ABSTRACT
Introduction
Saliva has gained increasing attention in the quest for disease biomarkers. Because it is a biological fluid that can be collected is an easy, painless, and safe way, it has been increasingly studied for the identification of oral cancer biomarkers. This is particularly important because oral cancer is often diagnosed at late stages with a poor prognosis.
Areas covered
The review addresses the evolution of the experimental approaches used in salivary proteomics studies of oral cancer over the years and outlines advantages and pitfalls related to each one. In addition, examines the current landscape of oral cancer biomarker discovery and translation focusing on salivary proteomic studies. This discussion is based on an extensive literature search (PubMed, Scopus and Google Scholar).
Expert opinion
The introduction of mass spectrometry has revolutionized the study of salivary proteomics. In the future, the focus will be on refining existing methods and introducing powerful experimental techniques such as mass spectrometry with selected reaction monitoring, which, despite their effectiveness, are still underutilized due to their high cost. In addition, conducting studies with larger cohorts and establishing standardized protocols for salivary proteomics are key challenges that need to be addressed in the coming years.
1. Introduction to saliva as a liquid biopsy source
Oral cancer is a subtype of head and neck cancer that starts in the squamous cells of the inner mucosa, being one of the most common malignant cancers worldwide. When diagnosed in advanced stages, oral cancer has a poor prognosis and is a very mutilating type of cancer [Citation1]. The main risk factors associated with the development of oral cancer are age, male gender, alcohol consumption and smoking. Due to the frequent late-stage diagnosis of oral cancer, there is a crucial need to identify biomarkers enabling earlier detection, allowing personalized prognosis prediction, and anticipating patient’s response to treatment [Citation2].
As powerful analytical techniques have evolved, saliva has gained increasingly recognition as a valuable source of liquid biopsy for identification of oral cancer biomarkers. This fluid is mainly produced by three major salivary glands – parotid, submandibular and sublingual glands – alongside minor salivary glands. A healthy individual typically produces around 500–2500 mL of saliva per day, with a composition primarily comprising 97 to 99% of water and a small percentage of lipids, proteins, and inorganic substances [Citation3]. Saliva mainly contains four groups of secretory proteins: proline-rich proteins, statherins, histatins and cystatins. Saliva collection is a noninvasive and safe procedure, enabling the collection of multiple samples with minimal infection risk. In the context of oral cancer, saliva has the advantage of being in the proximity of tumor cells that may be reflected in its molecular composition [Citation4,Citation5]. However, the salivary molecular profile is very susceptible to several factors such as circadian rhythm, salivary flow rate, type of saliva, genetic polymorphisms, clinical and epidemiological characteristics of the patient [Citation6–9]. After collection of this fluid, hydrolases are activated, increasing the molecular complexity of this body fluid. For instance, proteases degrade the proteins into peptides which makes the analysis of the salivary peptidome extremely important for the discovery of biomarkers since peptides are more than just protein degradation products. Integrating the biological and molecular functions of salivary peptides and proteins is pivotal for gaining a deeper understanding of the disease mechanisms underlying the development and progression of oral cancer [Citation10]. However, the lack of standardized protocols for sample collection, processing and proteome characterization and validation has hampered the effective translation of salivary biomarkers to the clinical setting [Citation5,Citation11,Citation12]. This review aims to critically analyze the main proteomics approaches employed in the identification of salivary biomarkers for oral cancer, highlighting their advantages and limitations.
To gain a comprehensive understanding of the knowledge surrounding salivary proteomics in the context of oral cancer, a bibliometric analysis was conducted using VosViewer. For this, the search formula [saliva and protein and (oral cancer) or oscc or (oral squamous cell carcinoma)] was used in Scopus, and 822 articles were obtained. After excluding literature reviews and articles in languages other than English, 650 articles were included in the bibliometric analysis being most of them published from 2010. Co-occurrence analysis was performed and a minimum of 2 citations for each keyword of interest was established as a filter. Through manual curation, from 2450 keywords the most relevant were selected resulting in the networks presented in Supplementary Figure S1A and Supplementary Figure S1B. It is possible to observe that when studies using mass spectrometry began, there was a significant increase in salivary proteins identified as oral cancer biomarkers. The first mass spectrometer was developed by J.J. Thomson in 1912 (Supplementary Figure S1C). However, due to the high costs associated with the use of this technique, mass use of this technique has only occurred in recent decades. This evolution has sparked a transformative impact on proteomics, particularly in the analysis of proteins in saliva samples, where analytes are present at low concentrations. The subsequent sections provide an overview of the key advancements in discovering oral cancer biomarkers through salivary proteomics, emphasizing both the challenges encountered and the notable achievements within this research domain.
2. Advancements in the discovery of salivary biomarkers
2.1. Workflow for biomarkers discovery and clinical implementation
Biomarkers are defined as characteristics that can be indicators of both physiological and pathogenic processes or can be used to assess the biological response to a particular exposure or intervention. Ideally, in the case of salivary biomarkers, they should be sensitive and specific and objectively quantifiable in saliva samples. Quantification must be reliable and reproducible [Citation13]. To enhance the translation of salivary biomarkers into clinical practice, there are some steps that should be taken in a systematic way. The main steps for identifying and developing salivary biomarkers are shown in . The first step is biomarker discovery. Once the biomarkers of interest have been selected, proof of concept is required to optimize the conditions relating to the identification and quantification of the biomarker of interest to ensure that the results are reliable and reproducible. The biomarker is then validated in a group of selected patients. If the results are sensitive, specific, reliable, and robust, there is potential for them to be translated into clinical practice [Citation13–15].
2.2. Optimizing saliva sample handling and standardization strategies
Standardizing the collection, processing, and storage of saliva samples is critical to ensure reliable and consistent results in proteomics applications. Before starting the collection, it is important to establish guidelines regarding the collection method, type of saliva, fasting duration, and whether to rinse the oral cavity immediately before collection. There are several methods of saliva collection, namely for unstimulated saliva, stimulated whole saliva and gland-specific saliva. Among these, the unstimulated saliva is generally considered as the most suitable for salivary proteomics studies aimed at identifying oral cancer biomarkers. In this case, saliva is collected from patients in a resting state using methods such as passive drooling, spitting/suction or absorbent materials. These methods are noninvasive, easy to implement and enable the collection of large volumes of samples. Several devices have been developed to support saliva collection (). Passive drooling involves minimal oral movement, minimizing the stimulation of salivary secretion and maintaining unaffected salivary rate. This method of saliva collection was validated by Khurshid et al. [Citation16]. In the spitting/suction method, the patient spits the saliva into a collection device. In the absorbent method, an absorbent material is placed on the floor of the mouth. The results obtained with these two methods may be influenced by salivary sampling and in the case of the absorbent method, biomarker absorption to the absorbent material may occur [Citation14,Citation15]. The collection of stimulated whole saliva involves stimulating salivary secretion prior to its collection. This stimulation can be done through masticatory or gustatory stimuli (chewing gum, wax blocks, cotton swabs, citric acid). The choice of the stimulant material may impact the composition of salivary proteins identified in oral cancer patients, leading to significant variations in study outcomes [Citation14]. When focusing on studying salivary proteins specific to salivary glands (parotid, submandibular/sublingual, or other minor glands) some methods are available. For investigating proteins secreted by the parotid gland, the Lashley cup or cannulation methods can be used [Citation13–15]. Lashley cup method is noninvasive and involves attaching a device to the mucosa of the inner cheek using a vacuum, allowing saliva to be collected into a tube [Citation17,Citation18]. On the other hand, the cannulation method requires the placement of a tube at the level of Stensen’s duct for selective saliva collection; however, it is an invasive approach. For submandibular and sublingual saliva collection, customized collectors or the suction method can be employed [Citation14]. In the case of the minor glands, the available methods include customized collectors, the suction method, and filter paper. Customized collectors allow selective collection but often require specialized personnel to collect the saliva samples. The suction method is noninvasive but may result in higher variability among the collected samples. Filter paper, while noninvasive, typically yields smaller sample volumes, and the filter paper itself can influence the results [Citation14].
Figure 2. Main characteristics of saliva as biofluid and types of devices for saliva collection. The image was created with the BioRender.
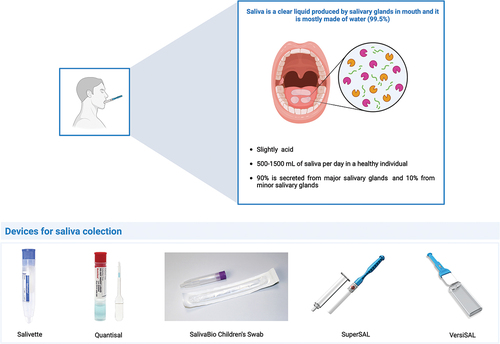
In addition to the collection method, there are other variables that should be considered when collecting saliva. Age, gender, circadian rhythm, recent food intake or smoking, hydration level, and oral cavity condition are some of the factors that may influence the composition of the saliva [Citation6–9]. Before collection, the patient should have restricted food intake and smoking for 30 to 60 min and should have rinsed the oral cavity with water for about 60 seconds. For saliva collection there are several devices, such as Salivette, Saliva Collection Device (SCS), Orapette, SuperSAL and VersiSAL [Citation9].
After saliva collection, there is another set of variables that need to be considered such as storage temperature, proteolysis, and radiation. In an attempt to create a protocol for a standardized handling of saliva samples, Chevalier et al. compared the stability of salivary proteins at different conditions using one and two-dimensional electrophoresis approaches. It was possible to verify that saliva samples should preferably be collected in the morning, 2 h after eating and following a rinse of the oral cavity with water [Citation19]. Before being stored, they should be placed in ice with protease inhibitor, centrifuged and the supernatant stored at − 80°C [Citation18,Citation19]. This protocol ensures the preservation of sample integrity. Salivary proteins have a very limited stability at room temperature, about 1 h [Citation19]. Therefore, it is important to place the samples in a container with ice immediately upon collection. The addition of protease inhibitors is questionable, since there are already collection tubes that come with a solution that allows stabilization of the proteins at the time of collection [Citation20–23]. While the addition of protease inhibitors may inhibit protein degradation, they may introduce complexity into proteomics analysis by interacting with proteins other than proteases. For long-term storage, typically up to approximately 5 years, saliva samples can be safely maintained at − 80°C [Citation24]. This freezing temperature effectively inhibits the degradation of the sample contents, allowing for future analyzes. Another important issue of processing saliva samples is centrifugation, which should ideally be done after sample collection. As an alternative, filtration can be performed but tends to be more time consuming and may result in some protein loss through the filters [Citation25].
2.3. Methods for protein extraction and separation
There are several methods for protein extraction and separation. The first step consists of lysing and solubilizing the saliva samples. This step can be done using either detergents [sodium dodecyl sulfate (SDS) or sodium deoxycholate (SDC)] or chaotropic agents [urea or guanidine hydrochloride (GndHCl)]. Subsequently, the saliva samples can be processed using different methods, namely, in-solution digestion (ISD), filter-aided sample preparation (FASP), solid-phase-enhanced sample preparation (SP3) and protein aggregation capture (PAC). Depending on the method and type of proteomics analysis, proteins may or may not be digested. The most used enzyme for digesting proteins presents in saliva samples is trypsin [Citation26].
Protein separation generally relies on electrophoresis and/or chromatography-based approaches. In terms of electrophoresis, one-dimensional SDS-polyacrylamide gel electrophoresis (1-DE), two-dimensional SDS-polyacrylamide gel electrophoresis (2-DE), two-dimensional difference gel electrophoresis (2D-DIGE) may be used. Briefly, 1-DE allows the separation of proteins based on their molecular weight. Proteins migrate along the polyacrylamide gel in response to the electrical field according to their molecular weight and, after gel staining, bands can be observed. The pore size depends on the concentration of acrylamide and bisacrylamide. In the proteome characterization of biological fluids such as saliva containing hundreds of proteins, this is not the best approach for the separations of proteins present in a complex mixture [Citation27,Citation28]. 2-DE was developed by O’Farrell and was one of the major advances in proteomics studies. This electrophoretic approach comprises 2 steps for the separation of proteins present in complex mixtures. First, proteins are separated according to their isoelectric point and then according to their molecular weight. Considering that hardly two proteins have the same isoelectric point and molecular weight, 2-DE allowed to overcome the problem of low resolution observed with 1-DE, being possible to identify a high number of proteins even if they are present in a small amount of sample. The proteins that are best identified using 2-DE are those with a molecular weight between 20 and 220 kDa and an isoelectric point between 3 and 9. It is a more expensive and poorly reproducible approach, but robust and high resolution [Citation28]. 2D-DIGE is a variation of 2-DE developed by Minden and colleagues that consists of labeling proteins with fluorescent cyanine probes that allow the identification and quantification of proteins without affecting molecular weight and isoelectric point. This method solves the gel-to-gel variations problem enabling the use of multiple samples in single gels. The sensitivity is better in relation to the other types of electrophoresis due to use of fluorescent dye. However, it is time consuming, and it is necessary that the operator has experience with this technique to obtain good results. It is not the best technique for separating proteins with very low or very high isoelectric points [Citation29]. Thus, one-dimensional electrophoresis methods are suitable for protein separation in simple samples, but when it comes to separating and identifying proteins present in a complex mixture, two-dimensional approaches should be preferred.
As an alternative or complement to 2D-PAGE separation, high-performance liquid chromatography (HPLC) is an excellent option due to the reproducibility of results and the possibility of being coupled with mass spectrometry (MS). There are several types of liquid chromatography, namely reversed-phase, ion-exchange, size-exclusion, and affinity chromatography [Citation30]. Reversed-phase chromatography (RPLC) is the most widely used type of chromatography due to its compatibility with the various MS methods. RPLC allows the separation of compounds with hydrophobic properties. While in standard liquid chromatography, the stationary phase is nonpolar and the mobile phase is polar, in RPLC it is the other way around. RPLC has the advantage of being less toxic, more economical and with less sample volume it can perform as well as aqueous normal-phase chromatography. When samples contain very large amounts of proteins and peptides in the hundreds of thousands, one-dimensional approaches are often not enough due to insufficient peak capacity. In this case, multi-dimensional approaches (ion-exchange chromatography-RPLC, affinity chromatography-RPLC, size-exclusion-RPLC) are often necessary [Citation30]. An overview of proteomics workflow is displayed in , highlighting the most frequently employed experimental approaches.
2.4. Methods for identification of proteins
MS has been increasingly used for large-scale protein characterization as it is a high-throughput technique. The principle is the formation of ions in the gas phase that are characterized in terms of mass-to-charge ratio (m/z) and relative abundance. A beam of high-energy electrons hits the molecules, fragmenting them. From the analysis of the m/z of the fragments and their relative abundance, it is possible to obtain information about the amino acid sequence and, consequently, on proteins present in the saliva samples. For salivary biomarker discovery, there are two strategies: top-down and bottom-up. In the top-down approach, intact proteins are analyzed, while in the bottom-up approach, peptides that result from the digestion of proteins by a specific protease such as trypsin are analyzed. In the case of the bottom-up approach, the study is usually complemented by HPLC, as the proteins when digested generate a very complex mixture of peptides. These peptides are separated according to their degree of hydrophobicity, with the most hydrophilic peptides being eluted the fastest.
After the sample peptides have passed the chromatographic column and separated based on hydrophobicity, they undergo ionization, followed by separation according to their m/z ratio and subsequent detection. The most important ionization methods include electrospray ionization (ESI), matrix-assisted laser desorption/ionization (MALDI), and surface-enhanced laser desorption/ionization (SELDI), although the latter two are less commonly employed in salivary proteomics. After ionization, the generated ions pass through a mass analyzer such as Time-of-flight (TOF), Orbitrap, Ion Trap, or Quadrupole to accurately determine the m/z ratio and the intensity of the signals under vacuum-induced electric fields.
ESI is a technique in which ions are generated using an electrospray when a high voltage is applied to a liquid to create an aerosol. It is particularly suitable for analyzing biomolecules such as peptides and proteins, making it a cornerstone of proteomics. ESI is characterized by its compatibility with liquid chromatography and enables seamless integration into LC-MS/MS workflows. The advantage of this method is that it can handle a wide range of molecular weights and enables a gentle ionization process that preserves non-covalent interactions. However, the sensitivity to sample preparation and matrix effects can be seen as a limitation. The application of ESI for the identification of salivary biomarkers is facilitated by its versatility in analyzing complex mixtures and provides insights into the proteomic profile of saliva samples [Citation31]. In MALDI-TOF/MS the analyte molecules are embedded in a matrix that can absorb ultraviolet light. When this matrix-analyte mixture is irradiated with a laser beam, the matrix absorbs the energy and supports the desorption and ionization of the analyte molecules into the gas phase. The ions are then separated based on their m/z value in the TOF mass analyzer. This technique is well suitable for the analysis of proteins with high molecular weight, typically greater than 100 kDa. MALDI-TOF-MS is valued for its sensitivity, rapid analysis, cost-effectiveness and minimal sample pre-treatment or sample volume is required. However, there are some limitations to consider. One of these limitations is the limited mass range of the analyzer, which may not be able to accommodate extremely high molecular weights. In addition, MALDI-TOF-MS can be sensitive to contaminants, which can affect the reproducibility of results. Finally, the sample preparation protocol can vary significantly depending on the specific characteristics and properties of the analyte, which may require different approaches for optimal results [Citation32]. SRM is a quantification method used in MS that focuses on the accurate measurement of specific proteins in complex samples. SRM involves the precise selection of a unique ion (MS1) that corresponds to the target protein and is identified by its unique m/z. This selected ion is then subjected to fragmentation, and the resulting fragment ions are further selected (MS2) according to their unique m/z values. This targeted approach enables the detailed analysis and quantification of proteins by tracking specific ion transitions, facilitating a highly selective and sensitive assessment of protein abundance in biological samples [Citation33]. The application of SRM-MS to the analysis of saliva from patients with lymph node metastases has shown significant down-regulation of proteins such as CSTB, LTA4H, PGK1, COL6A1, ITGAV and NDRG1, highlighting the utility of this approach in uncovering critical molecular insights into disease progression and metastatic potential [Citation34].
Data-dependent acquisition (DDA) and data-independent acquisition (DIA) are the main discovery platforms, being DDA the most used. These platforms differ primarily in their approach to isolating precursor ions for fragmentation and subsequent acquisition of MS2 spectra. DDA essentially involves the selection, accumulation and fragmentation of precursor ions based on the analysis of data or signals from an initial MS1 surveillance scan in real time. DIA, on the other hand, systematically works through a predetermined range of precursor ion isolation windows. Within these windows, all precursor ions are fragmented simultaneously, eliminating the need to select precursor ions in real time [Citation35]. DIA tends to generate more complex MS2 spectra and multiplex chromatograms, which can lead to a reduction in selectivity for individual precursor ions. This complexity necessitates the use of advanced computational tools specifically designed for the interpretation of DIA data [Citation36]. Over the past twenty years, DIA methods have evolved significantly, with numerous acquisition strategies developed and applied to various MS instrument platforms. This evolution has significantly pushed the boundaries of what can be achieved in terms of sensitivity, specificity, reproducibility and analytical throughput using DIA techniques.
DDA and DIA can both be combined with isotope labeling for protein quantification. The methods for quantification of proteins using MS comprise label-free and label-based MS quantification. The results are cross-referenced with databases using software such as Sequest, Omssa and Andromeda for peptide identification [Citation37–39].
The use of label-free MS quantification comprises two different types of quantification: spectral counting or spectrometric precursor signal intensity measure of the protein expression. In the first case, the number of spectra obtained for each peptide in the different saliva samples is quantified and the results of all peptides are integrated. Precursor signal intensity is the most used and consists of extracting signals from peptides at the MS1 level by applying high-resolution scan. The main techniques used with this type of quantification are liquid chromatography-tandem mass spectrometry (LC-MS/MS), matrix-assisted laser desorption⁄ionization time-of-flight mass spectrometry (MALDI-TOF-MS) and selected reaction monitoring (SRM).
The application of targeted quantification techniques such as Multiple Reaction Monitoring (MRM), Consecutive Reaction Monitoring (CRM) and Parallel Reaction Monitoring (PRM) has revolutionized the field of proteomics by providing highly reproducible, sensitive and specific methods for the quantification of proteins [Citation40,Citation41]. MRM is particularly characterized by its precision in measuring targeted peptides in complex biological samples, making it indispensable for the validation of potential biomarkers in saliva. In studies using MRM, key proteins associated with oral cancer have been successfully identified and quantified, demonstrating the potential of this technique for clinical diagnostics [Citation42,Citation43]. For an introduction to targeted quantification techniques and their impact on biomarker discovery the previous paper [Citation35] provides a comprehensive overview that is accessible to non-specialists.
The use of stable isotope labeling techniques, specifically Isobaric Tags for Relative and Absolute Quantitation (iTRAQ), Tandem Mass Tags (TMT), Isotope-Coded Affinity Tags (ICAT), and Stable Isotope Labeling by Amino acids in Cell Culture (SILAC), has greatly enhanced the ability to simultaneously quantify protein expression in multiple samples [Citation44]. For example, iTRAQ has helped to reveal differential protein expression in saliva samples from oral cancer patients, providing new insights into the proteomic landscape of the disease [Citation45,Citation46]. Similarly, TMT labeling has expanded the scope of quantifiable proteins in saliva, enabling the identification of more than 1400 proteins in certain studies, which reflects its sensitivity and throughput [Citation47]. The evolution of TMT from a 2-plex to an 18-plex system, including the latest TMTpro 16-plex system enhanced with NeuCode isotopes [Citation48], illustrates the technological advances in improving analytical throughput and efficiency. These developments have paved the way for comprehensive proteomic profiling of saliva, revealing potential biomarkers for oral cancer and other diseases in unprecedented depth and specificity [Citation49]. Such advances underscore the critical role of MS in advancing diagnostic and therapeutic research and promise a future in which saliva-based diagnostics could become a routine part of clinical practice.
2.5. Methods for salivary protein verification and validation
After the identification of salivary biomarkers, a validation step is necessary so that these biomarkers can be translated to clinical practice. Enzyme-linked immunosorbent assay (ELISA) and western blotting have been traditionally used for validation and verification of data retrieved from MS-based proteomics [Citation35,Citation50]. There are several types of ELISA tests, namely, direct, indirect, sandwich, and competitive ELISAs [Citation51]. Overall, ELISA is a very sensitive technique with good reproducibility and specificity, when using well-characterized and validated antibodies. However, it requires a considerable sample volume and does not allow to control antibody specificity [Citation51]. Western blot enables the determination of the molecular weight of the target protein, confirming the identity of a biomarker by detecting its size and ensuring, to a certain extent, the specificity of antibody detection [Citation52]. However, western blot is more time-consuming and inherently semi-quantitative, though it requires a relatively smaller sample volume [Citation53,Citation54]. Nevertheless, compared to MS, which typically offers unambiguous protein identification via unique peptides at a given false discovery rate, techniques like ELISA, western blot, Olink, or SomaScan (mentioned below) rely on the specificity of affinity reagents, which may be influenced, for example, by posttranslational modifications [Citation55]. This limitation underscores the importance of cautious interpretation when employing affinity-based techniques for protein analysis. Immunohistochemistry is also important to validate in tumor tissue samples the biomarkers found in saliva samples from oral cancer patients. However, in addition to the limitations mentioned for affinity-based techniques, there are salivary biomarkers that can be found in saliva samples from oral cancer patients that do not originate from tumor tissue.
Recent advances in proteomics have significantly novel approaches for biomarker searching and validation. Immunoassays such as ELISA only allow one analyte to be measured at a time. The application of multiplex assays for proteome profiling has made it possible to extract information on multiple analytes present in the same sample in a single analysis. It is a much more efficient method than ELISA as it saves time, costs, and material. There are two types of multiplex assays, namely planar microarray (protein chips) and suspension array (microparticle or bead microarray) [Citation56]. Protein microarray is similar to sandwich immunoassays. That is, several proteins can be processed in the same analysis. There are three types of microarrays: analytical microarrays, functional protein microarrays and reverse phase protein microarrays (RPPA). In analytical microarrays, antibodies are immobilized on a matrix. The protein of interest binds to the primary antibody and then a secondary antibody coupled to a fluorophore binds to the protein, causing light emission. In functional microarrays, proteins are immobilized on the array and biochemical properties of the proteins can be studied. In RPPA, cells are isolated and lysed. The resulting product of cell lysis is immobilized on a matrix and antibodies are added that bind to a given protein of interest with light emission. It is widely used to study post-translational modifications [Citation57,Citation58]. However, specificity may be an issue thus requiring optimization of the sample volume to be used. In the case of bead-based arrays, each bead binds a specific capture antibody and emits a specific fluorescence intensity. In this way, several proteins can be quantified in the same sample using different beads. The capture antibody binds to the protein of interest and then a fluorochrome-conjugated antibody is added to detect the proteins using flow cytometry. The intensity of the fluorescence emission is proportional to the amount of protein present in the sample [Citation56]. shows that most of the studies using multiplex assays for identification of salivary biomarkers evaluate cytokines.
Table 1. Salivary biomarkers for oral cancer and the main techniques used in each study.
Proximity extension assays (PEA) combine the principles of sandwich ELISA with the precision of DNA-based readout methods such as quantitative PCR or next-generation sequencing (NGS). The result is a powerful tool for liquid biopsy detection with a broad dynamic range. PEA uses pairs of antibodies labeled with DNA oligos that hybridize after binding to the target molecule, enabling PCR amplification and precise quantification of proteins. PEA has a wide range of applications, from the identification of prognostic biomarkers in colorectal cancer to the profiling of different cancer types, with high sensitivity and specificity. Despite its power, the highly complex variant of PEA faces challenges in library preparation and NGS, requiring careful validation due to potential bias and variation in large-scale studies [Citation145,Citation146]. With the advance of bioinformatics in recent years, there has been a need to extract as much information as possible from a given sample.
Olink technology harnesses the power of PEA, employing pairs of antibodies tethered to DNA oligonucleotides. These antibody pairs selectively bind to target proteins within a sample. A standout feature of Olink technology is its capacity to concurrently measure numerous proteins in a single sample, facilitating a thorough analysis of protein profiles. This high-throughput capability renders Olink assays exceptionally valuable in biomarker discovery and validation in clinical research contexts using saliva samples [Citation147].
Aptamers, i.e. short strands of DNA, RNA or peptides, fold into unique tertiary structures that can bind to target proteins with high specificity and affinity. The slow off-rate modified aptamers (SOMA) scan assay, a notable application of this technology, uses these aptamers or SOMAmers with photocleavable linkers and fluorescent markers to capture and quantify proteins and determine their abundance in samples [Citation148]. Aptamers offer advantages over antibodies, such as higher affinity, specificity, and easier synthesis, which facilitates scaling up for high-throughput applications. This has enabled the simultaneous profiling of over 7000 proteins [Citation114,Citation149,Citation150]. In clinical diagnostics, aptamer-based assays have shown great promise. For example, stool-based profiling with aptamers has identified characteristic protein patterns for the diagnosis of colorectal cancer. Similarly, in non-small cell lung cancer, aptamer-based studies have identified several protein biomarkers that have led to the development of a clinically useful biomarker panel for early detection.
3. Harmonization of techniques used on salivary proteomics
MS-based approaches have shown a growing efficacy in the analysis of salivary analytes. However, several preanalytical factors, namely the methodology and conditions of saliva collection, as well as the intrinsic quality of the collected biological fluid, influence the results obtained. Thus, the creation of standard operating procedures (SOPs) for each of the steps inherent to conducting salivary proteomics studies are crucial to ensure reliable and consistent results. Furthermore, harmonization of the techniques used will increase reproducibility, reduce technical noise, and overcome the problem of low sample size studies. Another important step is the introduction of quality control steps in proteomics studies. Bourmaud et al. developed a simple internal quality control procedure that has been tested on plasma samples analyzed by LC-MS/MS and can be easily incorporated into proteomics studies with saliva [Citation149]. This procedure consists in the introduction of a mixture of exogenous proteins, followed by the addition of isotopically labeled peptides to the reference samples to assess the performance of the sample handling and MS technique. This procedure provides a necessary system suitability test before starting the actual sputum analysis as well as allows continuous monitoring of the instrument performance and sample preparation allowing more comparable, robust, and reproducible results [Citation145,Citation150]. The main advantage is that in addition to ensuring optimal conditions during the proteomics study, deviations or malfunctions can be corrected in real time. In this way, the creation of SOPs to control preanalytical factors together with the implementation of internal quality control procedures will ensure the generation of reliable and consistent data for advancing the field of salivary proteomics in oral cancer research and beyond. A case in point is the work of Voß et al., who created HarmonizR, a data harmonization tool specifically tailored for tissue analysis from oral cancer patients [Citation146]. In this study, data from several proteomic LC-MS/MS datasets from online repositories were harmonized. The datasets derived from different tissue preservation techniques, LC-MS/MS instrumentation setups and quantification techniques. This work forms the cornerstone for integrating data across various datasets within a specific domain, enabling large-scale data analysis. This type of strategy can be expanded to salivary proteomics studies, offering a fresh perspective on existing datasets with saliva samples from oral cancer patients that would otherwise be challenging to analyzed in an integrated way. Nevertheless, not all laboratories and research groups have the same resources and budget. In this sense, there must always be a balance between costs and performance when deciding on techniques, and this consideration should be incorporated into protocols to ensure standardization across research laboratories, regardless of their individual conditions.
4. Limitations of salivary proteomics: the importance of small and large cohort studies
The development of increasingly effective strategies for the early detection of oral cancer and for predicting prognosis and response to treatment tailored to each patient is only possible if the results of scientific research are generalizable and reliable. Exploratory studies are often initially conducted at an early stage. However, studies with small sample sizes often have several limitations that hinder this goal. The main limitations include low statistical power, overfitting and high variance, bias and confounding variables, less generalizable results, and high influence of outliers. Determining the sample size is one of the essential steps in study design. A small sample size, together with high variability and small effect size, can decrease the statistical power of the study and jeopardize the integrity of the results. It is recommended that the statistical power should be at least 80%. Beyond 80%, the identification of statistically significant differences or associations become more challenging, as the study may be too sensitive and reveal effects that are statistically significant but may not be of practical significance. Such oversensitivity can lead to overfitting, a statistical problem in which a model is so finely tuned to the training data that it cannot be generalized to new, unseen data. This phenomenon can lead to overly optimistic results in initial studies, but non-reproducible results in subsequent studies [Citation151].
In the case of proteomics studies with two experimental groups, the effect size is calculated based on the difference in protein abundances between the two different sample groups. The main issue is that many of the proteomic studies aimed at identifying salivary biomarkers for oral cancer test many proteins, each with its own effect size and variance. However, exploratory studies with small sizes are important because they allow estimating a mean variance value to correctly determine the appropriate sample size for further validation of proteins in larger cohorts. In addition, small salivary proteomic studies are more likely to have biases and confounding variables. For example, sampling bias may occur if the sample is not representative of the population, distorting the results. Confounding variables may not be evenly distributed across groups in small studies, leading to misleading associations. Due to the limited number of participants, results obtained in salivary proteomics studies with small sample sizes may not be representative of the population, being associated with lower external validity and limiting the generalizability of the results to the general population.
Finally, when low sample sizes are used, any abnormal results may disproportionately affect the results leading to erroneous or inconclusive conclusions. Thus, exploratory studies are important to optimize the study design, but must be complemented with large cohort studies so that the proteins of interest can be translated into clinical practice [Citation145,Citation150,Citation152–154]. Large cohort studies allow for more robust, accurate and reproducible results. This type of study represents the cornerstone for the validation of salivary biomarkers for oral cancer and subsequent implementation into clinical practice. Large cohort studies have several advantages in relation to small salivary proteomic studies such as greater statistical power (higher probability of detecting a true effect), better representativeness (more generalizable results to the population), less influence of outliers (more accurate estimates of parameters and relationships), detection of subtle effects that are often missed in low-sample salivary proteomics studies, better treatment of confounding variables (more accurate estimate of the true effect of the variables of interest) and long-term follow-up (monitorization of the progression of oral cancer over time). Thus, despite the higher cost and logistical burden, large cohort studies offer significant advantages by improving the validity, reliability, and generalizability of research findings [Citation152,Citation153]. They are essential for progress in areas such as salivary proteomics for the detection and treatment of oral cancer.
5. Verification of salivary biomarkers through tumor tissue analysis
Immunohistochemistry (IHC) may be used for the verification of biomarkers found in the saliva of oral cancer patients through proteomics with potential origin in HNSCC, through the analysis of tumor tissue samples. IHC involves the specific binding of proteins of interest within HNSCC tissue to antibodies conjugated to an enzyme (peroxidase or alkaline phosphatase) or a fluorophore. The main disadvantages are related to result quantification and susceptibility to human error, as it involves a subjective analysis [Citation155,Citation156]. These studies are extremely important because they allow us to verify whether the proteins identified in the saliva of patients with oral cancer reflect tumor biology. The composition of the salivary proteome varies according to the physiological state of the patients, and many diseases can influence the protein profile in saliva. By comparing salivary biomarkers with tissue IHC findings, valuable information about the factors contributing to HNSCC-induced salivary proteome remodeling can be extracted. This approach may significantly enhance our comprehension of HNSCC pathobiology. In recent years, the branch of pathomics has emerged. Digital pathomics takes advantage of deep learning and machine learning algorithms to extract information from high-resolution whole-slide images of tissue sections that allows the generation of data regarding various phenotypic characteristics of this type of sample. Combining proteomics with pathomics studies constitutes an extremely powerful weapon for translating salivary biomarkers. For example, Bankhead et al. created QuPath which is a digital pathology software that allows the integration of histopathology data with genomics and medical imaging data to predict response to immunotherapy treatments, which has been shown to perform very well on unimodal measures including tumor mutational burden and PD-L1 score [Citation157,Citation158].
6. Validation of biomarkers in patients with potentially malignant lesions
Normando et al. performed a comprehensive meta-analysis showing an association between a set of proteins and the malignant transformation of oral lesions [Citation159]. The inclusion of individuals with potentially malignant oral lesions in oral cancer proteomics studies provides a better understanding of the salivary proteome across various stages of oral cancer development. In addition, this validation step has the potential to uncover biomarkers capable of predicting the risk of malignant transformation of oral lesions.
The process of malignant transformation in the context of oral cancer comprises several phases of cell differentiation. For each stage of disease, there are genetic, epigenetic, environmental and tumor microenvironment factors that facilitate or promote this differentiation and are schematized in .
Figure 4. Temporal evolution of salivary biomarkers and techniques used in its identification. Vosviewer network of most cited salivary biomarkers and techniques used in proteomics for biomarkers discovery in oral cancer (a). Vosviewer overlay showing the temporal distribution of the most cited salivary biomarkers and techniques used in proteomics for biomarkers discovery in oral cancer (b). Chronological discovery of the most relevant techniques used in studies of proteomics using saliva as source of liquid biopsy for discovery of biomarkers of oral cancer (c).
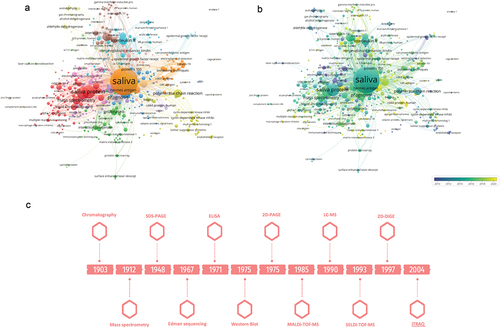
The use of saliva as liquid biopsy and source of potential biomarkers for the diagnosis of oral potentially malignant lesions (OPMD) is also extremely important. Some meta-analyzes justify the potential use of salivary biomarkers in OPMD. Arroyo et al. demonstrated that salivary carcinoembryonic antigen (CEA) and soluble fragment of cytokeratin 19 (CYFRA21) can be useful for the differential diagnosis between OPMD and OSCC, being CYFRA 21 also capable of differentiating OPMD from healthy individuals [Citation160]. Salivary levels of the cytokines IL-8, IL-6, TNF-α and IL-1β are significantly increased in patients with OSCC compared to OPMD. The cytokines IL-6 and TNF-α in turn are found at significantly higher levels in OPMD compared to healthy individuals. This panel of cytokines is useful in the screening of both OSCC and OPMD [Citation161,Citation162]. A meta-analysis performed by Velázquez et al. showed that salivary LDH is useful in the diagnosis of OSCC and OPMD. However, more studies are still needed to define the cut off values for use of this biomarker [Citation163].
7. Salivary protein biomarkers in oral cancer: current status
Several proteins have been identified and validated in recent years as oral cancer biomarkers, with particular interest in cytokines, growth factors, matrix metalloproteinases and acute phase proteins [Citation164,Citation165]. Nonetheless, many of the salivary biomarkers of oral cancer that have been proposed do not originate directly from the tumor. This is an issue that should be addressed in the design of future proteomics studies. However, the fact that these biomarkers have not been identified in OSCC tumor tissue does not mean that they cannot be a good OSCC biomarker. Their role in the pathophysiology of OSCC may not yet be known, and this could be an opportunity to expand our knowledge of OSCC. From the salivary biomarkers proposed for oral cancer, as shown in , the two most studied ones are IL-6 and IL-8. TNF-α, MMP9, cyfra 21.1 and MMP1 have also been extensively studied in recent years. A meta-analysis performed by Benito-Ramal et al. showed that salivary IL-6, IL-8 and TNF-α are suitable to be used in the diagnosis and prognosis of oral cancer [Citation166]. Hema Shree et al. and AlAli et al. concluded that MMP9 and cyfra 21.1 are specific and sensitive biomarkers of oral cancer [Citation167,Citation168]. However, they report the need for studies with larger patient cohorts. On ClinicalTrials.gov there are three registered clinical trials aiming to validate salivary proteins for oral cancer. NCT05049408 aimed to validate the MMP1 protein as a diagnostic biomarker for oral cancer. About 1100 patients were recruited (269 with oral cancer, 518 with oral pre-malignant diseases and 313 healthy controls) from whom saliva samples were collected and analyzed by ELISA for MMP1 identification. In this study, MMP1 showed a sensitivity of 69.5% and specificity of 95% in detecting oral cancer at an earlier stage [Citation60]. In addition, it was shown to be a relevant biomarker in monitoring disease progression and recurrence as well as predicting neck lymph node metastasis. The other two clinical trials have no published results. However, NCT03148665 has already been completed and aimed to validate CD44 in the diagnosis of oral cancer using OncAlert as a collection method. NCT03529604 has no information on its stage but involves the validation of three biomarkers of which two are proteins, SCCA and TROP2. In NCT03529604 trial, 100 patients were recruited and the analysis of samples for protein identification involves the use of techniques such as ELISA and liquid chromatography.
8. Conclusion
The identification of salivary biomarkers in oral cancer is a very promising area that may change the paradigm of this disease. Saliva as liquid biopsy source has many advantages in the identification of oral cancer biomarkers. The collection is noninvasive, easy, allows repetitive sampling and establishes a close relationship with the main structures involved in oral cancer. Several salivary biomarkers have been validated for diagnosis and prognosis of oral cancer. The techniques used in proteomics are increasingly high – throughput. The advent of MS has revolutionized proteomics studies and, today, LC-MS/MS stands as the most widely employed MS variant. It is anticipated that as the field progresses, researchers will move toward increasingly sophisticated techniques that offer improvements in sensitivity, specificity, reproducibility and overall robustness of study results. Such optimization is expected to increase the likelihood that salivary biomarkers will be successfully translated into clinical practice. As these biomarkers are increasingly incorporated into routine diagnostic and prognostic protocols, patients will benefit from personalized and timely interventions. The integration of reliable, noninvasive biomarker-based tests into the clinical workflow may ultimately lead to better outcomes for patients with oral cancer and represent a paradigm shift in the management of this difficult disease.
9. Expert opinion
The potential of saliva as a liquid biopsy source for identifying biomarkers of oral cancer is undisputed. It has ushered in a new era in oral cancer diagnostics by providing a noninvasive, easily accessible, and patient-friendly medium for disease detection and monitoring. With the introduction and refinement of various proteomic techniques, the proteomics field has made remarkable progress over the years. Despite these promising advances, the field is not yet mature, as we must fully realize the potential of saliva in oral cancer diagnostics. Technological advances, particularly in MS, have greatly improved the landscape of salivary proteomics. Selected reaction monitoring/multiple reaction monitoring (SRM/MRM) MS techniques have been proven to be valuable tools in this field. Compared to the DIA- and DDA-MS approaches, MRM-MS has the highest quantitative accuracy allowing relative protein quantification. The main disadvantages are that it only detects and quantifies up to 100 proteins per analysis and that it requires the synthesis of ‘heavy peptides’ as internal quantitative standards. MRM-MS is not used as a protein discovery strategy, but very promising studies are beginning to emerge that combine DIA-MS with MRM for biomarker discovery and validation. In this way, MRM-MS can also be incorporated into proteomic discovery studies. However, it is imperative to overcome the high costs and accessibility issues of MRM-MS technology to make it available to a broader range of laboratories. Reducing costs and increasing the efficiency of MS platforms, standardizing the experimental approaches used in proteomics studies, creating biobanks, and establishing collaborative projects, will lead to an expansion of clinical cohort sizes. This will make it possible to overcome issues relating to the statistical power of proteomics studies enabling translation of the protein candidates to clinical practice. Multi-Omics approaches are extremely attractive because they can integrate several levels of information, namely genomics, proteomics, epigenomics, transcriptomics and post-translational modifications. Nevertheless, large amounts of data imply the need for superior computing power and better data analysis software. These advancements will bring us one step closer to translating research findings into meaningful clinical applications that can significantly improve oral cancer diagnosis and patient outcomes.
Over the next five years, the field of salivary proteomics in oral cancer diagnostics is poised for a transformative evolution that will revolutionize the way we diagnose and track this disease. Although sophisticated techniques such as MS with SRM/MRM have shown immense potential, their high cost has been a barrier to widespread use. As these methods become more widely used and more laboratories adopt them, costs are expected to decrease significantly due to economies of scale. This reduction in cost will not only democratize access to cutting-edge technologies, but also pave the way for their wider use in salivary proteomics research. Targeted approaches, such as the ones based on aptamers, are expected to expand the diagnostic arsenal available to medical professionals. At the same time, the proteomics research landscape will be redefined by the emergence of international consortia dedicated to standardizing research protocols. These collaborative networks will focus on developing universally accepted best practices spanning every phase of a proteomics study – from sample collection and processing to data analysis and reporting. This harmonization of methods across laboratories will not only eliminate inconsistencies resulting from different techniques but will also improve the reproducibility and comparability of research results.
In parallel, we expect to see an increase in collaborative studies with large, diverse patient cohorts. The consolidation of resources and expertise will enable research initiatives on an unprecedented scale, providing more robust data and improving the statistical power and validity of results. Most importantly, this collaborative approach will facilitate the comprehensive validation of potential salivary biomarkers in diverse populations and clinical contexts – an indispensable step for their subsequent clinical application.
Finally, artificial intelligence (AI) models incorporating machine learning (ML) and deep learning (DL) strategies present promising approaches for salivary protein analysis, particularly with large datasets from MS-based proteomics. Capable of handling unstructured data and autonomously extracting high-quality features, these AI models enhance the accuracy of data analysis, thereby advancing biomarker discovery in oral cancer research. The ongoing evolution of technology suggests that the seamless integration of salivary biomarker discovery and AI-driven analytics holds significant potential to revolutionize oral cancer management, paving the way for targeted and individualized care. Overall, we are entering an exceptionally vibrant and dynamic era of research and innovation in salivary proteomics for the diagnosis of oral cancer. With greater financial access to cutting-edge technologies, the development of standardized methods, and an increasingly collaborative research ecosystem, breakthrough discoveries are within reach. However, it is important to recognize that this optimistic outlook requires a sustained, collaborative effort by all stakeholders, including researchers, clinicians, and policymakers. Only by confronting the remaining obstacles and seizing the opportunities that present themselves can we hope to fully realize this transformative vision for the future of oral cancer diagnosis and treatment.
Article highlights
The establishment of harmonized protocols for consistent research results.
Saliva is becoming increasingly important in medical research due to its simple, painless, and safe collection method.
Saliva is a source of biomarkers for oral cavity cancer, a malignancy that has a high mortality rate when detected late.
Early detection of oral cavity cancer can significantly improve prognosis and contribute to personalized treatment.
A thorough overview of the improvements needed in study design for such research is provided.
Declaration of interest
The authors have no relevant affiliations or financial involvement with any organization or entity with a financial interest in or financial conflict with the subject matter or materials discussed in the manuscript. This includes employment, consultancies, honoraria, stock ownership or options, expert testimony, grants or patents received or pending, or royalties.
Reviewer disclosures
Peer reviewers on this manuscript have no relevant financial or other relationships to disclose.
Declaration of competing interest
The authors declare no conflicts of interest. All authors have read the journal’s authorship agreement and policy on disclosure of potential conflicts of interest.
Supplemental Material
Download TIFF Image (379.4 KB)Supplementary material
Supplemental data for this article can be accessed online at https://doi.org/10.1080/14789450.2024.2343585
Additional information
Funding
References
- Julian RS, Woo BM, Rabey EC. Oral Cavity and Oropharyngeal Cancer: Etiology, Diagnosis and Staging. J California Dental Association. 2021;49(3):163–170. doi: 10.1080/19424396.2021.12222685
- Rivera C. Essentials of oral cancer. Int J Clin Exp Pathol. 2015;8(9):11884–11894.
- Michalke B, Rossbach B, Göen T, et al. Saliva as a matrix for human biomonitoring in occupational and environmental medicine. Int Arch Occup Environ Health. 2015;88(1):1–44. doi: 10.1007/s00420-014-0938-5
- Rao PV, Reddy AP, Lu X, et al. Proteomic identification of salivary biomarkers of type-2 diabetes. J Proteome Res. 2009;8(1):239–245. doi: 10.1021/pr8003776
- Pfaffe T, Cooper-White J, Beyerlein P, et al. Diagnostic potential of saliva: current state and future applications. Clin Chem. 2011;57(5):675–687. doi: 10.1373/clinchem.2010.153767
- Scarano E, Fiorita A, Picciotti PM, et al. Proteomics of saliva: personal experience. Acta Otorhinolaryngol Ital. 2010;30(3):125–130.
- Castagnola M, Picciotti PM, Messana I, et al. Potential applications of human saliva as diagnostic fluid. Acta Otorhinolaryngol Ital. 2011;31(6):347–357.
- Laine M, Pienihakkinen K, Leimola-Virtanen R. The effect of repeated sampling on paraffin-stimulated salivary flow rates in menopausal women. Arch Oral Biol. 1999;44(1):93–95. doi: 10.1016/S0003-9969(98)00097-1
- Topkas E, Keith P, Dimeski G, et al. Evaluation of saliva collection devices for the analysis of proteins. Clin Chim Acta. 2012;413(13–14):1066–1070. doi: 10.1016/j.cca.2012.02.020
- Amado F, Lobo MJ, Domingues P, et al. Salivary peptidomics. Expert Rev Proteomics. 2010;7(5):709–721. doi: 10.1586/epr.10.48
- Granger DA, Kivlighan KT, Fortunato C, et al. Integration of salivary biomarkers into developmental and behaviorally-oriented research: problems and solutions for collecting specimens. Physiol Behav. 2007;92(4):583–590. doi: 10.1016/j.physbeh.2007.05.004
- Ferguson DB. Current diagnostic uses of saliva. J Dent Res. 1987;66(2):420–424. doi: 10.1177/00220345870660020601
- Khurshid Z, Zohaib S, Najeeb S, et al. Human saliva collection devices for proteomics: an update. Int J Mol Sci. 2016;17(6):846. doi: 10.3390/ijms17060846
- Bellagambi FG, Lomonaco T, Salvo P, et al. Saliva sampling: methods and devices. An overview. Trends Analyt Chem. 2020;124:115781. doi: 10.1016/j.trac.2019.115781
- Baum BJ. Evaluation of stimulated parotid saliva flow rate in different age groups. J Dent Res. 1981;60(7):1292–1296. doi: 10.1177/00220345810600070101
- Khurshid Z, Moin SF, Khan RS, et al. Human salivary protein extraction from RNAPro.SAL, Pure.SAL, and passive drooling method. Eur J Dent. 2017;11(3):385–389. doi: 10.4103/ejd.ejd_183_17
- Heinzerling CI, Stieger M, Bult JH, et al. Individually modified saliva delivery changes the perceived intensity of saltiness and sourness. Chem Percept. 2011;4(4):145–153. doi: 10.1007/s12078-011-9099-z
- Henson BS, Wong DT. Collection, storage, and processing of saliva samples for downstream molecular applications. Methods Mol Biol. 2010;666:21–30.
- Chevalier F, Hirtz C, Chay S, et al. Proteomic studies of saliva: a proposal for a standardized handling of clinical samples. Clin Proteomics. 2007;3(1–4):13–21. doi: 10.1007/s12014-007-9000-x
- Schipper RG, Silletti E, Vingerhoeds MH. Saliva as research material: biochemical, physicochemical and practical aspects. Arch Oral Biol. 2007;52(12):1114–1135. doi: 10.1016/j.archoralbio.2007.06.009
- Chiang SH, Thomas GA, Liao W, et al. Rnapro*sal: a device for rapid and standardized collection of saliva RNA and proteins. Biotechniques. 2015;58(2):69–76. doi: 10.2144/000114254
- Xiao H, Wong DT. Method development for proteome stabilization in human saliva. Anal Chim Acta. 2012;722:63–69. doi: 10.1016/j.aca.2012.02.017
- Esser D, Alvarez-Llamas G, de Vries MP, et al. Sample stability and protein composition of saliva: implications for its use as a diagnostic fluid. Biomark Insights. 2008;3:25–27. doi: 10.4137/BMI.S607
- Janardhanam SB, Zunt SL, Srinivasan M. Quality assessment of saliva bank samples. Biopreserv Biobank. 2012;10(3):282–287. doi: 10.1089/bio.2011.0039
- Kawashima Y, Fukutomi T, Tomonaga T, et al. High-yield peptide-extraction method for the discovery of subnanomolar biomarkers from small serum samples. J Proteome Res. 2010;9(4):1694–1705. doi: 10.1021/pr9008018
- Carrillo-Rodriguez P, Selheim F, Hernandez-Valladares M. Mass spectrometry-based proteomics workflows in cancer research: the relevance of choosing the right steps. Cancers (Basel). 2023;15(2):555. doi: 10.3390/cancers15020555
- Brunelle JL, Green R. One-dimensional SDS-polyacrylamide gel electrophoresis (1D SDS-PAGE). Methods Enzymol. 2014;541:151–159.
- Magdeldin S, Enany S, Yoshida Y, et al. Basics and recent advances of two dimensional- polyacrylamide gel electrophoresis. Clin Proteomics. 2014;11(1):16. doi: 10.1186/1559-0275-11-16
- Arentz G, Weiland F, Oehler MK, et al. State of the art of 2D DIGE. Proteomics Clin Appl. 2015;9(3–4):277–288. doi: 10.1002/prca.201400119
- Shi Y, Xiang R, Horvath C, et al. The role of liquid chromatography in proteomics. J Chromatogr A. 2004;1053(1–2):27–36. doi: 10.1016/S0021-9673(04)01204-X
- Banerjee S, Mazumdar S. Electrospray ionization mass spectrometry: a technique to access the information beyond the molecular weight of the analyte. Int J Anal Chem. 2012;2012:282574. doi: 10.1155/2012/282574
- Li D, Yi J, Han G, et al. MALDI-TOF mass spectrometry in clinical analysis and research. ACS Meas Sci Au. 2022;2(5):385–404. doi: 10.1021/acsmeasuresciau.2c00019
- Neagu AN, Jayathirtha M, Baxter E, et al. Applications of Tandem Mass Spectrometry (MS/MS) in protein analysis for biomedical research. Molecules. 2022;27(8):2411. doi: 10.3390/molecules27082411
- Carnielli CM, Macedo CCS, De Rossi T, et al. Combining discovery and targeted proteomics reveals a prognostic signature in oral cancer. Nat Commun. 2018;9(1):3598. doi: 10.1038/s41467-018-05696-2
- Birhanu AG. Mass spectrometry-based proteomics as an emerging tool in clinical laboratories. Clin Proteomics. 2023;20(1):32. doi: 10.1186/s12014-023-09424-x
- Lou R, Shui W. Acquisition and analysis of DIA-Based proteomic data: a comprehensive survey in 2023. Mol Cell Proteomics. 2024;23(2):100712. doi: 10.1016/j.mcpro.2024.100712
- Murray KK, Boyd RK, Eberlin MN, et al. Definitions of terms relating to mass spectrometry (IUPAC recommendations 2013). Pure Appl Chem. 2013;85(7):1515–1609. doi: 10.1351/PAC-REC-06-04-06
- Kooren JA, Rhodus NL, Tang C, et al. Evaluating the potential of a novel oral lesion exudate collection method coupled with mass spectrometry-based proteomics for oral cancer biomarker discovery. Clin Proteomics. 2011;8(1):13. doi: 10.1186/1559-0275-8-13
- Alhendi AS. A review: protein identification by LC-MS: principles, instrumentation, and applications. Iraqi J Sci. 2020;61(10):2448–2466. doi: 10.24996/ijs.2020.61.10.2
- Chang CY, Picotti P, Huttenhain R, et al. Protein significance analysis in selected reaction monitoring (SRM) measurements. Mol Cell Proteomics. 2012;11(4):M111 014662. doi: 10.1074/mcp.M111.014662
- Duncan MW, Yergey AL, Patterson SD. Quantifying proteins by mass spectrometry: the selectivity of SRM is only part of the problem. Proteomics. 2009;9(5):1124–1127. doi: 10.1002/pmic.200800739
- Unwin RD, Griffiths JR, Whetton AD. Simultaneous analysis of relative protein expression levels across multiple samples using iTRAQ isobaric tags with 2D nano LC–MS/MS. Nat Protoc. 2010;5(9):1574–1582. doi: 10.1038/nprot.2010.123
- Pan S, Aebersold R. Quantitative proteomics by stable isotope labeling and mass spectrometry. Methods Mol Biol. 2007;367:209–218.
- Chen X, Sun Y, Zhang T, et al. Quantitative proteomics using isobaric labeling: a practical guide. Int J Geno Prot. 2021;19(5):689–706. doi: 10.1016/j.gpb.2021.08.012
- Chu HW, Chang KP, Hsu CW, et al. Identification of salivary biomarkers for oral cancer detection with untargeted and targeted quantitative proteomics approaches. Mol Cell Proteomics. 2019;18(9):1796–1806. doi: 10.1074/mcp.RA119.001530
- Shan J, Sun Z, Yang J, et al. Discovery and preclinical validation of proteomic biomarkers in saliva for early detection of oral squamous cell carcinomas. Oral Dis. 2019;25(1):97–107. doi: 10.1111/odi.12971
- van Leeuwen SJM, Proctor GB, Staes A, et al. The salivary proteome in relation to oral mucositis in autologous hematopoietic stem cell transplantation recipients: a labelled and label-free proteomics approach. BMC Oral Health. 2023;23(1):460. doi: 10.1186/s12903-023-03190-w
- St John MA, Li Y, Zhou X, et al. Interleukin 6 and interleukin 8 as potential biomarkers for oral cavity and oropharyngeal squamous cell carcinoma. Arch Otolaryngology–Head & Neck Surgery. 2004;130(8):929–935. doi: 10.1001/archotol.130.8.929
- Kumar P, Gupta S, Das BC. Saliva as a potential non-invasive liquid biopsy for early and easy diagnosis/prognosis of head and neck cancer. Transl Oncol. 2024;40:101827. doi: 10.1016/j.tranon.2023.101827
- Parker CE, Borchers CH. Mass spectrometry based biomarker discovery, verification, and validation–quality assurance and control of protein biomarker assays. Mol Oncol. 2014;8(4):840–858. doi: 10.1016/j.molonc.2014.03.006
- Alhajj M, Zubair M, Farhana A. Enzyme Linked Immunosorbent Assay. In: StatPearls. Treasure Island (FL); 2024. https://www.ncbi.nlm.nih.gov/books/NBK555922/
- Begum H, Murugesan P, Tangutur A. Western blotting: a powerful staple in scientific and biomedical research. Biotechniques. 2022;73(1):58–69. doi: 10.2144/btn-2022-0003
- Singh KK, Gupta A, Bharti C, et al. Emerging techniques of western blotting for purification and analysis of protein. Future J Pharm Sci. 2021;7(1):239. doi: 10.1186/s43094-021-00386-1
- Bass JJ, Wilkinson DJ, Rankin D, et al. An overview of technical considerations for Western blotting applications to physiological research. Scandinavian J Med Sci Sports. 2017;27(1):4–25. doi: 10.1111/sms.12702
- Dunphy K, Dowling P, Bazou D, et al. Current methods of post-translational modification analysis and their applications in blood cancers. Cancers (Basel). 2021;13(8):1930. doi: 10.3390/cancers13081930
- Ahsan H. Monoplex and multiplex immunoassays: approval, advancements, and alternatives. Comp Clini Pathol. 2022;31(2):333–345. doi: 10.1007/s00580-021-03302-4
- Huang Y, Zhu H. Protein Array-based Approaches for Biomarker Discovery in Cancer. Int J Geno Prot. 2017;15(2):73–81. doi: 10.1016/j.gpb.2017.03.001
- Fasolo J, Snyder M. Protein microarrays. Methods Mol Biol. 2009;548:209–222.
- Honarmand M, Saravani R, Farhad-Mollashahi L, et al. Salivary Lactate Dehydrogenase, C-Reactive Protein, and Cancer Antigen 125 Levels in Patients with oral lichen planus and oral squamous cell carcinoma. International Journal Of Cancer Management. 2021;14(3):e108344. doi: 10.5812/ijcm.108344
- Chang YT, Chu LJ, Liu YC, et al. Verification of saliva matrix metalloproteinase-1 as a strong diagnostic Marker of oral cavity cancer. Cancers (Basel). 2020;12(8):2273. doi: 10.3390/cancers12082273
- Faria PCB, Carneiro AP, Binato R, et al. Upregulation of tropomyosin alpha-4 chain in patients’ saliva with oral squamous cell carcinoma as demonstrated by phage display. Sci Rep. 2019;9(1):18399. doi: 10.1038/s41598-019-54686-x
- Deepthi G, Nandan SR, Kulkarni PG. Salivary tumour necrosis factor-α as a biomarker in oral leukoplakia and oral squamous cell carcinoma. Asian Pac J Cancer Prev. 2019;20(7):2087–2093. doi: 10.31557/APJCP.2019.20.7.2087
- van der Merwe L, Wan Y, Cheong HJ, et al. A pilot study to profile salivary angiogenic factors to detect head and neck cancers. BMC Cancer. 2018;18(1):734. doi: 10.1186/s12885-018-4656-3
- Peisker A, Raschke GF, Fahmy MD, et al. Salivary MMP-9 in the detection of oral squamous cell carcinoma. Med Oral Patol Oral Cir Bucal. 2017;22(3):e270–275. doi: 10.4317/medoral.21626
- Yu JS, Chen YT, Chiang WF, et al. Saliva protein biomarkers to detect oral squamous cell carcinoma in a high-risk population in Taiwan. Proc Natl Acad Sci USA. 2016;113(41):11549–11554. doi: 10.1073/pnas.1612368113
- Metgud R, Bajaj S. Altered serum and salivary C-reactive protein levels in patients with oral premalignant lesions and oral squamous cell carcinoma. Biotechnic Histochem. 2016;91(2):96–101. doi: 10.3109/10520295.2015.1077393
- Saluja TS, Spadigam A, Dhupar A, et al. Equating salivary lactate dehydrogenase (LDH) with LDH-5 expression in patients with oral squamous cell carcinoma: An insight into metabolic reprogramming of cancer cell as a predictor of aggressive phenotype. Tumour Biol. 2016;37(4):5609–5620. doi: 10.1007/s13277-015-4415-x
- Giebultowicz J, Wroczynski P, Samolczyk-Wanyura D. Comparison of antioxidant enzymes activity and the concentration of uric acid in the saliva of patients with oral cavity cancer, odontogenic cysts and healthy subjects. J Pathology Med. 2011;40(9):726–730. doi: 10.1111/j.1600-0714.2011.01045.x
- Brinkmann O, Kastratovic DA, Dimitrijevic MV, et al. Oral squamous cell carcinoma detection by salivary biomarkers in a Serbian population. Oral Oncol. 2011;47(1):51–55. doi: 10.1016/j.oraloncology.2010.10.009
- Arellano-Garcia ME, Hu S, Wang J, et al. Multiplexed immunobead-based assay for detection of oral cancer protein biomarkers in saliva. Oral Dis. 2008;14(8):705–712. doi: 10.1111/j.1601-0825.2008.01488.x
- Rhodus NL, Ho V, Miller CS, et al. NF-kappaB dependent cytokine levels in saliva of patients with oral preneoplastic lesions and oral squamous cell carcinoma. Cancer Detect Prev. 2005;29(1):42–45. doi: 10.1016/j.cdp.2004.10.003
- Contucci AM, Inzitari R, Agostino S, et al. Statherin levels in saliva of patients with precancerous and cancerous lesions of the oral cavity: a preliminary report. Oral Dis. 2005;11(2):95–99. doi: 10.1111/j.1601-0825.2004.01057.x
- Balicki R, Grabowska SZ, Citko A. Salivary epidermal growth factor in oral cavity cancer. Oral Oncol. 2005;41(1):48–55. doi: 10.1016/j.oraloncology.2004.06.004
- Korostoff A, Reder L, Masood R, et al. The role of salivary cytokine biomarkers in tongue cancer invasion and mortality. Oral Oncol. 2011;47(4):282–287. doi: 10.1016/j.oraloncology.2011.02.006
- Sivadasan P, Gupta MK, Sathe G, et al. Salivary proteins from dysplastic leukoplakia and oral squamous cell carcinoma and their potential for early detection. J Proteomics. 2020;212:103574. doi: 10.1016/j.jprot.2019.103574
- Hsiao YC, Lin SY, Chien KY, et al. An immuno-MALDI mass spectrometry assay for the oral cancer biomarker, matrix metalloproteinase-1, in dried saliva spot samples. Anal Chim Acta. 2020;1100:118–130. doi: 10.1016/j.aca.2019.12.006
- Ishikawa S, Ishizawa K, Tanaka A, et al. Identification of salivary proteomic biomarkers for oral cancer Screening. Vivo (Athens, Greece). 2021;35(1):541–547. doi: 10.21873/invivo.12289
- Kang Y, Chen J, Li X, et al. Salivary KLK5 and uPA are potential biomarkers for malignant transformation of OLK and OLP. Cancer Biomark. 2021;31(4):317–328. doi: 10.3233/CBM-203105
- Nakamichi E, Sakakura H, Mii S, et al. Detection of serum/salivary exosomal Alix in patients with oral squamous cell carcinoma. Oral Dis. 2021;27(3):439–447. doi: 10.1111/odi.13565
- Babiuch K, Kusnierz-Cabala B, Kesek B, et al. Evaluation of Proinflammatory, NF-kappaB Dependent Cytokines: IL-1α, IL-6, IL-8, and TNF-α in Tissue specimens and saliva of patients with oral squamous cell carcinoma and oral potentially malignant disorders. J Clin Med. 2020;9(3):867. doi: 10.3390/jcm9030867
- Zielinska K, Karczmarek-Borowska B, Kwasniak K, et al. Salivary IL-17A, IL-17F, and Tnf- α Are Associated with Disease Advancement in Patients with Oral and Oropharyngeal Cancer. J Immunol Res. 2020;2020:1–8. doi: 10.1155/2020/3928504
- Rathore AS, Katyal S, Jain A, et al. Biochemical analysis of cytokeratin fragment 21-1 concentration and expression of cytokeratin 19 in oral potentially malignant disorders. J Cancer Res Ther. 2020;16(3):452–457. doi: 10.4103/jcrt.JCRT_893_17
- Marton IJ, Horvath J, Labiscsak P, et al. Salivary IL-6 mRNA is a robust biomarker in oral squamous cell carcinoma. J Clin Med. 2019;8(11):1958. doi: 10.3390/jcm8111958
- Lin YH, Eguez RV, Torralba MG, et al. Self-assembled strap for global proteomics and salivary biomarker discovery. J Proteome Res. 2019;18(4):1907–1915. doi: 10.1021/acs.jproteome.9b00037
- Feng Y, Li Q, Chen J, et al. Salivary protease spectrum biomarkers of oral cancer. Int J Oral Sci. 2019;11(1):7. doi: 10.1038/s41368-018-0032-z
- Janssen EM, Dy SM, Meara AS, et al. Analysis of patient preferences in lung cancer - estimating acceptable tradeoffs between treatment benefit and side effects. Patient Prefer Adherence. 2020;14:927–937. doi: 10.2147/PPA.S235430
- Zheng J, Sun L, Yuan W, et al. Clinical value of Naa10p and CEA levels in saliva and serum for diagnosis of oral squamous cell carcinoma. J Oral Pathology Med. 2018;47(9):830–835. doi: 10.1111/jop.12767
- Lopez-Verdin S, Soto-Avila JJ, Zamora-Perez AL, et al. Patients with advanced oral squamous cell carcinoma have high levels of soluble E-cadherin in the saliva. Med Oral Patol Oral Cir Bucal. 2017;22(6):e694–e701. doi: 10.4317/medoral.21907
- Csosz E, Labiscsak P, Kallo G, et al. Proteomics investigation of OSCC-specific salivary biomarkers in a Hungarian population highlights the importance of identification of population-tailored biomarkers. PLOS ONE. 2017;12(5):e0177282. doi: 10.1371/journal.pone.0177282
- Zanotti L, Paderno A, Piazza C, et al. Epidermal growth factor receptor detection in serum and saliva as a diagnostic and prognostic tool in oral cancer. Laryngoscope. 2017;127(11):E408–E414. doi: 10.1002/lary.26797
- Ghallab NA, Shaker OG. Serum and salivary levels of chemerin and MMP-9 in oral squamous cell carcinoma and oral premalignant lesions. Clin Oral Investig. 2017;21(3):937–947. doi: 10.1007/s00784-016-1846-8
- Honarmand MH, Farhad-Mollashahi L, Nakhaee A, et al. Salivary Levels of ErbB2 and CEA in oral squamous cell carcinoma patients. Asian Pac J Cancer Prev. 2016;17(S3):77–80. doi: 10.7314/APJCP.2016.17.S3.77
- Malhotra R, Urs AB, Chakravarti A, et al. Correlation of Cyfra 21-1 levels in saliva and serum with CK19 mRNA expression in oral squamous cell carcinoma. Tumour Biol. 2016;37(7):9263–9271. doi: 10.1007/s13277-016-4809-4
- Ajona D, Pajares MJ, Chiara MD, et al. Complement activation product C4d in oral and oropharyngeal squamous cell carcinoma. Oral Dis. 2015;21(7):899–904. doi: 10.1111/odi.12363
- Winck FV, Prado Ribeiro AC, Ramos Domingues R, et al. Insights into immune responses in oral cancer through proteomic analysis of saliva and salivary extracellular vesicles. Sci Rep. 2015;5(1):16305. doi: 10.1038/srep16305
- Rajkumar K, Ramya R, Nandhini G, et al. Salivary and serum level of CYFRA 21-1 in oral precancer and oral squamous cell carcinoma. Oral Dis. 2015;21(1):90–96. doi: 10.1111/odi.12216
- Goncalves AS, Arantes DA, Bernardes VF, et al. Immunosuppressive mediators of oral squamous cell carcinoma in tumour samples and saliva. Hum Immunol. 2015;76(1):52–58. doi: 10.1016/j.humimm.2014.11.002
- Patel S, Metgud R. Estimation of salivary lactate dehydrogenase in oral leukoplakia and oral squamous cell carcinoma: a biochemical study. J Cancer Res Ther. 2015;11(1):119–123. doi: 10.4103/0973-1482.138193
- Varun C, Dineshkumar T, Jayant VS, et al. Salivary Her2/neu levels in differentiation of oral premalignant disorders and oral squamous cell carcinomas. Asian Pac J Cancer Prev. 2015;16(14):5773–5777. doi: 10.7314/APJCP.2015.16.14.5773
- Arduino PG, Menegatti E, Cappello N, et al. Possible role for interleukins as biomarkers for mortality and recurrence in oral cancer. Int J Biol Markers. 2015;30(2):e262–266. doi: 10.5301/jbm.5000142
- Hsu CW, Yu JS, Peng PH, et al. Secretome profiling of primary cells reveals that THBS2 is a salivary biomarker of oral cavity squamous cell carcinoma. J Proteome Res. 2014;13(11):4796–4807. doi: 10.1021/pr500038k
- Jou YJ, Hua CH, Lin CD, et al. S100A8 as potential salivary biomarker of oral squamous cell carcinoma using nanoLC-MS/MS. Clin Chim Acta. 2014;436:121–129. doi: 10.1016/j.cca.2014.05.009
- Mu AK, Chan YS, Kang SS, et al. Detection of host-specific immunogenic proteins in the saliva of patients with oral squamous cell carcinoma. J Immunoassay Immunochem. 2014;35(2):183–193. doi: 10.1080/15321819.2013.836535
- Jessie K, Jayapalan JJ, Rahim ZH, et al. Aberrant proteins featured in the saliva of habitual betel quid chewers: an indication of early oral premalignancy? Electrophoresis. 2014;35(24):3504–3511. doi: 10.1002/elps.201400252
- Chaiyarit P, Utrawichian A, Leelayuwat C, et al. Investigation of trefoil factor expression in saliva and oral mucosal tissues of patients with oral squamous cell carcinoma. Clin Oral Investig. 2012;16(6):1549–1556. doi: 10.1007/s00784-011-0667-z
- Szanto I, Mark L, Bona A, et al. High-throughput screening of saliva for early detection of oral cancer: a pilot study. Technol Cancer Res Treat. 2012;11(2):181–188. doi: 10.7785/tcrt.2012.500248
- Sartini D, Pozzi V, Renzi E, et al. Analysis of tissue and salivary nicotinamide N-methyltransferase in oral squamous cell carcinoma: basis for the development of a noninvasive diagnostic test for early-stage disease. Biol Chem. 2012;393(6):505–511. doi: 10.1515/hsz-2012-0112
- Stott-Miller M, Houck JR, Lohavanichbutr P, et al. Tumor and salivary matrix metalloproteinase levels are strong diagnostic markers of oral squamous cell carcinoma. Cancer Epidemiol Biomarkers Preven. 2011;20(12):2628–2636. doi: 10.1158/1055-9965.EPI-11-0503
- Jou YJ, Lin CD, Lai CH, et al. Salivary zinc finger protein 510 peptide as a novel biomarker for detection of oral squamous cell carcinoma in early stages. Clin Chim Acta. 2011;412(15–16):1357–1365. doi: 10.1016/j.cca.2011.04.004
- Shintani S, Hamakawa H, Ueyama Y, et al. Identification of a truncated cystatin SA-I as a saliva biomarker for oral squamous cell carcinoma using the SELDI ProteinChip platform. Int J Oral Maxillofacial Surg. 2010;39(1):68–74. doi: 10.1016/j.ijom.2009.10.001
- Hu S, Arellano M, Boontheung P, et al. Salivary proteomics for oral cancer biomarker discovery. Clin Cancer Res. 2008;14(19):6246–6252. doi: 10.1158/1078-0432.CCR-07-5037
- Pickering V, Jordan RC, Schmidt BL. Elevated salivary endothelin levels in oral cancer patients–a pilot study. Oral Oncol. 2007;43(1):37–41. doi: 10.1016/j.oraloncology.2005.12.027
- Zhong LP, Zhang CP, Zheng JW, et al. Increased Cyfra 21-1 concentration in saliva from primary oral squamous cell carcinoma patients. Arch Oral Biol. 2007;52(11):1079–1087. doi: 10.1016/j.archoralbio.2007.05.005
- Rhodus NL, Cheng B, Myers S, et al. The feasibility of monitoring NF-kappaB associated cytokines: TNF-alpha, IL-1alpha, IL-6, and IL-8 in whole saliva for the malignant transformation of oral lichen planus. Mol Carcinog. 2005;44(2):77–82. doi: 10.1002/mc.20113
- Xie H, Onsongo G, Popko J, et al. Proteomics analysis of cells in whole saliva from oral cancer patients via value-added three-dimensional peptide fractionation and tandem mass spectrometry. Mol Cell Proteomics. 2008;7(3):486–498. doi: 10.1074/mcp.M700146-MCP200
- Xiao H, Zhang Y, Kim Y, et al. Differential proteomic analysis of human saliva using tandem Mass tags quantification for gastric cancer detection. Sci Rep. 2016;6(1):22165. doi: 10.1038/srep22165
- Gold L, Ayers D, Bertino J, et al. Aptamer-based multiplexed proteomic technology for biomarker discovery. PLOS ONE. 2010;5(12):e15004. doi: 10.1371/journal.pone.0015004
- Wang K, Shen Y, Xu J, et al. Evaluation of synuclein-gamma levels by novel monoclonal antibody in saliva and cancer tissues from oral squamous cell carcinoma patients. Neoplasma. 2020;67(3):707–713. doi: 10.4149/neo_2020_190619N523
- Kawahara R, Bollinger JG, Rivera C, et al. A targeted proteomic strategy for the measurement of oral cancer candidate biomarkers in human saliva. Proteomics. 2016;16(1):159–173. doi: 10.1002/pmic.201500224
- Nosratzehi F, Nosratzehi T, Alijani E, et al. Salivary beta2-microglobulin levels in patients with erosive oral lichen planus and squamous cell carcinoma. BMC Res Notes. 2020;13(1):294. doi: 10.1186/s13104-020-05135-w
- Singh P, Verma JK, Singh JK. Validation of salivary markers, IL-1beta, IL-8 and Lgals3bp for detection of oral squamous cell carcinoma in an Indian population. Sci Rep. 2020;10(1):7365. doi: 10.1038/s41598-020-64494-3
- Bernardes VF, Gleber-Netto FO, Sousa SF, et al. Clinical significance of EGFR, Her-2 and EGF in oral squamous cell carcinoma: a case control study. J Exp Clin Cancer Res. 2010;29(1):40. doi: 10.1186/1756-9966-29-40
- Sabarathinam J, Selvaraj J, Devi S. Estimation of levels of glutathione peroxidase (gpx), malondialdehyde (mda), tumor necrosis factor alpha (Tnf Alpha) and alpha feto protein (Afp) in saliva of potentially malignant disorders and oral squamous cell carcinoma. Biomed Pharm J. 2019;12(4):1881–1886. doi: 10.13005/bpj/1818
- Smriti K, Ray M, Chatterjee T, et al. Salivary MMP-9 as a biomarker for the diagnosis of oral potentially malignant disorders and oral squamous cell carcinoma. Asian Pac J Cancer Prev. 2020;21(1):233–238. doi: 10.31557/APJCP.2020.21.1.233
- Csosz E, Markus B, Darula Z, et al. Salivary proteome profiling of oral squamous cell carcinoma in a Hungarian population. FEBS Open Bio. 2018;8(4):556–569. doi: 10.1002/2211-5463.12391
- Cai M, Zheng Z, Bai Z, et al. Overexpression of angiogenic factors and matrix metalloproteinases in the saliva of oral squamous cell carcinoma patients: potential non-invasive diagnostic and therapeutic biomarkers. BMC Cancer. 2022;22(1):530. doi: 10.1186/s12885-022-09630-0
- Geng XF, Du M, Han JX, et al. Saliva CA125 and TPS levels in patients with oral squamous cell carcinoma. Int J Biol Markers. 2013;28(2):216–220. doi: 10.5301/jbm.5000001
- Lisa Cheng YS, Jordan L, Gorugantula LM, et al. Salivary interleukin-6 and -8 in patients with oral cancer and patients with chronic oral inflammatory diseases. J Periodontol. 2014;85(7):956–965. doi: 10.1902/jop.2013.130320
- Vajaria BN, Patel KR, Begum R, et al. Salivary glyco-sialylation changes monitors oral carcinogenesis. Glycoconj J. 2014;31(9):649–659. doi: 10.1007/s10719-014-9561-7
- Aziz S, Ahmed SS, Ali A, et al. Salivary Immunosuppressive Cytokines IL-10 and IL-13 are significantly elevated in oral squamous cell carcinoma patients. Cancer Invest. 2015;33(7):318–328. doi: 10.3109/07357907.2015.1041642
- Krapfenbauer K, Drucker E, Thurnher D. Identification of tumour-related proteins as potential screening markers by proteome analysis-protein profiles of human saliva as a predictive and prognostic tool. EPMA Journal. 2014;5(1):20. doi: 10.1186/1878-5085-5-20
- Li SX, Yang YQ, Jin LJ, et al. Detection of survivin, carcinoembryonic antigen and ErbB2 level in oral squamous cell carcinoma patients. Cancer Biomark. 2016;17(4):377–382. doi: 10.3233/CBM-160651
- Chianeh YR, Manjunath R, Prabhu K, et al. Protein thiols and butyrylcholinesterase in saliva of oral cancer patients. Indian J Clin Biochem. 2014;29(2):238–241. doi: 10.1007/s12291-013-0352-x
- Vajaria BN, Patel KR, Begum R, et al. Evaluation of serum and salivary total sialic acid and alpha-l-fucosidase in patients with oral precancerous conditions and oral cancer. Oral Surg Oral Med Oral Pathol Oral Radiol. 2013;115(6):764–771. doi: 10.1016/j.oooo.2013.01.004
- Ko HH, Peng HH, Cheng SJ, et al. Increased salivary AKR1B10 level: association with progression and poor prognosis of oral squamous cell carcinoma. Head & Neck. 2018;40(12):2642–2647. doi: 10.1002/hed.25370
- Seyedmajidi S, Seyedmajidi M, Foroughi R, et al. Comparison of salivary and serum soluble CD44 levels between patients with oral scc and healthy controls. Asian Pac J Cancer Prev. 2018;19(11):3059–3063. doi: 10.31557/APJCP.2018.19.11.3059
- Heawchaiyaphum C, Pientong C, Phusingha P, et al. Peroxiredoxin-2 and zinc-alpha-2-glycoprotein as potentially combined novel salivary biomarkers for early detection of oral squamous cell carcinoma using proteomic approaches. J Proteomics. 2018;173:52–61. doi: 10.1016/j.jprot.2017.11.022
- Jou YJ, Lin CD, Lai CH, et al. Proteomic identification of salivary transferrin as a biomarker for early detection of oral cancer. Anal Chim Acta. 2010;681(1–2):41–48. doi: 10.1016/j.aca.2010.09.030
- Gholizadeh N, Mohebbi AH, Mirzaii-Dizgah I, et al. α1 adrenergic receptors in serum and saliva of patients with oral squamous cell carcinoma. Clin Transl Oncol. 2021;23(8):1705–1710. doi: 10.1007/s12094-021-02571-3
- Shpitzer T, Hamzany Y, Bahar G, et al. Salivary analysis of oral cancer biomarkers. Br J Cancer. 2009;101(7):1194–1198. doi: 10.1038/sj.bjc.6605290
- Gleber-Netto FO, Yakob M, Li F, et al. Salivary biomarkers for detection of oral squamous cell carcinoma in a Taiwanese population. Clin Cancer Res. 2016;22(13):3340–3347. doi: 10.1158/1078-0432.CCR-15-1761
- Sato J, Ohuchi M, Abe K, et al. Correlation between salivary interleukin-6 levels and early locoregional recurrence in patients with oral squamous cell carcinoma: preliminary study. Head Neck. 2013;35(6):889–894. doi: 10.1002/hed.23056
- Wu CC, Chang YT, Chang KP, et al. Salivary auto-antibodies as noninvasive diagnostic markers of oral cavity squamous cell carcinoma. Cancer Epidemiol Biomarker Prevent. 2014;23(8):1569–1578. doi: 10.1158/1055-9965.EPI-13-1269
- Rai AK, Panda M, Das AK, et al. Dysbiosis of salivary microbiome and cytokines influence oral squamous cell carcinoma through inflammation. Arch Microbiol. 2021;203(1):137–152. doi: 10.1007/s00203-020-02011-w
- Schork K, Podwojski K, Turewicz M, et al. Important issues in planning a proteomics experiment: statistical considerations of quantitative proteomic data. Methods Mol Biol. 2021;2228:1–20.
- Voss H, Schlumbohm S, Barwikowski P, et al. HarmonizR enables data harmonization across independent proteomic datasets with appropriate handling of missing values. Nat Commun. 2022;13(1):3523. doi: 10.1038/s41467-022-31007-x
- Wik L, Nordberg N, Broberg J, et al. Proximity extension assay in combination with next-generation sequencing for high-throughput proteome-wide analysis. Mol Cell Proteomics. 2021;20:100168. doi: 10.1016/j.mcpro.2021.100168
- Kraemer S, Vaught JD, Bock C, et al. From SOMAmer-based biomarker discovery to diagnostic and clinical applications: a SOMAmer-based, streamlined multiplex proteomic assay. PLOS ONE. 2011;6(10):e26332. doi: 10.1371/journal.pone.0026332
- Bourmaud A, Gallien S, Domon B. A quality control of proteomic experiments based on multiple isotopologous internal standards. EuPA Open Proteom. 2015;8:16–21. doi: 10.1016/j.euprot.2015.07.010
- Zhou C, Simpson KL, Lancashire LJ, et al. Statistical considerations of optimal study design for human plasma proteomics and biomarker discovery. J Proteome Res. 2012;11(4):2103–2113. doi: 10.1021/pr200636x
- Serdar CC, Cihan M, Yucel D, et al. Sample size, power and effect size revisited: simplified and practical approaches in pre-clinical, clinical and laboratory studies. Biochem med (Online). 2021;31(1):27–53. doi: 10.11613/BM.2021.010502
- Hajian-Tilaki K. Sample size estimation in diagnostic test studies of biomedical informatics. J Biomed Informat. 2014;48:193–204. doi: 10.1016/j.jbi.2014.02.013
- Andrade C. Sample size and its importance in research. Indian J Psychol Med. 2020;42(1):102–103. doi: 10.4103/IJPSYM.IJPSYM_504_19
- Gumpili SP, Das AV. Sample size and its evolution in research. IHOPE Journal Of Ophthalmology. 2022;1:9–13. doi: 10.25259/IHOPEJO_3_2021
- Magaki S, Hojat SA, Wei B, et al. An Introduction to the Performance of Immunohistochemistry. Methods Mol Biol. 2019;1897:289–298.
- Matos LL, Trufelli DC, de Matos MG, et al. Immunohistochemistry as an important tool in biomarkers detection and clinical practice. Biomark Insights. 2010;5:9–20. doi: 10.4137/BMI.S2185
- Gupta R, Kurc T, Sharma A, et al. The emergence of Pathomics. Curr Pathobiol Rep. 2019;7(3):73–84. doi: 10.1007/s40139-019-00200-x
- Bankhead P, Loughrey MB, Fernandez JA, et al. QuPath: open source software for digital pathology image analysis. Sci Rep. 2017;7(1):16878. doi: 10.1038/s41598-017-17204-5
- Normando AGC, Dos Santos ES, Sa JO, et al. A meta-analysis reveals the protein profile associated with malignant transformation of oral leukoplakia. Front Oral Health. 2023;4:1088022. doi: 10.3389/froh.2023.1088022
- Arroyo E, Donis SP, Petronacci CMC, et al. Usefulness of protein-based salivary markers in the diagnosis of oral potentially malignant disorders: A systematic review and meta-analysis. Cancer Biomark. 2021;32(4):411–424. doi: 10.3233/CBM-203043
- Piyarathne NS, Rasnayake R, Angammana R, et al. Diagnostic salivary biomarkers in oral cancer and oral potentially malignant disorders and their relationships to risk factors - a systematic review. Expert Rev Mol Diagn. 2021;21(8):789–807. doi: 10.1080/14737159.2021.1944106
- Chiamulera MMA, Zancan CB, Remor AP, et al. Salivary cytokines as biomarkers of oral cancer: a systematic review and meta-analysis. BMC Cancer. 2021;21(1):205. doi: 10.1186/s12885-021-07932-3
- Iglesias-Velazquez O, Lopez-Pintor RM, Gonzalez-Serrano J, et al. Salivary LDH in oral cancer and potentially malignant disorders: a systematic review and meta-analysis. Oral Dis. 2022;28(1):44–56. doi: 10.1111/odi.13630
- Sa JO, Trino LD, Oliveira AK, et al. Proteomic approaches to assist in diagnosis and prognosis of oral cancer. Expert Rev Proteomics. 2021;18(4):261–284. doi: 10.1080/14789450.2021.1924685
- Amenabar JM, Da Silva BM, Punyadeera C. Salivary protein biomarkers for head and neck cancer. Expert Rev Mol Diagn. 2020;20(3):305–313. doi: 10.1080/14737159.2020.1722102
- Benito-Ramal E, Egido-Moreno S, Gonzalez-Navarro B, et al. Role of selected salivary inflammatory cytokines in the diagnosis and prognosis of oral squamous cell carcinoma. A systematic review and meta-analysis. Med Oral Patol Oral Cir Bucal. 2023;28(5):e474–e486. doi: 10.4317/medoral.25889
- Hema Shree K, Ramani P, Sherlin H, et al. Saliva as a diagnostic tool in oral squamous cell carcinoma – a systematic review with meta analysis. Pathol Oncol Res. 2019;25(2):447–453. doi: 10.1007/s12253-019-00588-2
- AlAli AM, Walsh T, Maranzano M. CYFRA 21-1 and MMP-9 as salivary biomarkers for the detection of oral squamous cell carcinoma: a systematic review of diagnostic test accuracy. Int J Oral Maxillofacial Surg. 2020;49(8):973–983. doi: 10.1016/j.ijom.2020.01.020