancer Index [Citation48]. It is evident that a considerable number of these assays are prohibitively expensive and necessitate centralized laboratory infrastructure, skilled technicians, and specialized equipment, which significantly restricts their availability in countries with limited resources. The widespread application of AI algorithms to digital H&E-stained WSIs, has the potential to ameliorate these problems, revolutionizing the pathologist’s workflow by automating tedious time-consuming tasks, improving time efficiency, and reducing workload burden.
Article highlights
Hematoxylin and Eosin tumor images contain a plethora of histopathological information.
The advancement of digital pathology has allowed the application of artificial intelligence algorithms to analyze the tumor microenvironment.
Artificial intelligence algorithms can be applied to analyze histopathological images for prognostic and predictive information.
Overcoming the challenges associated with artificial intelligence can allow for its widespread adoption in the field of oncology.
Declaration of interest
The authors have no relevant affiliations or financial involvement with any organization or entity with a financial interest in or financial conflict with the subject matter or materials discussed in the manuscript. This includes employment, consultancies, honoraria, stock ownership or options, expert testimony, grants or patents received or pending, or royalties.
Reviewer disclosures
Peer reviewers on this manuscript have no relevant financial or other relationships to disclose.
Acknowledgments
We regret that we could not cite all the relevant papers in the research field due to space limitations.
Additional information
Funding
References
- Mobadersany P, Yousefi S, Amgad M, et al. Predicting cancer outcomes from histology and genomics using convolutional networks. Proc Natl Acad Sci, USA. 2018;115(13):E2970–e2979. doi: 10.1073/pnas.1717139115
- Boiesen P, Bendahl PO, Anagnostaki L, et al. Histologic grading in breast cancer–reproducibility between seven pathologic departments. South Sweden breast cancer group. Acta Oncol. 2000;39(1):41–45. doi: 10.1080/028418600430950
- Jahn SW, Plass M, Moinfar F. Digital pathology: advantages, limitations and emerging perspectives. J Clin Med. 2020;9(11) doi: 10.3390/jcm9113697
- Ibrahim A, Gamble P, Jaroensri R, et al. Artificial intelligence in digital breast pathology: techniques and applications. Breast. 2020;49:267–273. doi: 10.1016/j.breast.2019.12.007
- Mazo C, Aura C, Rahman A, et al. Application of artificial intelligence techniques to predict risk of recurrence of breast cancer: a systematic review. J Pers Med. 2022;12(9) doi: 10.3390/jpm12091496
- Fernandez G, Prastawa M, Madduri AS, et al. Development and validation of an AI-enabled digital breast cancer assay to predict early-stage breast cancer recurrence within 6 years. Breast Cancer Res. 2022;24(1):93. doi: 10.1186/s13058-022-01592-2
- Arnold M, Sierra MS, Laversanne M, et al. Global patterns and trends in colorectal cancer incidence and mortality. Gut. 2017;66(4):683. doi: 10.1136/gutjnl-2015-310912
- Polyak K. Heterogeneity in breast cancer. J Clin Invest. 2011;121(10):3786–3788. doi: 10.1172/JCI60534
- Colleoni M, Sun Z, Price KN, et al. Annual hazard rates of recurrence for breast cancer during 24 years of follow-up: results from the International breast cancer study group trials I to V. J Clin Oncol. 2016;34(9):927–935. doi: 10.1200/JCO.2015.62.3504
- Bhattacharyya GS, Doval DC, Desai CJ, et al. Overview of breast cancer and implications of overtreatment of early-stage breast cancer: an Indian perspective. JCO global oncology. 2020;6(6):789–798. doi: 10.1200/GO.20.00033
- Cui M, Zhang DY. Artificial intelligence and computational pathology. Lab Invest. 2021;101(4):412–422. doi: 10.1038/s41374-020-00514-0
- Ahmad Z, Rahim S, Zubair M, et al. Artificial intelligence (AI) in medicine, current applications and future role with special emphasis on its potential and promise in pathology: present and future impact, obstacles including costs and acceptance among pathologists, practical and philosophical considerations. A comprehensive review. Diagn Pathol. 2021;16(1):24. doi: 10.1186/s13000-021-01085-4
- Bera K, Schalper KA, Rimm DL, et al. Artificial intelligence in digital pathology — new tools for diagnosis and precision oncology. Nat Rev Clin Oncol. 2019;16(11):703–715. doi: 10.1038/s41571-019-0252-y
- Adadi A, Berrada M. Peeking inside the black-box: a survey on explainable artificial intelligence (XAI). IEEE Access. 2018;6:52138–52160. doi: 10.1109/ACCESS.2018.2870052
- Janiesch C, Zschech P, Heinrich K. Machine learning and deep learning. Electron Markets. 2021;31(3):685–695. doi: 10.1007/s12525-021-00475-2
- Ching T, Himmelstein DS, Beaulieu-Jones BK, et al. Opportunities and obstacles for deep learning in biology and medicine. J R Soc Interface. 2018;15(141):1.
- Zhu J, Liu M, Li X. Progress on deep learning in digital pathology of breast cancer: a narrative review. Gland Surg. 2022;11(4):751–766. doi: 10.21037/gs-22-11
- Baxi V, Edwards R, Montalto M, et al. Digital pathology and artificial intelligence in translational medicine and clinical practice. Mod Pathol. 2022;35(1):23–32. doi: 10.1038/s41379-021-00919-2
- Iqbal MJ, Javed Z, Sadia H, et al. Clinical applications of artificial intelligence and machine learning in cancer diagnosis: looking into the future. Cancer Cell Int. 2021;21(1):270. doi: 10.1186/s12935-021-01981-1
- Betmouni S. Diagnostic digital pathology implementation: learning from the digital health experience. Digit Health. 2021;7:20552076211020240. doi: 10.1177/20552076211020240
- Rahman A, Jahangir C, Lynch SM, et al. Advances in tissue-based imaging: impact on oncology research and clinical practice. Expert Rev Mol Diagn. 2020;20(10):1027–1037. doi: 10.1080/14737159.2020.1770599
- Pantanowitz L, Sinard JH, Henricks WH, et al. Validating whole slide imaging for diagnostic purposes in pathology: guideline from the College of American Pathologists pathology and Laboratory Quality Center. Arch Pathol Lab Med. 2013;137(12):1710–1722. doi: 10.5858/arpa.2013-0093-CP
- Maibach F, Sadozai H, Seyed Jafari SM, et al. Tumor-infiltrating lymphocytes and their prognostic value in cutaneous melanoma. Front Immunol. 2020;11:2105. doi: 10.3389/fimmu.2020.02105
- Barnes TA, Amir E. HYPE or HOPE: the prognostic value of infiltrating immune cells in cancer. Br j cancer. 2017;117(4):451–460. doi: 10.1038/bjc.2017.220
- Acs B, Ahmed FS, Gupta S, et al. An open source automated tumor infiltrating lymphocyte algorithm for prognosis in melanoma. Nat Commun. 2019;10(1):5440. doi: 10.1038/s41467-019-13043-2
- Hendry S, Salgado R, Gevaert T, et al. Assessing tumor infiltrating lymphocytes in solid tumors: a practical review for pathologists and proposal for a standardized method from the International Immuno-Oncology Biomarkers Working Group: part 1: assessing the host immune response, TILs in invasive breast carcinoma and ductal carcinoma in situ, metastatic tumor deposits and areas for further research. Adv Anat Pathol. 2017;24(5):235.
- Hendry S, Salgado R, Gevaert T, et al. Assessing tumor infiltrating lymphocytes in solid tumors: a practical review for pathologists and proposal for a standardized method from the International Immuno-Oncology Biomarkers Working Group: part 2: TILs in melanoma, gastrointestinal tract carcinomas, non-small cell lung carcinoma and mesothelioma, endometrial and ovarian carcinomas, squamous cell carcinoma of the head and neck, genitourinary carcinomas, and primary brain tumors. Adv Anat Pathol. 2017;24(6):311.
- Swisher SK, Wu Y, Castaneda CA, et al. Interobserver agreement between pathologists assessing tumor-infiltrating lymphocytes (TILs) in breast cancer using methodology proposed by the international TILs working group. Ann Surg Oncol. 2016;23(7):2242–2248. doi: 10.1245/s10434-016-5173-8
- Klauschen F. Scoring of tumor-infiltrating lymphocytes: from visual estimation to machine learning. Seminars in Cancer Biology; 2018.
- Page DB, Broeckx G, Jahangir CA, et al. Spatial analyses of immune cell infiltration in cancer: current methods and future directions: a report of the International Immuno-Oncology Biomarker Working Group on breast cancer. J Pathol. 2023;260(5):514–532. doi: 10.1002/path.6165
- Thagaard J, Broeckx G, Page DB, et al. Pitfalls in machine learning-based assessment of tumor-infiltrating lymphocytes in breast cancer: a report of the International Immuno-Oncology Biomarker Working Group on breast cancer. J Pathol. 2023;260(5):498–513. doi: 10.1002/path.6155
- Bai Y, Cole K, Martinez-Morilla S, et al. An open-source, automated tumor-infiltrating lymphocyte algorithm for prognosis in triple-negative breast cancer. clin cancer res, 2021. Clin Cancer Res. 2021;27(20):5557–5565. doi: 10.1158/1078-0432.CCR-21-0325
- Heindl A, Sestak I, Naidoo K, et al. Relevance of spatial heterogeneity of immune infiltration for predicting risk of recurrence after endocrine therapy of ER+ breast cancer. JNCI: Journal Of The National Cancer Institute. 2018;110(2):166–175. doi: 10.1093/jnci/djx137
- Thagaard J, Stovgaard ES, Vognsen LG, et al. Automated Quantification of sTIL Density with H&E-Based Digital Image Analysis Has Prognostic Potential in Triple-Negative Breast Cancers. Cancers (Basel). 2021;13(12):3050. doi: 10.3390/cancers13123050.
- Makhlouf S, Wahab N, Toss M, et al. Evaluation of tumour infiltrating lymphocytes in luminal breast cancer using artificial intelligence. Br J Cancer. 2023;129(11):1747–1758. doi: 10.1038/s41416-023-02451-3.
- Selli C, Sims AH. Neoadjuvant therapy for breast cancer as a Model for translational research. Breast Cancer. 2019;13:1178223419829072. doi: 10.1177/1178223419829072
- Esserman LJ, Berry DA, DeMichele A, et al. Pathologic complete response predicts recurrence-free survival more effectively by cancer subset: results from the I-SPY 1 TRIAL—CALGB 150007/150012, ACRIN 6657. J Clin Oncol. 2012;30(26):3242–3249. doi: 10.1200/JCO.2011.39.2779
- Cortazar P, Zhang L, Untch M, et al. Pathological complete response and long-term clinical benefit in breast cancer: the CTNeoBC pooled analysis. Lancet. 2014;384(9938):164–172. doi: 10.1016/S0140-6736(13)62422-8
- Haque W, Verma V, Hatch S, et al. Response rates and pathologic complete response by breast cancer molecular subtype following neoadjuvant chemotherapy. Breast Cancer Res Treat. 2018;170(3):559–567. doi: 10.1007/s10549-018-4801-3
- Colleoni M, Viale G, Zahrieh D, et al. Chemotherapy is more effective in patients with breast cancer not expressing steroid hormone receptors: a study of preoperative treatment. Clin Cancer Res. 2004;10(19):6622–6628. doi: 10.1158/1078-0432.CCR-04-0380
- Lee HJ, Cho SY, Cho EY, et al. Artificial intelligence (AI)–powered spatial analysis of tumor-infiltrating lymphocytes (TIL) for prediction of response to neoadjuvant chemotherapy (NAC) in triple-negative breast cancer (TNBC). J Clin Oncol. 2022;40(16_suppl):595–595. doi: 10.1200/JCO.2022.40.16_suppl.595
- Fanucci KA, Bai Y, Pelekanou V, et al. Image analysis-based tumor infiltrating lymphocytes measurement predicts breast cancer pathologic complete response in SWOG S0800 neoadjuvant chemotherapy trial. npj breast cancer. NPJ Breast Cancer. 2023;9(1):38. doi: 10.1038/s41523-023-00535-0
- Choi S, Cho SI, Jung W, et al. Deep learning model improves tumor-infiltrating lymphocyte evaluation and therapeutic response prediction in breast cancer. NPJ Breast Cancer. 2023;9(1):71. doi: 10.1038/s41523-023-00577-4
- Loi S, Salgado R, Schmid P, et al. Association between biomarkers and clinical outcomes of pembrolizumab monotherapy in patients with metastatic triple-negative breast cancer: KEYNOTE-086 exploratory analysis. JCO Precision Oncol. 2023;7(7):e2200317. doi: 10.1200/PO.22.00317
- Park S, Ock C-Y, Kim H, et al. Artificial intelligence–powered spatial analysis of tumor-infiltrating lymphocytes as complementary biomarker for immune checkpoint inhibition in non–small-Cell lung cancer. J Clin Oncol. 2022;40(17):1916–1928. doi: 10.1200/JCO.21.02010
- Li S, Zhang N, Zhang H, et al. Artificial intelligence learning landscape of triple-negative breast cancer uncovers new opportunities for enhancing outcomes and immunotherapy responses. J Big Data. 2023;10(1):132. doi: 10.1186/s40537-023-00809-1
- Tang L, Zhang Z, Fan J, et al. Comprehensively analysis of immunophenotyping signature in triple-negative breast cancer patients based on machine learning. Front Pharmacol. 2023;14. 10.3389/fphar.2023.1195864
- Győrffy B, Hatzis C, Sanft T, et al. Multigene prognostic tests in breast cancer: past, present, future. Breast Cancer Res. 2015;17(1):11. doi: 10.1186/s13058-015-0514-2
- Guillaud M, Ye Q, Leung S, et al. Large-scale DNA organization is a prognostic marker of breast cancer survival. Med Oncol. 2018;35(1):9. doi: 10.1007/s12032-017-1068-1
- Liu KYP, Zhu SY, Harrison A, et al. Quantitative nuclear phenotype signatures predict nodal disease in oral squamous cell carcinoma. PLOS ONE. 2021;16(11):e0259529. doi: 10.1371/journal.pone.0259529
- Enfield KSS, Martin SD, Marshall EA, et al. Hyperspectral cell sociology reveals spatial tumor-immune cell interactions associated with lung cancer recurrence. J Immunother Cancer. 2019;7(1):13. doi: 10.1186/s40425-018-0488-6
- MacAulay C, Keyes M, Hayes M, et al. Quantification of large scale DNA organization for predicting prostate cancer recurrence. cytometry part a. Cytometry Part A. 2017;91(12):1164–1174. doi: 10.1002/cyto.a.23287
- Macaulay C, Guillaud M, Enfield K, et al. P3.09-11 genomic organization at large scales (GOALS) within nuclei and cell sociology for predicting lung cancer outcomes. J Thorac Oncol. 2018;13(10):S952. doi: 10.1016/j.jtho.2018.08.1780
- Rakha EA, Reis-Filho JS, Baehner F, et al. Breast cancer prognostic classification in the molecular era: the role of histological grade. Breast Cancer Res. 2010;12(4):207. doi: 10.1186/bcr2607
- Mahmood T, Arsalan M, Owais M, et al. Artificial intelligence-based mitosis detection in breast cancer histopathology images using faster R-CNN and deep CNNs. J Clin Med. 2020;9(3):9(3. doi: 10.3390/jcm9030749
- Veta M, Pluim JPW, van Diest PJ, et al. Breast cancer histopathology image analysis: a review. IEEE Trans Biomed Eng. 2014;61(5):1400–1411. doi: 10.1109/TBME.2014.2303852
- Pantanowitz L, Hartman D, Qi Y, et al. Accuracy and efficiency of an artificial intelligence tool when counting breast mitoses. Diagn Pathol. 2020;15(1):80. doi: 10.1186/s13000-020-00995-z
- van Bergeijk SA, Stathonikos N, Ter Hoeve ND, et al. Deep learning supported mitoses counting on whole slide images: a pilot study for validating breast cancer grading in the clinical workflow. J Pathol Inform. 2023;14:100316.
- Ibrahim A, Jahanifar M, Wahab N, et al. Artificial intelligence-based mitosis scoring in breast cancer: clinical application. Mod Pathol. 2024;37(3):100416. doi: 10.1016/j.modpat.2023.100416
- Jaroensri R, Wulczyn E, Hegde N, et al. Deep learning models for histologic grading of breast cancer and association with disease prognosis. NPJ Breast Cancer. 2022;8(1):113. doi: 10.1038/s41523-022-00478-y
- Gisselsson D, Björk J, Höglund M, et al. Abnormal nuclear shape in solid tumors reflects mitotic instability. Am J Pathol. 2001;158(1):199–206. doi: 10.1016/S0002-9440(10)63958-2
- Whitney J, Corredor G, Janowczyk A, et al. Quantitative nuclear histomorphometry predicts oncotype DX risk categories for early stage ER+ breast cancer. BMC Cancer. 2018;18(1):610. doi: 10.1186/s12885-018-4448-9
- Beck AH, Sangoi AR, Leung S, et al. Systematic analysis of breast cancer morphology uncovers stromal features associated with survival. Sci Transl Med. 2011;3(108):108ra113. doi: 10.1126/scitranslmed.3002564
- Mina L, Soule SE, Badve S, et al. Predicting response to primary chemotherapy: gene expression profiling of paraffin-embedded core biopsy tissue. Breast Cancer Res Treat. 2007;103(2):197–208. doi: 10.1007/s10549-006-9366-x
- Flanagan MB, Dabbs DJ, Brufsky AM, et al. Histopathologic variables predict oncotype DX™ recurrence score. Mod Pathol. 2008;21(10):1255–1261. doi: 10.1038/modpathol.2008.54
- Romo-Bucheli D, Janowczyk A, Gilmore H, et al. A deep learning based strategy for identifying and associating mitotic activity with gene expression derived risk categories in estrogen receptor positive breast cancers. Cytometry Part A. 2017;91(6):566–573. doi: 10.1002/cyto.a.23065
- Romo-Bucheli D, Janowczyk A, Gilmore H, et al. Automated tubule nuclei quantification and correlation with Oncotype DX risk categories in ER+ breast cancer whole slide images. Sci Rep. 2016;6(1):32706. doi: 10.1038/srep32706
- Lu C, Romo-Bucheli D, Wang X, et al. Nuclear shape and orientation features from H&E images predict survival in early-stage estrogen receptor-positive breast cancers. Lab Invest. 2018;98(11):1438–1448. doi: 10.1038/s41374-018-0095-7
- Dodington DW, Lagree A, Tabbarah S, et al. Analysis of tumor nuclear features using artificial intelligence to predict response to neoadjuvant chemotherapy in high-risk breast cancer patients. Breast Cancer Res Treat. 2021;186(2):379–389. doi: 10.1007/s10549-020-06093-4
- Saednia K, Lagree A, Alera MA, et al. Quantitative digital histopathology and machine learning to predict pathological complete response to chemotherapy in breast cancer patients using pre-treatment tumor biopsies. Sci Rep. 2022;12(1):9690. doi: 10.1038/s41598-022-13917-4
- Shen B, Saito A, Ueda A, et al. Development of multiple AI pipelines that predict neoadjuvant chemotherapy response of breast cancer using H&E-stained tissues. J Pathol. 2023;9(3):182–194. doi: 10.1002/cjp2.314.
- Wang M, Zhao J, Zhang L, et al. Role of tumor microenvironment in tumorigenesis. J Cancer. 2017;8(5):761–773. doi: 10.7150/jca.17648
- Wu J, Liang C, Chen M, et al. Association between tumor-stroma ratio and prognosis in solid tumor patients: a systematic review and meta-analysis. Oncotarget. 2016;7(42):68954–68965. doi: 10.18632/oncotarget.12135
- Micke P, Strell C, Mattsson J, et al. The prognostic impact of the tumour stroma fraction: a machine learning-based analysis in 16 human solid tumour types. EBioMedicine. 2021;65:103269. doi: 10.1016/j.ebiom.2021.103269.
- Yan D, Ju X, Luo B, et al. Tumour stroma ratio is a potential predictor for 5-year disease-free survival in breast cancer. BMC Cancer. 2022;22(1):1082. doi: 10.1186/s12885-022-10183-5
- Millar EK, Browne LH, Beretov J, et al. Tumour stroma ratio assessment using digital image analysis predicts survival in triple negative and luminal breast cancer. Cancers (Basel). 2020;12(12):3749. doi: 10.3390/cancers12123749
- Abubakar M, Zhang J, Ahearn TU, et al. Tumor-Associated Stromal Cellular Density as a predictor of recurrence and mortality in breast cancer: results from ethnically diverse study populations. Cancer Epidemiol Biomarkers Prev. 2021;30(7):1397–1407. doi: 10.1158/1055-9965.EPI-21-0055
- Raskov H, Orhan A, Gaggar S, et al. Cancer-associated fibroblasts and tumor-associated macrophages in cancer and cancer immunotherapy. Front Oncol. 2021;11:668731. doi: 10.3389/fonc.2021.668731
- Ni Y, Zhou X, Yang J, et al. The role of tumor-stroma interactions in drug resistance within tumor microenvironment. Front Cell Dev Biol. 2021;9:637675. doi: 10.3389/fcell.2021.637675
- Valkenburg KC, de Groot AE, Pienta KJ. Targeting the tumour stroma to improve cancer therapy. Nat Rev Clin Oncol. 2018;15(6):366–381. doi: 10.1038/s41571-018-0007-1
- Li F, Yang Y, Wei Y, et al. Predicting neoadjuvant chemotherapy benefit using deep learning from stromal histology in breast cancer. NPJ Breast Cancer. 2022;8(1):124. doi: 10.1038/s41523-022-00491-1
- Li F, Yang Y, Wei Y, et al. Deep learning-based predictive biomarker of pathological complete response to neoadjuvant chemotherapy from histological images in breast cancer. J Transl Med. 2021;19(1):348. doi: 10.1186/s12967-021-03020-z
- Li B, Li F, Liu Z, et al. Deep learning with biopsy whole slide images for pretreatment prediction of pathological complete response to neoadjuvant chemotherapy in breast cancer: A multicenter study. Breast. 2022;66:183–190. doi: 10.1016/j.breast.2022.10.004
- Xu F, Zhu C, Tang W, et al. Predicting axillary lymph node metastasis in early breast cancer using deep learning on primary tumor biopsy slides. Front Oncol. 2021;11:11. doi: 10.3389/fonc.2021.759007
- Phan NN, Hsu C-Y, Huang C-C, et al. Prediction of breast cancer recurrence using a deep convolutional neural network without region-of-interest labeling. Front Oncol. 2021;11:734015. doi: 10.3389/fonc.2021.734015
- Yang J, Ju J, Guo L, et al. Prediction of HER2-positive breast cancer recurrence and metastasis risk from histopathological images and clinical information via multimodal deep learning. Computat Struct Biotechnol j. 2022;20:333–342.
- Lazard T, Bataillon G, Naylor P, et al. Deep learning identifies morphological patterns of homologous recombination deficiency in luminal breast cancers from whole slide images. Cell Reports Med. 2022;3(12):100872. doi: 10.1016/j.xcrm.2022.100872
- Wahab N, Toss M, Miligy IM, et al. AI-enabled routine H&E image based prognostic marker for early-stage luminal breast cancer. NPJ Precis Oncol. 2023;7(1):122. doi: 10.1038/s41698-023-00472-y
- Yao Y, Lv Y, Tong L, et al. ICSDA: a multi-modal deep learning model to predict breast cancer recurrence and metastasis risk by integrating pathological, clinical and gene expression data. Brief Bioinform. 2022;23(6): doi: 10.1093/bib/bbac448
- Farahmand S, Fernandez AI, Ahmed FS, et al. Deep learning trained on hematoxylin and eosin tumor region of interest predicts HER2 status and trastuzumab treatment response in HER2+ breast cancer. Mod Pathol. 2022;35(1):44–51. doi: 10.1038/s41379-021-00911-w.
- Hoang D-T, Ben-Zvi D, Hermida LC, et al. Predicting patient response to cancer therapy via histopathology images. J Clin Oncol. 2022;40(16_suppl):e13561–e13561. doi: 10.1200/JCO.2022.40.16_suppl.e13561
- Huang Z, Shao W, Han Z, et al. Artificial intelligence reveals features associated with breast cancer neoadjuvant chemotherapy responses from multi-stain histopathologic images. NPJ Precis Oncol. 2023;7(1):14. doi: 10.1038/s41698-023-00352-5.
- Tizhoosh HR, Pantanowitz L. Artificial intelligence and Digital Pathology: challenges and opportunities. J Pathol Inform. 2018;9(1):38–38. doi: 10.4103/jpi.jpi_53_18
- Collins GS, Dhiman P, Andaur Navarro CL, et al. Protocol for development of a reporting guideline (TRIPOD-AI) and risk of bias tool (PROBAST-AI) for diagnostic and prognostic prediction model studies based on artificial intelligence. BMJ Open. 2021;11(7):e048008. doi: 10.1136/bmjopen-2020-048008
- Clunie D, Hosseinzadeh D, Wintell M, et al. Digital imaging and communications in medicine whole slide imaging connectathon at digital pathology association pathology visions 2017. J Pathol Inform. 2018;9(1):6. United States. doi: 10.4103/jpi.jpi_1_18.
- Colling R, Pitman H, Oien K, et al. Artificial intelligence in digital pathology: a roadmap to routine use in clinical practice. J Pathol. 2019;249(2):143–150. doi: 10.1002/path.5310
- Sakamoto T, Furukawa T, Lami K, et al. A narrative review of digital pathology and artificial intelligence: focusing on lung cancer. Transl Lung Cancer Res. 2020;9(5):2255–2276. doi: 10.21037/tlcr-20-591
- Drogt J, Milota M, Vos S, et al. Integrating artificial intelligence in pathology: a qualitative interview study of users’ experiences and expectations. Mod Pathol. 2022;35(11):1540–1550. doi: 10.1038/s41379-022-01123-6
- Flach RN, Fransen NL, Sonnen AFP, et al. Implementation of artificial intelligence in diagnostic practice as a next step after going digital: the UMC utrecht perspective. Diagn (Basel). 2022;12(5):12(5. doi: 10.3390/diagnostics12051042
- Van Es SL. Digital pathology: semper ad meliora. Pathology. 2019;51(1):1–10. doi: 10.1016/j.pathol.2018.10.011
- Calderaro J, Kather JN. Artificial intelligence-based pathology for gastrointestinal and hepatobiliary cancers. Gut. 2021;70(6):1183. doi: 10.1136/gutjnl-2020-322880
- Hanna MG, Hanna MH. Current applications and challenges of artificial intelligence in pathology. Human Pathol Rep. 2022;27:300596. doi: 10.1016/j.hpr.2022.300596
- Coccia M. Deep learning technology for improving cancer care in society: new directions in cancer imaging driven by artificial intelligence. Technol Soc. 2020;60:101198. doi: 10.1016/j.techsoc.2019.101198
- Viswanathan VS, Toro P, Corredor G, et al. The state of the art for artificial intelligence in lung digital pathology. J Pathol. 2022;257(4):413–429. doi: 10.1002/path.5966
- Cheng JY, Abel JT, Balis UGJ, et al. Challenges in the development, deployment, and regulation of artificial intelligence in anatomic pathology. Am J Pathol. 2021;191(10):1684–1692. doi: 10.1016/j.ajpath.2020.10.018
- Go H. Digital Pathology and Artificial Intelligence Applications in Pathology. Brain Tumor Res Treat. 2022;10(2):76–82. doi: 10.14791/btrt.2021.0032
- Stephens K. FDA authorizes prostate AI software. ProQuest: AXIS Imaging News; 2021.
- Lu MY, Chen TY, Williamson DFK, et al. AI-based pathology predicts origins for cancers of unknown primary. Nature. 2021;594(7861):106–110. doi: 10.1038/s41586-021-03512-4