Abstract
Quantitative structure-activity relationship (QSAR) studies have been carried out on 4-anilino-3-quinolinecarbonitriles, a set of novel Src kinase inhibitors, with the aim of dissecting the structural requirements for Src inhibitory activities. After outlier identification using robust principal component analysis (robust PCA), linear models based on forward selection combined with multiple linear regression, (FS-MLR), enhanced replacement method followed by multiple linear regression (ERM) and a nonlinear model using support vector regression (SVR) were constructed and compared. All models were rigorously validated using leave-one-out cross-validation (LOOCV), 5-fold cross-validations (5-CV) and shuffling external validation (SEVs). ERM seems to outperform both FS-MLR and SVR evidenced by better prediction performance (n = 35, R2training = 0.918, R2pred = 0.928). Robustness and predictive ability of ERM model were also evaluated. The generated QASR model revealed that the Src inhibitory activity of 4-anilino-3-quinolinecarbonitriles could be associated with the size of substituents in the C7 position and the steric hindrance effect. The results of the present study may be of great help in designing novel 4-anilino-3-quinolinecarbonitriles with more potent Src kinase inhibitory activity.
Introduction
The Src family kinases (SFKs), a family of nonreceptor tyrosine kinases, including Src, Yes, Lck, Fyn, Lyn, Fgr, Hck, Blk and Yrk, are involved in the regulation of a wide variety of normal cellular transduction pathways, such as cell growth, differentiation, survival, adhesion and migration [Citation1], and are maintained in an inactive conformation in the absence of extracellular and intracellular stimuli. However, considerable evidences implicates elevated expression and/or activity of Src kinases in many human cancers (e.g., colon, rectal, or stomach cancer) [Citation2,Citation3], osteoporosis [Citation4], cardiovascular disorders [Citation5] and immune system dysfunction [Citation6]. Thus, this family of protein tyrosine kinase now exists as intriguing targets for both basic research and drug discovery.
Currently, numerous efforts have been devoted to the design of Src kinase inhibitors, with most attentions through an ATP-competitive inhibition mechanism. Several Src kinase inhibitors have been identified to date. These include various heteroaromatic compounds, such as pyrazolopyrimidines, pyrrolopyrimidines, pyridopyrimidines, quinazolines, quinolines, indolinones, isoquinoline and others [Citation7]. A series of 4-anilino-3-quinolinecarbonitriles developed by Boschelli and co-workers [Citation8–10] exhibited potent Src kinase inhibiting activity. Considering the important role of Src kinase in regulating normal cellular functions and recent interest in development of such inhibitors, a QSAR investigation of these compounds is carried out. The objective of this study is to analyze the physicochemical and structural requirements of these inhibitors to exhibit optimal inhibitory potency of Src kinase which will in turn help in rationalizing the design of these molecules as Src kinase inhibitors, as well as to provide a strategy for predicting activities of novel 4-anilino-3-quinolinecarbonitriles with high accuracy.
Materials and methods
Dataset and descriptors
The data set consisting of 37 4-anilino-3-quinolinecarbonitriles together with their Src kinase inhibitory activities, expressed as log(1/IC50), was obtained from references [Citation8–10]. The structure of the compounds were drawn using MDL® ISIS/Draw (Symyx Technologies, Inc.) implemented in the ISIS 2.5 package and pre-optimized using molecular mechanics force field (MM+) encoded in HyperChem (Version 8.04, Hypercube, Inc.). The final refined equilibrium molecular geometrics were obtained using the semiempirical method PM3 (Parametric Method-3). We chose a gradient norm limit of 0.01 kcal/Ao for the geometry optimization. More than one thousand meaningful descriptors were calculated for each compound using E-Dragon version 1.1 [Citation11], encoding different aspects of the molecular structures. These descriptors consist of constitutional, topological, electronic, thermodynamic, geometric descriptors, etc. Descriptors with same entries for most of the training compounds were removed from the pool of variables considered. Pairs of variables with correlation coefficients greater than 0.90 were considered as inter-correlated, and one of them in each correlated pair was deleted. Finally, the resulting data matrix was utilized for further analysis.
Robust PCA
Although principal component analysis (PCA) is a very popular dimension reduction technique, the results are highly affected by anomalous observations in the data. To avoid the sensitivity towards outliers, various robust PCA algorithms [Citation12,Citation13] have recently been developed. The algorithm of ROBPCA utilized in this study combines projection pursuit techniques with robust covariance estimation in lower dimensions and could be concluded as three stages: first, the data matrix is processed by reducing its data space to the affine subspace spanned by the number of observations; then a measure of outlyingness is computed for each data point, which is obtained by projecting the high-dimensional data points on many univariate directions; the last stage of ROBPCA consists of selecting the number of principal components (k) to retain and projecting the data points onto the k-dimensional subspace spanned by the k largest eigenvectors and of computing their center and shape by means of the reweighted MCD estimator. The eigenvectors of this scatter matrix then determine the robust principal components, and the MCD estimation estimate serves as a robust center. For visualization, we also represent the result of the PCA analysis by means of a diagnostic plot based on orthogonal distance and score distances. The orthogonal distance measures the distance between an observation and its projection in the k-dimensional PCA subspace, while the score distance is measured within the PCA subspace. Thus robust PCA might serve as a valuable tool for outlier detection [Citation13,Citation14], and any observations with large orthogonal distance or score distance would be identified as potential outliers. More information about ROBPCA algorithm could be obtained in reference [Citation15]. ROBPCA is carried out by using the Matlab Toolbox [Citation16] for Robust Calibration.
FS-MLR
For simplicity and interpretability, multiple linear regression (MLR) [Citation17] was employed as the modeling method and a multiple-term linear equation is built step-by-step using forward selection (FS) strategy. To illustrate the process concisely, two descriptor pools need to be defined first. Pool1 indicates the descriptors which have been selected into the MLR model and pool2 deposits the remaining descriptors. In each step, the performance of each descriptor in pool2 in combination with those in pool1 is evaluated and the best one would be transferred from pool2 to pool1. The search process is terminated when stepping is no longer possible or when a specified maximum number of steps has been reached. FS-MLR model is achieved using JMP (Version 5.1, SAS.) with parameters of ‘Prob to Enter’ and ‘Prob to leave’ set default as 0.250 and 0.100, respectively.
ERM
Enhanced Replacement Method (ERM) is a modified version of replacement method (RM) proposed by Andrew G. Mercader et al [Citation18,Citation19] for variable selection in linear models. For RM, it approaches the minimum of standard error of regression (S) by judiciously taking into account the relative error of the coefficients of the least-squares model given by a set of d descriptors. ERM follows the same RM philosophy but exhibits less propensity for remaining in local minima and at the same time is less dependent on the initial solution. More information of this algorithm could be obtained in reference [Citation18]. ERM is run by using Matlab (Version 7.2b, The Mathworks, Inc.).
Support vector regression (SVR)
Support vector machine (SVM), developed by Vapnik and Cortes [Citation20], as a novel type of machine learning method, is gaining increasing popularity due to many attractive features and promising empirical performances. Besides the basic aim of SVM for data classification, an extension of this algorithm named support vector regression (SVR) has been developed to address regression problems. Briefly, a regression task usually involves training and test data which consist of some data instances. Each instance in the training set contains one “target value” (property value) and several “attributes” (features). The goal of SVM is to produce a model which predicts target value of data instances in the test set which are given only the attributes. In this study, SVR was performed with RBF as the kernel function. The parameters C and γ were set default, with C=1 and γ=1/k, where k means the number of attributes in the input data. All calculations in this work were carried out by using Matlab (Version 7.2b, The Mathworks, Inc.) and the SVM toolbox was developed by Chih et al. [Citation21] The calculations were performed on a 1.80GHz Intel Pentium Dual E2160 with 2G RAM under windows XP.
Cross-validation
Cross-validation techniques [Citation22,Citation23] including leave-one-out cross-validation (LOOCV) and 5-fold cross-validation (5-CV) were employed to evaluate the performance of both linear an nonlinear models. In LOOCV, only one sample is selected as the test set for each time, and the other samples are used as training set to predict the selected sample. The process is repeated until all the samples have been removed once. While for 5-CV, the whole dataset is classified into 5 subsets. Each time, samples in one of the subsets are selected as the test set, while remaining samples are used as training set to predict the test set. This procedure is repeated for 5 times until each subset has been removed once as the test set. However, because the 5-CV results vary for each run due to random partitioning of the data set, the whole process is repeated for 20 times to eliminate the effect of random sample partitioning in this study. The average result of the multiple cross-validation runs provides an unbiased assessment of the model performance in predicting unknown compounds. The models were evaluated by measuring the prediction R2 (explained variance), RMS (root-mean-square error), and RSE (relative standard error), with the formulations shown as follows:
In the above equations, yexp and ypred are experimental and predicted log(1/IC50) values, respectively; n is the number of samples in the data set of interest. d is the number of variables.
External validation, shuffling external validations and training set selection
It needs to be emphasized that no matter how robust, significant and validated a QSAR model may be, it can not be expected to reliably predict the modeled activity for the entire universe of compounds. Therefore, the performance of the selected descriptors was further evaluated by external validation. However, it is also well known that a QSAR model’s ability to predict the properties of unknown chemicals depends largely on the nature of the training set and a model’s predictive accuracy and confidence for different unknown chemicals varies according to how well the training set represents the unknown chemicals. Thus 28 representative compounds were carefully selected as the training set using principal component analysis (PCA), taking sample distribution into consideration.
Moreover, considering the fact that the results of external validation are to some extent highly unstable due to the different selection of training sets, we also employed shuffling external validations (SEVs) to eliminate as most as possible the effect of different training sets and to evaluate model performance in a more objective way. Concisely, in each shuffling, 28 compounds are randomly selected as training set and the others as test set, ensuring that activity of compounds in the training set covering the range of 5.400 to 9.120. This procedure is repeated 20 times to eliminate the effects of random selection of training samples, with the averaged results used for model evaluation.
Y-randomization and predictive ability analysis of ERM model
Y-randomization analysis [Citation24] is implemented for further ensuring the robustness of ERM model. The dependent variable (log(1/IC50) values) is randomly shuffled and a new QSAR model is developed using the original independent variable matrix. The new QSAR models (after several repetitions) are expected to have low R2, high RMS and S. If the opposite happens, then an acceptable QSAR model cannot be obtained for the specific modeling method and data.
According to the Tropsha et al. [Citation25], the predictive power of a QSAR model can be conveniently estimated by the following equations:
Calculations relating to R2cv,ext, Ro2 and the slope k and k′ are based on regression of observed values against predicted values and vice versa. They were discussed in detail in reference [Citation25,Citation26].
Results and discussion
As a first step, robust principal component analysis (robust PCA) was performed on a complete set of 37 4-anilino-3-quinolinecarbonitriles to ensure whether potential outliers exist in this data set. The resulting plot of orthogonal distance versus score distance is illustrated in , where large deviations from the cluster center for samples 36 and 37 indicate the potential outlying nature of these compounds. Restated, large orthogonal distance indicates the large deviation between these two compounds to their projection in the k-dimensional PCA subspace, while large score distance implies a large distance between the projections of them to that of the other samples in the k−dimensional PCA space. Thus samples 36 and 37 are removed from further analysis as potential outliers.
The biological activity values [IC50 (nM)] reported in the literature were converted to molar units [mol/l] and then further to –log scale and subsequently used as the response variable for the QSAR analysis. The log(1/IC50) values, along with the structure of all compounds including outliers are presented in . The compounds excluding outliers were divided into training and test sets containing 28 and 7 molecules respectively, with the detailed distribution shown in . The training set has been used for QSAR model development and the test set was used to test the ability of developed QSAR model in predicting the Src kinase inhibiting activity.
Table 1. Compound, experimental and calculated log(1/IC50) values, as well as corresponding residuals based on ERM model.
Linear models (FS-MLR, ERM)
For simplicity and interpretability, multiple linear regression model was developed using both forward selection (FS) and enhanced replacement method (ERM) as variable selection strategy. For each model, a specific set of four descriptors were finally involved. The resulting regression model combined with forward selection was as follows:
n = 28, R2 = 0.886, RMS = 0.268, RSE = 0.033 (training set)
n = 7, R2 = 0.892, RMS = 0.263, RSE = 0.033 (test set)
ERM in combination with MLR results in a better regression model, with the equation shown as follows:
n = 28, R2 = 0.918, RMS = 0.228, RSE = 0.028 (training set)
n = 7, R2 = 0.928, RMS = 0.241, RSE = 0.030 (test set)
In the models above, n is the number of compounds, R2 is explained variance, RMS is root mean square error and RSE is relative standard error. The figures given in the parentheses with ± sign in the model are 95% confidence limits. It should be noted that the same training and test sets are utilized for FS-MLR and ERM, which ensures the comparability of both models. Since ERM outperforms FS as a variable selection strategy evidenced by significantly higher value of R2, only the results obtained using ERM would be illustrated in detail. For visualization, a graphical representation of the experimental versus predicted log(1/IC50) values, as well as the residuals, is illustrated in and , respectively. The detailed information of selected descriptors is shown in . As a confirmation, the model mentioned above was also utilized to predict samples 36 and 37. The abnormal large residuals for both samples shown in confirmed their outlyingness to a large extent.
Figure 4. Plot of residuals against the experimental log(1/IC50) values using ERM for samples in training and test sets, as well as outliers.
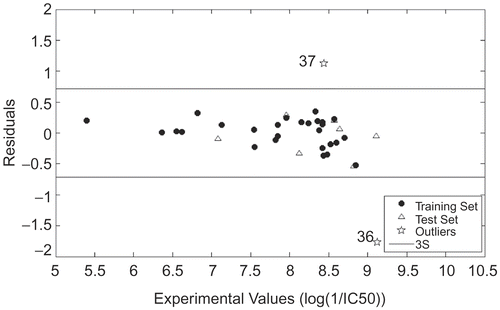
Table 2. Molecular descriptors selected in models.
Nonlinear model (SVR)
Support vector regression (SVR) is an extension of support vector machine (SVM), with the aim of addressing regression problems. For SVR, forward variable selection method was also employed. By stepwise addition of the most important descriptors, the best SVR model was achieved when another four descriptors (RDF150e, Mor18u, C-025, C-034) were involved, with R2training = 0.855 and R2test = 0.804 for samples in training and test sets, respectively. Descriptors utilized in SVR are also illustrated clearly in .
Models validation and comparison
Cross-validation techniques including LOOCV and 5-CV were employed to evaluate the performance of these models. However, it needs to be emphasized that the real performance of any model could only be revealed using an external validation set. Therefore, the performance of these models was further evaluated by external validation. The detailed validation results are shown in , demonstrating that despite the much more sophisticated algorithm of SVR, ERM significantly outperforms SVR and FS-MLR with better performances. Considering that such inferiority of SVR could also be due to the selection bias of training samples, shuffling external validations (SEVs) was also implemented. The superiority of this method to the traditional external validation could be concluded as follows: first, compared to the random selection of training samples utilized in traditional external validation, this method takes sample distribution into consideration, ensuring samples in the training set covering the activity range of 5.400 to 9.120 in each shuffling; Moreover, average of 20 shuffles is chosen as the final external validation result, excluding to a large extent the bias resulted from different selection of training samples. The results of SEVs demonstrated in confirmed the significantly better performance of ERM. Thus only descriptors selected in ERM model would be extensively discussed.
Table 3. Statistical results of performance validation.
Y-randomization and predictive ability analysis of ERM model
Y-randomization analysis is implemented for further ensuring the robustness of ERM model, with detailed results shown in . The low R2 and high RMS indicate that the good results in our models are not due to a chance correlation or structural dependency of the training set. Finally, the ERM model also passed the predictive ability analysis, with detailed results illustrated in .
Table 4. Y-randomization result of ERM model.
Table 5. Predictive ability analysis result of ERM model.
Explanation of molecular descriptors
Considering the significantly better performance, only descriptors selected in ERM model would be extensively discussed in this study. The inter-correlation of these descriptors was evaluated and illustrated in , indicating no significant information overlapping among them. For evaluating the significance of each descriptor, we ranked the descriptors in ERM model according to their effect on increasing the value of S when removed from the model. In this case, the order found is:
JGI6 > Mor23v > RDF060e > JGI9
Table 6. Correlation matrix for descriptors selected in ERM model.
The most important descriptor JGI6 and the least one JGI9 belong to the family of topological charge index JGI. [Citation27,Citation28] Galvez Charge Indices GGIk and JGIk are defined as:
where N is the number of vertices (atoms different to hydrogen) in the molecular graph, and k the length of each path. CTij= mij-mji. ‘m′ stands for the elements of the M matrix, M=A×D*, A is the adjacency (N×N) matrix of the molecular graph, D* is the inverse square distance matrix, in which their diagonal entries are assigned as 0, and δ is Kronecker’s delta. Thus, JGIk represents the average of the CTij terms, with Dij=k, being Dij the entries of the topological distance matrix (D). In the Charge Indices terms, the presence of heteroatoms is taken into account by introducing their electronegativity values in the corresponding entry of the main diagonal of the adjacency matrix. These indices represent a strictly topological quantity plausibly correlating with the charge distribution inside the molecule. In other words, the topological distance of substituents in C7 position plays an important role in determining the Src inhibitory activity. The positive coefficient of JGI6 indicates that the more the substituents with path lengths of 6, the higher the Src inhibitory activity might be, while the negative sign of JGI9 indicates an opposite effect, when path length is 9. This distribution is an important property, which conditions the behavior of many physiochemical and biological properties.
The 3D-MoRSE type of descriptor is obtained considering a molecular transform derived from an equation used in electron diffraction studies. [Citation27,Citation29] The electron diffraction does not directly yield atomic coordinates, but provides diffraction patterns from which the atomic coordinates are derived by mathematical transformations. These codes are defined in order to reflect the contribution at a prescribed scattering angle of an atomic property such as mass (m), polarizability (p), electronegativity (e) or volume (v) to the property under investigation, and so enabling to differentiate the nature of atoms. Mor23v, with the scattering angle of 23 Å−1, is weighted by atomic volumes, and the negative coefficient might indicate the adverse molecular volume in improving the Src inhibitory activity.
RDF060e [Citation27,Citation30] belongs to the family of radial distribution function, which can act as a structure coding technique referred to as radial distribution function code (RDF code) to transform the 3D coordinates of the atoms of molecules into a structure code that has a fixed number of descriptors irrespective of the size of a molecule. Radial distribution function provides, besides information about interatomic distances in a whole molecule, the opportunity to gain access to other valuable information, for example, bond distance, ring types, planar and nonplanar systems and atoms types. RDF060e has a negative influence in the studied property, possibly decreasing the Src kinase inhibiting activity. This descriptor is weighted with atomic Sanderson electronegativities, and most significantly, this descriptor is corresponding to a sphere radius of 6.0 angstroms. Formally, the radial distribution function of an ensemble of n atoms can be interpreted as the probability distribution to find an atom in a spherical volume of radius R. In this sense, according to our ERM model, a spherical molecular volume with this dimension could have certain restrictions to the addition of substituents. This interpretation suggests that substituent in C6 position of 4-anilino-3-quinolinecarbonitriles might contribute negatively to the Src inhibitory activity when bulky substituents exist in C7 positions at the same time. This observation agrees with the explanation reported by Berger et al [Citation9].
Conclusions
Summarizing the above discussion, the present study gives rise to QSAR model with good statistical significance and predictive capacity for Src kinase inhibitory activity of 4-anilino-3-quinolinecarbonitriles. The result of this study suggests that the variables like RDF060e, Mor23v, JGI6 and JGI9 index play an important role in defining such inhibitory activity. The analysis, based on validation procedures, offers not only an accurate strategy for predicting Src inhibitory activity of novel 4-anilino-3-quinolinecarbonitriles, but also a useful guidance to synthesize novel analogs with potent activity against Src kinase.
Acknowledgment
Declaration of interest: The authors report no conflicts of interest. The authors alone are responsible for the content and writing of the paper.
References
- Thomas SM, Brugge JS. Cellular functions regulated by Src family kinases. Annu Rev Cell Dev Biol (1997); 13: 513–609.
- Cartwright CA, Meisler AI, Eckhart W. Activation of the pp60c-src protein kinase is an early event in colonic carcinogenesis. Proc Natl Acad Sci USA (1990); 87: 558–562.
- Mao WG, Irby R, Coppola D, Fu L, Wloch M, Turner J, Yu H, Garcia R, Jove R, Yeatman TJ. Activation of c-Src by receptor tyrosine kinases in human colon cancer cells with high metastatic potential. Oncogene (1997); 15: 3083–3090.
- Soriano P, Montgomery C, Geske R, Bradley A. Targeted disruption of the c-src proto-oncogene leads to osteopetrosis in mice. Cell (1991); 64: 693–702.
- Paul R, Zhang ZG, Eliceiri BP, Jiang Q, Boccia AD, Zhang RL, Chopp M, Cheresh DA. Src deficiency or blockade of Src activity in mice provides cerebral protection following stroke. Nat Med (2001); 7: 222–227.
- Kamens JS, Ratnofsky SE, Hirst GC. Lck inhibitors as a therapeutic approach to autoimmune disease and transplant rejection. Curr Opin Invest Drugs (2001); 2: 1213–1219.
- Parang K, Sun GQ. Recent advances in the discovery of Src kinase inhibitors. Expert Opin Ther Pat (2005); 15:1183–1207.
- Boschelli DH, Wang DY, Ye F, Yamashita A, Zhang N, Powell D, Weber J, Boschelli F. Inhibition of Src kinase activity by 4-anilino-7- thienyl-3-quinolinecarbonitriles. Bioorg Med Chem Lett (2003); 12: 2011–2014.
- Berger D, Dutia M, Powell D, Wissner A, De Morin F, Raifeld Y, Weber J, Boschelli F. Substituted 4-anilino-7-phenyl-3-quinolinecarbonitriles as Src kinase inhibitors. Bioorg Med Chem Lett (2003); 12: 2989–2992.
- Boschelli DH, Wu B, Ye F, Wang Y, Golas JM, Boschelli F. Synthesis and Src kinase inhibitory activity of a series of 4-[(2,4-dichloro-5-methoxyphenyl)amino]-7-furyl-3-quinolinecarbonitriles. J Med Chem (2006); 49: 7868–7876.
- Tetko IV, Gasteiger J, Todeschini R, Mauri A, Livingstone D, Ertl P, Palyulin VA, Radchenko EV, Zefirov NS, Makarenko AS, Tanchuk VY, Prokopenko VV. Virtual computational chemistry laboratory - design and description. J Comput Aid Mol Des (2005); 19: 453–63.
- Hubert M, Rousseeuw PJ, Verboven S. A fast method for robust principal components with applications to chemometrics. Chemom Intell Lab Syst (2002); 75: 101–111.
- Hubert M, Engelen S. Robust PCA and classification in biosciences. Bioinformatics (2004); 20: 1728–1736.
- Jackson DA, Chen Y. Robust principal component analysis and outlier detection with ecological data. Environmetrics (2004); 15: 129–139.
- Hubert M, Rousseeuw PJ, Vanden Branden K. ROBPCA: a new approach to robust principal component analysis. Technometrics (2005); 47:64–79.
- Verboven S, Hubert M. LIBRA: a MATLAB library for robust analysis. Chemom Intell Lab Syst (2005); 75: 127–136.
- Sharma BK, Sharma SK, Singh P, Sharma S. Quantitative structure-activity relationship study of novel, potent, orally active, selective VEGFR-2 and PDGFR alpha tyrosine kinase inhibitors: Derivatives of N-Phenly-N ‘-{4-(4-quinolyloxy)phenyl)urea as antitumor agents. J Enz Inhib Med Chem (2008); 23: 168–173.
- Mercader AG, Duchowicz PR, Fernández FM, Castro EA. Modified and enhanced replacement method for the selection of molecular descriptors in QSAR and QSPR theories. Chemom Intell Lab Syst (2008); 92: 138–144.
- Mercader AG, Duchowicz PR, Fernández FM, Castro EA, Bennardi DO, Autino J C, Romanelli GP. QSAR prediction of inhibition of aldose reductase for flavonoids. Bioorg Med Chem (2008); 16: 7470–7476.
- Cortes C, Vapnik V. Support- vector networks. Mach Learn (1995); 20: 273–297.
- Chang CC, Lin CJ. LIBSVM, a library for support vector machines. <http://www.csie.ntu.edu.tw/∼cjlin/libsvm >
- Efron B. Estimating the error rate of a prediction rule: improvement on cross-validation. J Am Stat Assoc (1983); 78: 316–331.
- Osten DW. Selection of optimal regression models via cross-validation. J Chemom (1988); 2: 39–48.
- Wold S, Eriksson L. In: van de Waterbeemd H, editor. Chemometrics Methods in Molecular Design. Wiley-VCH: Weinheim. (1995); 309–318.
- Tropsha A, Gramatica P, Gombar VK. The importance of being earnest: validation is the absolute essential for successful application and interpretation of QSPR models. QSAR Comb Sci (2003); 22: 69–77.
- Golbraikh A, Tropsha A. Beware of q2!. J Mol Graph Model (2002); 20: 269–276.
- Todeschini R, Consonni V. Handbook of Molecular Descriptors. Wiley-VCH: Weinheim. (2000).
- Fernandez M, Caballero J, Helguera AM, Castro EA, Gonzalez MN. Quantitative structure–activity relationship to predict differential inhibition of aldose reductase by flavonoid compounds. Bioorg Med Chem (2005); 13: 3269–3277.
- Schuur J, Selzer P, Gasteiger J. The coding of three-dimensional structure of molecules by molecular transforms and its application to structure-spectra correlations and studies of biological activity. J Chem Inf Model (1996); 36: 334–344.
- Gonzalez MP, Teran C, Teijeira M, Helguera AM. Radial distribution function descriptors: an alternative for predicting A2A adenosine receptors agonists. Eur J Med Chem (2006); 41: 56–62.