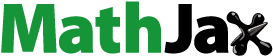
Abstract
This study aims to assess the adoption and impact of Artificial Intelligence (A.I.) tools in higher education, focusing on a private university in Latin America. Guided by the question, “What is the impact, as perceived by university students, of using Artificial Intelligence tools on various dimensions of learning and teaching within the context of higher education?” the study employs a rigorously validated 30-item instrument to examine five key dimensions: 1) Effectiveness use of A.I. tools, 2) Effectiveness use of ChatGPT, 3) Student’s proficiency using A.I. tools, 4) Teacher’s proficiency in A.I. and 5) Advanced student skills in A.I. These dimensions form a synthetic index used for comprehensive evaluation. Targeting 4,127 students from the university’s schools of Engineering, Business, and Arts, the study garnered 21,449 responses, analyzed using Confirmatory Factor Analysis for validity. Findings indicate a significantly positive impact of A.I. tools on student academic experiences, including enhanced comprehension, creativity, and productivity. Importantly, the study identifies areas with low and high A.I. integration, serving as an institutional diagnostic tool. The data underscores the importance of A.I. proficiency among both educators and students, advocating for its integration as a pedagogical evolution rather than just a technological shift. This research has critical implications for data-driven decision-making in higher education, offering a robust framework for institutions aiming to navigate the complexities of A.I. implementation.
PUBLIC INTEREST STATEMENT
This study introduces a pioneering tool, a survey instrument, crafted to gauge the impact of Artificial Intelligence (AI) tools on the university educational experience. Through this instrument, which has been rigorously validated at a private university in Latin America, other institutions can now assess how their students and faculty are utilizing AI. By administering the survey to over 4,000 students, we have found that AI significantly benefits students’ understanding, creativity, and productivity. The developed synthetic index provides universities with a tangible way to measure AI integration and promote its effective use. The findings of this study not only highlight AI as a crucial pedagogical advancement but also offer a robust framework for data-driven decision-making in higher education.
1. Introduction
Artificial Intelligence (A.I.) has emerged as a disruptive force that is profoundly reshaping various aspects of modern society. Since its beginnings in the 1950s as an interdisciplinary field, A.I. has been on an exciting path of discoveries and advancements. In its early days, researchers strove to create machines capable of emulating human intelligence in specific tasks, such as chess and language processing. Over time, A.I. has faced challenges and ups and downs, but has seen a significant resurgence thanks to advances in machine learning and the ability to process huge volumes of data.
There are a variety of definitions of Artificial Intelligence, for the analysis of their study Popenici and Kerr (Citation2017), they define A.I. as computer systems capable of carrying out human-like processes, such as learning, adaptation, synthesis, self-correction and the use of data for complex processing tasks.
According to Purdy and Daugherty (Citation2016), AI refers to various technologies that can be combined in different ways to sense, comprehend, and act. These three competencies are based on the ability to learn from experience and adapt.
According to Popenici and Kerr (Citation2017), they define Machine Learning as a subfield of artificial intelligence that includes software capable of recognizing patterns, making predictions, and applying the newly discovered patterns to situations that were not included or covered by its initial design.
Within the educational field, A.I. has proven to be a tool with immense potential to transform teaching and learning processes, from elementary education to higher education. In essence, A.I. has become a valuable resource that enables the personalization of education, the automation of administrative tasks, and the provision of predictive analytics.
In the context of higher education, the future is closely linked to the continuous development of new technologies and the capacity of intelligent machines. This constantly evolving environment offers a series of unprecedented opportunities and challenges in the field of teaching and learning (Popenici & Kerr, Citation2017).
In particular, university education faces dynamic challenges in the 21st century. College students have a wide variety of learning styles, expectations, and levels of technological proficiency. In response to these complexities, Artificial Intelligence in Higher Education (AIEd) technologies have emerged as an innovative solution. AIEd ranges from adapting learning and providing virtual tutoring to anticipating student performance, automating administrative tasks and improving educational feedback.
Artificial Intelligence can enhance the educational domain through the automation of administrative tasks, provision of intelligent tutoring, and customization of content to meet the needs of each student. This advancement redefines the teacher’s role by freeing them from everyday tasks to focus on more complex skills such as fostering critical thinking and creativity (León & Viña, Citation2017).
Students are in a leadership position in relation to the various opportunities and challenges that arise in the field of learning and teaching in higher education. Currently, advanced solutions have been developed that facilitate interaction and collaboration between individuals and artificial intelligence systems, with the purpose of providing support to people with disabilities (Popenici & Kerr, Citation2017).
However, the incorporation of Artificial Intelligence technologies in educational environments (AIEd) might progress slowly due to the scarcity of resources and a lack of evidence supporting its effectiveness. The question arises whether intelligent tutors could replace educators, although the notion that these tutors could enable teachers to focus on more complex skills is advocated. The introduction of AI in education also alters the transmission of values. Emphasis is placed on integrating ethical and social values, such as honesty and responsibility, alongside educational competencies. The future of education lies in the coexistence and collaboration between human and artificial intelligence, as indicated by León and Viña (Citation2017).
As higher education institutions seek to stay ahead in an ever-transforming educational environment, it is critical to understand both the opportunities AIEd offers and the ethical, pedagogical, and technical challenges that come with its adoption. This understanding is essential to fully embrace this new educational horizon.
The acceptance of AIEd technologies is also influenced by concerns about the risks associated with Artificial Intelligence in general and specifically with AIEd. A framework of AIEd risks has been outlined, prioritizing aspects such as pedagogical incompatibility of AI, misuse of AI resources, potential liability, privacy vulnerability, lack of transparency, perceived risk, bias, and misinterpretation of the human-centered AI concept. These authors argue that managing these risks should be addressed at all stages, from design and development to the acquisition and application of these technologies (Rodway & Schepman, Citation2023).
The purpose of technology in higher education is not limited to simply transmitting information, monitoring or evaluating. Rather, its fundamental objective is to enrich the human thinking capacity and significantly improve the educational process (Popenici & Kerr, Citation2017). A.I. thus becomes a powerful ally for educators and students in their search for more effective and personalized learning in higher education.
2. Literature review
2.1. Educational transformation—The integration of artificial intelligence in higher education (AIEd)
The integration of Artificial Intelligence in Education (AIEd) is revolutionizing higher education by personalizing learning experiences to meet the diverse needs and styles of students. This transformation, highlighted by Ocaña-Fernández et al. (Citation2019), leverages the synergy between human intelligence and information technologies, enhancing educational effectiveness and efficiency. AIEd significantly improves the teacher-student relationship by handling routine tasks, allowing teachers to focus on deeper interactions and student engagement (Kang & Im, Citation2013; Seo et al., Citation2021).
Central to AIEd is the interaction between students and content, peers, and teachers, with student-teacher interactions being especially influential in the learning process (Assaf Silva, Citation2020; Brown & Moore, Citation2012; Martin & Bolliger, Citation2018). AI’s adaptive capabilities are transforming educational methods, dynamically aligning curricula with changing societal and professional requirements.
Despite these advancements, concerns arise about AI’s influence on human aspects of education. Seo et al. (Citation2021) identified challenges including unrealistic student expectations and the necessity for improved AI literacy among educators and learners (Long et al., Citation2021). These insights underscore the importance of balancing technological integration with the preservation of human elements in the educational journey.
The prioritization of digital skills in higher education is vital. Digital literacy goes beyond operating technological tools; it involves the attitudes and skills needed to utilize these tools productively. Universities are tasked with fostering and evaluating digital competencies to prepare graduates for evolving technological environments. Addressing the digital competency generation gap is essential to ensure inclusive benefits from technological advancements in education.
AI’s ongoing evolution and its influence across various domains necessitate an ethical and responsible approach. García et al. (Citation2020) emphasize the importance of the FATE principles (fairness, accountability, transparency, and ethics) in guiding AI’s development and application. Understanding and addressing the challenges posed by AI’s opacity is crucial for its ethical integration into education (Memarian & Doleck, Citation2023).
2.2. Opportunities and threats of A.I. in higher education
Artificial Intelligence (AI) is revolutionizing higher education, shifting the paradigm towards a more student-centered approach. By automating routine administrative tasks, AI facilitates personalized and adaptable learning experiences, thereby redefining the educator’s role. Teachers can now focus on nurturing advanced skills like critical thinking and creativity, instead of being bogged down by mundane tasks. However, the integration of AI in educational institutions faces challenges, such as resource limitations and a need for proven effectiveness (León & Viña, Citation2017). While there’s apprehension about AI tutors replacing human teachers, these tools are seen as an opportunity to enhance the teacher’s role, focusing on imparting critical skills and ethical values.
The synergy between human and AI intelligence is becoming increasingly crucial in education. Understanding AI’s ethical and social implications is paramount to its successful implementation. The use of chatbots, particularly in flipped classrooms, exemplifies AI’s potential in enhancing distance learning. Chatbots, simulating human conversations, have proven effective in increasing student engagement and learning outcomes (Abbas et al., Citation2022; Baskara, Citation2023; Hew et al., Citation2022). In flipped learning environments, they facilitate group discussions, offer personalized feedback, and promote active learning (Diwanji et al., Citation2018; Gonda & Chu, Citation2019). While increasing student autonomy, chatbots should complement, not replace, human interactions, necessitating educators’ proficiency in utilizing these technologies effectively.
2.3. Artificial intelligence in education, the three paradigms
Ouyang and Jiao’s (Citation2021) exploration of Artificial Intelligence (AI) in education identifies three paradigms shaping AI-student interaction. The first paradigm positions AI as an assistant guiding learning, the second as a tool supporting active student collaboration, and the third empowers students to lead their learning. Highlighting the need to integrate educational theories, such as constructivism and situated learning, the article underscores the significance of aligning AI applications with theoretical foundations. This approach enriches AI’s educational impact, addressing the challenge of empowering students to navigate their learning journey within the complexity of modern educational processes.
2.4. ChatGPT in education
Since its inception in November 2022, OpenAI’s ChatGPT, a Generative Pre-trained Transformer with an extensive database of 570 GB (300 billion words) and 175 billion parameters, has been reshaping human-computer interactions. Its primary function is to respond to prompts using natural language processing, with significant implications for educational reform. ChatGPT offers personalized learning experiences, enabling students to engage with content tailored to their unique needs and pace, enhancing the overall educational process without replacing teachers (Javaid et al., Citation2023).
Beyond traditional classroom applications, ChatGPT assists in research, feedback, and writing skill enhancement, further distinguished by its multilingual translation capabilities. This AI tool stands apart from standard search engines by providing precise, targeted responses. However, reliance on ChatGPT raises concerns about students’ deep understanding and potential biases in the AI’s training data. Ethical and legal issues, including data privacy and intellectual property, also accompany its use in educational settings, necessitating careful consideration and responsible application to harness its full potential for enriching learning experiences (Javaid et al., Citation2023).
2.5. Challenges and Difficulties of ChatGPT
The introduction of ChatGPT in higher education has sparked concerns regarding academic integrity, highlighting issues like inaccuracies, biases, and the potential for plagiarism (Liu et al., Citation2023). This AI technology’s limitations, such as its inability to form opinions, reliance on outdated information, inaccessibility to external databases, lack of referencing, susceptibility to mathematical errors, and limited creativity and critical thinking, present significant challenges (Sullivan et al., Citation2023).
Despite its potential to revolutionize mentoring and personalized learning, ChatGPT’s propensity to invent plausible-sounding data raises concerns about the dissemination of incorrect information. Issues regarding copyright, privacy, and data security also need addressing. As educational institutions embrace AI, it becomes imperative to establish protocols that safeguard academic integrity and fair assessment.
Looking ahead, the successful integration of ChatGPT and similar AI tools in education could bring numerous benefits. These tools could offer personalized courses and feedback, allowing teachers to devote more time to course planning and improvement, thus enhancing the overall educational experience. However, to fully realize these benefits, it’s essential to bridge the digital divide and ensure equitable access to technology for all students (Sabzalieva & Valentini, Citation2023). This balanced approach to AI integration in education will be crucial in harnessing its potential while addressing the challenges it presents.
2.6. Research questions
The primary objective of the present research is to diagnose the current state of adoption of Artificial Intelligence (A.I.) tools in teaching and learning processes within higher education. Specifically, this study aims to identify faculty members, courses, and departments that display low levels of A.I. implementation in their subjects, as well as those that demonstrate a high degree of integration and effectiveness in its use. The guiding research question for this work was: What is the impact, as perceived by university students, of using A.I. tools on various dimensions of learning and teaching within the context of higher education?
To effectively address both the research question and the objectives of the study, a quantitative indicator has been developed that allows for comparisons among the various educational strata of the aforementioned institution. The findings of this research have highly relevant practical implications for university administration. For example, the results can be used to formulate specific strategies aimed at strengthening the implementation of A.I. in underperforming subjects. At the same time, they capitalize on the successful experiences of faculty with high levels of A.I. utilization, enabling them to share best practices and foster enthusiasm for integrating A.I. into the educational process with their colleagues.
3. Methods and materials
This section outlines the methodology, detailing the procedures for developing the survey instrument, conducting the pilot study, and collecting data on students’ perceived impact on different dimensions of learning and teaching using A.I. tools applied in higher education. This phase is designed to lay a robust foundation for the study by tailoring the instrument to assess adoption, drawing upon existing research.
3.1. Construction of the study instrument and pilot study
The research tool employed in this study was meticulously developed through an iterative process involving consultations with experts in Artificial Intelligence (A.I.) and pedagogy, as well as pilot testing and successive revisions of the proposed items. Initially, a total of 46 items were considered, covering various dimensions of A.I., including its applicability in educational, corporate, and current labor market contexts. Subsequently, due to the scope of the university and the practicalities of data collection, the focus was narrowed to the educational sphere, specifically targeting pedagogical activities that university faculty can conduct in their classrooms. The result of the study has been delimited by five dimensions showed in the Figure , based on perceptions of the students and explained bellow.
3.2. Dimension 1: Effectiveness use of A.I. tools
Dimension 1 of our investigation delves into the Effectiveness use of A.I. tools within the realm of higher education and comprises nine items. This pivotal dimension aims to elucidate students’ perceptions and experiences with these tools. Our objective is to critically assess the influence of A.I. tools on students’ academic journey.
Our exploration encompasses several facets: How proficiently do these tools address and clarify students’ uncertainties? To what degree do they enhance comprehension of course materials? Can they foster creativity and bolster productivity? Furthermore, we scrutinize their role in knowledge assessment and gauge students’ propensity to advocate for their adoption by peers.
Given the burgeoning influence of artificial intelligence in shaping contemporary educational paradigms, it becomes paramount to ascertain the tangible benefits these tools confer upon students. Grasping their impact on student learning and engagement is crucial for recognizing their indispensable role in contemporary higher education, thereby fostering a more impactful and holistic educational experience.
According to Popenici and Kerr (Citation2017), “With the capacity to guide learning, monitor participation, and student engagement with the content, A.I. can customize the ‘feed’ of information and materials into the course according to learner’s needs, provide feedback and encouragement” (p. 10), highlighting the significance of students being able to greatly benefit from receiving feedback, understanding topics better, and consequently being encouraged to learn
3.3. Dimension 2: Effectiveness use of ChatGPT
In the second facet of our research, denominated Effectiveness use of ChatGPT we delve into the experiential dynamics and application of ChatGPT by students within the educational landscape and comprises seven items. Our objective is to elucidate the transformative influence of this tool on students’ communicative interactions, encompassing both peer-to-peer dialogues and student-teacher exchanges.
Our investigative framework addresses pivotal dimensions. Primarily, we scrutinized ChatGPT’s proficiency in streamlining communication, gauging its adeptness in query formulation and the retrieval of cogent responses. In a complementary vein, we assessed ChatGPT’s capability to help in research, a quintessential feature for academic endeavors such as coursework, assignments, and scholarly projects.
It is an established fact that the new mode of interaction with intelligent chatbots has transformed the way humans communicate with machines. As Baskara (Citation2023) contends, Chatbots can be used to answer student questions, provide explanations, and give feedback on student work, which can help to increase student engagement and motivation in the learning process. According to this author, qualitative methods such as interviews and focus group discussions should be employed to delve deeper into student perceptions of chatbot usage, assisting them not only in summarizing information but also in analyzing it.
Through this analytical lens, our aim is to discern the potential of ChatGPT to augment the scholastic journey of students, serving as a robust conduit for communication and precise information acquisition. Venturing deeper into this domain, we anticipate uncovering strategies by which ChatGPT can elevate the operational efficiency of educational systems.
3.4. Dimension 3: Student’s proficiency using A.I. tools
In the third dimension of our research, denominated Student’s proficiency using A.I. tools our objective is to meticulously assess the proficiency and ease with which students navigate the realm of artificial intelligence tools within the higher education framework and comprises six items. Our investigative queries are designed to probe the depth of students’ confidence and adeptness in employing A.I. tools pertinent to their academic disciplines. We further into their mastery over ChatGPT, gauging its integration into their specialized domains and discerning whether its utilization is perceived as intuitive. An additional facet of our inquiry revolves around the frequency of A.I. tool deployment in their scholastic pursuits.
By adopting this analytical perspective, we endeavor to extract nuanced insights regarding the assimilation and application of sophisticated A.I. tools by students in education. Such insights will enhance our comprehension of the pivotal role these technological advancements play in optimizing student learning trajectories and fostering academic excellence.
Taking into consideration the statement by Ouyang and Jiao (Citation2021), which asserts, “We argue that the future development of the AIEd field must lead to the iterative development of learner-centered, data-driven, personalized learning in the current knowledge age” (p. 5), it underscores the importance of focusing learning on students in light of the new tools they have at their disposal today. If students are adept at using these tools correctly, it will undoubtedly impact their skills and, consequently, enhance their learning.
3.5. Dimension 4: Teacher’s proficiency in A.I.
We begin the explanation of this dimension by considering Popenici and Kerr (Citation2017), who are quoted as saying, the “crossbreed” of the human brain and a machine is already possible, and this will fundamentally challenge teachers to discover new dimensions, functions, and radically new pedagogies for a different context of learning and teaching. All authors of this article are in full agreement with this assertion, recognizing that the use of AI will change the methodologies by which teachers instruct certain subjects. Moreover, if teachers do not keep pace with the students’ knowledge, they risk falling behind and being taken by surprise.In the fourth dimension of our scholarly exploration, designated as Teacher’s proficiency in A.I. we endeavor to critically assess the pedagogical acumen and proactive initiatives of educators concerning the incorporation of artificial intelligence tools within their curriculum and comprises four items. This investigative trajectory is designed to illuminate the depth of educators’ preparedness and their unwavering commitment to this transformative domain.
Our empirical inquiries delve into discerning the extent to which educators exhibit a profound command over A.I. tools and their enthusiasm in championing the use of platforms like ChatGPT within their instructional paradigms. Furthermore, we probe into the potential influence educators might exert on students’ selection of A.I. tools and ascertain the degree of integration of these tools within the pedagogical content and delivery.
By adopting this analytical lens, we aim to unravel the pivotal role educators assume in seamlessly weaving A.I. tools into the educational area. Recognizing and amplifying the intrinsic competencies and proactive endeavors of educators is paramount to elevating the efficacy of education in this burgeoning age of artificial intelligence.
3.6. Dimension 5: Advanced student skills in A.I.
In the fifth dimension denominated as Advanced student skills in A.I. we embark on a meticulous assessment of students’ adeptness and strategic initiatives concerning the deployment of artificial intelligence tools within their academic pursuits and comprises four items. This investigative framework is meticulously crafted to elucidate the nuanced ways in which students harness these tools to augment their scholarly outputs and to critically appraise the pertinence of pedagogical feedback.
Our empirical exploration is centered in the ways the students employ I.A. to refine their compositions, to either elaborate or distill content, and to autonomously critique their submissions prior to educator evaluations. Additionally, we seek to fathom the weightage students accord to the pedagogical feedback in the context of A.I.-enhanced submissions.
Through this analytical prism, we aspire to decode the proficiency and agility with which students leverage artificial intelligence tools to elevate their academic trajectory. Unraveling the intricate tapestry of how students assimilate these avant-garde technologies into their educational journey is quintessential to harnessing the full spectrum of advantages that A.I. instruments present in the realm of tertiary education.
According to Popenici and Kerr (Citation2017), innovation in education is not merely about integrating more technology into classrooms; it is about transforming teaching methodologies to equip students with the skills essential for excelling in competitive global economies. This clearly suggests that in a globalized market, students must possess substantial skills to be competitive, and the advanced use of AI will undoubtedly aid in enhancing their productivity.
Our study methodically developed and validated a comprehensive instrument to assess the use and impact of Artificial Intelligence (AI) tools in higher education. This process involved an iterative approach, with input from experts in AI and pedagogy, culminating in a pilot test conducted with 102 students. The feedback from this test led to minor adjustments in the survey’s vocabulary for clarity, ensuring its suitability for the broader student audience.
The instrument comprises 30 items, structured on a four-point Likert scale ranging from 1 (strongly disagree) to 4 (strongly agree), as detailed in Table . This format is designed to capture a wide spectrum of student opinions and experiences with AI tools in their educational journey. The reliability of the instrument was underscored by high Cronbach’s alpha values, ranging from 0.96 to 0.98 during the pilot phase, indicative of its internal consistency and dependability.
Table 1. Developed survey instrument
The validation of this research tool is a critical step, ensuring the precision and relevance of our findings in the dynamic, interdisciplinary realm of AI in higher education. This tool does not only provide valuable insights for this specific study but also stands as a significant contribution to future research in this evolving field. The careful design and rigorous testing of the instrument make it a valuable asset for further exploration and understanding of the role and effectiveness of AI tools in enhancing educational outcomes.
The measurement model, which encompasses five proposed dimensions and consists of 30 items, is depicted in Figure .
3.7. Participants and population size
The study comprehensively targeted the entire student body of a private university in Latin America, involving a diverse population of 4,127 students across three schools: Engineering, Business, and Arts. The Engineering school, with nine specialized programs, accounted for 1,050 students. The School of Business, offering eight programs, had the largest enrollment with 2,282 students. The School of Arts, focusing on three programs related to arts and communication, comprised 795 students. This demographic diversity provided a rich and varied perspective on the integration of Artificial Intelligence (AI) in higher education.
The survey, methodically administered through the university’s academic system, spanned a broad spectrum of academic disciplines, allowing for a multi-faceted exploration of AI tool usage. The participation of such a large and varied student cohort underscored the effectiveness of our approach, which was designed to be inclusive and representative of the entire university population. The study’s methodical design, which included pilot testing and iterative adjustments based on initial student feedback, was instrumental in achieving a high response rate. Moreover, the university’s proactive stance in integrating and promoting AI tools like ChatGPT significantly contributed to student engagement. This approach not only resulted in a comprehensive dataset that captured a wide array of perceptions on AI tools but also demonstrated the institution’s commitment to understanding and advancing the role of AI in shaping modern educational paradigms.
3.8. Faculty AI Proficiency and development
It is necessary to emphasize that the university has addressed the integration of AI tools into education with seriousness and promptness, particularly since the emergence of ChatGPT in November 2022. The directive to explore and adopt these technologies came directly from the rectorate, highlighting their potential in the educational process. In the months following the launch of ChatGPT, a group of teachers from various disciplines began experimenting with the tool, leading to a series of institutional initiatives to promote its effective use among the faculty.
A series of initiatives were undertaken to enhance the integration of ChatGPT in teaching, commencing with a gathering of educators who shared their insights. To support and expand on these insights, a dedicated web domain focusing on education and artificial intelligence was established. Workshops titled “Introduction to Artificial Intelligence Tools for Teaching” were also conducted. Subsequently, a short course that outlined the basics of ChatGPT was developed and made available on our Learning Management System (LMS). Through these events, faculty members significantly enhanced their skills and expertise in the application of AI to their teaching practices, contributing to the advancement of educational methodologies within the institution.
Furthermore, various heads of departments have been actively encouraging their faculty to use these tools. Although ChatGPT was the initial tool, over time, others have emerged, many specialized in specific fields of knowledge.
Targeted training courses were conducted in the three schools: Engineering, Business, and Arts, focusing on the specific needs of each school. The foundation of these courses was the widespread use of the ChatGPT tool, due to its more generalized adoption.
3.9. Procedure
In line with the organizational structure described earlier, the survey instrument was disseminated via the university’s academic system, ensuring easy access for all students. The survey was administered at the conclusion of each course the students participated in, spanning from March to June 2023, which covers three-fourths of the first semester of that year. Consequently, each student had the opportunity to respond to the survey multiple times, providing insights about their perceived impact of using A.I. tools in learning and teaching in each subject they were enrolled in.
During the analysis period, the average student was enrolled in five different subjects. This multi-point data collection strategy resulted in a comprehensive dataset comprising a total of 21,449 responses, which serve as the foundation for the study’s analysis.
3.10. Data analysis methodology
We applied Confirmatory Factor Analysis (CFA) to assess the structure of our measurement model (as seen in Figure ), a method outlined by Brown and Moore (Citation2012). This technique is vital for verifying the internal coherence and validity of our proposed set of survey questions, which we refer to as indicators. These indicators, rather than being simple or continuous variables, are qualitative with multiple ordered choices, ranging up to four categories. Such a complex nature of the indicators necessitates a specialized approach for analysis.
To effectively analyze these indicators, we employed polychoric correlations. This method is particularly suitable for evaluating relationships between pairs of indicators where responses fall into ordered categories. Polychoric correlations offer a more nuanced understanding of these relationships compared to standard correlation measures, making them ideal for our study’s indicators.
The analysis centers around a meticulously constructed model (referred to as EquationEquation 1(1)
(1) ), wherein each survey question or indicator (denoted as
) is intricately associated with a specific dimension (denoted as d). These dimensions encompass critical aspects of AI tool usage in education, such as the Effectiveness use of A.I. tools, Effectiveness use of ChatGPT, Student’s proficiency using A.I. tools, Teacher’s proficiency in A.I., and Advanced student skills in A.I.
In this model, the factorial score () represents the overall score for each dimension d. The relationship between an individual indicator and its corresponding dimension is quantified through a metric called factorial loading (
). This loading reflects the strength and direction of the association between the indicator and the dimension it belongs to.
Additionally, each indicator possesses a unique component (), which is the part of the indicator’s variance not explained by the factorial score. This uniqueness aspect captures the distinct characteristics of each survey question that aren’t directly attributable to the common underlying dimension.
We ensured that the set of indicators for each dimension had a strong interrelationship, a verification process supported by the Kaiser-Meyer-Olkin (KMO) statistic. This statistic ranges between 0 and 1, with higher values indicating a more significant interrelation among indicators. Generally, a KMO value above 0.7 is considered acceptable, suggesting that the indicators are sufficiently interrelated to summarize a complex concept into fewer dimensions.
Our methodology also included using various goodness-of-fit measures to assess the accuracy of our model. These measures include the Chi-Square Test (χ2), Root Mean Square Error of Approximation (RMSEA), Comparative Fit Index (CFI), Standardized Root Mean Square Residual (SRMR), and the Goodness-of-Fit Index (GFI). Each measure provides a different perspective on how well our model fits the observed data, with lower values in chi-square and RMSEA indicating a better fit and values closer to 1 in CFI suggesting a good fit. The combination of these indices offers a comprehensive assessment of our model’s validity (Cudeck, Citation2000).
Upon confirming the suitability of our model, we constructed a synthetic index using the model. This index integrates all considered indicators, capturing the perceived impact of using AI tools on various dimensions of learning and teaching in higher education. This approach allows us to understand the complex relationships between different aspects of AI tool usage in an educational setting.
4. Results
4.1. Descriptive statistics
To conduct this study, a total of 4,127 students from three distinct schools participated by completing a specially designed survey. Of these participants, 1,050 (25.4%) were enrolled in the School of Engineering, 2,282 (55.3%) in the School of Business, and 795 (19.3%) in the School of Arts.
Table displays the means, standard deviations, skewness, and kurtosis for each variable (item) analyzed in this study. We found that the average perception, based on a Likert scale ranging from 4 (strongly agree) to 1 (strongly disagree), was as follows: For the dimension “Effectiveness use of A.I. Tools” the mean was 3.05 out of 4. For “Effectiveness use of ChatGPT” it was 2.98. The “Student’s proficiency using A.I. Tools” dimension had a mean of 2.99, while ‘Teacher’s proficiency in A.I.” reported a mean of 3. Finally, for the ‘Advanced student skills in A.I.’ dimension the mean score was 2.92. Across all five dimensions, the mean scores fell into the ‘somewhat agree’ category on the established Likert scale. This indicates a generally positive impact on students” perceptions regarding the teaching and learning process, as evidenced by the skewness and kurtosis statistics.
Table 2. Summary of item level descriptive statistics
4.2. Validity of the proposed dimensional structure
The factor analysis process was integral in validating the framework of our study. Initially, from a broader set of 46 items, 30 were meticulously chosen, each tailored specifically to aspects of university pedagogy and organized into five distinct dimensions. This careful selection was then subjected to a rigorous Confirmatory Factor Analysis (CFA), a crucial methodological step in affirming the validity of our dimensional structure. The CFA process revealed a singular dominant eigenvalue for each dimension, highlighting a robust and meaningful interrelation among the indicators. This thorough analysis not only upheld the statistical rigor of our study but also ensured its alignment with the theoretical nuances of AI integration in higher education.
The study, we successfully established and validated five key dimensions that encapsulate the utilization of Artificial Intelligence (AI) tools in higher education. These dimensions encompass a range of aspects central to the integration and effectiveness of AI in the academic milieu.
Firstly, the “Effectiveness of AI Tools” dimension, comprising nine indicators, focuses on the overall utility and impact of these technologies in educational contexts. This dimension assesses how effectively AI tools are enhancing learning experiences and academic outcomes. Secondly, the “Effectiveness of ChatGPT” dimension, with seven indicators, zeros in on the specific contributions of ChatGPT, a prominent AI tool, in modern teaching and learning environments. Its effectiveness in various educational applications is evaluated in this dimension.
The third dimension, “Student’s Proficiency Using AI Tools”, includes six indicators and delves into how well students are able to use and integrate AI technologies into their learning processes. This dimension emphasizes the importance of student engagement and competence in utilizing AI tools effectively. Following this, the fourth dimension, “Teacher’s Proficiency in AI”, assesses educators’ ability to integrate AI tools into their teaching practices. Comprising four indicators, it evaluates the technical and pedagogical mastery of AI tools among educators, as well as their effectiveness in employing these tools in the educational process.
Lastly, the “Advanced Student Skills in AI” dimension, also with four indicators, gauges the depth of students’ understanding and mastery of AI tools, particularly in advanced applications and critical engagement with AI-generated content.
Each dimension, distinct and non-repetitive, offers a unique perspective on AI tool usage in higher education. We utilized a polychoric correlation matrix for each set of indicators to validate these dimensions, ensuring a cohesive explanation by a common underlying factor, as indicated by the presence of a singular eigenvalue exceeding one for each dimension.
The Kaiser-Meyer-Olkin (KMO) statistic further reinforced the validity of our dimensional structure, demonstrating high interrelations among indicators within each dimension. This statistical validation was crucial for ensuring the reliability and applicability of our findings in the evolving field of AI in higher education. The consistency and validity of the proposed dimensional structure were confirmed, with high KMO values across all dimensions, affirming the effective summarization of each set of indicators within its respective dimension (see Table ).
Table 3. KMO statistic by dimension
4.3. Synthetic Index of Use of Artificial Intelligence Tools applied to higher education (SIUAIT)
A synthetic index, termed the Synthetic Index of Use of Artificial Intelligence Tools applied to higher education (SIUAIT), was constructed, and analyzed to capture the perceived impact of using A.I. tools on various dimensions of learning and teaching in higher education.
The previous analysis provided a quantitative assessment of each defined dimension. To consolidate these findings into a unified metric for evaluating the use of A.I. tools in higher education, we conducted a Confirmatory Factor Analysis (CFA) on the entire dataset. Our analyses confirmed the presence of five pivotal dimensions, accounting for 90.54% of the original variability in the set of 30 indicators. Maintaining this extent of variability is vital when formulating the SIUAIT applied to education, as it ensures a nuanced differentiation among respondents based on their perceptions.
These interrelations are quantified by the factorial loads (loadings) presented in Table . Factorial loads measure the magnitude and direction of the relationship between each indicator and the factors that make up the SIUAIT.
Table 4. Factor loadings based on CFA and promax rotation with Kaiser normalization
Once the factorial loads for each indicator in each dimension have been estimated, and the subsequent factorial score in each factor for all individuals in the sample, it is possible to combine this information to create a SIUAIT applied to education. The weights for each of the dimensions are presented in the last row of Table . Approximately, the Effectiveness use of A.I. tools accounts for 31.42%, Effectiveness use of ChatGPT accounts for 26.22%, Student’s proficiency using A.I. tools accounts for 16.84%, Teacher’s proficiency in A.I. accounts for 15.31%, and Advanced student skills in A.I. accounts for 10.21% of the total weight of the index. The tested model showed good fit with a comparative fit index (CFI) of 0.92 (≥0.90), root mean square error of approximation (RMSEA) of 0.046 (<0.05) and mean absolute correlation residual (SRMR) of 0.47 (<0.10).
The study meticulously analyzed 30 indicators, encapsulating the diverse aspects of AI tools’ impact in higher education. This comprehensive approach, as depicted in Figure , revealed five critical dimensions that collectively account for 90.54% of the original data variability. This significant retention of variability is crucial in the construction of a SIUAIT in higher education. Such a high retention rate ensures that this SIUAIT is not only a robust aggregative measure but also retains the nuanced differentiation among individual perceptions. This index effectively integrates the insights from our multidimensional analysis and empirical data, providing a valuable tool for future research and practical applications in the realm of AI integration in educational settings.
Figure , featuring a scree plot, plays a pivotal role in our methodological framework. It graphically elucidates the factor structure of the survey instrument, highlighting the eigenvalues derived from the Confirmatory Factor Analysis (CFA). This visual representation is critical in determining the cut-off point for factor retention, an essential step in our analysis. Adhering to Kaiser’s criterion, our study identified five key dimensions with eigenvalues exceeding one. These dimensions—Effectiveness use of A.I. tools, Effectiveness use of ChatGPT, Student’s proficiency using A.I. tools, Teacher’s proficiency in A.I., and Advanced student skills in A.I.—were chosen through a rigorous process informed by the scree plot and further validated by statistical measures such as the Kaiser-Meyer-Olkin test and Cronbach’s alpha values. This careful selection ensures a thorough and comprehensive understanding of the multiple facets of AI tool usage in educational settings.
4.4. SIUAIT by School
In this section, we outline the values of the SIUAIT in higher education along with its five core dimensions, across three distinct academic disciplines: the School of Engineering, the School of Business, and the School of Arts (Figure for details). We observed that both the School of Engineering and the School of Business scored relatively high on the SIUAIT, with average scores of 66.03 and 65.34, respectively. These figures exceed the overall university score of 63.92 and are not statistically different at a 95% confidence interval. Conversely, the School of Arts scored the lowest on the SIUAIT, registering an average of 57.32 out of 100. This score is significantly different at the 95% confidence level compared to the Engineering and Business Schools, thus showing a distinct profile in AI tool utilization. Furthermore, all three schools exhibited the same pattern of behavior in each of the five validated dimensions, as previously described.
5. Discussion
In this study, we successfully aligned the survey items with the proposed theoretical model. Expert consultations in A.I. and pedagogy guided the development of a 30-item instrument, which closely adhered to the key dimensions of A.I.‘s impact in education. This alignment was confirmed by strong internal consistency, as indicated by high Cronbach’s alpha values, and further validated through Confirmatory Factor Analysis. This process ensured that our survey accurately reflected the complex role of A.I. tools in enhancing the higher education experience as perceived by students.
In Figure , located in the results section, a classification of the programs according to three distinct schools is presented, derived from the academic areas in which the study instrument was applied.
The five factors identified in the statistical analysis, conceptually detailed in the methods section and visualized in Figure , are distributed among the aforementioned schools. It is important to highlight that the study as a whole has the capability to generate specific reports for each program, evaluating these five factors. Additionally, it is possible to analyze the five factors at the individual level of each teacher, thereby allowing for more precise and detailed decision-making. This feature of the study can lead to areas of improvement at the teacher level, as well as identifying potential deficiencies that the teacher should address. Although the granularity of the study is notably detailed, for the purposes of this article, the discussion will be limited to the school level, which nonetheless provides an effective representation of the current state of Artificial Intelligence (A.I.) implementation in the university.
Next, we will proceed to analyze in depth each of the factors associated with the respective schools.
5.1. Factor 1: Effectiveness use of A.I. tools
Factor 1 assesses the effectiveness in the implementation and use of A.I. tools within the educational context. According to Holmes et al. (Citation2023), the adoption of these tools has unleashed new pedagogical possibilities for educators and students, some of which challenge traditional teaching methodologies. This factor encompasses the application of A.I. to enhance learning, including addressing queries, improving content comprehension, finding answers to questions more rapidly, delving deeper into advanced topics, and optimizing the completion of academic tasks.
It is important to note that, given the diversity of programs and academic disciplines, students have had the opportunity to employ A.I. across various fields of knowledge. As Shubhendu and Vijay (Citation2013) point out, A.I. in education has undergone an evolution across multiple areas, significantly contributing to individual productivity. In this context, students have acknowledged an improvement in their academic performance by integrating various A.I. tools into their learning processes.
It is worth noting that while ChatGPT was the most frequently used tool by both teachers and students, the use of other tools such as Perplexity, Bard, Copilot, Wolfram, Bing, Dall-e, MidJourney, QuillBot and Canva can be discerned. Their usage likely varied depending on the subject matter and academic program.
In quantitative terms, the score for Factor 1 at the university is 68.33 out of 100. Breaking down this result by schools, we observe that the Engineering School surpasses the score with 71.27, while the Business School is slightly behind with 69.95. These data suggest that both schools have effectively adopted A.I. tools, which can largely be attributed to the active role of educators in technological promotion and innovation. However, the school of arts presents a considerably lower score, at 60.25.
As the use of A.I. continues to expand, it is likely that students will increasingly utilize these tools. Recent debates in the field of education have underscored the need to develop policies and strategies that promote not only the responsible use of technology in learning, particularly in the realm of Artificial Intelligence. In this context, García (Citation2023) emphasizes the importance of adapting and strengthening academic policies to balance technological innovation with educational ethics, given the rapid evolution of artificial intelligence (AI) tools in education.
5.2. Factor 2: Effectiveness use of ChatGPT
Factor 2 of our study centers on the use of ChatGPT, a leading AI tool developed by OpenAI, which has gained significant prominence since its launch in November 2022. This tool exemplifies the transformative capabilities of AI in various sectors, including education. Rahaman et al. (Citation2023) recognize ChatGPT’s role in the evolution of AI, emphasizing its adoption by major corporations like OpenAI, Bard, Bing, and Meta. Given ChatGPT’s versatility across multiple knowledge domains, it has been selected as the primary focus for this study. The study acknowledges that other institutions might use different text-generating tools, and the research instrument could be adapted accordingly.
In the educational sphere, ChatGPT’s applications are diverse, ranging from facilitating interactions between educators and students, aiding in problem-solving, to supporting academic research. Studies by Castonguay et al. (Citation2023), Gill et al. (Citation2024), Hinojo-Lucena et al. (Citation2019), and Javaid et al. (Citation2023) suggest ChatGPT’s potential to revolutionize education, functioning as a co-tutor and aiding in content creation. Its ability to provide instant responses can foster critical thinking, thereby enhancing the learning experience. Nonetheless, users must be aware of ChatGPT’s limitations and possible inaccuracies, as highlighted by Gill et al. (Citation2024). A critical approach to its responses and cross-referencing with other information sources is recommended.
Quantitatively, the university scored 66.12 out of 100 in utilizing ChatGPT, with the Engineering School achieving 68.91 and the Business School 67.98. These scores indicate positive adoption, though unexpectedly, the Business School did not surpass Engineering, despite the assumption that its subject matter might align more closely with ChatGPT’s capabilities. The Arts School scored lower at 57.51, likely due to ChatGPT’s focus on text processing rather than visual or graphic content, which is more relevant to their disciplines. This disparity highlights the need for diverse AI tools catering to different academic areas to fully harness AI’s educational potential.
On the other hand, there are specific concerns regarding the use of ChatGPT in the educational context, particularly in relation to student data privacy and copyright infringement. These concerns highlight the need for collaboration among educational institutions, teachers, and technology developers to establish clear guidelines and train students in the ethical use of these tools. Sullivan et al. (Citation2023) acknowledge these challenges but also emphasize the potential of ChatGPT and other generative AI tools to enhance learning, provided they are used ethically and appropriately.
5.3. Factor 3: Students’ proficiency using A.I. tools
Factor 3 focuses on the students’ ability to employ A.I. tools in their educational process. While educators play a pivotal role in promoting and facilitating the use of these tools in the classroom, it’s imperative that students are adequately trained to harness them. This training involves not just the technical use of the tools but also the skill to integrate what they’ve learned in class into their practices, projects, and other academic activities, thereby strengthening their understanding and practical application of the content.
For the assessment of this factor, it was deemed essential that students not only be familiar with A.I. tools but also demonstrate a seamless interaction with them, regularly using these tools to address queries and challenges. Although many of the tools used in the educational realm are geared towards text processing and obtaining answers, it’s crucial for students to establish an appropriate interaction with A.I. to achieve the desired outcomes (Chai et al., Citation2021; Lee, Citation2023; Zhai & Wibowo, Citation2023). In this context, Labrague et al. (Citation2023) pinpoint barriers students might face, such as: limited digital competencies, an insufficient understanding of the advantages A.I. can offer in their respective study areas, and lastly, time constraints. It’s worth noting that if students lack basic computer skills, they are likely to encounter difficulties in understanding and efficiently using A.I. tools, which could lead to frustration and a potential abandonment of these technologies in their subject learning.
The university’s overall score for Factor 3 is 66.82 out of 100. Analyzing this result by schools, the Engineering School achieves a score of 69.79, while the Business School records 68.51. These results suggest that both schools have successfully instilled robust competencies in A.I. usage among their students. Given the information provided in Factors 1 and 2, these outcomes were anticipated. However, the school of arts scores lower, with 58.54, consistent with previous results, indicating a lesser adoption and mastery of A.I. tools by its students.
5.4. Factor 4: Teacher’s proficiency in A.I.
Factor 4 addresses the proficiency of educators in the implementation and use of A.I. tools within the educational context. Teachers, as the primary mediators of the teaching-learning process, bear the responsibility of selecting and adapting the most suitable A.I. tools for their respective subjects, ensuring effective integration and guiding students in their proper application.
To assess this proficiency, criteria such as the technical and pedagogical mastery of A.I. tools, active promotion of their use in the classroom, and the frequency and efficacy of their application in the educational context were considered. According to Namatherdhala et al. (Citation2022), the integration of A.I. in education manifests in three main dimensions: (1) instructional design, (2) teaching process, and (3) administrative aspects. In the first dimension, tools like tutorials, reading materials, structured didactic activities, and evaluations are essential (Ulate de Brooke, Citation2011). A.I. can be a valuable ally for educators, especially those requiring support in instructional design, offering guidance and resources to optimize the planning and execution of activities, even assisting in the realization of practices and tasks (Su et al., Citation2023; Zhao & Li, Citation2022). The second dimension, the teaching process, is pivotal as it directly determines the quality of student learning. In this context, Computer-Assisted Intelligent Teaching (AIED) can be highly beneficial. Choi et al. (Citation2022) study highlights that educator with a constructivist pedagogical orientation, based on Vygotsky (Citation1978) theories, show a greater inclination towards implementing A.I. compared to those with transmissive orientations. This suggests a direct correlation between an educator’s teaching methodology and their predisposition towards technological innovation for the implementation of A.I. tools. Lastly, the third dimension pertains to how A.I. can enhance administrative aspects and the services offered to students.
From a quantitative perspective, the overall score for Factor 4 at the university is 66.18 out of 100. Breaking down these results, the Engineering School scores 69.28, while the Business School achieves 67.76. These figures reflect a notable proficiency of educators in both schools in integrating A.I. tools into their subjects. However, the school of arts, with a score of 58.08, demonstrates a lesser degree of adoption and mastery of these technologies, consistent with the findings observed in Factors 1 and 2.
5.5. Factor 5: Advanced student skills in A.I.
Factor 5 in our study focuses on students’ advanced proficiency with AI tools, a critical aspect in today’s AI-integrated educational landscape. This factor assesses students’ abilities to effectively manage texts using AI, such as enhancing, expanding, and synthesizing content, and evaluating assignments and practices. A key skill in this context is the ability to formulate and reformulate AI prompts to achieve precise results, thereby improving their learning outcomes and adapting AI tools to their specific academic needs. This advanced proficiency is not limited to their core academic areas but extends to a broader mastery of AI technologies, suggesting a transformative impact on their educational journey (Baidoo-Anu & Owusu Ansah, Citation2023; Mayer et al., Citation2022; Pavlik, Citation2023).
In our evaluation, Factor 5 scored 63.90 out of 100 university-wide, with the Engineering School and Business School scoring 66.10 and 65.88, respectively. These scores indicate effective adoption and use of AI tools in these schools. However, the School of Arts lagged with a lower score of 55.47, suggesting less proficiency and integration of AI tools in their curriculum. This disparity could be due to the varying nature of AI tools, as tools for text generation are more developed than those for graphic generation, which is more relevant to arts disciplines. This necessitates targeted training strategies to improve AI integration in such areas.
The Synthetic Index of Use of Artificial Intelligence Tools (SIUAIT) reveals an overall index value of 63.92 out of 100 for the university, with the Engineering and Business schools showing more effective leverage of AI tools. In contrast, the School of Arts recorded a lower value of 57.32, highlighting the need for enhanced awareness and training in the use of AI tools within this faculty.
Our study aligns with the AI implementation paradigms proposed by Ouyang and Jiao (Citation2021), identifying three distinct approaches: AI-Directed (learner-as-recipient), AI-Supported (learner-as-collaborator), and AI-Empowered (learner-as-leader). These paradigms reflect different levels of AI integration and interaction in education, ranging from AI guiding the learning process to empowering students as leaders of their learning journey. The study indicates that a blend of these paradigms is at play in the university, with effective teacher-student interaction crucial for fostering an AI-empowered learning environment. The findings highlight the importance of multidimensional AI integration in enhancing educational processes and preparing students for a technologically advanced future.
6. Conclusions
The integration of A.I. tools into higher education has become a focal point of academic discourse in recent times. The conclusions drawn from the study’s findings are as follows:
6.1. Development, validation of the instrument and creation of the Synthetic Index
The validation of the tool consisting of 30 items, ensures the reliability and applicability of the study’s findings. It’s important to note that the data collected mainly reflect students’ perceptions of A.I. tools, rather than direct measurements of their skills or competencies with these technologies. The derivation of a SIUAIT is one of the main contributions of this paper to the scientific community, which can be utilized from the instrument by any educational unit wishing to measure students’ perceptions of using A.I. tools.
6.2. Effectiveness of A.I. tools
The results indicate that A.I. tools have a significant and positive impact on students’ academic experiences. However, these findings are based on self-reported perceptions, and further objective evaluation is needed to substantiate these claims.
6.3. Role of ChatGPT
ChatGPT provides immediate and relevant answers, combined with its application in academic research, makes it a valuable asset in the suite of educational tools that educators. These observations are drawn from qualitative feedback and require further empirical validation to confirm their generalizability.
6.4. Student proficiency with A.I. tools
The study underscores the importance of students not only being familiar with but also proficient in A.I. tools.
6.5. Role and proficiency of educators
Educators play a pivotal role in the successful integration of A.I. tools. Their technical and methodological competence can determine the effective use of these tools in the classroom. The actual proficiency levels of educators with A.I. tools remain an area for further investigation.
6.6. Advanced student skills in A.I.
The study emphasizes the importance of students possessing advanced skills in A.I., especially in content generation. Their ability to efficiently use A.I. tools to enhance, expand, and synthesize content is crucial.
6.7. A.I. implementation paradigms
The integration of A.I. tools in higher education is not merely a technological shift but a methodological evolution, it is imperative to approach this transformation with a critical understanding of the gap between perceived and actual abilities in using these tools.
As A.I. continues to shape the educational landscape, it’s imperative that institutions, educators and students adopt, adapt, and harness these tools effectively. This study provides a snapshot of the current state, but the journey of A.I. in higher education is just beginning. The future promises even more transformative changes, and the academic community must be prepared to navigate this exciting frontier.
7. Limitations and future studies
This study’s limitations stem from its specific context and methodological choices. Conducted in a single educational institution, its applicability to the broader higher education landscape is limited. The study overlooked key factors such as digital literacy, economic accessibility, and familiarity with AI technology, which could significantly affect the adoption and effectiveness of AI tools. Ethical issues like data privacy and responsible AI usage were also not deeply explored. The reliance on self-rated and perception-based measures introduces potential biases, possibly skewing the results and not accurately reflecting actual AI tool competencies or experiences.
For future research, a longitudinal study design is recommended to trace the evolution of AI tool adoption in education. Incorporating qualitative methods like interviews and focus groups would deepen the understanding of AI’s role and perception among educational stakeholders. Extending the study to various university types would help identify challenges and characteristics influencing AI tool use. Comparative studies on different AI tools could highlight their effectiveness in educational settings. Understanding discipline-specific barriers to AI adoption could guide tailored implementation strategies. Developing additional indices might also provide clearer links between AI adoption, student performance, and teacher evaluations, thus enhancing insights into AI’s educational impact.
8. Authors’ contributions
In the study, Johnny Burgos, Alberto Grájeda, and Alberto Sanjinés contributed to its conception and design. Pamela Córdova oversaw the process of data collection, database development, and data processing and analysis. Alberto Grájeda contributed to data interpretation. The manuscript draft was written by Johnny Burgos, Alberto Grájeda and Pamela Córdova. All authors participated in reading the final manuscript and approved its submission.
Availability of data and materials
The datasets generated and analyzed during the current study are not publicly available due to privacy restrictions but are available from the corresponding author on reasonable request.
Disclosure statement
No potential conflict of interest was reported by the author(s).
Additional information
Notes on contributors
Alberto Grájeda
Alberto Grájeda, Ph.D. in Business Innovation from the Universitat Politècnica de València, Spain, leads the Center for Innovation in Information Technology for Education at UPB. His expertise lies in the effective implementation of educational tools utilizing ICT.
Johnny Burgos
Johnny Burgos, professor and researcher in Artificial Intelligence, specializing in international marketing and pricing. He is a doctoral candidate in Business Administration at UPB, Bolivia. Currently, he serves as an academic advisor to the president at UPB.
Pamela Córdova
Pamela Córdova, an expert in Health Economics and Impact Evaluation, holds a Ph.D. in Economics from UPB. She is a professor, researcher at the Economics Center, and currently serves as the Dean of the Faculty of Business Sciences and Law.
Alberto Sanjinés
Alberto Sanjinés, an expert in Business Ethics, Compliance, and Social Responsibility, holds a Ph.D. in Business Administration from UPB, Bolivia. He currently serves as the Academic Vice President at UPB, leading the process of academic and tech-ed innovation.
References
- Abbas, N., Whitfield, J., Atwell, E., Bowman, H., Pickard, T., & Walker, A. (2022). Online chat and chatbots to enhance mature student engagement in higher education. International Journal of Lifelong Education, 41(3), 308–24. https://doi.org/10.1080/02601370.2022.2066213
- Assaf Silva, N. A. J. (2020). The learner-interface interaction´s future, a vision from EdTech. Apertura, 12(2), 150–165. https://doi.org/10.32870/ap.v12n2.1910
- Baidoo-Anu, D., & Owusu Ansah, L. (2023). Education in the era of generative Artificial Intelligence (A.I.): Understanding the potential benefits of ChatGPT in promoting teaching and learning. SSRN Electronic Journal. https://doi.org/10.2139/ssrn.4337484
- Baskara, F. R. (2023). Chatbots and flipped learning: Enhancing student engagement and learning outcomes through personalised support and collaboration. IJORER: International Journal of Recent Educational Research, 4(2), 223–238. https://doi.org/10.46245/ijorer.v4i2.331
- Brown, T. A., & Moore, M. T. (2012). Confirmatory Factor Analysis. In R. H. Hoyle (Ed.), Handbook of Structural Equation Modeling (pp. 361–379). New York, NY: Guilford Publications.
- Castonguay, A., Farthing, P., Davies, S., Vogelsang, L., Kleib, M., Risling, T., & Green, N. (2023). Revolutionizing nursing education through A.I. integration: A reflection on the disruptive impact of ChatGPT. Nurse Education Today, 129, 105916. https://doi.org/10.1016/j.nedt.2023.105916
- Chai, C. S., Lin, P. Y., Jong, M. S. Y., Dai, Y., Chiu, T. K., & Qin, J. (2021). Perceptions of and behavioral intentions towards learning artificial intelligence in primary school students. Educational Technology & Society, 24(3), 89–101. https://www.jstor.org/stable/27032858
- Choi, S., Jang, Y., & Kim, H. (2022). Influence of pedagogical beliefs and perceived trust on teachers’ acceptance of educational Artificial Intelligence tools. International Journal of Human–Computer Interaction, 39(4), 910–922. https://doi.org/10.1080/10447318.2022.2049145
- Cudeck, R. (2000). Exploratory Factor analysis. Handbook of Applied Multivariate Statistics and Mathematical Modeling, 265–296. https://doi.org/10.1016/b978-012691360-6/50011-2
- Diwanji, P., Hinkelmann, K., & Witschel, H. (2018). Enhance classroom preparation for flipped classroom using A.I. and analytics. Proceedings of the 20th International Conference on Enterprise Information Systems. https://doi.org/10.5220/0006807604770483
- García, F. J. (2023). La percepción de la Inteligencia Artificial en contextos educativos tras el lanzamiento de ChatGPT: disrupción o pánico. Education in the Knowledge Society (EKS), 24, e31279. https://doi.org/10.14201/eks.31279
- García, V. R., Mora, A. B., & Ávila, J. A. (2020). La inteligencia artificial en la educación. Dominio de las Ciencias, 6(3), 648–666. https://dominiodelasciencias.com/ojs/index.php/es/article/view/1421
- Gill, S. S., Xu, M., Patros, P., Wu, H., Kaur, R., Kaur, K., Fuller, S., Singh, M., Arora, P., Parlikad, A. K., Stankovski, V., Abraham, A., Ghosh, S. K., Lutfiyya, H., Kanhere, S. S., Bahsoon, R., Rana, O., Dustdar, S., Sakellariou, R. & Buyya, R. (2024). Transformative effects of ChatGPT on modern education: Emerging era of A.I. Chatbots. Internet of Things and Cyber-Physical Systems, 4, 19–23. https://doi.org/10.1016/j.iotcps.2023.06.002
- Gonda, D. E., & Chu, B. (2019). Chatbot as a learning resource? Creating conversational bots as a supplement for teaching assistant training course. 2019 IEEE International Conference on Engineering, Technology and Education (TALE). https://doi.org/10.1109/tale48000.2019.9225974
- Hew, K. F., Huang, W., Du, J., & Jia, C. (2022). Using chatbots to support student goal setting and social presence in fully online activities: Learner engagement and perceptions. Journal of Computing in Higher Education, 35(1), 40–68. https://doi.org/10.1007/s12528-022-09338-x
- Hinojo-Lucena, F. J., Aznar-Díaz, I., Cáceres-Reche, M. P., & Romero-Rodríguez, J. M. (2019). Artificial Intelligence in higher education: A bibliometric study on its impact in the scientific literature. Education Sciences, 9(1), 51. https://doi.org/10.3390/educsci9010051
- Holmes, W., Bialik, M., & Fadel, C. (2023). Artificial intelligence in education. Data Ethics: Building Trust: How Digital Technologies Can Serve Humanity, 621–653. https://doi.org/10.58863/20.500.12424/4276068
- Javaid, M., Haleem, A., Singh, R. P., Khan, S., & Khan, I. H. (2023). Unlocking the opportunities through ChatGPT tool towards ameliorating the education system. BenchCouncil Transactions on Benchmarks, Standards and Evaluations, 3(2), 100115. https://doi.org/10.1016/j.tbench.2023.100115
- Kang, M., & Im, T. (2013). Factors of learner-instructor interaction which predict perceived learning outcomes in online learning environment. Journal of Computer Assisted Learning, 29(3), 292–301. https://doi.org/10.1111/jcal.12005
- Labrague, L. J., Aguilar-Rosales, R., Yboa, B. C., & Sabio, J. B. (2023). Factors influencing student nurses’ readiness to adopt artificial intelligence (A.I.) in their studies and their perceived barriers to accessing A.I. technology: A cross-sectional study. Nurse Education Today, 130, 105945. https://doi.org/10.1016/j.nedt.2023.105945
- Lee, A. V. Y. (2023). Supporting students’ generation of feedback in large-scale online course with artificial intelligence-enabled evaluation. Studies in Educational Evaluation, 77, 101250. https://doi.org/10.1016/j.stueduc.2023.101250
- León, G. D. L. C., & Viña, S. M. (2017). La inteligencia artificial en la educación superior. Oportunidades y amenazas. INNOVA Research Journal, 2(8.1), 412–422. https://doi.org/10.33890/innova.v2.n8.1.2017.399
- Liu, A., Bridgeman, D., & Miller, B. (2023). As uni goes back, here’s how teachers and students can use ChatGPT to save time and improve learning. The Conversation. https://theconversation.com/as-uni-goes-back-heres-how-teachers-and-students-can-use-chatgpt-to-save-time-and-improve-learning-199884
- Long, D., Blunt, T., & Magerko, B. (2021). Co-designing AI literacy exhibits for informal learning spaces. Proceedings of the ACM on Human-Computer Interaction, 5(CSCW2), 1–35. https://doi.org/10.1145/3476034
- Martin, F., & Bolliger, D. U. (2018). Engagement matters: Student perceptions on the importance of engagement strategies in the online learning environment. Online Learning, 22(1). https://doi.org/10.24059/olj.v22i1.1092
- Mayer, C. W. F., Ludwig, S., & Brandt, S. (2022). Prompt text classifications with transformer models! An exemplary introduction to prompt-based learning with large language models. Journal of Research on Technology in Education, 55(1), 125–141. https://doi.org/10.1080/15391523.2022.2142872
- Memarian, B., & Doleck, T. (2023). Fairness, Accountability, Transparency, and Ethics (FATE) in Artificial Intelligence (A.I.) and higher education: A systematic review. Computers and Education: Artificial Intelligence, 5, 100152. https://doi.org/10.1016/j.caeai.2023.100152
- Namatherdhala, B., Mazher, N., & Sriram, G. K. (2022). A comprehensive overview of conversational artificial intelligence trends. International Research Journal of Modernization in Engineering Technology and Science. https://doi.org/10.56726/irjmets30740
- Ocaña-Fernández, Y., Valenzuela-Fernández, L. A., & Garro-Aburto, L. L. (2019). Inteligencia artificial y sus implicaciones en la educación superior. Propósitos y Representaciones, 7(2). https://doi.org/10.20511/pyr2019.v7n2.274
- Ouyang, F., & Jiao, P. (2021). Artificial intelligence in education: The three paradigms. Computers and Education: Artificial Intelligence, 2, 100020. https://doi.org/10.1016/j.caeai.2021.100020
- Pavlik, J. V. (2023, January 7). Collaborating with ChatGPT: Considering the implications of generative Artificial Intelligence for journalism and media education. Journalism & Mass Communication Educator, 78(1), 84–93. https://doi.org/10.1177/10776958221149577
- Popenici, S. A. D., & Kerr, S. (2017). Exploring the impact of artificial intelligence on teaching and learning in higher education. Research and Practice in Technology Enhanced Learning, 12(1). https://doi.org/10.1186/s41039-017-0062-8
- Purdy, M. & Daugherty, P. (2016). Why artificial intelligence is the future of growth. Remarks at AI now: the social and economic implications of artificial intelligence technologies in the near term, 1–72. https://dl.icdst.org/pdfs/files2/2aea5d87070f0116f8aaa9f545530e47.pdf
- Rahaman, M. S., Ahsan, M. M. T., Anjum, N., Rahman, M. M., & Rahman, M. N. (2023). The A.I. Race is on! Google’s Bard and Openai’s chatgpt head to head: An opinion article. SSRN Electronic Journal. https://doi.org/10.2139/ssrn.4351785
- Rodway, P., & Schepman, A. (2023). The impact of adopting AI educational technologies on projected course satisfaction in university students. Computers and Education: Artificial Intelligence, 5, 100150. https://doi.org/10.1016/j.caeai.2023.100150
- Sabzalieva, E., & Valentini, A. (2023). ChatGPT and artificial intelligence in higher education: Quick start guide. UNESCO International Institute for Higher Education in Latin America and the Caribbean. https://unesdoc.unesco.org/ark:/48223/pf0000385146
- Seo, K., Tang, J., Roll, I., Fels, S., & Yoon, D. (2021). The impact of artificial intelligence on learner–instructor interaction in online learning. International Journal of Educational Technology in Higher Education, 18(1). https://doi.org/10.1186/s41239-021-00292-9
- Shubhendu, S., & Vijay, J. (2013). Applicability of artificial intelligence in different fields of life. International Journal of Scientific Engineering and Research, 1(1), 28–35. https://scholar.google.es/scholar?hl=es&as_sdt=0,5&q=Shubhendu,+S.,+%26+Vijay,+J.+(2013).+Applicability+of+Artificial+Intelligence
- Sullivan, M., Kelly, A., & McLaughlan, P. (2023, March 21). ChatGPT in higher education: Considerations for academic integrity and student learning. Journal of Applied Learning & Teaching, 6(1), 1–10. https://doi.org/10.37074/jalt.2023.6.1.17
- Su, J., Ng, D. T. K., & Chu, S. K. W. (2023). Artificial Intelligence (A.I.) literacy in early childhood education: The challenges and opportunities. Computers and Education: Artificial Intelligence, 4, 100124. https://doi.org/10.1016/j.caeai.2023.100124
- Ulate de Brooke, R. (2011). Enfoques en los modelos educativos, planes de estudio y su correspondencia con la planeación didáctica (diseño instruccional) en la educación a distancia. Focus on educational models, curriculum and teaching planning correspondence (instructional design). Revista Electrónica Calidad En La Educación Superior, 2(2), 168–192. https://doi.org/10.22458/caes.v2i2.428
- Vygotsky, L. S. (1978). Mind in society: Development of higher psychological processes. Harvard university press.
- Zhai, C., & Wibowo, S. (2023). A systematic review on artificial intelligence dialogue systems for enhancing English as foreign language students’ interactional competence in the university. Computers and Education: Artificial Intelligence, 4, 100134. https://doi.org/10.1016/j.caeai.2023.100134
- Zhao, J., & Li, Q. (2022). Big data–Artificial Intelligence fusion technology in education in the context of the new crown epidemic. Big Data, 10(3), 262–276. https://doi.org/10.1089/big.2021.0245