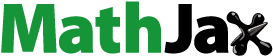
Abstract
Scientific research is vital for student’s education, fostering critical thinking, problem-solving skills, and deepening subject knowledge. To assess students’ attitudes towards research, the attitude towards research scale was developed (EACIN). This study addresses three gaps regarding this instrument: inconsistent latent structure, lack of comparison between available versions, and absence of gender invariance analysis given previously found gender differences. We analyzed data from 358 Chilean undergraduates, assessing the different version’s dimensionality, reliability. Results revealed inadequate fit indices for all EACIN available versions. Consequently, we proposed a new solution through exploratory structural equation modeling, EACIN-23 (or the Attitude toward Research Scale, ATRS-23), with a three-factor latent structure (replicating a previously found latent structure by the scale authors) and acceptable to good goodness-of-fit. The ATRS-23 demonstrated adequate to optimal factor loadings and good reliability. Moreover, it achieved scalar invariance across genders and demonstrated excellent criterion validity. This study provides a reliable and valid psychometric version of the EACIN, capable of accurately measuring and predicting attitudes towards research, including discriminating between students with and without intentions for postgraduate studies and research careers. Given that the ATRS-23 is only available in Spanish, we provide its English version. These findings have significant implications for undergraduate education and scientific research training.
PUBLIC INTEREST STATEMENT
In education, students’ outlook on research plays a big role in their experiences and career choices. Therefore, it’s important to have a reliable tool to measure these attitudes. The ATRS-23 scale, a modified version of the original, is a reliable and valid instrument that works equally well for men and women. Its previous versions did not match up well with the data. However, the ATRS-23 abides by high standards of scientific rigor, supporting well its theoretical model. The present study provides evidence its statistical properties and capacity to predict student’s career choices. Therefore, the ATRS-23 is a promising tool for researchers and educators to better assess and understand these attitudes not only in students but also in educators and administrative staff.
1. Introduction
Scientific research is a crucial component of undergraduate education, as it enables students to develop their analytical and critical thinking skills, problem-solving abilities, and gain a deeper understanding of their fields of study (Petrella & Jung, Citation2008). Some of the benefits of undergraduate research activities (e.g., development of thinking and communication skills; Bradforth et al., Citation2015; Lopatto, Citation2003) extend beyond the individual, as they also contributes to the advancement of knowledge in various academic fields (Kardash, Citation2000). Likewise, students who engage in research activities are more likely to pursue graduate studies and careers in research-related fields (Lopatto, Citation2006). Thus, understanding what students already know and any potential preconceived ideas they may have about scientific research is a crucial element of their education, with far-reaching implications.
One effective approach to gaining insight in this matter is by exploring their attitudes. These refer to the general evaluations or judgments that individuals hold about specific objects, people, or events (Wood, Citation2000). Attitudes play a significant role in shaping students’ behaviors, including their course selection, academic performance, and educational outcomes. A positive attitude towards learning has been linked to higher academic achievement, greater persistence, and motivation to learn (Chen et al., Citation2018; Gardner & MacIntyre, Citation1993), as well as to correlate with higher grades and better performance (Mao et al., Citation2021). Similarly, attitudes have shown to influence student’s performance (Else-Quest et al., Citation2013), as well as career choices (Curran & Rosen, Citation2006; Hofstein et al., Citation2010; Wiebe et al., Citation2018), potentially having long lasting repercussions from as early as primary school (Turner & Ireson, Citation2010).
Amongst the multitude of variables that modulate student’s attitudes towards scientific research and science, school and university students often report gender differences (Osborne et al., Citation2003; Sofiani et al., Citation2017), varying by country (Emily Oon & Subramaniam, Citation2023), field of scientific interest (Khan et al., Citation2022), etc. The magnitude of gender differences towards science have been so that Jones et al. (Citation2000) predicted that the gender landscape of STEM fields in the USA will remain the same despite 20 years of efforts to change that, a prediction that has largely been corroborated (National Science Foundation, Citation2014). Given that student’s (Cheng & Lo, Citation2022) and also teacher’s attitudes (Kollmayer et al., Citation2020) have shown to contribute to this reality, it becomes crucial to be able to accurately measure and compare attitudes within individuals and across parties.
As a way to objectively evaluate this phenomenon, Aldana et al. (Citation2016) developed the attitude towards research scale (EACIN). Its initial latent model is based on McGuire three-factor model of attitudes (McGuire, Citation1969): affective (i.e., what the subject feels or the emotions that research produces in the subject), cognitive (i.e., what the subject knows or thinks they know about research), and behavioral (i.e., what the subject does or is willing to do with respect to research). Originally composed of 34 items measured through a five-points Likert scale, the scaled has been revised into two new versions, one with 32 (Quezada-Berumen et al., Citation2019) with a three-factorial latent structure (i.e., affective-behavior, cognitive, and behavioral-affective factors), and another with 28 (EACIN-R; Aldana et al., Citation2020) with a different three-factorial latent structure (i.e., disinterest in research, vocation for research, and research valuation). Studies evaluating the EACIN/EACIN-R psychometric properties have shown mix results in terms of their goodness of fit and explanatory power, yet only the EACIN-R latent structure has been replicated with good fit indicators, and by different groups of researchers (Aldana et al., Citation2020; Hidalgo et al., Citation2023). The reason as to why the latent structure varies across studies could be methodological (e.g., differences in the study samples, design, techniques, etc.), theoretical (e.g., different emphasis of the underlying theory), or likely both (e.g., different samples leading to different factor loadings, and ultimately leading to a different interpretation of the latent construct).
There is, to our knowledge, five other alternatives to this scale that measure the same or a similar construct. The attitude towards research (ATR; Papanastasiou, Citation2005) is perhaps the better known of all. It is a self-report tool measuring students’ attitudes towards scientific research as a field, regardless of their orientation (i.e., quantitative, qualitative, or mixed methods). Moreover, Castro Molinares (Citation2017) developed a scale to measure attitude towards formative research in university students. The scale is composed by 26 items measured though a Likert scale, with a five-factor structure explaining 56% of the variance, and with good overall reliability (Cronbach’s alpha = .827). Likewise, Ozturk (Citation2011) developed a similar scale to measure attitudes towards education research, with an eight-factor structure measured through 29-Likert items. Similarly, Muthuswamy et al. (Citation2017) proposed also a scale measuring attitudes towards research, with a six-factor structure, measured through 28-Likert items. Finally, Muñoz et al. (Citation2020) developed a scale to measure attitude towards research in university students. The scale is composed by 17 items measured through a Likert scale, with a three-factor structure, and alpha coefficients ranging between .700–.901. Neither of these scales, except the ATR, have been replicated by other psychometric and independent studies. While there are also other scales that also assess student’s perceptions of scientific research (e.g., Gregory, Citation2010; Kirk, Citation1981), they are a bit more specific to other contexts and phenomena.
1.1. Statement of the problem
The study of student’s attitudes towards scientific research provides valuable insight of its impact in student’s skill development, performance, career choices, etc. While there are alternatives as to how to measure this phenomenon, these are unable to compare student’s attitude with other relevant roles such as teachers and academic managers. However, the EACIN-R stands out due to its versatility by being able to compare across these educational roles. Yet, the inconsistency of its theoretical structure and capability to meaningfully compare between men and women remain unexplored. Namely, 1) the internal latent structure of the EACIN/EACIN-R is not consistent throughout studies and lacks an explanation as to what its dimensions represent; 2) there has not been a comparison of the original and revised versions to evaluate which one offers a better fit to the data; and 3) neither of the previous studies provides an invariance analysis of the instrument for any variable. Therefore, the following study evaluated the internal structure of the scale by comparing its different versions, so as to ascertain which factorial structure and item structure version provided a better fit to the data, as well as to offer a gender invariance analysis.
2. Methods
2.1. Design
The study used a cross-sectional design, using both the EACIN and EACIN-R scales by including all 34 original items, yet analysing all versions separately. We chose not to include #35 from Quezada et al. study (Quezada-Berumen et al., Citation2019), yet still assessed its 31-items version so as to evaluate how the original EACIN items would adjust without item #35. We assessed the scales psychometric properties to provide a definitive version of it. This study was pre-registered before the data was analyzed in the open science framework (https://osf.io/xtdyn/), which did not include modifications made after the peer-review process.
2.2. Participants
The sample was originally comprised of 408 Chilean undergraduate students of Psychology from different Universities from Iquique and Arica, from all year classes. The study used a non-probabilistic convenience sample of ≥18 years old participants. After removing participants who did not provide consent to participate, and repeated responses, the final database was comprised of 358 people. Participant’s descriptive statistics are presented in Table . Individuals whose gender responses were “non-binary” or “others” were not included in the invariance and validity analyses given their small sample size.
Table 1. Sample descriptive statistics
2.3. Procedure
The project was conducted online between June 2022 and March 2023. Our study was evaluated and authorized by the University of Tarapacá’s Ethic Committee (N°35/2022). Participants who responded consented to participate completed an anonymous self-report survey on an online Google form platform that lasted approximately 15–20 min.
2.4. Instruments
The project took into account the following demographic variables characteristics related to career choices: gender, have they performed as a teaching assistant, intention to go into graduate school, intention to work as a researcher.
The Attitudes towards Research (EACIN) is a scale developed by Aldana de Becerra and collaborators (Aldana de Becerra & Joya Ramírez, Citation2011; Aldana et al., Citation2016, Citation2020). Initially, the original scale was based on a multidimensional model and is composed of 34 items answered Likert style, distributed in three dimensions: affective, cognitive, and behavioral. Furthermore, Quezada et al. proposed a similar structure blending some aspects of the original dimensions into each other (Quezada-Berumen et al., Citation2019). Subsequently, Hidalgo et al. elaborated the revised version, EACIN-R, with the following subscales: (a) disinterest in research; (b) vocation for research, and (c) valuing research (Hidalgo et al., Citation2023). Since we aimed to determine the latent factorial structure of the EACIN, we tested all three versions of it to evaluate which latent factorial structure would provide a better fit for the data.
2.5. Statistical analyses
The statistical plan as per pre-registered was composed of the following steps: data imputation, descriptive analysis, test of dimensionality, test of reliability, measurement invariance, and criterion validity test.
We planned to analyse missing values in the database to identify the type of missing data pattern. However, contrary to what was expected in the pre-registration, we had no missing data, hence imputation was unnecessary. Thus, we first used demographic statistics of central tendency and dispersion to describe the sample, items, and dimensions for all EACIN scale versions (i.e., M, SD, n, %, Skewness and Kurtosis, see Tables ).
Table 2. Descriptive statistics for all EACIN items
In order to confirm the internal structural validity of the model, due to the ordinal nature of the EACIN items, and the lack of multivariate normality in the data detected through the Mardia’s test (Mardia, Citation1970) (bSkew = 211.425; bKurt = 1395.329; ps < .001), the Weighted Least Squares Means- and Variance-Adjusted (WLSMV) was used as the estimation method for model and invariance testing (Barrett et al., Citation2008; Browne & Cudeck, Citation1992; Finney & DiStefano, Citation2013; Kline, Citation2015).
The tested models were evaluated through commonly used goodness of fit indices (Marsh & Hau, Citation2005; Schermelleh-Engel et al., Citation2003): Comparative Fit Index (CFI ≥ .90 adequate; ≥ .95 good), Tucker—Lewis index (TLI ≥ .90 adequate; ≥ .95 good), and Root-Mean-Square Error of Approximation with its 90% confidence interval (RMSEA ≤ .08 adequate, ≤ .05 good) and SRMR < .08 good (Schreiber, Citation2017). Regarding factor loadings, we will use the criteria proposed by Jöreskog & Sörbom (Jöreskog & Sörbom, Citation1993), optimal (λ > .7) and adequate (.7 < λ > .3). Furthermore, the criteria of strong (r > .7) and moderate (.7 < r > 0.3) correlations were used for correlations between dimensions (Cohen, Citation1992). Furthermore, to assess the scale’s criterion validity, we used student’s career plans (see Table ) as a way to evaluate the scale’s capability to discriminate high and low research attitudes, which have been previously found to correlate with career choices related to science (Balta et al., Citation2023; Sellami et al., Citation2023; Wiebe et al., Citation2018). Finally, we performed a discriminant validity assessment invariance-based SEM test using the Heterotrait-monotrait (HTMT) ratio of correlations in order to assess the distinctiveness of the factors, while also prevent multicollinearity issues (Hamid et al., Citation2017; Henseler et al., Citation2015).Footnote1 The HTMT value is contrasted with a predefined threshold value, typically established at 0.85 (Kline, Citation2022). If the computed HTMT ratio falls below this threshold, it indicates that the two constructs are separate and exhibit satisfactory discriminant validity.
We reported reliability for each dimension and whole scale using Cronbach’s alpha coefficient. We also used McDonald’s hierarchical omega in order to report a more efficient reliability criteria (Goodboy & Martin, Citation2020; Hayes & Coutts, Citation2020; McNeish, Citation2018). Acceptable levels of reliability has been established to be ≥ .7; however, this criterion is a guide, more than an empirical one (Cho & Kim, Citation2015).
Finally, given the often reported gender differences/disparities in attitudes towards scientific research (e.g., Osborne et al., Citation2003), and therefore to assess whether the EACIN-R can be interpreted in the same way across gender, we performed tests of measurement invariance (Vandenberg & Lance, Citation2000). We tested three levels of invariance: configural (i.e., same structure), metric (i.e., same factor loadings), scalar (i.e., same item intercepts). Finally, to establish the evidence of criterion validity with other variables, we performed one-way ANOVA analyses and independent sample Student t-test’s using career choices as variables of interest. All results were conducted through Mplus 7.0 and SPSS 22.
3. Results
3.1. Study 1 - EACIN previous versions
3.1.1. Test of dimensionality
Standardized factor loadings and reliabilities of both three-factors, 34-items and 28-items, models CFA can be seen in Table . The standardized factor loadings for the 34-items version ranged .101–.841 (ps < .001, except for item 28, p = .07), whereas the inter-factor correlations were positive and moderate (r = .556–.606; p < .01). Meanwhile, standardized factor loadings for the 28-items version ranged .381–.804 (ps < .001), whereas the inter-factor correlation were positive and negative, while also weak-to-moderate (r = .201–.691; p < .001). Moreover, both models yielded overall and dimensional acceptable-to-good levels of reliability.
Table 3. Previous EACIN version’s factor loadings and reliability
All versions yielded unacceptable fit indices to the data (34-items: WLSMV χ2 (524) = 2,107.935, p < .001, CFI = .798, TLI = 0.784, RMSEA = .092, SRMR = .091 [90% CI = .088–.096]; 31-items: WLSMV χ2 (431) = 1226.803, p < .001, CFI = .895, TLI = .887, RMSEA = .072, SRMR = .067 [90% CI = .067–.077]; 28-items: WLSMV χ2 (374) = 1183.135, p < .001, CFI = .877, TLI = .865, RMSEA = .082, SRMR = .07 [90% CI = .077–.087]; see Table ).
Table 4. Fit indices for all attitude towards research scale versions (EACIN, EACIN-R, and EACIN-23)
In light of the current findings, and while it was not part of the pre-registration, we explored a new factorial solution through structural equation modeling using the same estimator and analyses to evaluate its goodness of fit.
3.2. Study 2 - EACIN-23: The Attitude Towards Research Scale (ATRS-23)
We first evaluated the face validity of all 34 items, ultimately keeping all of them. Subsequently, we conducted exploratory structural equation modeling (eSEM) analyses on different iterations of the scale until we found a suitable version of it, by eliminating items step-by-step following three established criteria: a) redundancy of items (Abad et al., Citation2011); b) high cross-loadings ≥ .3 (Muthén & Asparouhov, Citation2012; Xiao et al., Citation2019); and c) low factor loadings (λ < .3). Based on these criteria, the scale was iteratively reduced until a solution of 23 items was reached (see Supplementary Table S1). Furthermore, given the lack of good fit to the data of previous models, we conducted measurement invariance only on the EACIN-23 (henceforth the Attitude Towards Research Scale [ATRS-23]) so as to ascertain potential structural differences in this version of the scale for gender (van de Schoot et al., Citation2012; Vandenberg & Lance, Citation2000). Finally, we conducted parametric comparisons evaluating the ATRS-23 association with theoretically relevant correlates to examine its criterion validity. We used independent sample t-test or one-way ANOVA’s (using the Bonferroni correction) to determine if the scale was able to differentiate amongst different alternatives to the sociodemographic questions related to research attitude in our study.
3.2.1. Test of dimensionality
The new revised version was ultimately composed of 23 items, and yielded a three-factor structure, keeping the same interpretation as the EACIN-R (Aldana et al., Citation2020; Hidalgo et al., Citation2023). This version yielded adequate to good goodness-of-fit (WLSMV χ2 (227) = 510.395, p < .001; CFI = .944, TLI = .938, RMSEA = .06; SRMR = .052 [90% CI = .053–.067]). Standardized factor loadings for this version ranged between adequate to optimal (λ = .409–.912), whereas the inter-factor correlation were positive, ranging from weak to moderate (r = −.17–.58). Reliability for the total scale and dimensions ranged from acceptable to good (see Table ).
Table 5. ATRS-23 factor’s loadings and reliability
Table shows the Heterotrait-Monotrait (HTMT) criterion assessing the discriminant validity of the ATRS-23 factors amongst themselves. Results show that all factors exhibit a good level of discriminant validity given that all scores fell below the established criterion (i.e., < .85).
Table 6. ATRS-23 factor’s discriminant validity through the HTMT criterion
3.3. Measurement invariance
Fit indices for the three-factor model are shown in Table for both groups examined. All factor loadings were statistically significant and similar between the groups (ps < .001). The configural model showed acceptable fit indices (CFI = .934; TLI = .927; RMSEA = .067 [90% CI = .059–.074]), whereas the lack of substantive differences to the fit detriment after imposing constraints (ΔCFIs = −.002; ΔTLIs = .006; ΔRMSEA = −.003; see Table ) indicate the ATRS-23 reached scalar level of invariance, suggesting that all parameters have an equivalent same latent structure, factor loadings, and intercepts, regardless if participants were men or women.
Table 7. Measurement invariance by gender for the ATRS-23
3.4. Validity test
The analyses demonstrated that students who have or currently perform as a teaching assistant scored significantly higher in “Vocation” and the Total score (ps < .004). Furthermore, students who intend to pursue postgraduate studies scored significantly higher in “Vocation” and the Total score, and significantly lower than those who do not plan to do so in “Disinterest” (ps < .001). Finally, students who plan to dedicate to do research for living scored significantly higher in all dimensions and the Total score than those who do not (ps < .001), whereas those who were “not sure” also scored significantly higher in “Vocation” and the Total score than those who intend not to do so (ps < .001; see Table ).
Table 8. Criterion validity analysis of the ATRS-23
4. Discussion
Attitudes towards research is a pivotal component of higher education, common to all scientific programs, able to modulate, and to some extend determine, students’ experiences, performance, and career decisions. Consequently, having a reliable and valid psychometric instrument capable of accurately measuring and predicting such attitudes becomes immensely valuable. Thus, our findings suggest that the EACIN-23/ATRS-23 scale is a reliable, valid, and gender-invariant version of the original scale, capable of distinguishing between students with and without intentions to seek-out postgraduate studies and to dedicate themselves to research for living.
After thorough examination of the psychometric properties of the ATRS-23 in a sample of university students, our study demonstrated that: 1) previous versions of the EACIN scale did not provide a good fit for the data, rendering them problematic; 2) the new version of the scale, ATRS-23, supported the three-factor structure of the EACIN-R (Aldana et al., Citation2020); 3) the new version possessed acceptable-to-good fit indices, acceptable-to-good reliability indices for its dimensions and whole scale, and good discriminant validity amongst its latent structure sub-dimensions; 4) the ATRS-23 is a gender-invariant tool capable of comparing results between men and women, and finally 5) the ATRS-23 provides a valid distinction between students with different attitudes towards research career choices.
Previous studies assessing the EACIN psychometric properties have shown mixed results regarding its factorial structure and goodness of fit, with three different factorial solutions offered. The first factorial solution (Aldana et al., Citation2016) proposed three dimensions resembling a basic theoretical attitude structure (McGuire, Citation1969). However, the authors did not perform any statistical analysis beyond calculating Cronbach’s alpha. The following study did perform a psychometric validation using an exploratory factor analysis (EFA), partially corroborating this three-factorial solution, with a good fit for the data (Quezada-Berumen et al., Citation2019). The authors proposed the following interpretation of their dimensions: affective-behavioral, behavioral-affective, and cognitive. These combine items from the three original factorial structure proposed. Finally, they deleted two items based on low communality, factor loading, inter-correlation, and reliability, while also added one. Meanwhile, the newer three-factor solution, EACIN-R (Aldana et al., Citation2020) conducted an EFA, using Horn’s parallel analysis (Garrido et al., Citation2013; Horn, Citation1965). Horn’s parallel analysis is a reliable technique to identify the number of underlying factors, yet it has been noted that the analysis performs best when factors are either minimally or uncorrelated (Crawford et al., Citation2010; Debelak et al., Citation2016), which has not been the case in any previous psychometric validation of the EACIN. Still, their factorial solution has been the only one replicated (Hidalgo et al., Citation2023; Maguiña Custodio, Citation2021), including the present study. They removed six items based solely on low reliability (i.e., < .30). However, neither of these studies provided an interpretation of their factorial latent structure, or compared the factorial solutions to evaluate which one provided a better fit to the data, or why. Thus, since the sample characteristics and statistical techniques used are the two main differences across validations, while using with robust psychometric techniques within the same sample, the present study demonstrated that all previous versions of the scale did not provide a good and consistent fit to the data, whereas our new solution, ATRS-23, did.
Our study provides further evidence to the three-factor solution proposed by Hidalgo et al (Hidalgo et al., Citation2023). as a better representation of the latent construct measured by the EACIN-R. After an initial assessment, we took all items representing these dimensions and asked Chat GPT to return the “common characteristic underlying all items”, one dimensions at the time. The software explained that the “Vocation” dimension items represent “an inclination or passion for research and scientific exploration”. Meanwhile, the “Valuation” dimension items represent “the recognition and belief in the importance and value of research”. Finally, the “Disinterest” dimension items represent “the lack of engagement or disconnection with current or ongoing activities and information”. We believe that its analysis is indeed a valid rendering of the latent factorial structure, which also replicates with the previous one offered by the scale authors (Hernández et al., Citation2017). Furthermore, the HTMT criterion provides further proof of the suitability of the factorial structure proposed in the ATRS-23 by virtue of the discriminant validity scores amongst its latent factors.
The ATRS-23 is a new, parsimonious proposal to the previous EACIN 34-, 32-, and 28-items versions, while replicating the scale author’s most recent latent factorial structure. Whereas the overall reliability of the ATRS-23 was not as good as in its previous versions, it is worth noticing that this version has 11 items less than the original, particularly leaving only five items in the “disinterest for research” dimension. Whereas it may be tempting to offer a low number of items as an explanation for the lower reliability, and to some extend there is true to that given due to more opportunities for inter-item correlations (Cortina, Citation1993), it has been shown that Conbach’s α can inflate reliability estimation (Kopalle & Lehmann, Citation1997) or even become a misleading estimator of it (Raykov, Citation2007, Citation2008), especially when its assumption are not taken into account (Cho & Kim, Citation2015). However, following McDonald’s ω, we do bring attention to this dimension reliability—potentially in need of a more in-depth theoretically-driven analysis to improve its reliability, not only necessarily because the reliability falling below .7, but mostly since this new version is yet to be replicated. This is because despite that .7 or .8 are often cited as the acceptable levels of reliability (Nunnally & Bernstein, Citation1994), these are not empirically driven, but the author’s intuition, and that McDonald’s ω is not the only alternative to Conbach’s α (Cho & Kim, Citation2015). Still, the ATRS-23 yielded the best fit for the data, good overall reliability, and excellent criterion validity able to distinguish across different levels of interest regarding student’s intentions of specialization and pursuing a career in research. Furthermore, the ATRS-23 is the only peer-review article demonstrating this scale is invariant when it comes to comparing men and women. There is, though, only one record—a non-published undergraduate thesis—that showed the EACIN-R has a similar goodness of fit to the ATRS-23, and invariant regarding gender (Maguiña Custodio, Citation2021).
The results of the present study also demonstrated that the latent factorial structure of the ATRS-23 is able to distinguish between students intentions related to research. On the one hand, the dimensions “Research Vocation” and “Research Disinterest” are able to distinguish significantly amongst students who have performed as teaching assistants or not, as well as amongst those who are sure they intend to pursue postgraduate studies, and plan to dedicate themselves to research for living than those who are not sure or will not. Nevertheless, “Research Vocation” yielded a much stronger effect size than any other ATRS-23 dimension, including the total score. On the other hand, the dimension “Research Valuation” was only able to distinguish between those who plan to dedicate themselves to research for living from those who will not, yet still with a small effect size than other comparisons. This does not mean this dimension has no discriminant value. In fact, Bolin et al. (Citation2012) demonstrated the perception of research utility/value was significantly related to a decreased research anxiety, much like Gredig and Bartelsen-Raemy (Citation2018) demonstrated that interest in research courses was predicted by research anxiety, the perceived value, the attributed usefulness, and the perceived unbiased nature of research. This could explain, in part, why this dimension was not able to distinguish between those who answered “yes” or “not sure” to the sociodemographic questions, assuming the latter may experience some degree of stressed or anxiety related to answering the question.
Amongst the other psychometric alternatives to measure attitudes towards research, Papanastasiou’ scale stands out, offering a different theoretical model than the EACIN’s (Papanastasiou, Citation2005). The original self-report scale consisted of 32 items, answered through a seven-Likert scale, from strongly disagree to strongly agree. Its initial exploratory analysis of its structure proposed a five-factor solution: “Research usefulness” (α = .919), “Research anxiety” (α = .918), “Positive research predisposition” (α = .929), “Relevance to life” (α = .767), and “Research difficulty” (α = .711). Subsequently, the authors suggested to drop two items (i.e., #6, #11) (Papanastasiou & Schumacker, Citation2014). However, a more recent confirmatory factor analysis proposed a three-factor solution (Research usefulness, Research anxiety, Positive research predisposition) only using 13 items, demonstrating good indicators of fit than the original model (Papanastasiou, Citation2014). Other studies using the ATR scale have replicated the latter factorial solution with good reliability levels (Howard & Michael, Citation2019; van der Westhuizen, Citation2015). More than suggesting which scale is better, these authors believe they serve similar purposes, and researchers and educators are provided with two instruments that measure the same construct with two different approaches, allowing them to choose which approach is best for their purposes. For instance, Sizemore & Lewandowski compared sophomore-level student’s knowledge and attitudes towards research and statistics at the beginning and end of the semester. They found that the course did improved their knowledge, yet their attitudes towards the subject declined significantly (Sizemore & Lewandowski, Citation2009). Thus, by using the ATR scale researchers would be able to assess how anxiety towards research and their predisposition may modulate these findings, whereas by using the ATRS-23 researchers would be able to ascertain if these results are based on a lack of interest or vocation for scientific research, meanwhile using both scales could represent an overall criterion validity to each other.
Since the ATRS-23 is only available in Spanish, we provide researchers an English version translated following Beaton’s guidelines for cross-cultural adaptations of self-report measures (Beaton et al., Citation2000). This offered English version was translated from Spanish to English by two experienced translators. Any discrepancy between the two versions was addressed, to come up with one revised English version, which was later on translated by a third translator into Spanish to be compared with the original (see Supplementary Table S2). The authors hope the evidence presented and this translated version of the ATRS-23 encourages others to conduct validations in different contexts, with samples of different programs, in different educational roles, in other cultures/countries and languages.
4.1. Limitations
The current study is offers a new, simpler, version of the EACIN scale, yet not without limitations. Our main caveats has to do with the diversity of the sample. We included only undergraduate university students of one program. Future validation studies should include not only students of different programs, but also from different levels (undergraduate and postgraduate), and people in other university roles, as the EACIN is capable to do so. An ideal validation would include a sample big in every role to also provide invariance analysis amongst these groups. Moreover, the provided criterion validity was performed only through sociodemographic questions. Future studies are encouraged to utilize other validated psychometric scales of constructs of interest theoretically relevant to research attitudes, such as anxiety (Kracker, Citation2002; Yau & Leung, Citation2018), self-efficacy (Wajid & Jami, Citation2020; Yau & Leung, Citation2018), student performance (Mao et al., Citation2021), etc.
Supplemental Material
Download MS Word (17.9 KB)Acknowledgments
The authors would like to thank José Piña for his contribution in the data collection process.
Disclosure statement
No potential conflict of interest was reported by the author(s).
Data availability statement
The study data was made available in the following link https://osf.io/r645c/
Supplementary material
Supplemental data for this article can be accessed online at https://doi.org/10.1080/2331186X.2023.2292825
Additional information
Funding
Notes
1. See the author’s website for a calculator of the HTMT criterion.
References
- Abad, F. J., Olea, J., Ponsoda, V., & García, C. (2011). Medición en ciencias sociales y de la salud. Síntesis. https://dialnet.unirioja.es/servlet/libro?codigo=552272
- Aldana, G., Babativa Novoa, D., & Caraballo, G. (2016). Escala para medir actitudes hacia la investigación (eacin): validación de contenido y confiabilidad. Aletheia Revista de Desarrollo Humano, Educativo y Social Contemporáneo, 8(2), 104–20. https://doi.org/10.11600/21450366.8.2aletheia.104.121
- Aldana, G., Babativa Novoa, D., Caraballo, G., & Rey, C. (2020). Escala de actitudes hacia la investigación (EACIN): Evaluación de sus propiedades psicométricas en una muestra colombiana. CES Psicología, 13(1), 89–103. https://doi.org/10.21615/cesp.13.1.6
- Aldana de Becerra, G. M., & Joya Ramírez, N. S. (2011). Actitudes Hacia la Investigación Científica en Docentes de Metodología de la Investigación. Tabula Rasa, 14(14), 295–309. https://doi.org/10.25058/20112742.428
- Balta, N., Japashov, N., Karimova, A., Agaidarova, S., Abisheva, S., & Potvin, P. (2023). Middle and high school girls’ attitude to science, technology, engineering, and mathematics career interest across grade levels and school types. Frontiers in Education, 8, 1013. https://doi.org/10.3389/feduc.2023.1158041
- Barrett, B., Brown, R., & Mundt, M. (2008). Comparison of anchor-based and distributional approaches in estimating important difference in common cold. Quality of Life Research, 17(1), 75–85. https://doi.org/10.1007/s11136-007-9277-2
- Beaton, D. E., Bombardier, C., Guillemin, F., & Ferraz, M. B. (2000). Guidelines for the process of cross-cultural adaptation of self-report measures. Spine (Phila Pa 1976), 25(24), 3186–3191. https://doi.org/10.1097/00007632-200012150-00014
- Bolin, B., Lee, K., Glenmaye, L., & Yoon, D. (2012). Impact of research orientation on attitudes toward research of Social work students. Journal of Social Work Education, 48(2), 223–243. https://doi.org/10.5175/JSWE.2012.200900120
- Bradforth, S. E., Miller, E. R., Dichtel, W. R., Leibovich, A. K., Feig, A. L., Martin, J. D., Bjorkman, K. S., Schultz, Z. D., & Smith, T. L. (2015). University learning: Improve undergraduate science education. Nature, 523(7560), 282–284. https://doi.org/10.1038/523282a
- Browne, M. W., & Cudeck, R. (1992). Alternative ways of assessing model fit. Sociological Methods & Research, 21(2), 230–258. https://doi.org/10.1177/0049124192021002005
- Castro Molinares, S. P. (2017). Diseño y validación de un instrumento para evaluar la actitud hacia la investigación formativa en estudiantes universitarios. Actualidades Pedagógicas, 1(70), 165–182. https://doi.org/10.19052/ap.3996
- Chen, L., Bae, S. R., Battista, C., Qin, S., Chen, T., Evans, T. M., & Menon, V. (2018). Positive attitude toward math supports early academic success: Behavioral evidence and neurocognitive mechanisms. Psychological Science, 29(3), 390–402. https://doi.org/10.1177/0956797617735528
- Cheng, M. F., & Lo, Y. H. (2022). Innovative STEM curriculum to enhance students’ engineering design skills and attitudes towards STEM. Concepts and Practices of STEM Education in Asia, 117–137. https://doi.org/10.1007/978-981-19-2596-2_7
- Cho, E., & Kim, S. (2015). Cronbach’s coefficient alpha: Well known but poorly understood. Organizational Research Methods, 18(2), 207–230. https://doi.org/10.1177/1094428114555994
- Cohen, J. (1992). A power primer. Psychological Bulletin, 112(1), 155–159. https://doi.org/10.1037/0033-2909.112.1.155
- Cortina, J. M. (1993). What is coefficient alpha? An examination of theory and applications. Journal of Applied Psychology, 78(1), 98–104. https://doi.org/10.1037/0021-9010.78.1.98
- Crawford, A. V., Green, S. B., Levy, R., Lo, W.-J., Scott, L., Svetina, D., & Thompson, M. S. (2010). Evaluation of parallel analysis Methods for determining the number of factors. Educational and Psychological Measurement, 70(6), 885–901. https://doi.org/10.1177/0013164410379332
- Curran, J., & Rosen, D. (2006). Student attitudes toward college courses: An examination of influences and intentions. Journal of Marketing Education, 28(2), 135–148. https://doi.org/10.1177/0273475306288401
- Debelak, R., Tran, U. S., & Ebrahimi, M. (2016). Comparing the effects of different smoothing algorithms on the assessment of dimensionality of ordered categorical items with parallel analysis. PLOS ONE, 11(2), e0148143. https://doi.org/10.1371/journal.pone.0148143
- Else-Quest, N. M., Mineo, C. C., & Higgins, A. (2013). Math and Science attitudes and achievement at the intersection of gender and ethnicity. Psychology of Women Quarterly, 37(3), 293–309. https://doi.org/10.1177/0361684313480694
- Emily Oon, P. T., & Subramaniam, R. (2023). Gender differences in attitudes towards science: Comparison of middle school students in England, Singapore and USA using complete TIMSS 2011 data. Research in Science & Technological Education, 1–20. https://doi.org/10.1080/02635143.2023.2177267
- Finney, S. J., & DiStefano, C. (2013). Nonnormal and categorical data in structural equation modeling. In G. R. Hancock & R. O. Mueller (Eds.), Structural equation modeling: A second course (2nd ed., pp. 439–492). IAP Information Age Publishing.
- Gardner, R. C., & MacIntyre, P. D. (1993). On the measurement of affective variables in second language learning. Language Learning, 43(2), 157–194. https://doi.org/10.1111/j.1467-1770.1992.tb00714.x
- Garrido, L. E., Abad, F. J., & Ponsoda, V. (2013). A new look at Horn’s parallel analysis with ordinal variables. Psychological Methods, 18(4), 454–474. https://doi.org/10.1037/a0030005
- Goodboy, A. K., & Martin, M. M. (2020). Omega over alpha for reliability estimation of unidimensional communication measures. Annals of the International Communication Association, 44(4), 422–439. https://doi.org/10.1080/23808985.2020.1846135
- Gredig, D., & Bartelsen-Raemy, A. (2018). Exploring social work students’ attitudes toward research courses: Predictors of interest in research-related courses among first year students enrolled in a bachelor’s programme in Switzerland. Social Work Education, 37(2), 190–208. https://doi.org/10.1080/02615479.2017.1389880
- Gregory, V. L. (2010). Gregory research beliefs scale: Factor structure and internal consistency. Research on Social Work Practice, 20(6), 641–650. https://doi.org/10.1177/1049731509353049
- Hamid, M., Sami, W., & Sidek, M. (2017). Discriminant validity assessment: Use of Fornell & Larcker criterion versus HTMT criterion. Journal of Physics Conference Series, 890, 012163. https://doi.org/10.1088/1742-6596/890/1/012163
- Hayes, A. F., & Coutts, J. J. (2020). Use omega rather than Cronbach’s alpha for estimating reliability. But …. Communication Methods and Measures, 14(1), 1–24. https://doi.org/10.1080/19312458.2020.1718629
- Henseler, J., Ringle, C. M., & Sarstedt, M. (2015). A new criterion for assessing discriminant validity in variance-based structural equation modeling. Journal of the Academy of Marketing Science, 43(1), 115–135. https://doi.org/10.1007/s11747-014-0403-8
- Hernández, R., Thieme, T., & Araos, F. (2017). Adaptación y Análisis Psicométrico de la Versión Española del Índice Internacional de Función Eréctil (IIEF) en Población Chilena. Terapia Psicológica, 35(3), 223–230. https://doi.org/10.4067/S0718-48082017000300223
- Hidalgo, J., Aldana, G., León, P., & Ucedo, V. (2023). Escala de actitudes hacia la investigación (EACIN-R): propiedades psicométricas en universitarios peruanos. Propósitos y Representaciones, 11(1), e1699. https://doi.org/10.20511/pyr2023.v11n1.1699
- Hofstein, A., Maoz, N., & Rishpon, M. (2010). Attitudes towards school Science: A comparison of participants and nonparticipants in extracurricular Science activities. School Science and Mathematics, 90(1), 13–22. https://doi.org/10.1111/j.1949-8594.1990.tb11988.x
- Horn, J. L. (1965). A rationale and test for the number of factors in factor analysis. Psychometrika, 30(2), 179–185. https://doi.org/10.1007/BF02289447
- Howard, A., & Michael, P. G. (2019). Psychometric properties and factor structure of the attitudes toward research scale in a graduate student sample. Psychology Learning & Teaching, 18(3), 259–274. https://doi.org/10.1177/1475725719842695
- Jones, M. G., Howe, A., & Rua, M. J. (2000). Gender differences in students’ experiences, interests, and attitudes toward science and scientists. Science Education, 84(2), 180–192. https://doi.org/10.1002/(SICI)1098-237X(200003)84:2<180:AID-SCE3>3.0.CO;2-X
- Jöreskog, K., & Sörbom, D. (1993). LISREL 8: Structural equation modeling with the SIMPLIS command language.
- Kardash, C. M. (2000). Evaluation of undergraduate research experience: Perceptions of undergraduate interns and their faculty mentors. Journal of Educational Psychology, 92(1), 191–201. https://doi.org/10.1037/0022-0663.92.1.191
- Khan, M., Abid, M., & Malone, K. L. (2022). Scientific attitudes: Gender differences, impact on physics scores and choices to study physics at higher levels among pre-college STEM students. International Journal of Science Education, 44(11), 1816–1839. https://doi.org/10.1080/09500693.2022.2097331
- Kirk, S. A. R. A. (1981). Research knowledge and orientation amongst social work students. In S. W. Briar & A. H. Rubin (Eds.), Research utilization in social work education (pp. 29–39). Council on Social Work Education.
- Kline, R. (2015). Principles and practice of structural equation modeling (4th ed.). The Guilford Press.
- Kline, R. (2022). Principles and practice of structural equation modeling.
- Kollmayer, M., Schultes, M. T., Lüftenegger, M., Finsterwald, M., Spiel, C., & Schober, B. (2020). REFLECT – a Teacher training program to promote gender equality in schools [article]. Frontiers in Education, 5. Article 136. https://doi.org/10.3389/feduc.2020.00136
- Kopalle, P. K., & Lehmann, D. R. (1997). Alpha inflation? The impact of eliminating scale items on Cronbach’s alpha. Organizational Behavior and Human Decision Processes, 70(3), 189–197. https://doi.org/10.1006/obhd.1997.2702
- Kracker, J. (2002). Research anxiety and students’ perceptions of research: An experiment. Part I. Effect of teaching Kuhlthau’s ISP model. Journal of the American Society for Information Science and Technology, 53(4), 282–294. https://doi.org/10.1002/asi.10040
- Lopatto, D. (2003). The essential features of undergraduate research. Council on Undergraduate Research Quarterly, 139. https://www.cur.org/assets/1/7/mar03toc.pdf
- Lopatto, D. (2006). Undergraduate research as a catalyst for liberal learning. Peer Review, 8, 22–25. https://www.proquest.com/docview/216599120?sourcetype=Scholarly%20Journals
- Maguiña Custodio, L. M. J. (2021). Escala de Actitudes hacia la Investigación-versión Revisada (EACIN-R): propiedades psicométricas y datos normativos en estudiantes universitarios, Lima Metropolitana, 2021. Psycométrica, Universidad César Vallejo. https://repositorio.ucv.edu.pe/handle/20.500.12692/70510
- Mao, P., Cai, Z., He, J., Chen, X., & Fan, X. (2021). The relationship between attitude toward Science and academic achievement in Science: A three-level meta-analysis [systematic review]. Frontiers in Psychology, 12. https://doi.org/10.3389/fpsyg.2021.784068
- Mardia, K. V. (1970). Measures of multivariate skewness and kurtosis with applications. Biometrika, 57(3), 519–530. https://doi.org/10.1093/biomet/57.3.519
- Marsh, H. W., & Hau, K. T. G. D. (2005). Goodness of fit evaluation in structural equation modeling. In A. M. J. Maydeu-Olivares (Ed.), Contemporary psychometrics (pp. 275–340). Erlbaum.
- McGuire, W. J. (1969). The nature of attitudes and attitude change. In G. A. Lindzey (Ed.), The handbook of social psychology (2nd ed., Vol. 3, pp. 136–314). Addison-Wesley.
- McNeish, D. (2018). Thanks coefficient alpha, we’ll take it from here. Psychological Methods, 23(3), 412–433. https://doi.org/10.1037/met0000144
- Muñoz, J. X., Hinostroza, A. R., Daza, N. F., Nunura, L. V., Rodriguez, B. K., & Abanto, W. I. (2020). Evidencia de los procesos psicométricos en la escala de actitud frente a la investigación en estudiantes universitarios de la ciudad de Trujillo. PAIAN, 11(1), 1–15. https://doi.org/10.26495/rcp.v11i1.1319
- Muthén, B., & Asparouhov, T. (2012). Bayesian structural equation modeling: A more flexible representation of substantive theory. Psychological Methods, 17(3), 313–335. https://doi.org/10.1037/a0026802
- Muthuswamy, P., Vanitha, R., Chandramohan, S., & Ramesh, P. (2017). A study on attitude towards research among the doctoral students. International Journal of Civil Engineering & Technology, 8, 811–823. https://iaeme.com/MasterAdmin/Journal_uploads/IJCIET/VOLUME_8_ISSUE_11/IJCIET_08_11_083.pdf
- National Science Foundation. (2014). Integrated postsecondary education data system, 2013, completions survey. https://webcaspar.nsf.gov
- Nunnally, J. C., & Bernstein, I. H. (1994). Psychometric theory (3rd ed.). McGraw-Hill.
- Osborne, J., Simon, S., & Collins, S. (2003). Attitudes towards science: A review of the literature and its implications. International Journal of Science Education, 25(9), 1049–1079. https://doi.org/10.1080/0950069032000032199
- Ozturk, M. (2011). Confirmatory factor analysis of the educators’ attitudes toward Educational research scale. Kuram ve Uygulamada Egitim Bilimleri, 11, 737–747. https://files.eric.ed.gov/fulltext/EJ927375.pdf
- Papanastasiou, E. (2014). Revised attitudes toward research scale (R-ATR). A first look at its psychometric properties. Journal of Research in Education, 24, 146–159. https://doi.org/10.1037/t35506-000
- Papanastasiou, E. C. (2005). Factor structure of the “attitudes toward research” scale. Statistics Education Research Journal, 4(1), 16–26. https://doi.org/10.52041/serj.v4i1.523
- Papanastasiou, E. C., & Schumacker, R. (2014). Rasch rating scale analysis of the attitudes toward research scale. Journal of Applied Measurement, 15(2), 189–199.
- Petrella, J. K., & Jung, A. P. (2008). Undergraduate Research: Importance, benefits, and challenges. International Journal of Exercise Science, 1(3), 91–95.
- Quezada-Berumen, L., Moral de la Rubia, J., & Landero-Hernández, R. (2019). Validación de la Escala de Actitud hacia la Investigación en Estudiantes Mexicanos de Psicología. Revista Evaluar, 19(1). https://doi.org/10.35670/1667-4545.v19.n1.23874
- Raykov, T. (2007). Reliability if deleted, not ‘alpha if deleted’: Evaluation of scale reliability following component deletion. The British Journal of Mathematical and Statistical Psychology, 60(2), 201–216. https://doi.org/10.1348/000711006x115954
- Raykov, T. (2008). Alpha if item deleted: A note on loss of criterion validity in scale development if maximizing coefficient alpha. The British Journal of Mathematical and Statistical Psychology, 61(2), 275–285. https://doi.org/10.1348/000711007x188520
- Schermelleh-Engel, K., Moosbrugger, H., & Müller, H. (2003). Evaluating the fit of structural equation models: Tests of significance and descriptive goodness-of-fit measures. Methods of Psychological Research Online, 8, 23–74. https://psycnet.apa.org/record/2003-08119-003
- Schreiber, J. B. (2017). Update to core reporting practices in structural equation modeling. Research in Social and Administrative Pharmacy, 13(3), 634–643. https://doi.org/10.1016/j.sapharm.2016.06.006
- Sellami, A., Al-Ali, A., Allouh, A., & Alhazbi, S. (2023). Student attitudes and interests in STEM in Qatar through the lens of the Social cognitive theory. Sustainability, 15(9), 7504. https://doi.org/10.3390/su15097504
- Sizemore, O. J., & Lewandowski, G. W. (2009). Learning might not equal liking: Research Methods course changes knowledge but not attitudes. Teaching of Psychology, 36(2), 90–95. https://doi.org/10.1080/00986280902739727
- Sofiani, D., Maulida, A. S., Fadhillah, N., & Sihite, D. Y. (2017). Gender differences in students’ attitude towards science. Journal of Physics Conference Series, 895, 012168. https://doi.org/10.1088/1742-6596/895/1/012168
- Turner, S., & Ireson, G. (2010). Fifteen pupils’ positive approach to primary school science: When does it decline? Educational Studies, 36(2), 119–141. https://doi.org/10.1080/03055690903148662
- Vandenberg, R. J., & Lance, C. E. (2000). A review and synthesis of the measurement invariance literature: Suggestions, practices, and recommendations for Organizational research. Organizational Research Methods, 3(1), 4–70. https://doi.org/10.1177/109442810031002
- van der Westhuizen, S. (2015). Reliability and validity of the attitude towards research scale for a sample of industrial psychology students. South African Journal of Psychology, 45(3), 386–396. https://doi.org/10.1177/0081246315576266
- van de Schoot, R., Lugtig, P., & Hox, J. (2012). A checklist for testing measurement invariance. European Journal of Developmental Psychology, 9(4), 486–492. https://doi.org/10.1080/17405629.2012.686740
- Wajid, U., & Jami, H. (2020). Research self-efficacy among students: Role of metacognitive awareness of reading strategies, research anxiety, and attitude towards research. Pakistan Journal of Psychological Research, 35(2), 271–293. https://doi.org/10.33824/PJPR.2020.35.2.15
- Wiebe, E., Unfried, A., & Faber, M. (2018). The relationship of STEM attitudes and career interest. Eurasia Journal of Mathematics, Science and Technology Education, 14(10). https://doi.org/10.29333/ejmste/92286
- Wood, W. (2000). Attitude change: Persuasion and Social influence. Annual Review of Psychology, 51(1), 539–570. https://doi.org/10.1146/annurev.psych.51.1.539
- Xiao, Y., Liu, H., & Hau, K.-T. (2019). A comparison of CFA, ESEM, and BSEM in test structure analysis. Structural Equation Modeling: A Multidisciplinary Journal, 26(5), 665–677. https://doi.org/10.1080/10705511.2018.1562928
- Yau, H. K., & Leung, Y. F. (2018). The relationship between self-efficacy and attitudes towards the use of Technology in learning in Hong Kong higher Education. International MultiConference of Engineers and Computer Scientists 2018 (IMECS 2018) (Vol. II, pp. 832–834): Newswood Limited.