Abstract
Previous research has found that Greek membership strongly predicts smoking and alcohol consumption. This paper examined the influence of popularity and conforming to perceived peer norms on smoking and drinking among college fraternity members using social network analysis. Social learning theory (Bandura, 1977) and diffusion of innovations (Rogers, 2003) were applied as theoretical frameworks. Results of a longitudinal study indicated that popularity significantly predicted both smoking and drinking behaviors. Strong homophily existed for smoking, but not for the number of drinks consumed per day. Smoking and drinking were significantly correlated, and diffusion of both behaviors occurred in the network over a period of time. Conforming to peer norms also mediated the effect of popularity on smoking and drinking within the peer social network. Popular members both smoked and drank more, but this occurred due to their greater likelihood of conforming to their peer group's smoking and drinking norms. Implications for future research are discussed.
Smoking is one of the top public health problems in the United States, despite tough anti-smoking legislation and widespread public awareness of negative health consequences: 80–90% of adult smokers begin smoking by age 18 (Alexander, Piazza, Mekos, & Valente, Citation2001), with peer influence identified as a leading correlate and probable cause of smoking (Hoffman, Sussman, Unger, & Valente, Citation2006). Peer norms can influence and encourage both positive and negative health habits. In particular, popular students exert a strong influence in setting peer group norms, providing cues to the acceptability or unacceptability of a behavior, and thus act as opinion leaders for a behavior within their social networks (Valente, Unger, & Johnson, Citation2005).
Social Learning Theory
A useful theoretical framework for the study of peer influence is social learning theory (Bandura, Citation1977), which states that behavior is learned through role modeling, whereby a behavior modeled is viewed as positive and attractive, leading to increased rates of uptake for the behavior. The presence of positive smoking models in one's social environment promotes an atmosphere of tolerance towards smoking, thereby increasing rates of smoking among adolescents (Hoffman et al., Citation2006). Popular students act as positive role models within their social groups, shaping the perceived norms, attitudes, and behaviors of other students (Valente et al., Citation2005). In turn their behavior is also heavily influenced by perceived norms of their social groups. They will attempt to set trends while not deviating too much from the norms of their group (Valente et al., Citation2005).
Diffusion of Innovations
Diffusion of innovations (Rogers, Citation2003) is another important theoretical construct used in the study of smoking and drinking within social groups. It posits that the adoption of an innovation is dependent on the behavior of opinion leaders in a person's social network (Rogers, Citation2003). A person's position within his or her social group determines when he or she will adopt a behavior, and the role that this person will play in spreading the behavior to others. Members of a social group may pick up smoking at different times, and as the number of smoking peers a person has increases, so does his or her likelihood of picking up the habit. Valente (Citation1995) found that innovations will first be adopted by opinion leaders, those with the highest number of friends’ nominations in a social group. In school settings these opinion leaders correspond to popular students, who are most often construed as positive role models in the spread of behavior.
Through a longitudinal study the current research examined whether group norms and perceived popularity influences smoking and drinking among a network of college fraternity members. First, social network analysis (SNA) was used to map the network of influence for smoking and drinking. It was also used to measure whether popularity was associated with greater smoking and drinking, as well as whether homophily (defined as a preference for associating or bonding with similar others) existed for both behaviors. SNA is an analytic technique that represents connections among individuals within social networks, showing how these relations can influence behavior (Valente, Citation1995). The overall patterns of relationships in networks are important because they help to accelerate or inhibit the diffusion of behaviors among group members. Second, the study assessed the diffusion of smoking and drinking in a fraternity network between 2007 and 2010, tracking the spread of these behaviors in the network over a period of time. Finally, mediation analysis (Baron & Kenny, Citation1986) tested whether there was a causal effect of popularity on smoking and drinking, and whether the relationship was affected by the tendency of popular students to conform to peer norms for smoking and drinking.
Smoking and drinking are major health problems in American colleges and universities. In the Greek system especially these behaviors are often normative and encouraged, with the result that there is a high prevalence of smokers and binge drinkers among fraternity and sorority members. Many studies have found that Greek membership significantly predicts smoking (Lantz, Citation2003; Morrell, Cohen, Bacchi, & West, Citation2005) and binge drinking (DeSimone, Citation2009; Sher, Bartholow, & Nanda, Citation2001). Students who smoke are also more likely to binge drink (Costa, Jessor, & Turbin, Citation2007; Lo & Globetti, Citation1995; Weitzman & Chen, Citation2005). Hence the prevalence of these unhealthy habits among college students, especially fraternity members, warrants research.
• | H1a: Popularity within the fraternity network is significantly associated with smoking. | ||||
• | H1b: Popularity within the fraternity network is significantly associated with drinking. | ||||
• | H2: Smoking and drinking are significantly correlated within the fraternity network. | ||||
• | H3a: Homophily exists among a group of fraternity members for smoking. | ||||
• | H3b: Homophily exists among a group of fraternity members for drinking. |
By tracking the smoking and drinking behavior of a fraternity network between 2007 and 2010, the study also examined whether popular students influence smoking and drinking within a network over a period of time. Another aim of the study was to examine whether popularity within the fraternity network had a causal influence on smoking and drinking, and whether conformity to peer norms mediated this relationship.
• | H4a: Popularity within the fraternity network is significantly associated with conforming to peer norms for smoking. | ||||
• | H4b: Popularity within the fraternity network is significantly associated with conforming to peer norms for drinking. | ||||
• | H5a: Conforming to peer norms for smoking mediates the association between popularity and smoking. | ||||
• | H5b: Conforming to peer norms for drinking mediates the association between popularity and drinking. |
Method
The college fraternity network studied was part of a large Greek-letter student organization at a private 4-year university in the southwestern United States. The network chosen consisted of 34 members of the fraternity, all of whom were freshmen who pledged in the fall semester of 2007. For time period one a link to an online questionnaire was sent to members of the network on November 1, 2007. For time period two the same 34 members of the network were contacted by email on November 1, 2010 (3 years after the initial questionnaire), and asked to fill out a follow-up questionnaire. All members of the network, now college seniors at the time of the follow-up, returned the completed questionnaires by January 2011.
In the time period one questionnaire respondents were asked to identify fraternity members they turned to for social support, hung out with on a regular basis, and trusted to talk about their problems. They were asked four questions about their smoking and four questions about their drinking. The smoking questions asked whether they smoked cigarettes, number of cigarettes smoked per day, intention to quit, and whether they were more likely to smoke when hanging out with friends who smoke. The drinking questions asked whether they drank alcohol, number of drinks consumed per day, intention to quit, and whether they were more likely to drink when hanging out with friends who drink. For time period two each member of the network was again asked the same eight questions regarding their smoking and drinking.
Results
Descriptive statistics
In the network 76.5% (26) were Caucasian, 17.6% (6) were Hispanic, and 5.9% (2) were Asian. Average age during time period one was 20.1 years. Of the participants 41.2% (14) were Christian, 38.2% (13) were Catholic, 17.6% (6) were Jewish, and 2.9% (1) were Buddhist; 61.8% (21) were from Los Angeles, 8.8% (3) were from Washington D.C., 5.9% (2) were from Miami, and the rest were from other cities in the United States. For smoking behavior, half of the network (N = 17) were smokers during both time periods, although various members quit or started smoking between then. In time period one 41.2% (7) smoked 16 to 20 cigarettes per day, compared to 35.3% (6) in time period two. A total of 41.2% (7) in time period one answered “definitely yes” on intention to quit smoking, compared to 52.9% (9) in time period two. In time period one 52.9% (9) were “definitely more likely” to smoke when around other smokers, compared to 58.8% (10) in time period two. For alcohol consumption, all members (N = 34) reported drinking alcohol in both time periods. In time period one 32.4% (11) reported having five to six drinks per day, compared to 14.7% (5) in time period two. 50.0% (17) answered “definitely no” on intention to quit drinking in time period one, compared to 20.6% (7) in time period two. A total of 14.7% (5) were “definitely more likely” to drink when around other drinkers in time period one, compared to 29.4% (10) in time period two.
Smoking behavior
Quadratic Assignment Procedure (QAP) correlation analyses were conducted on the three networks: “turn to for social support”, “hang out with on a regular basis”, and “trust to talk about problems”. QAP correlations calculate measures of nominal, ordinal, and interval association between the relations in two matrices (Hanneman & Riddle, Citation2005). Results revealed that they were all highly correlated (QAPs > .94). Because of that, and also because of the highly similar friendship connections across the network diagrams, this study conducted social network analysis (SNA) on the “Hang out with on a regular basis” network, as smoking and drinking are social activities usually undertaken in the company of friends.
shows the distribution of smokers in the network during time period one (2007). The network consisted of three major clusters: one large group of smokers and two groups of non-smokers. Nodes 2, 3, 5, 7, 14, 21, and 30 formed the main non-smoking group, with nodes 9, 11, 15, 18, 19, and 34 forming the second non-smoking group. The large smoking group consisted of nodes 4, 8, 13, 22, 24, 25, and 27.
Figure 1. Distribution of smokers and non-smokers in the fraternity network during 2007 (time period one). Smoker = square, non-smoker = circle. [To view this figure in color, please visit the online version of this Journal.]
![Figure 1. Distribution of smokers and non-smokers in the fraternity network during 2007 (time period one). Smoker = square, non-smoker = circle. [To view this figure in color, please visit the online version of this Journal.]](/cms/asset/a794a3d5-cc05-4e37-bc9f-e683d29f97f8/psif_a_584445_o_f0001g.gif)
Interestingly, among this group of smokers was node 20, a non-smoker who was nonetheless highly connected to the smokers in the network. Four peripheral nodes (6, 23, 29, and 33) were all connected to a smoker, and all four of these nodes also smoked. A few nodes acted as connectors between the smokers and non-smokers, namely nodes 4 and 26 (smokers who were also connected to the main non-smoking group) and nodes 3, 14, and 30 (who were non-smokers but connected with smokers). The clusters of smokers and non-smokers show that smokers tended to hang out with other smokers, and non-smokers with other non-smokers. ANOVA density models were then used to test whether there is evidence of homophily. The regression coefficient was = 0.10 (standardized), p<.05, hence supporting H3a. There is evidence of homophily in the network for smoking. This is a reasonable expectation, since smoking is a social activity and people tend to hang out with others who share their same habits. Further, since people are influenced by their peers the smoking or non-smoking behavior of other members may be a factor of the group in which they belong.
shows the distribution by number of cigarettes smoked during time period one (2007). Four of the most central nodes (22, 24, 13, and 25) were also the heaviest smokers, while the less-central nodes tended to be non-smokers. Nodes 22 and 24 also had the highest centrality, and hence were the most popular members of the network. As such, it can be said that popularity and heavier smoking are highly inter-related in the network. The person-by-person network based on smoking behavior was found to be significantly correlated with the “Hang out” network, and QAP correlation = 0.101, p<.05. Smoking behavior therefore significantly predicts who the members of the fraternity hang out with. Smokers tended to hang out with other smokers, and non-smokers tended to hang out with non-smokers.
Figure 2. Distribution of the fraternity network by number of cigarettes smoked per day during 2007 (time period one). Circle = 0, square = 1 to 5, triangle = 6 to 10, lined square = 16 to 20, diamond = more than 20 cigarettes. [To view this figure in color, please visit the online version of this Journal.]
![Figure 2. Distribution of the fraternity network by number of cigarettes smoked per day during 2007 (time period one). Circle = 0, square = 1 to 5, triangle = 6 to 10, lined square = 16 to 20, diamond = more than 20 cigarettes. [To view this figure in color, please visit the online version of this Journal.]](/cms/asset/1429a544-ecd9-47af-8feb-da509f7aa520/psif_a_584445_o_f0002g.gif)
shows the distribution of smokers and non-smokers in the fraternity network during time period two (2010). Nodes 9, 20, and 18 were non-smokers but hung out mostly with smokers, and all of them picked up smoking between 2007 and 2010. Additionally node 19, who was directly connected to the three new smokers in the network (nodes 9, 20, and 18), also picked up smoking. As such, it can be seen that non-smokers who hung out with other smokers are also highly likely to pick up smoking over a period of time. However, the opposite effect was also observed during time period two, with several smokers who were directly connected to non-smokers quitting smoking between 2007 and 2010. The smokers who quit smoking were nodes 1, 10, 26, and 28. As seen in these nodes were connected to more non-smokers than smokers during time period one, and therefore became non-smokers because they most likely modeled the smoking behavior of the network members whom they hung out with. Nodes 1 and 17 were only connected to each other, with node 1 being a smoker, and node 17 being a non-smoker at time period one. However, at time period two, instead of node 17 becoming a smoker, node 1 became a non-smoker. Diffusion of smoking therefore worked in both directions. The more someone hung out with people who smoked or did not smoke, the greater the likelihood of them conforming to the smoking norms of these people. During time period two the fraternity network was more evenly distributed into two main clusters, with smokers and non-smokers forming two distinct groups.
Figure 3. Distribution of smokers and non-smokers in the fraternity network during 2010 (time period two). Smoker = square, non-smoker = circle. [To view this figure in color, please visit the online version of this Journal.]
![Figure 3. Distribution of smokers and non-smokers in the fraternity network during 2010 (time period two). Smoker = square, non-smoker = circle. [To view this figure in color, please visit the online version of this Journal.]](/cms/asset/b6c6a7ad-f80d-487b-9176-02064231c0e7/psif_a_584445_o_f0003g.gif)
Statistical analyses for smoking behavior in time period two also shows support for homophily. The regression coefficient found from the ANOVA density models was = 0.15, p<.05, showing that in time period two, homophily continued to exist for smoking within the fraternity network, hence supporting H3a. Additionally, the person-by-person network based on smoking behavior was also found to be significantly correlated with the “Hang out” network, with QAP correlation = 0.128, p<.05. Therefore, in 2010, smoking behavior continued to significantly predict whom one hangs out with in the fraternity network, with smokers and non-smokers hanging out in separate cliques.
Drinking behavior
All 34 members of the fraternity network reported drinking alcohol in both time periods one and two, supporting DeSimone's (Citation2009) assertion that fraternity membership is strongly associated with drinking. shows the distribution by number of drinks consumed per day in the network during time period one.
Figure 4. Distribution by number of drinks per day in the fraternity network during 2007 (time period one). Circle = 1 to 2 drinks, square = 3 to 4 drinks, triangle = 5 to 6 drinks, diamond = 7 to 8 drinks per day. [To view this figure in color, please visit the online version of this Journal.]
![Figure 4. Distribution by number of drinks per day in the fraternity network during 2007 (time period one). Circle = 1 to 2 drinks, square = 3 to 4 drinks, triangle = 5 to 6 drinks, diamond = 7 to 8 drinks per day. [To view this figure in color, please visit the online version of this Journal.]](/cms/asset/e47d1bf2-6027-4bce-a8ba-7ae2bce2a43e/psif_a_584445_o_f0004g.gif)
During time period one (2007) members who hung out together also tended to have the same number of drinks per day. Nodes 19, 23, and 32 were all connected to each other, and had one to two drinks a day. Nodes 13, 30, 25, and 27 (in the main smoking group) and nodes 2, 14, and 21 (in the main non-smoking group) all had three to four drinks per day. Similarly, nodes 3, 5, 7, and 26 were all connected to each other, and had five to six drinks per day. An interesting finding is that the two most central nodes, 22 and 24, were also the heaviest drinkers. Node 22 had seven to eight drinks per day while node 24 had five to six drinks a day. As such, popularity within the network can be interpreted as being associated with heavier drinking. The person-by-person network based on number of drinks per day shows that the largest group had three to four drinks per day, followed by five to six drinks, one to two drinks, and seven to eight drinks. The QAP correlation between the “Hang out” network and the number of drinks per day was found to be non-significant (QAP correlation = .027, p = .28). ANOVA density models were then used to look for evidence of homophily, or whether members who have the same number of drinks per day hang out together. Regression coefficient obtained was = 0.0003 (standardized), p = .55, which was also non-significant, thereby H3b was not supported. As such, there is no correlation between the number of drinks per day, and whom one hangs out with in the network.
shows the distribution by number of drinks per day in the network during time period two (2010). As can be seen, nodes 6 and 22, both of whom reported having seven to eight drinks per day during time period one (2007), reduced their alcohol consumption to only three to four drinks per day during time period two (2010). In addition, nodes 4, 10, 11, 12, 18, 24, and 33, all of whom reported having five to six drinks per day during time period one, also reduced their alcohol consumption to three to four drinks per day during time period two. Nodes 4, 10, 11, 12, and 33 were only connected to others who consumed less alcohol than they did in time period one, and therefore they gradually reduced their alcohol consumption due to the influence of the members they hung out with. Nodes 18 and 24 also reduced their alcohol consumption along with their connections, nodes 6 and 22, who were the heaviest drinkers during time period one. In contrast, nodes 19 and 29 increased their alcohol consumption from one to two drinks per day to three to four drinks, due to their connections to heavier drinkers. The same was observed for node 21, who increased his alcohol consumption from three to four drinks per day to five to six drinks, due to his connections to heavier drinkers. Hence alcohol consumption diffused through the network over time. Those who hung out with others who consumed more alcohol increased their number of drinks per day, and those who hung out with others who consumed less alcohol decreased their number of drinks per day. Consuming three to four drinks per day became normative within the network, occupying the most central positions. Hence peer influence was a strong influence on drinking in the network.
Figure 5. Distribution by number of drinks per day in the fraternity network during 2010 (time period two). Circle = 1 to 2 drinks, square = 3 to 4 drinks, triangle = 5 to 6 drinks, diamond = 7 to 8 drinks per day. [To view this figure in color, please visit the online version of this Journal.]
![Figure 5. Distribution by number of drinks per day in the fraternity network during 2010 (time period two). Circle = 1 to 2 drinks, square = 3 to 4 drinks, triangle = 5 to 6 drinks, diamond = 7 to 8 drinks per day. [To view this figure in color, please visit the online version of this Journal.]](/cms/asset/6481ad39-68e0-4f0e-a678-23d0745a6bc3/psif_a_584445_o_f0005g.gif)
Statistical analyses for drinking behavior in time period two was also conducted. The regression coefficient obtained from the ANOVA density models was = 0.19 (standardized), p<.05, showing strong evidence of homophily. This is in direct contrast to the results from time period one, showing that in 2010, but not in 2007, homophily exists for the number of drinks consumed per day. As such, H3b is supported for the data in 2010, but not for 2007. Additionally, the QAP correlation between the “Hang out” network and the number of drinks per day was = 0.134, p<.05, showing that in time period two, members of the network hung out with others who consumed the same number of drinks per day. As such, the network not only became more homogeneous in terms of the number of drinks consumed per day, but the members also hung out significantly more regularly with others who consumed the same number of drinks per day.
Correlation of smoking and drinking
To test whether smoking and drinking were correlated, QAP correlation analysis was performed on the two person-by-person networks: smoking and number of drinks per day. Smoking was found to be significantly correlated with the number of drinks per day for the fraternity members (QAP Correlation = 0.11, p<.05), thereby supporting H2. As such, smokers in the fraternity network also tended to have a greater number of alcoholic drinks per day, compared to non-smokers in the network.
For the mediation analysis (Baron & Kenny, Citation1986), the survey data was converted to SPSS-compatible format, and analyzed using SPSS 17. Popularity was measured by the number of in-degree friend nominations. Smoking behavior was measured by the number of cigarettes smoked per day. Drinking behavior was measured by the number of drinks per day. Conforming to peer norms for both smoking and drinking were measured by whether the respondent was more likely to smoke or drink when hanging out with other smokers or drinkers.
Popularity
Bivariate correlation analyses were performed to test H1a/b and H4a/b. Popularity within the network was positively associated with more cigarettes smoked per day (R = .71, p<.01, N = 34, one-tailed), so H1a was supported. Popularity was also positively associated with number of drinks per day (R = .67, p<.05, N = 34, one-tailed), hence H1b was also supported. Additionally, popularity was positively associated with likelihood of smoking more when around other smokers (R = .81, p<.01, N = 34, one-tailed), therefore H4a was supported. Popularity was also positively associated with likelihood of drinking more when around other drinkers (R = .79, p<.01, N = 34, one-tailed), hence H4b was supported.
Mediation analyses
Tests were done to determine whether the relationship between popularity and smoking (R = .81, p<.01) and popularity and drinking (R = .79, p<.01) was mediated by conformity to peer norms (H5a/b), i.e., the likelihood of smoking/drinking when around other smokers/drinkers (). Likelihood of smoking more when around other smokers was positively associated with more cigarettes smoked per day (R = .68, p<.05, N = 34, one-tailed), while likelihood of drinking more when around other drinkers was also positively associated with number of drinks per day (R = .83, p<.01, N = 34, one-tailed). Hence the mediator was significantly related to both smoking and drinking as outcome measures.
Figure 6. Relationship between popularity, conforming to peer norms, and smoking and drinking behavior among the fraternity network.
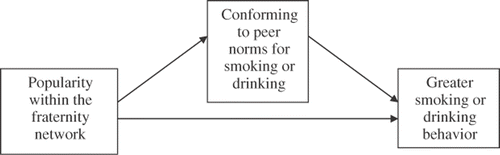
Partial correlation analysis was then performed to test H5a/b, controlling for conforming to peer norms. Holding conforming to peer norms constant, popularity no longer had a significant effect on smoking behavior (R = .12, p = .18, N = 34, one-tailed). The Sobel Test statistic (Sobel, Citation1982) was 2.59, p<.02, indicating that conforming to peer norms significantly mediated that relationship (H5a). For drinking, holding conforming to peer norms constant, popularity also no longer had a significant effect on drinking (R = .08, p = .55, N = 34, one-tailed). The Sobel statistic was 2.67, p<.02, again indicating that conformity significantly mediated the observed relationship, supporting H5b.
Next, popularity was tested as a mediator. Holding popularity constant, conforming to peer norms continued to have a significant effect on smoking (R = .67, p<.05, N = 34, one-tailed), indicating that popularity did not mediate the relationship. For drinking, holding popularity constant, conforming to peer norms also continued to have a significant effect on number of drinks (R = .54, p<.05, N = 34, one-tailed), indicating that popularity did not mediate the relationship.
Discussion
Results of this study indicate that smoking and drinking are highly correlated (H2). The QAP correlation between the smoking and drinking person-by-person networks shows a significant positive association between smoking and number of drinks per day. Smokers tend to also be heavier drinkers within the network. Analyses also revealed that smokers tend to hang out with other smokers, and non-smokers with other non-smokers in both 2007 and 2010; hence a tendency towards homophily for smoking (H3a). For drinking in 2007, the same was not found: the correlation between the number of drinks per day and the “Hang out” network was not significant. However, in 2010 the correlation between the number of drinks per day and the “Hang out” network became highly significant, suggesting that members of the network hung out with others who had the same number of drinks per day (H3b). Since all 34 members of the network drink alcohol, with fraternities being social organizations whereby like-minded students self-select to live together, it can be said that drinkers hang out with other drinkers. Overall, the study found stronger homophily for smoking than for drinking between 2007 and 2010. Since smoking and drinking are social activities, it is fair to say that members of the fraternity socialize with others like themselves, resulting in a high level of homophily.
Additionally, this study also found that between 2007 and 2010 smoking and drinking diffused through the network. For smoking, non-smokers who hung out mainly with smokers in 2007 picked up smoking by 2010, and vice versa. For drinking, those members who hung out mainly with others who consumed a greater number of drinks per day also increased their alcohol consumption between 2007 and 2010. Similarly, those who mainly hung out with others who consumed less alcohol per day also reduced their number of drinks per day between the two time periods. Fraternity members therefore influenced others whom they were directly connected to for both smoking and drinking.
Additionally, popularity within the network is strongly associated with smoking and drinking. The more popular a member is (i.e., the greater number of in-degree nominations he has), the more likely he is to smoke (H1a), or be a heavier drinker (H1b). Also, the more popular one is within the fraternity network, the more likely one is to conform to peer norms regarding smoking (H4a) and drinking (H4b). The more one conforms to the perceived peer norm of smoking or drinking, the more one is likely to smoke, or have more drinks per day respectively. Further, conforming to peer norms with regards to smoking and drinking mediates the relationships between popularity and smoking (H5a), and popularity and drinking (H5b).
Therefore popularity in the fraternity network significantly predicts smoking and heavier drinking. A significant correlation was also found between smoking and drinking. The more an individual drank, the more he smoked, and vice versa. There is also a strong tendency towards homophily for smoking. Smokers socialize with other smokers, and non-smokers with other non-smokers. As the study shows, conforming to peer norms for smoking and drinking is the real reason behind the phenomenon of popular members of the fraternity network smoking and drinking more. It can be said that popular students are more likely to smoke or drink more heavily when hanging out with other smokers or drinkers respectively, and this predicts their own smoking or drinking behavior more accurately than their popularity within the fraternity network. Popular students, while setting trends for smoking and drinking within their social networks, also model socially accepted behaviors among their peers (Valente et al., Citation2005). As such, these students’ conforming to peer norms for smoking and drinking when hanging out with their peers strongly predicts their own smoking and drinking.
Conclusion
Smoking and drinking are both unhealthy behaviors that, unfortunately, are prevalent among college students. Because of the “cool” factor often associated with smoking and drinking at that age, college students who want to fit in with their social groups may be influenced to try them. They are also especially prevalent in Greek organizations, due to the social nature of smoking and drinking (DeSimone, Citation2009; Sher et al., Citation2001). This study examined members of a college fraternity network to find out whether peer-perceived popularity had an influence on one's likelihood of smoking and drinking. As previous studies found, popular students set trends within their social networks while modeling socially acceptable behaviors (Alexander et al., Citation2001; Hoffman, Monge, Chou, & Valente, Citation2007). Results indicated that popular members of the network were also more likely to smoke and drink more. However, this was mediated by popular students’ greater likelihood of conforming to the socially accepted smoking and drinking norms of their peers, rather than their status as being popular among their peers. Further, smokers within the network tended to hang out with each other, while non-smokers hung out with other non-smokers. Smoking and drinking were also strongly inter-related, testifying to their co-occurrence among college-age adults.
A limitation associated with this study is that since network data collected from a small group of fraternity members was used, results cannot be generalized to the general collegiate population. Because fraternities attract like-minded students who specifically self-select to live together, the effect of homophily may be much stronger than in the general college student population. Future studies should be conducted among the general college population to further address whether popularity predicts smoking and drinking behaviors.
Popular students set trends within their networks, acting as role models for group behavior. They also have a stronger influence in setting peer group norms and providing cues to the acceptability or unacceptability of smoking and drinking (Valente et al., Citation2005). In networks where smoking and drinking prevalence is high, peer influence and the likelihood of conforming to socially acceptable peer norms, promote these activities. Popular students act as models for group behavior, therefore their smoking and drinking is more likely to be followed by their peers. Because popular students conform strongly to the smoking and drinking norms of their peer groups, in order to maintain their social status within the groups, they may be in greater danger of developing more unhealthy habits. A greater understanding of how peer groups within collegiate social networks operate is needed in order for researchers to administer interventions to curb these unhealthy behaviors, and mitigate negative health consequences.
References
- Alexander , C , Piazza , M , Mekos , D and Valente , TW . 2001 . Peers, schools and adolescent cigarette smoking . Journal of Adolescent Health , 29 : 22 – 30 .
- Bandura , A . 1977 . Social learning theory , Englewood Cliffs, NJ : Prentice-Hall .
- Baron , RM and Kenny , DA . 1986 . The moderator-mediator distinction in social psychological research: Conceptual, strategic and statistical considerations . Journal of Personality and Social Psychology , 51 ( 6 ) : 1173 – 1182 .
- Costa , FM , Jessor , R and Turbin , MS . 2007 . College student involvement in cigarette smoking: The role of psychosocial and behavioral protection and risk . Nicotine and Tobacco Research , 9 ( 2 ) : 213 – 224 .
- DeSimone , JS . 2009 . Fraternity membership and drinking behavior . Economic Inquiry , 47 ( 2 ) : 337 – 350 .
- Hanneman , RA and Riddle , M . 2005 . Introduction to social network methods , Riverside, CA : University of California, Riverside .
- Hoffman , BR , Monge , PR , Chou , CP and Valente , TW . 2007 . Perceived peer influence and peer selection on adolescent smoking . Addictive Behavior , 32 ( 8 ) : 1546 – 1554 .
- Hoffman , BR , Sussman , S , Unger , JB and Valente , TW . 2006 . Peer influences on adolescent cigarette smoking: A theoretical review of the literature . Substance Use and Misuse , 41 ( 1 ) : 103 – 155 .
- Lantz , PM . 2003 . Smoking on the rise among young adults: Implications for research and policy . Tobacco Control , 12 : 60 – 70 .
- Lo , CC and Globetti , G . 1995 . The facilitating and enhancing roles Greek associations play in college drinking . Substance Use & Misuse , 30 ( 10 ) : 1311 – 1322 .
- Morrell , H , Cohen , LM , Bacchi , D and West , J . 2005 . Predictors of smoking and smokeless tobacco use in college students: A preliminary study using web-based survey methodology . Journal of American College Health , 54 ( 2 ) : 108 – 115 .
- Rogers , EM . 2003 . Diffusion of Innovations, , 5th , New York : Free Press .
- Sher , KJ , Bartholow , BD and Nanda , S . 2001 . Short- and long-term effects of fraternity and sorority membership on heavy drinking: A social norms perspective . Psychology of Addictive Behaviors , 15 ( 1 ) : 42 – 51 .
- Sobel , ME . 1982 . “ Asymptotic confidence intervals for indirect effects in structural equation models ” . In Sociological methodology 1982 , Edited by: Leinhardt , S . 290 – 312 . Washington, DC : American Sociological Association .
- Valente , TW . 1995 . Network models of the diffusion of innovations , Cresskill, NJ : Hampton Press .
- Valente , TW , Unger , JB and Johnson , CA . 2005 . Do popular students smoke? The association between popularity and smoking among middle school students . Journal of Adolescent Health , 37 : 323 – 329 .
- Weitzman , ER and Chen , YY . 2005 . The co-occurrence of smoking and drinking among young adults in college: National survey results from the United States . Drug and Alcohol Dependence , 80 : 377 – 386 .