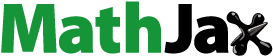
Abstract
The core of the educational system is students’ academic performance, which demands sensitive measures. In this situation, teacher-made tests (TMTs) are more promising, but they can be susceptible to measurement error if not well designed. Hence, this study aimed to investigate the relationship between the properties of TMTs and students’ academic performance in Ethiopian public universities, evidencing English language communicative skills courses. The research employs a postpositivist research paradigm, quantitative approach, cross-sectional design, purposive sampling size technique, and CTTs. It revealed that the relationship that exists between the properties of TMTs and students’ academic performance is strong positive, ranging from r=.773 to .886. This implies that the variance shared between these two variables was r2=.59 to .75/59% to 75%. A one-way ANOVA depicts (F1.202 at p = .30>.05) there was no statistically significant mean difference among public universities in students’ academic performance, suggesting their homogeneity. In addition to this, in all public universities, students’ score mean was less than the minimum requirement [mean of 37.42 out of 80, which is < 40 (50%) at P =.326 >.05], needing concern. Design-thinking utilizing correlation enabled the creation of a novel model, while the limitation of correlation statistics was maintained by computing r2. The model, ‘The Psychometric and Performance Properties of TMTs in Measuring Students’ Academic Performance’, requires further enhancement through interventional studies.
1. Introduction
1.1. Background of the study
Educational practices are based on educational measurement, which is essential to determining the effectiveness of curriculum, instruction, and measures in the process of enhancing quality education (Bijlsma et al., 2021; Marsevani, Citation2022; Nodoushan, Citation2022). Measures and students’ academic performance are key factors in this process (Bijlsma et al., 2021; Marsevani, Citation2022; Chamo, Citation2018). The degree of their association enables us to describe the quality of the curriculum, instruction, measures (tests) themselves, and the students’ performance in their learning.
Scholars emphasize that students’ academic performance is what determines whether a nation succeeds or fails (Narad & Abdullah, Citation2016; Kumar et al., Citation2021), and it is reflected in test scores (Hannah, 2021; Kumar et al., Citation2021; Chamo, Citation2018). Hence, researchers, parents, policymakers, and planners prioritize academic measurement activities in higher education institutions (HEIs) because they serve as the hub around which many critical components (curriculum, instruction, and measure) of the educational system revolve (Kumar et al., Citation2021).
From the aforementioned scholars’ viewpoints, it can be inferred that academic performance is linked to the quality of curriculum, instruction, and testing. Tests of content sensitivity, instructional sensitivity, and performance sensitivity detect these effects. This reasoning is compatible with Naumann’s et al. (Citation2019) and Polikoff’s (Citation2010) sensitivity arguments. In this sense, tests serve a dual purpose: detecting the effects of curriculum and instruction on students’ academic performance while also affecting students’ learning. The reason for the significance of the tests is that tests are crucial in the behavioral sciences, as alternative behavior measures are difficult to obtain and evaluate the success of the education system.
Tests can be standardized (STs), diagnostic, or teacher-made (TMTs), with TMTs consisting of final exams, unit tests, or weekly tests (Adom et al., Citation2020). Diagnostic tests gather student development information, while STs assess general educational goals (Razmi, 2021). Scholars from around the world, such as Bausell and Glazier (Citation2018), Loeb and Byun (Citation2019), Razmi (2021), Pietromonaco (Citation2021), and Quansah et al. (Citation2019), argue that STs are less promissory than TMTs in measuring students’ academic performance. They argue that STs encourage superficial thinking, increase stress, and encourage cheating, while TMTs are more reliable.
Scholars, on the other hand, underline the significance of TMTs, arguing that teachers’ duty to develop appropriate measuring tools has been called into question (Quansah et al., Citation2019). TMTs are thus expected to be constructed in a way that meets theoretical assumptions about psychometric and performance aspects in order to be worthwhile measures in detecting curriculum, instruction, and students’ academic performance (Cordova & Tan, Citation2018; Nodoushan, Citation2022; Suppiah Shanmugam et al., Citation2020). These aspects include validity, reliability, difficulty level (P), discrimination power (D), and distractor effectiveness (DE). These assumptions are critical for ensuring accurate, unbiased, and objective student measurement (Butakor, Citation2022).
Studies from around the globe, such as those by Tan and Cordova (Citation2019), Espinoza Molina et al. (Citation2021), GDE (Citation2017), and Zatul (Citation2020), contend that educational institutions should rely on TMT data to make reliable judgments on the academic performance of their students. This suggests that TMTs are critical, and TMTs and the academic performance of students are related to and dependent on one another. Moreover, a good number of studies from different global angles, such as Butakor (Citation2022), Espinoza Molina et al. (Citation2021), Hakim and Irhamsyah (Citation2020), Marsevani (Citation2022), and Duy (Citation2019), have raised the issue of TMT and students’ academic performance relationship in their study reports, needing further investigations.
Moreover, Duy (Citation2019) and Butakor (Citation2022) emphasize the significance of psychometric and performance property analyses of TMTs in teaching to ensure proper, fair, and objective student measurement. Duy (Citation2019) argues that when measuring students’ academic performance, their test scores can offer evidence regarding their mastery level and the quality of the measure itself. Espinoza Molina et al. (Citation2021) highlights the importance of validity and reliability in TMTs, with content validity influencing the P-level and D-power of the test item. Odili (2010), cited in Butakor (Citation2022), highlights the need for analyses of test properties in relation to students’ academic performance to maintain equality.
All of the scholars around the globe mentioned above emphasized the importance of TMT qualities in measuring the academic performance of students. Their reasoning, however, is on a hypothetical level, with no empirical evidence regarding the relationship between each variable, such as psychometric properties (validity and reliability), performance properties (P-level, D-power, and DE), and students’ academic performance. This implies that practitioners in this area in all countries should emphasize this issue.
Accordingly, when it comes to the Ethiopian context, in an era when higher education is seen as a prerequisite for success in a technologically, economically, and politically sophisticated world, troubling questions about students’ academic performance and its measure sensitivity have emerged in HEIs (Demissie et al., Citation2021; Shimekit and Oumer, Citation2021), which is probably an appealing study area, particularly the relationship between TMTs properties and students’ academic performance.
Attempts to grasp the attributes of measures, namely the psychometric and performance properties of TMTs in measuring students’ academic performance in full-fledged and their relationship were not prominent in earlier Ethiopian scholars’ works. In fact, the issue is not unique to Ethiopia; it is also a global issue (Tan and Cordova, Citation2019; Espinoza Molina et al., Citation2021; GDE, Citation2017; Quansah et al., Citation2019; Zatul, Citation2020), because the properties of TMTs in conjunction with students’ academic performance have yet to be investigated in all nations at all educational levels, both public and private, around the world. As an alternative approach, a rigorous investigation conducted in one scenario is likely to be generalizable in another.
Thus, the present study could be a novel investigation due to its rarity, value, complexity, and unfamiliarity. It offers a new model for measuring academic performance using correlation and is valuable for advancing measurement science. The study also clarifies the complexity surrounding the psychometric and performance properties of TMTs in measuring students’ academic performance using correlation. It is unfamiliar due to the fact that both psychometric and performance properties simultaneously correlate with students’ academic performance. It is new thematic research in the area, as neither cyclic nor structural research has been conducted.
1.2. Statement of the problem
Test resistance groups in the education system, including parents, teachers, scholars, and community members, have emerged globally and locally (Campos Martinez et al., Citation2022). Above all, in Ethiopia, low-quality exam provision has damaged the country’s image (Chala and Agago, Citation2022), particularly in terms of testing and competence for university graduate students (Demissie et al., Citation2021; Shimekit and Oumer, Citation2021), indicating the exceptionality of Ethiopia’s test quality.
Ethiopian scholars’ research on the psychometric and performance aspects of TMTs, as well as their association with students’ academic performance, is totally ignored in the literature. Alebachew and Minda (Citation2019) study at Ambo University, for example, focused on how TMT content validity affects instructors’ and students’ views, attitudes, motivations, activities, and teaching material selection. However, other qualities such as reliability, P-level, and D-power were overlooked.
Another Ethiopian scholar, Motuma Hirpassa (Citation2019) study on EFL TMTs at Ambo University, discovered low content validity in relation to the intended curriculum, which indicates that assessments had a detrimental impact on course teaching and learning. This gap is comparable to Alebachew & Minda (Citation2019) work, in which evaluating the quality level of TMTs based only on content validity in relation to curriculum by ignoring students’ academic performance research is problematic. Moreover, Gashaye and Degwale (Citation2019) study on English language course content validity in Ethiopian preparatory school highlights a research gap and suggests future studies should explore TMTs’ reliability, validity, P-level, and D-power.
From the Ethiopian government’s side, exit STs have been implemented to improve the quality of university measures and to assess students’ attainment of learning outcomes (Ayenew & Yohannes, Citation2022). However, this may create a knowledge vacuum because preserving TMT validity and reliability through STs is problematic. On the other side, critics and disputes have been raised on the part of many European, North American, South American, and Asian countries about using STs (Campos Martinez et al., Citation2022; Clayton et al., Citation2019; Montero et al., Citation2018). For this reason, focusing on TMTs is a suitable method for accurately measuring students’ academic performance, as the analysis of variable relationships demonstrates the quality of the measurement.
As a result, we discovered the association between the features of TMTs in combination and students’ academic performance study gaps as critical difficulties. Thus, the aim of this study was to address gaps by performing baseline surveys on the relationship between psychometric and performance properties of TMTs and students’ academic performance in an Ethiopian public university setting.
1.3. Basic research questions
What is the relationship between the psychometric and performance properties of TMTs and students’ academic performance?
What are the differences observed in students’ academic performances at Ethiopian public universities?
2. Review of related literatures
2.1. Students’ academic performance
Academic performance is defined as learning information, developing knowledge, skills, and competencies, obtaining great grades and comparable academic achievements, obtaining a progressive career, and having a drive and tenacity toward education (Kumar et al., Citation2021). Academic performance is a complex notion since it encompasses a wide range of criteria, from obtaining a professional degree to students’ moral development (York et al., Citation2015).
Academic performance is an important aspect in determining whether a nation succeeds or fails, as it indicates greater employment opportunity and a secure future (Narad & Abdullah, Citation2016; Kumar et al., Citation2021). It is also important for economic and social development since improved performance leads to the creation of skilled labor, which helps the nation’s development and sustainability (Akinleke, Citation2017; Kumar et al., Citation2021; Singh et al., Citation2016). This is a great reason for policymakers and practitioners to give the highest priority to students’ academic performance.
While placing a first-rate on the students’ success, test scores allow to determine the students’ academic performance (Hannah, 2021; Kumar et al., Citation2021; Chamo, Citation2018) and are affected by the quality of curriculum, instruction, and testing (Bijlsma et al., 2021; Marsevani, Citation2022; Nodoushan, Citation2022). Tests have a dual role in this context: sensing the effects of the curriculum and instruction on students’ academic performance (Naumann et al., Citation2019; Polikoff, Citation2010) and influencing their learning (Kirkland, Citation2016; Suppiah Shanmugam et al., Citation2020). This provides researchers with a compelling rationale to prioritize academic performance measures.
The main focus should be on properly measuring students’ academic performance because measurement matters in the educational system’s judgments (Nodoushan, Citation2022), and measurement quality affects students’ motivation (Kirkland, Citation2016; Suppiah Shanmugam et al., Citation2020) and involvement in using their full potential in their learning (Obilor & Miwari, Citation2022; Singh et al., Citation2022). GPA, continuous assessments, diagnostic tests, the mid-exam, and the final exam results are only a few examples of evidence that can be used to make a decision.
Due to its direct relationship to general aptitude and professional prospects, the GPA is a frequently utilized yardstick for judging students’ performance (Kumar et al., Citation2021). Other researchers have evaluated students’ performance based on previous year’s results or subject outcomes (Yousuf et al., Citation2011; Kumar et al., Citation2021). Classroom tests, continuous assessments, mid-semester exam results, and prior assessments have all been used to judge performance (Yousuf et al., Citation2011; cited in Kumar et al., Citation2021). Furthermore, academic performance, according to Narad and Abdullah (Citation2016), is the knowledge acquired and judged through teacher marks and educational goals set by students and teachers.
This paper focuses on academic performance in determining whether it has a relationship or not with TMTs properties to judge the working measuring qualities. There is reason to focus on it because academic performance can be viewed as the nucleus around which many significant components of the education system revolve, above all, which is why the academic performance of students and its measuring qualities, particularly those belonging to HEIs, have piqued the interest of researchers, parents, policy framers, and planners (Kumar et al., Citation2021; Duy, Citation2019; Chamo, Citation2018).
2.2. The relationship between the psychometric properties of TMTs and students’ academic performance
Psychometrics, a field in behavioral sciences, measures traits (Nodoushan, Citation2022) and prioritizes outcomes over learning processes (Duy, Citation2019). Tests must meet criteria for validity and reliability, as there is a bilateral relationship between measures and behaviors (Espinoza Molina et al., Citation2021). This study prioritizes these features in relation to students’ academic performance.
2.2.1. Validity of TMTs in measuring students’ academic performance
Validity refers to the predictability of test interpretations based on psychological theory and empirical evidence (GDE, Citation2017). Content, construct, and criterion validity are three types of evidence related to testing validity (Nodoushan, Citation2022; Gashaye & Degwale Citation2019). Content validity is crucial for measuring students’ academic performance and is more suitable in the TMT context (Gashaye and Degwale, Citation2019).
Content validity is crucial in teaching and learning as it ensures consistency between curriculum, test objectives, and content. It helps measure the achievement of objectives and course content (Nodoushan, Citation2022; Gashaye & Degwale Citation2019). Higher content validity increases test accuracy. Students may avoid studying or practicing courses that do not appear frequently in tests, focusing on the areas tested (Yibrah, Citation2017; Gashaye & Degwale Citation2019). As English proficiency becomes a criterion for admission, research on proficiency and measurement in English has become fundamental (Alghadri, Citation2019; Ehsan et al., Citation2019; Gashaye & Degwale Citation2019). However, global-scale validity, particularly content validity, is lacking in TMTs (Hartmann, 2018; Hakim and Irhamsyah, Citation2020; Quansah et al., Citation2019).
In the Ethiopian context, Motuma Hirpassa (Citation2019) research at Ambo University revealed the low content validity of TMTs in relation to intended curriculum. The study highlights the need for scientific investigation to determine the validity of TMTs in relation to students’ academic performance. Gashaye and Degwale (Citation2019) study on preuniversity schools found overemphasis, underemphasize, or ignored topics in TMTs. Motuma Hirpassa (Citation2019) suggests that teachers should conduct regular validity checks on test content to improve assessment effectiveness. This suggests that, as literature highlights, studying the relationship between TMT content validity and students’ academic performance is critical.
With the aforementioned gaps in mind, as well as their viability for TMTs, the current study conducted a baseline survey study at the university level in Ethiopia on the content validity of TMTs as one variable in the study.
2.2.2. Reliability of TMTs in measuring students’ academic performance
Validity and reliability are closely related concepts, with validity focusing on the accuracy of a test’s measurement and reliability on its consistency (Espinoza Molina et al., Citation2021; Ugwu, Citation2019). In public universities, evaluating TMT reliability is crucial. Nodoushan (Citation2022) discusses the major test reliability forms in education, including test-retest, interrater, parallel, and internal consistency (IC). IC is the most widely used and feasible reliability estimator in classroom test contexts, as it applies to groups of items measuring different aspects of the same concept. As a result, in this paper, it is chosen to represent reliability, just as content validity represents validity.
According to the authors’ literature review, the use of fullness in studies on the content validity and IC reliability of TMTs is due to their relationships. Content validity is a link between a test and an external criterion, whereas IC reliability is a quality of the test within itself. The content validity of a test is specific to a specific goal, whereas its IC reliability is independent of that purpose.
The review reveals no evidence from previous baseline surveys or intervention studies about the psychometric properties of TMTs at Ethiopian public universities that directly correlates validity and reliability with students’ academic performance. Therefore, studying the relationship between TMTs and students’ academic performance could be beneficial in the education world, as it focuses on understanding students’ abilities, aptitudes, and attitudes (Tan and Cordova, Citation2019), which are crucial for the overall education system.
2.3. The relationship between performance properties of TMTs and students’ academic performance
The performance properties of TMTs significantly impact students’ academic performance, as they aid in measuring their performance through item response analysis. This process, which involves collecting, summarizing, and using student responses, is crucial for evaluating the quality of TMTs and students’ academic performance (Marsevani, Citation2022). In many universities, without a thorough comprehension of the subject matter, students struggle to answer test-item questions (Polat, Citation2020). Hence, teachers must generate relevant questions with considerable, complex, and subjective judgment, which takes time.
To assess the effectiveness of TMTs in distinguishing between low and high scores, it is essential to analyze and evaluate items using difficulty index (P), discrimination power (D), and distractor efficiency (DE) in relation to students’ academic achievement (Marsevani, Citation2022). Studies on medical students have found that 81% of questions were about recalling information, indicating low P-indices (Kowash et al., Citation2019). Additionally, Rehman et al. (Citation2018) found that majority of TMT multiple-choice questions (MCQs) given to dentistry undergraduate students in Islamabad were of poor quality, requiring updating.
Purwoko and Mundijo (Citation2018) discovered another piece of research that agreed with the previous medical investigations of TMT MCQs quality at Muhammadiyah University. According to the findings, more than half of the questions should be rewritten because they had a low D-level, a low P-index, and a low DE. Obon and Rey (Citation2019) discovered that the majority of the questions should be amended or eliminated after investigating the TMT quality of pharmacology students utilizing P- and D-indices with DE. This is due to the poor quality of the items. However, about 70% of the distractions were kept.
According to the previous studies, the majority of the studies investigated item analysis of TMT MCQs in medical subjects solely. Furthermore, the research was not about the association. As a result, no studies have addressed the properties of TMT and their relationship with students’ academic performance in nonmedical disciplines, notably language areas. Thus, the purpose of this study was to examine the P-level and D-power as one research variable of TMTs, an English subject test for university students.
2.4. Theoretical framework
The theoretical framework is critical for assuring a study’s construct validity. According to Kivunja (Citation2018), it consists of scholar research theories employed for data analysis and interpretation. The framework summarizes previously developed concepts and theories, giving a theoretical foundation for data analysis and interpretation of study results.
Ayang (Citation2019), Bijlsma et al. (2021), Butakor (Citation2022), Cherly (2019), Tan and Cordova (Citation2019), Nodoushan (Citation2022), Malloy (Citation2018), Polat (Citation2022), and Salmani Nodoushan (Citation2021) are among the authors who explore test theories in the development and validation of TMTs. Classical test theory (CTT), item response theory (IRT), and generalizability theory (GT) are three extensively utilized theories that analyze the relationship between psychometric and performance features of TMTs and students’ academic performance.
The literature study reveals no definitive association between IRT, CTT, and GT in test validation. While some scholars argue IRT is better for item selection, it lacks reliability (Polat, Citation2022). Therefore, CTT was used as the theoretical framework in this study, which focuses on the relationship between psychometric and performance features of TMTs and students’ academic performance. Above all, the CTT approach is widely recognized in psychological and educational sciences (Bijlsma et al., 2021; Butakor, Citation2022), easy to understand, and requires minimal mathematical and statistical skill (Bijlsma et al., 2021).
2.4.1. Classical test theory (CTT)
CTT is a widely used measurement theory for test development and validation, particularly for small-scale tests like TMTs. It consists of three concepts: ‘O’ (observed score), ‘T’ (true score), and ‘E’ (error score). Mathematically, O = T + E. The assumption is that T and E scores are independent, with T indicating an examinee’s knowledge and E resulting from factors outside their control (Nodoushan, Citation2022). Psychometricians eliminate E to produce an E-free score. In an ideal environment, O = T would yield an E = 0 measurement (Ayang, Citation2019). Although CTT only examines random E, both ideas are presented in this study.
Bijlsma et al. (2021) explains that random E affects the same examinee differently across different testing sessions, affecting score reliability. Systematic E, on the other hand, is consistent across uses of the measurement tool and affects validity but not reliability. Nodoushan’s (Citation2022) research on TMTs divides E into student-related and teacher-related errors. Examinee-related errors, such as mood changes and fatigue, can differ from examinee to examinee. Teacher-related errors, like device bias and administration conditions, can also impact student performance.
Further clarity of the aforementioned variance is mathematically shown in Nodoushan’s (Citation2022) study and discussed as follows. The variance of a set of test scores can be characterized as comprising two components: s2O= s2t+ s2e, where s2O= the observed score variance, s2t= the true score variance, and s2e= the error score variance. This suggests that reliability is the ratio of the variance in T to the variance in O, and this can be represented in the following equation:
In the present study, IC is the concerned reliability. To this end, Cronbach’s coefficient alpha (α) formula will be applied. where N is the number of components (items or test lets),
is the variance of the observed total test scores, and
is the variance of component i. Alternatively, the standardized Cronbach’s α can also be defined as
where N is the number of components (items or test lets),
equals the average variance and
is the average of all covariance between the components. Test reliability falls between 0 and 1, but no test can ever achieve complete (perfect) reliability, so you would always expect a value smaller than 1 when you perform an estimation of test reliability. A test with a reliability index larger than 0.9 is excellent, but a range between 0.7 and 0.9 is also acceptable.
The CTT model predicts that E-scores are unsystematic and uncorrelated with T (rte = 0), however some claim that they are negatively associated in MCQs. The T variance and measurement error are unsystematic and random sources of variance (Nodoushan, Citation2022). The T score can be used to determine the quality of teachers’ instruction, and this project intends to study the efficiency of TMTs in detecting students’ T scores using standard error of measurement (SE/SEM).
2.4.1.1. Standard error of measurement (SE/SEM)
As discussed in Nodoushan’s (Citation2022) study, SE can be taken as the standard deviation of the error term from the CTT. The closer to zero the SE is the better. Zero reflects the absence of measurement error; thus, O = T. The SE is never larger than its s; the SE is only computed for a group, not an individual; once computed, the SEM can be used to construct an interval, wherein we expect an examinee’s T to lie; and the smaller the SEM is, the narrower the interval. Narrow intervals are more precise at estimating an individual’s T.
SE = σ* where SE = standard error of measurement σ = standard deviation Pxx = test reliability coefficient. The magnitude of the SEM is inversely related to the reliability coefficient. As ‘r’ increases, SE decreases; measurement tools with a large SE tend to be unreliable; the SE tends to remain stable across populations, reminding the researcher that any test score (or other score) is nothing more than an estimate that can vary from a subject’s ‘T’. Psychometrically speaking, SEM shows the degree of confidence that a test taker’s T falls within a particular band score (Nodoushan, Citation2022).
Nodoushan’s (Citation2022) study report on CTT identifies three test validity perspectives: criterion-related validity, construct validity, and content validity. Criterion-related validity involves using two tests measuring the same characteristic, while concurrent validity occurs when both tests are administered simultaneously, and predictive validity focuses on test performance prediction. The formula is σ2.1= The validity coefficient measures a test’s criterion-related validity, indicating its relationship with student performance. It ranges from 0 to 1, with larger coefficients increasing prediction confidence. However, a single test cannot predict performance entirely, and predictive validity in TMTs is less feasible than content validity (Gashaye and Degwale, Citation2019).
Construct validity is an assessment of a test’s capacity to evaluate a predefined trait. It is determined by the item domain person’s correlation coefficient, r. Validity coefficients rarely surpass r = 0.40. Due to the feasibility issue with TMTs, this paper does not investigate construct validity, which is related to criterion validity. Whereas content validity (CV) is the degree to which an assessment instrument’s elements are relevant and representative of the targeted construct for a specific purpose (Yusoff, Citation2019), it is more important for overall validity and more feasible in classroom settings than other validities (Gashaye and Degwale, Citation2019; Yusoff, Citation2019). Thus, when criterion and concept validity have been overestimated, content validity has been stressed in this study.
As Yusoff (Citation2019) stated, content validity estimation is a method that computes the relationship between sample test papers and courses using four rating scales (i.e., not relevant, somewhat relevant, quite relevant, and highly relevant). Experts are selected to rate the course content using the CVI procedures, formulas, and assumptions. Six steps of content validation are followed: preparing the form, selecting a review panel, conducting content validation, critically reviewing the course domain and test items, and scoring each item independently. The final step is calculating the CVI at the item and scale levels. The cutoff point for CVI is determined by the number of experts, with the cutoff point being at least 0.80 for two experts, 1.00 for three to five experts, 0.83 for six to eight experts, and 0.78 for nine experts.
CTT, a test-taking method, focuses on psychometric features but also includes performance properties like P-level and D-power tests. The P-index, or p value, is the proportion of correct answers from all students in a group, calculated using scores ranging from 100% (all students), 54% (27% from top and bottom when a sufficient number of scores is available >100), or 50% (25% from top and bottom if the number of students is less than 50). The mathematical formula: where P = difficulty index, R = examinees who accurately respond to an item, and N = the sum of examinees. The item P-index, as per Butakor (Citation2022), ranges from 0.0 to 1.0, indicating a higher response rate. The average P of a test is the average of individual item Ps, with an ideal P of 0.60 for maximum D among students. The interpretation is as follows: ≥ 0.75 is easy, ≤ 0.25 is difficult, and between 0.25 - 0.75 is average.
Regarding item D-power, Butakor (Citation2022) defines D-power as a numerical indicator that determines if a question discriminates between lower-scoring and higher-scoring students (i.e., either 27% or 25% from top and bottom). It is calculated using the D-index and D-coefficient, with a minimum value for difficult items. D-power is crucial for educational settings, and the D-index technique remains applicable for simple D-power calculations. It can be calculated using the formula where Pu and Pi represent accurate responses in the upper and lower groups, respectively, and ‘n’ is the group with the highest number of students. The index, ranging from -1 to +1, a negative index indicates a higher percentage of the lower group correctly answered the item, while a positive index indicates a higher percentage of the upper group
Butakor’s (Citation2022) D-coefficient/point biserial correlation is dependent on the type of question being answered and solves the drawback of traditional D, which only considers 54% of examinees, dismissing 46%. Hence, this method assures that each examinee contributes. The mathematical formula for a point-biserial correlation coefficient (rpbi) is where Mp = whole-test mean of examinees who answered an item correctly, Mq = whole-test mean for examinees who answered an item incorrectly, St = standard deviation for the whole test, and p = proportion of examinees answering correctly. q = proportion of examinees answering incorrectly.
The D-index formulas suggest that homogeneous class students’ ability and achievement levels lead to similar test performance, resulting in minimal discrimination between high and low groups. Questions with a P-index of 1.00 or 0.00 are not included in D-indices calculations, as they do not discriminate between students. As a guideline for interpreting item D-indices demonstrated in Butakor (Citation2022), if > +0.40, it is positive strong or very good; if +0.20 to +0.40, it is positive moderate or good; if -0.20 to +0.20, it is none/weak; and if <-0.20, it is negative moderate to strong or very weak.
The statistical features of items P and D are influenced by the sample group parameters applied to the test. This means that the P and D values obtained from one group may differ from those obtained from another group, making it impossible to predict future values (Nodoushan, Citation2022). This is a limitation in CTT item statistics, which may not be relevant for representative samples. This critique may not be particularly relevant where successive samples are reasonably representative and do not vary across time.
In summary, despite its limitations, CTT is a test theory that can provide user-friendly statistical models that enable the estimation of students’ academic performance by measuring tool effectiveness, students’ performance, and teachers’ teaching quality (Bijlsma et al., 2021; Nodoushan, Citation2022; Salmani Nodoushan, Citation2021; Subali et al., Citation2021).
3. The methodology of the study
A research methodology is a philosophical structure with crucial suppositions directing the research (Kivunja & Kuyini, Citation2017; Olugboyega, Citation2022). Thus, this section discusses the research paradigm, approach, design, and methods (sampling, data collection, and data quality control) of this baseline survey study.
3.1. The research paradigm
Researchers analyze the methodological aspects of their research project through a paradigm lens, such as postpositivism, constructivism, transformative, positivist/postpositivist, and pragmatic (Creswell & Plano Clark, Citation2018). This paper examined the relationship between the properties of TMTs and students’ academic performance using a postpositivist paradigm.
3.1.1. Postpositivists
acknowledge imperfections in reality and probabilistic truth, allowing observations without experiments or testing hypotheses, shaping research on human behavior in educational settings (Kivunja & Kuyini, Citation2017). Postpositivism is suitable for assessing TMT effectiveness in student test results, as it acknowledges that reality can only be approximated. In this paradigm, additional intervention studies are permitted to explore aspects of students’ academic performance using measuring tools, requiring ontology, epistemology, methodology, and axiology.
3.1.2. Ontology
Postpositivists disagree with the notion that truth can only be discovered within certain probability regions, even though reality exists and can only be partially grasped as a result of researcher limitations (Kivunja & Kuyini, Citation2017). This viewpoint, which is founded on objective facts, aids current researchers in comprehending reality.
3.1.3. Epistemology
The postpositivist paradigm, as highlighted by Kivunja and Kuyini (Citation2017), contends that relationships in research are influenced by the researcher’s beliefs. In this study, TMTs are used to assess the academic performance of students, while teachers’ ties with the tests are both indirect and direct. To determine whether the study’s findings are accurate, the researcher discusses knowledge sources, TMT data patterns, reactions, and the theories of reputable experts.
3.1.4. Methodology
The postpositivist research paradigm, according to Kivunja and Kuyini (Citation2017), emphasizes a quantitative research approach. To handle a scenario using a quantitative research approach, the researchers used a cross-sectional survey design, concentrating on the situation’s current condition and relevant occurrences. This study design delivers a trustworthy database for numerous research types and is suitable for ongoing investigation.
3.1.5. Axiology
The postpositivist viewpoint places a strong emphasis on researchers’ moral obligation to carry out high-quality research with an eye toward beneficence, respect, and fairness. This method places a strong emphasis on eliminating personal bias, collecting exhaustive data, and accepting the shortcomings of empirical studies (Kivunja & Kuyini, Citation2017). Hence, in this study, researchers attempted to assure ethical responsibility in their work by maximizing benefits for science, humanity, and participants and showing care for others.
3.2. Research approach
Research approaches include plans and procedures for carrying out investigations, including broad hypotheses and particular methods for gathering, analyzing, and interpreting data (Creswell & Plano Clark, Citation2018). The primary choice is dependent on the researcher’s philosophical assumptions, study designs, and procedures for gathering, analyzing, and interpreting data.
Three research methodologies were defined by Creswell and Plano Clark (Citation2018) and Olugboyega (Citation2022) as mixed, qualitative, and quantitative. By measuring variables and utilizing instrumentation to analyze the data, this baseline survey study tested objective predictions. Due to the relationship between the psychometric and performance properties of TMTs and students’ academic performance, which are more likely to test theoretical presumptions, this technique seemed appropriate.
3.3. Study design
Creswell and Plano Clark (Citation2018) defines research designs as forms of inquiry that fall under the qualitative, quantitative, and mixed approaches and provide explicit direction for the processes in a research study. Researchers used a nonexperimental quantitative survey design in this work, favoring cross-sectional designs over longitudinal ones. With the aim of studying relationships and prediction potential across time, this methodology offers a quantitative representation of the attitudes, beliefs, and behaviors of a population. The association between TMTs and the academic performance of students was examined using a cross-sectional survey design to draw conclusions for further research.
3.4. Methods
The various methods for gathering data, analyzing it, interpreting it, and controlling it that researchers suggest for their studies, as well as sampling-based research techniques (Creswell & Plano Clark, Citation2018). As a result, each step that was taken during this research is described below.
3.4.1. Study area and sampling
Ferede et al. (Citation2021), Borden and Abbott (Citation2018), and Creswell (2014) emphasize how crucial it is to choose the sample frame of potential study participants. Due to shared curricula and MOE directions (Ayenew & Yohannes, Citation2022; Alebachew & Minda, Citation2019), this baseline survey study was conducted across Ethiopia’s public universities, Jimma, Wollega, and Ambo. This made it possible for researchers to efficiently handle the investigation’s plan.
In addition to the aforementioned factor, the study considered established, new, and emerging universities (Ferede et al., Citation2021), with a sample size of two classrooms each. Moreover, it is difficult to manage a large number of students from different universities and to analyze test items for each student in different universities when the scope of the study goes beyond the selected university.
The study focuses on the English language as a crucial factor in Ethiopian public universities, as it is used as a medium of instruction. English is a key foreign language for both native and nonnative speakers (Hariharasudan & Kot, Citation2018; Sukarno, Citation2020), classified as a lingua franca (ELF), and widely used in fields such as technology, science, and commerce (Hariharasudan & Kot, Citation2018). Studies have shown that English continues to be taught in universities even when other languages are used to teach curriculum content (Alghadri, Citation2019; Ehsan et al., Citation2019). It makes sense to pay special attention to English language instruction given its significance, necessity, and intermediary nature (Görkem & Enisa, Citation2021). Thus, it is suggested that the study’s supporting data come from an English language communicative skills course that is available to all disciplines.
The researcher utilized a purposive and full population sample strategy for respondent sampling. The entire class was used for the poll because there were no more than 100 students in one classroom.
3.4.2. Target population
According to Creswell and Plano Clark (Citation2018), establishing the sample frame of potential respondents in the population is essential since it helps the researcher select the best resources and sampling techniques. The first-year student scores from the 2022 G.C. academic year at Jimma, Wollega, and Ambo universities, as well as the English language communicative skill-I TMT sheets, serve as the sample frame for the study.
3.4.3. Data collection instruments
3.4.3.1. Test
Tests were used as data collection instruments in the study to evaluate the psychometric and performance features of TMTs in measuring students’ academic performance at an Ethiopian public university. The use of this tool is justified because test scores have the potential to reflect the quality of the curriculum, instruction, and the test itself (Bijlsma et al., 2021; Polat, Citation2022). This is due to the fact that much statistical data can be derived from test results. For instance, content validity index (CVI), internal consistency (IC) reliability, difficulty (P) level, and discrimination (D) power are some of those that can allow us to judge the education system in general (Bichi & Embong, Citation2018; Suppiah Shanmugam et al., Citation2020).
In this baseline survey study, instructors were requested to submit every test they had administered throughout the academic year 2022. In order to address the study problem, which was about the relationship between the properties of TMTs and students’ academic performance, we determined the CVI, IC reliability, P-level, and D-power statistical parameters from the respective tests. To determine the CVI, six experts were requested to independently score each TMT item on the relevant scale (not relevant = 1, somewhat relevant = 2, quite relevant = 3, and highly relevant = 4), whereas to determine the IC reliability, P-value, and D-power of TMTs, students’ responses to the items were utilized.
As a result of the respective statistical parameters (CVI, IC reliability, P-level, and D-power), we were able to quantify the predictive value of TMTs, compute the association between TMTs and students’ academic performance, and estimate the current academic performance status of students. Therefore, it can be concluded that the data obtained from the respective tool enabled authors to answer the basic research question of this study.
3.4.4. Methods of data analysis
The researcher’s data analysis strategy, which was mostly CTT-rooted statistical analysis and interpretation assumptions, was utilized (Creswell & Plano Clark, Citation2018). To maintain the statistical validity of the data, the researcher in this instance initially collected data from the databases of the English language departments of the three public universities: Jimma, Wallaggaa, and Ambo.
Accordingly, all TMTs obtained from respective universities’ content relevance were estimated based on four rating scales (i.e., not relevant, somewhat relevant, quite relevant, and highly relevant) that were rated by six experts in accordance with the CVI formula and assumptions shown in Yusoff (Citation2019). This was done to estimate the psychometric and performance properties of TMTs in measuring students’ academic performance. Based on student test item responses, test performance (P-level and D-power) and IC reliability by Cronbach’s alpha were estimated, employing the formula and underlying assumptions from Butakor’s (Citation2022) work.
3.4.5. Data quality controlling mechanisms (rigor) of the study
Quantitative data usually rely on quality criteria, including validity and reliability (Cecilia, Citation2021).
3.4.5.1. Research validity
Research validity refers to the quality of a study, its components, conclusions, and applications. The validity debate encompasses four domains: internal, external, construct, and statistical. In this study, homogeneous participants (from the same department, year, semester, and class) were used to maintain internal validity, while representative samples from various universities (both natural and social science from established, new, and emerging universities) were used for external validity. Construct validity was maintained through the CTT, and descriptive and inferential statistics were used to optimize statistical validity.
Accordingly, in RQ1, the relationship between TMT qualities and students’ academic performance was computed by the Pearson correlation coefficient. RQ2 about the statistically significant difference between Ethiopian public universities in students’ academic performance was tested by one-way ANOVA. These are to follow the view of the previous study by Perez (Citation2019), which says statistical validity refers to the quantitative evaluation of the soundness of the conclusions drawn from the results of a study. Thus, steps are believed to maximize the predictive validity of the research tools and the soundness of the findings.
3.4.5.2. Research reliability
Research reliability is based on repetition-based consistency, which ensures that a study may be duplicated under identical conditions using the same methods and yielding results comparable to previous studies (Daud et al., Citation2018). External and internal reliability are the two categories. External reliability is achieved by comparing the findings of a study to earlier empirical investigations conducted by independent investigators. To that purpose, we examined all of the data to determine whether it was consistent with past empirical studies. Internal reliability is concerned with the consistency of data collection and interpretation procedures. Again, the researchers followed the scenic processes of the area while retaining the interdependence of the study components from beginning to end for this objective.
3.5. Ethical issues
Since the study’s goal is strictly academic and is endorsed by an official letter from the universities, it was carried out with their permission on ethical grounds. The researchers took into account moral concerns across all stages, such as dependability, balance, fairness, and respect for interpersonal relationships between researchers and institutions.
4. Results of the baseline survey
This study analyzes the relationship between TMT quality and students’ academic performance in Ethiopian public universities. Data from three categories, including established, new, and emerging universities, were collected for the 2022 academic year. All universities developed English language communicative skills at the English language department level.
The analysis uses student scores, item responses, and validation experts to assess TMT quality variables, including the CVI, Cronbach’s alpha, P-level, and D-power. This systematic analysis consists of two parts: describing respondents and examining background information. The second part focuses on the relationship between TMT quality variables and students’ academic performance and public universities’ differences.
4.1. Characteristics of the respondents
This research utilized Ethiopian public universities’ English language communicative skills TMT sheets, developed at the English language department level, to measure teachers’ and universities’ measuring qualities. A content relevancy rating scale was prepared for experts, revealing major characteristics such as established universities, new categories, students’ departments, and experts’ educational levels.
4.1.1. Descriptions of respondents by university categories
Universities were chosen in accordance with their classifications to ensure that the data collected were representative. The Ferede et al. (Citation2021) study’s classification criteria were employed, according to which Ethiopian public universities can be divided into three generations based on their founding year and student population sizes: established, new, and emerging. Jimma University, Wollega University, and Ambo University were chosen as established, new, and emerging universities, respectively, for the current baseline survey study.
In terms of student mode of learning classification, we focused on regular first-year students. The rationale for focusing on them is that at this level, students are divided into social and natural sciences only. But in the second year, they will be placed into fields of specialization depending on their ability and their interests. As a result, year one is at the intersection where students with different potentials may be addressed so that external validity for generalizability can be sustained. Refer to for more information.
Table 1. Descriptions of respondents by university.
The characteristics of the participants in terms of universities are shown in . As seen in the table, out of the total number of participants, 2 classrooms (1 classroom equals 16.67% social and 1 classroom equals 16.67% natural sciences) were from each university category (i.e., 33.34% from established, new, and emerging universities, respectively), and there were a total of 6 classrooms (3 classrooms equals 50% social and 3 classrooms equals 50% natural sciences), while 243 (47% social and 53% natural sciences) students were the study’s respondents. As a result, the study is able to collect representative data and preserve proportionality throughout the analysis.
4.1.2. Descriptions of experts
In this study, the content’s relevance has to be verified by professionals. Although most recommendations call for a minimum of six experts, it can be acknowledged that two experts are the minimum acceptable number for content validation (Yusoff, Citation2019). The number of specialists for content validation should be at least six and not more than ten, taking the recommendations and the researchers’ expertise into account. Six specialists were therefore involved in this study.
indicates that six experts were selected for the content validation (CVI) process, and their educational background (PhD) would enable them to accurately verify the quality of TMTs, directly contributing to the CVI. Therefore, generalizations concerning the content validity of TMTs at Ethiopian public universities can be made with confidence based on the background of the experts’ collected data.
Table 2. Descriptions of experts.
4.2. Findings and procedures
4.2.1. Procedures
For the sake of data result analysis, this part of the study was further categorized into two themes, each considering the basic RQs on which the data of the study were collected. The categories are the relationship between the psychometric and performance properties of TMTs and students’ academic performance and the differences observed in students’ academic performances at Ethiopian public universities.
The raw data collected on the RQs were entered into SPSS version 28 software. The quantitative data were obtained from TMT sheets, and the researcher first edited, categorized, and finally described it using statistical techniques such as percentage, mean, and standard deviation. CVI, Cronbach’s alpha, P-level, and D-power statistical parameters have been used to indicate the TMT's quality status, and then the relationship they have with students’ academic performance was computed by the Person product correlation coefficient. Finally, based on students’ mid and final exam scores, students’ academic performance differences with respect to their universities were computed by one-way ANOVA.
The relationship that exists between the variables was interpreted as indicated in Berhanu Nigussie Worku (Citation2020): r = below.20 for a very weak relationship, r =.20 –.39 for a weak relationship, r =.40–.59 for a moderate relationship, r =.60 –.79 for a high relationship, and r =.80 and higher for a very high relationship. Negative values were also considered in the same manner. Regarding significance level, since scholars in this area suggest that statistical significance should be reported but ignored and that the focus should be directed at the amount of shared variance, the researcher ignored them in the analysis. This is because in a small sample (e.g., N = 30), we may have moderate correlations that do not reach statistical significance at the traditional p<.05 level. In large samples (N = 100+), however, very small correlations may be statistically significant.
Moreover, a one-way ANOVA was employed to identify whether there were significant mean differences between universities in students’ academic performance. In this case, students’ academic performance is a dependent variable (DV), whereas universities are independent variables (IVs). For the one-way ANOVA interpretation, as suggested by Berhanu Nigussie Worku (Citation2020), the cutoff point is the alpha value of.05 that is usually used by scholars. That is, if lower than this alpha value, the difference is significant; if not, it is false. Therefore, the fates of further post hoc analysis and effect size are determined by the significance level. According to Berhanu Nigussie Worku (Citation2020) and Tekle (Citation2018), omega square (ῳ2) is used to show the effect size or the magnitude of the difference between the various groups. The guideline for the interpretation of the values suggested by Tekle (Citation2018) shows that.01 is a small effect,.06 is a moderate effect, and.14 is a large effect.
4.2.2. Major findings
4.2.2.1. The relationship between the psychometric and performance properties of TMTs and students’ academic performances in Ethiopian public universities
Person-product-moment correlation analysis was used to describe the strength, direction of the linear relationship, and coefficient of determination (r2) between the study variables. In the case of Ethiopian public universities’ TMT investigation, the strength of the relationship between the properties of TMTs and students’ academic performance was estimated. In this computation, the number of students who answered the item was considered the students’ academic performance.
To simplify the complexity and make it clearer, we computed all parameters (psychometric properties: content validity and IC reliability; performance properties: difficulty level and discrimination power) separately, and lastly, we computed the relationship they have with students’ academic performance using SPSS-28, as shown in the following .
Table 3. The content validity of TMTs in measuring students’ academic performance in Ethiopian public universities.
Table 4. The IC reliability of TMTs in measuring students’ academic performance in Ethiopian public universities.
Table 5. The difficulty level (P) of TMTs in measuring students’ academic performance in Ethiopian public universities.
Table 6. The D-power of TMTs in measuring students’ academic performance in Ethiopian public universities.
Table 7. The relationship between the properties of TMTs and Students’ academic performances in ethiopian public universities.
Table 8. Students’ differences in academic performance in Ethiopian public universities.
Table 9. Students’ academic performance homogeneity in Ethiopian public universities.
attests Ethiopian public universities’ TMT content validity is below CTT standard, with an S-CVI below. 83.
reveals Ethiopian public universities’ TMT IC reliability (α < 0.6) is below CTT accepted standard.
depicts that Ethiopian public universities’ TMTs are at average level (P < .6) didn’t fit the CTT excellent standard.
depicts Ethiopian public universities’ TMTs D-power (D < .2) is below the CTT accepted standard.
The data in (CVI), 4.4 (IC reliability value), 4.5 (P-value), and 4.6 (D-power) are not the objective of the study. Due to this fact, they were interpreted neither in this part nor in the discussion parts, but they are presented in order to clarify the procedure used to arrive at the results of the relationship between these variables and students’ academic performance.
Therefore, for the satisfaction of the present study objective, the relationship between the foregoing data results (CVI, IC reliability value, P-value, and D-power) and students’ academic performance was computed using person-product-moment correlation, as shown in .
above reveals that there was a strong positive correlation between CVs and students’ academic performance. Thus, between the two variables [r =.78, n = 228, p =.001<.05], CV and students’ academic performance. Hence, r2=.61 or 61% of the variation in students’ academic performance is accounted for by the CV. A similar positive correlation [r =.77, n = 228, p =.001<.05] was revealed between IC reliability and students’ academic performance. Therefore, r2=.59, or 59% of the variation in students’ academic performance, is explained by IC reliability. Again, a positive correlation [r =.886, n = 228, p =.001<.05] was revealed between P-level and students’ academic performance. Thus, r2=.75, or 75% of the variation in students’ academic performance, is explained by difficulty level. Again, a positive correlation [r =.865, n = 228, p =.001<.05] was revealed between D-power and students’ academic performance. Therefore, r2 =.74, or 74% of the variation in students’ academic performance, is explained by D-power. These results imply that there is a good relationship between variables.
4.2.2.2. Student differences in academic performance in Ethiopian public universities
In this analysis, students’ mid- and final exam scores (grade points) out of 80% were utilized to compute the academic performance differences. Reasons for the utilization of students’ scores as academic performance: From scholars’ points of view, academic performance can be understood as the quantifiable and apparent behavior of a student within a definite period and is an aggregate of scores fetched by a scholar in various evaluations through class tests, mid- and end-semester examinations, etc. (Yousuf et al., Citation2011; cited in Kumar et al., Citation2021). To check for differences between Ethiopian public university students’ academic performance, a one-way ANOVA was employed.
In above, the one-way ANOVA result shows that F = 1.202 at p = .302 >.05. This value failed to demonstrate the existence of a difference at the.05 level. Thus, no statistically significant difference was observed among Ethiopian public universities’ students’ academic performances. This implies that there is homogeneity in students’ academic status.
In above, in the one-way ANOVA, the homogenous subsets (Tukey HSD) result show that established, new, and emerging universities have a mean value of 38.07, 35.70, and 38.49 out of 80, which are < 40 (50%) (Less than the requirement) at P =.326 >.05, respectively. This implies that, despite their homogeneity, in all Ethiopian public university categories, students’ grade point mean is less than the minimum requirement of 50%.
5. Discussion
The discussion of the study is to interpret the quantitative findings, linking them with the theoretical framework and empirical studies. This is to show to what extent these quantitative findings support or challenge the working theoretical framework and what kind of research ought to be done in the future to build up the study. To this end, the researchers compared and contrasted the study’s basic RQ results with CTT assumptions and previous studies.
Accordingly, RQ1: ‘What is the relationship between the psychometric and performance properties of TMTs and students’ academic performance?’ The person-product correlation utilized reveals that there is a strong positive correlation between the psychometric properties of TMTs (CVI’s r =.784, r2=.61 or 61% & IC’s r=.773, r2=.59 or 59%) and students’ academic performance. The effect size of psychometric properties of TMTs is r2 =.61 for CVI and.59 >.14 for IC reliability, which failed in the large effect size range. These findings support Espinoza Molina et al.’s (Citation2021) assertion that psychometric properties are fundamental aspects of TMTs linked with students’ performance. Similarly, there is a strong positive correlation between TMT performance (P-level’s r=.866, r2=.75 or 75% & D-power’s r=.865, r2=.74 or 74%) and students’ academic performance. The effect size of performance properties of TMTs is r2 =.75 for P-level and .74 > .14 for D-power, which failed in the large effect size range. These findings also support the arguments of Butakor (Citation2022), who says the performance properties of TMT have associations with individual students’ performance.
The RQ1 findings imply that current students’ academic performance status evidence is largely connected with TMT's qualities; however, other variables are not yet being neglected since they require attention.
Despite the novelty of the correlation evidence study finding between TMT properties and students’ academic performance in educational measurement, the study confirmed previous claims of Butakor (Citation2022), Espinoza Molina et al. (Citation2021), Hakim and Irhamsyah (Citation2020), Marsevani (Citation2022), and Duy (Citation2019) studies that TMT psychometric and performance properties are important test qualities in measuring students’ academic performance. Butakor (Citation2022) underline the necessity of TMT quality analysis in teaching to ensure proper, fair, and objective evaluation of students. Test scores demonstrate competence level and test quality (Duy, Citation2019), whereas validity and reliability are essential qualities of TMTs (Espinoza Molina et al., Citation2021). By combining these scholars’ points of view, they implicitly hypothesize that there is a link between TMT qualities and student performance. As a result, RQ1 findings confirmed this fact.
The finding of a relationship between TMT properties and students’ academic performance is significant because it can generate a design thinking environment for the development of a conceptual model of TMT for measuring students’ academic performance. In addition, this data can be used in future interventional studies and will provide field academics with replicability input. Aside from its strengths, the existing limitation is that because the existence of a relationship informs us little about cause and effect, more research is required to demonstrate a cause-and-effect relationship through thorough experimentation.
With respect to RQ2: ‘What are the differences observed in students’ academic performances at Ethiopian public universities?’ The one-way ANOVA result shows that an F1.202 at p = .302 >.05, which was not a statistically significant mean difference, was observed among Ethiopian public universities’ students’ academic performances. In turn, this suggests the strengths and weaknesses that exist in Ethiopian public universities with regard to TMT quality status are homogeneous. Probably this inference is well consistent with Duy’s (Citation2019) argument that test scores reveal students’ mastery level and test quality.
In other words, the homogeneity finding confirms what Alebachew and Minda (Citation2019) informs us in his study: Ethiopian public universities have almost harmonized curricula and similar directives, policies, rules, or regulations for assessments and exam procedures. This consistency validates the trustworthiness of the current study findings for generalization to other public universities, as well as providing a suitable foundation for researchers in the area who wish to conduct additional intervention studies utilizing any of them as experimental and control groups.
In addition to their homogeneity, in all Ethiopian public university categories, students’ grade point mean is less than the minimum requirement of 50%. That is, their grand mean = 37.42 out of 80, which is < 40 (50%) at P =.326 >.05. It can be inferred that the curriculum, instruction, and measurement of public universities are in question. This is due to the fact that a large number of academics, including Bijlsma et al. (2021), Marsevani (Citation2022), and Chamo (Citation2018), concur that student scores are direct indicators of teaching-learning quality, offering accurate evidence on learning progress, course quality, and instruction qualities. Again, the present empirical study finding justifies the Shimekit and Oumer (Citation2021) study that describes Ethiopian university undergraduate students’ competence as always being questioned by parents, employers, and customers.
Finding obtained on students’ academic performance is significant for concerned stakeholders because it exposed how much Ethiopian public universities are in question because, as Narad and Abdullah (Citation2016) and Kumar et al. (Citation2021) mentioned in their research, at the basic level, the successes or failures of any academic institution depend largely upon the academic performance of its students. Above all, this finding alarms the future of students, and the country is at stake because, as scholars such as Akinleke (Citation2017), Kumar et al. (Citation2021), and Singh et al. (Citation2016) have highlighted, the academic performance of students is enormously important, as the economic, social, and political development and sustainability of any country are all attributable to student academic performance.
In addition to their strengths, RQ2 findings have limitations, including the controversial inability to determine whether the low academic performance problem is attributed to curriculum, instruction, or measurement rather than evidence of the existence of quality problems in general. As a result, this needs to be triangulated with study results that show the sensitivity of the tests.
However, the authors of the current research argue that using logical inference to conclude TMT's quality concern from the findings of RQ1 and RQ2. The first premise is what RQ1 revealed: that the large size of the variation in students’ academic performance is explained by TMTs properties (content validity, IC reliability, item P-level, and item D-power), whereas the second premise is what RQ2 demonstrated: that students’ academic performance in all public universities uniformly fell below the expected CTT standard. As a result of these two premises, we may argue that the quality of TMTs is a major issue at public universities.
The authors’ argument and conclusion are well consistent with previous studies’ realities, such as those of Alebachew and Minda (Citation2019) and Chala and Agago (Citation2022), who describe Ethiopian education’s image as severely tarnished as a result of poor-quality test provision. Demissie et al. (Citation2021) and Shimekit and Oumer (Citation2021) contend, in particular, that Ethiopian universities’ graduate students’ competency testing predictive is suspect. Despite the harmonization of syllabi and evaluation methods, the truthfulness of TMTs in evaluating students’ academic performance in public universities is questionable (Alebachew & Minda, Citation2019).
6. Conclusion and recommendation
6.1. Conclusion
In sum, the study investigated the relationship between TMT properties and students’ academic performance in an ELCS course at Ethiopian public universities, using literature and empirical data. Methodologically, the study employed a postpositivist research paradigm, a quantitative approach, a cross-sectional design, purposive sampling, and the CTT framework.
Accordingly, from the investigation into the literature, the authors of this study came to the reality that tests’ outcomes determine students’ academic performance and are affected by curriculum, instruction, and testing quality. In this context, tests detect the impact of these factors on students’ performance and influence their learning. However, there is no full-fledged research on the relationship between the psychometric and performance properties of TMTs and academic performance in Ethiopia and other countries at different educational levels, both public and private. As a result, the authors came up with the strong claim that a rigorous investigation in one setting may be generalized to another similar context. This means a rigorous investigation conducted in an Ethiopian public university setting may be generalizable to another HEI context.
The current study’s authors claim that employing logical inference to determine TMT's quality concern from the study’s RQs is appropriate. The first premise is what RQ1 ‘What is the relationship between the psychometric and performance properties of TMTs and students’ academic performance?’ revealed: those TMT properties explain the large size of the variation in students’ academic performance, whereas the second premise is what RQ2 ‘What are the differences observed in students’ academic performances at Ethiopian public universities?’ depicted: that students’ academic performance in all public universities uniformly fell below the expected requirement. As a result of these two premises, we may claim that the quality of TMTs at public universities is a big concern.
The study contains strengths as well as limitations. The study found a link between TMT quality attributes and academic performance in students, generating a design-thinking environment for the development of a novel TMT model. It underlines the study’s limitations, including generalizability to all HEIs, disregard for university TMT sheets, basic restrictions on correlation statistics, and financial constraints. To address these, the authors followed rigorous procedures by focusing on public universities and selecting one university from each generation for the ELCS course. We also used TMTs from the 2022 academic calendar for easier access. The study also attempted to compute r2 and r coefficients to fill correlation statistics limitations and strengthen the findings.
The study, in general, indicates university uniformity, enabling future interventional studies on assessments by classifying them as experimental and control groups. This classification of experimental and control groups enables the investigation of case-and-effect correlations as well as a better understanding of TMT properties such as curricular or instructional sensitivity while measuring students’ academic performance.
6.2. Recommendations
6.2.1. For educational assessment and evaluation scholars
Educational assessment and evaluation researchers are expected to prove the present researchers’ model, which is the ‘Model of the Psychometric and Performance Properties of TMTs in Measuring Public University Students’ Academic Performance’, through experimental studies.
6.2.2. The proposed model of the psychometric and performance properties of TMTs in measuring students’ academic performance in public universities
In accordance with the review of related literature and empirical data findings, in particular based on the relationship between the qualities of TMTs and students’ academic performance confirmed through this baseline survey study, the researchers have proposed a ‘Model of the Psychometric and Performance Properties of TMTs in Measuring Students’ Academic Performance in Public Universities’ that needs further testing through experimental studies. This is because although there is a good relationship between variables, since the existence of a relationship tells us little about cause and effect, further study is needed to establish a cause-and-effect relationship by conducting a rigorous experiment ().
Figure 1. Model of the psychometric and performance properties of TMTs in measuring students’ academic performance in public universities.

6.2.2.1. Psychometric properties
This model focuses on both content validity and internal consistency reliability to maintain the qualities of TMTs in measuring students’ academic performance.
6.2.2.2. Content validity (CV)
concerns the representativeness and relevance of TMTs in addressing learning content in the intended curriculum and measuring students’ academic performance. In the present model, it is the fundamental component of the qualities of TMTs in measuring students’ academic performance. That is, it has its own unique contribution to the group as well as being able to affect other qualities directly or indirectly. This implies that CV has the potential to play the role of others and has qualities for measuring students’ academic performance. To maintain this, a critically designed TMT table of specifications (TOS) is needed. Even though TOS is designed to maintain CV, other components are indirectly addressed through TOS.
6.2.2.3. Internal consistency (IC) reliability
This is the sustainability of TMT items in addressing the intended general learning objective while measuring students’ academic performance. The IC reliability of TMTs may be enhanced by clarity of expression, lengthening the TMT items, and utilizing item analysis. In this model, item analysis is considered the most effective way to increase the IC reliability of TMTs. This analysis consists of the computation of item difficulty (P) and item discrimination (D) indices, the latter index involving the computation of correlations between the items and the sum of the item scores of the entire test. If items that are too difficult, too easy, and/or have near-zero or negative discrimination are replaced with better items, the IC reliability of the TMTs will increase.
6.2.2.4. Performance of TMTs
This model is about giving attention to both difficulty level (P) and discrimination power (D) to maintain the qualities of TMTs in measuring students’ academic performance. In addition to their unique contribution, they can, in turn, affect the IC reliability of TMTs.
6.2.2.5. Difficulty Level (P)
The present model addresses the three learning objectives (Boolean Taxonomy) at three complexity levels that range from simple to complex. It maintains a normal distribution by making 25% easy (the first-level learning orders) and 25% difficult (the last-level learning orders) in constructing TMTs.
6.2.2.6. Discrimination Power (D)
This is developing TMT items in a way that can fit university-level competency measurement. That is it should examine and develop students’ study habits by constructing test items that can be answered only by well-prepared students and cannot be answered by any unprepared student.
6.2.2.7. Teacher’s made tests (TMTs)
a ready test that passed through the test’s developmental stage.
6.2.2.8. Department validation
This is probably the level of knowledge gap that a teacher has that will be filled by the department’s test validation and updating team.
6.2.2.9. Item analysis validation
This is the empirical stage where the weaknesses and strengths of TMTs are identified by the classroom teacher based on students’ item responses. This analysis consists of the computation of item difficulty (P) and item discrimination (D) indices. If items that are too difficult, too easy, and/or have near-zero or negative discrimination are replaced with better items, the IC reliability of the TMTs will increase.
6.2.2.10. Measuring students’ academic performance
This is the level at which TMT's score can serve academic estimation.
Statement of the authors
We declare that this study, entitled ‘The Relationship between the Psychometric and Performance Properties of Teacher-Made Tests and Students’ Academic Performance in Ethiopian Public Universities: Baseline Survey Study’, is our work and that all sources of materials used for this study have been appropriately acknowledged. We seriously declare that this study is not submitted to any other institution anywhere for the award of any degree or diploma.
Brief quotations from this study are allowable without special permission if accurate acknowledgment of the source is made. However, requests for permission for extended quotations from or reproduction in part of this manuscript may be granted by the author.
Supplemental Material
Download MS Excel (34.5 KB)Acknowledgments
The study is being carried out at Jimma University (JU). Thanks are due to JU for its coordination. The researchers also extend their gratitude to Wollega University and Ambo University, which voluntarily participated in providing the required information.
Disclosure statement
The authors declare that there exists no competing financial interest or personal relationships that could have appeared to influence the work reported in this paper.
Additional information
Notes on contributors
Tekalign Geleta Kenea
Tekalign Geleta Kenea, BA in Educational Planning and Management, BEd in Information Technology, MA in Educational Measurement and Evaluation, is currently a doctoral candidate in Educational Assessment and Evaluation at Jimma University, researching the qualities of public universities’ student learning measures.
Fisseha Mikire
Fisseha Mikre (PhD), associate professor of educational psychology in the department of psychology, Jimma University, Ethiopia.
Zenebe Negawo
Zenebe Negawo (PhD), assistant professor of educational psychology in the department of psychology, Jimma University, Oromia, Ethiopia.
References
- Adom, D., Adu Mensah, J., & Dake, D. A. (2020). Test, measurement, and evaluation: Understanding and use of the concepts in education. International Journal of Evaluation and Research in Education (IJERE), 9(1), 109–119. https://doi.org/10.11591/ijere.v9i1.20457
- Akinleke, W. O. (2017). Impact of family structure on the academic performance of secondary school students in Yewa local government area of Ogun State. Nigeria. International Journal of Sociology and Anthropology Research, 3(1), 1–10.
- Alebachew, M. G., & Minda, M. H. (2019). Washback Effect of EFL Teacher-Made Test on Teaching-Learning Process of Communicative English Skills Course at Ambo University. IJOHMN (International Journal Online of Humanities), 5(6), 29. ISSN: 2395-5155. https://doi.org/10.24113/ijohmn.v5i6.146
- Alghadri, A. M. A. (2019). Students’ Academic Performance Compared With Their Entry Level Academic Results: A Case Of Islamic University Of Technology (IUT [M.SC.TE dissertation]. Department of Technical and Vocational Education (TVE), Islamic University of Technology.
- Ayang, E. E. (2019). A comparative analysis of variability of item difficulty indices between classical test theory and item response theory using WAEC chemistry 2013.
- Ayenew, E., & Yohannes, A. G. (2022). Assessing higher education exit exam in ethiopia: practices, challenges and prospects. Science Journal of Education, 10(2), 79–86. https://doi.org/10.11648/j.sjedu.20221002.15
- Bausell, S. B., & Glazier, J. A. (2018). New teacher socialization and the testing apparatus. Harvard Educational Review, 88(3), 308–333. https://doi.org/10.17763/1943-5045-88.3.308
- Berhanu Nigussie Worku. (2020). Module for advanced statistical methods.
- Bichi, A. A., & Embong, R. (2018). Evaluating the quality of Islamic civilization and Asian civilizations examination questions. Asian People Journal, 1(1), 93–109.
- Bijlsma, H., van der Lans, R., Mainhard, T., & den Brok, P. (2021a). Student feedback on teaching in schools. International Journal Springer.
- Bijlsma, R. van der Lans, R., Mainhard, T., & den Brok, P. (2021b). A reflection on student perceptions of teaching quality from three psychometric perspectives: CCT, IRT and GT. https://doi.org/10.1007/978-3-030-75150-0_2
- Borden, K., & Abbott, B. B. (2018). Research design and methods: A process approach (10th ed.). McGraw-Hill Education.
- Butakor, P. K. (2022). Using classical test and item response theories to evaluate psychometric quality of teacher-made Test in Ghana. European Scientific Journal, ESJ, 18(1), 139. https://doi.org/10.19044/esj.2022.v18n1p139
- Campos Martinez, J., Falabella, A., Holloway, J., & Santori, D. (2022). Antistandardization and testing opt-out movements in education: Resistance, disputes and transformation. Education Policy Analysis Archives, 30(132) https://doi.org/10.14507/epaa.30.7506
- Cecilia, P., R. (2021). Mixed-method research protocol: Development and evaluation of a nursing intervention in patients discharged from the intensive care unit. Nursing Open, 8(6), 36666–33676.
- Chala, L., & Agago, M, Corresponding author, College of Education, Department of Teacher Education, Hawassa Univesity, Ethiopia, [email protected]. (2022). Exploring national examination malpractice mechanisms and countermeasures: An Ethiopian perspective. International Journal of Instruction, 15(3), 413–428. https://doi.org/10.29333/iji.2022.15323a
- Chamo, W. (2018). Evaluation of the Psychometric Quality and Validity of a Student Survey of Instruction in. Bangkok University.
- Clayton, G., Bingham, A. J., & Ecks, G. B. (2019). Characteristics of the opt-out movement: Early evidence for Colorado. Education Policy Analysis Archives, 27(33), 33. https://doi.org/10.14507/epaa.27.4126
- Cordova, C., & Tan, D. A. (2018). Mathematics Proficiency, Attitude and Performance of Grade 9 Students in Private High School in Bukidnon, Philippines‖. Asian Academic Research. Research Journal of Social Sciences and Humanities, 5(2), 103–116.
- Creswell, J. W., & Plano Clark, V. L. (2018). Designing and conducting mixed methods research (3rd ed.). SAGE Publications, Inc.
- Daud, K. A. M., Khidzir, N. Z., Ismail, A. R., & Abdullah, F. A. (2018). Validity and reliability of instrument to measure social media skills among small and medium entrepreneurs at Pengkalan Datu River. International Journal of Development and Sustainabilty, 7(3), 1026–1037.
- Demissie, M. M., Herut, A. H., Yimer, B. M., Bareke, M. L., Agezew, B. H., Dedho, N. H., & Lebeta, M. F. (2021). Graduates’ Unemployment and Associated Factors in Ethiopia: Analysis of Higher Education Graduates’ Perspectives. Education Research International, 2021, 1–9. https://doi.org/10.1155/2021/4638264
- Duy, P. (2019). Bringing Learning Back In: Examining Three Psychometric Models Bringing Learning Back. In: Examining Three Psychometric Models for Evaluating Learning Progression Theories.
- Ehsan, N., Vida, S., & Mehdi, N. (2019). The impact of cooperative learning on developing speaking ability and motivation toward learning English. Journal of Language and Education, 5(3), 83–101.
- Espinoza Molina, F. E., Arenas Ramirez, B. D. V., Aparicio Izquierdo, F., & Zúñiga Ortega, D. C. (2021). Road safety perception questionnaire (RSPQ) in Latin America: a development and validation study. International Journal of Environmental Research and Public Health, 18(5), 2433. https://doi.org/10.3390/ijerph18052433
- Ferede, B., et al. (2021). Determinants of instructors’ educational ICT use in Ethiopian higher education. Springer Science.
- Gashaye, S., & Degwale, Y. (2019). The Content Validity of High School English Language Teacher Made Tests: The Case of Debre Work Preparatory School, East Gojjam, Ethiopia. International Journal of Research in Engineering, IT and Social Sciences, 9(11), 41–50.
- Georgia Department of Education. (2017). An assessment & accountability brief: 2016-2017 Georgia milestones validity and reliability.
- Görkem E., & Enisa, M. (2021). Evaluating an english preparatory program using CIPP model and exploring the motivational beliefs for learning. Journal of Education and Educational Development8(1), 53–76. https://doi.org/10.22555/joeed.v8i1.109
- Hakim, L., & Irhamsyah, I. (2020). The analysis of the teacher-made test for senior high school at state senior high Hchool Kutacane, Aceh Tenggara. JURNAL ILMIAH DIDAKTIKA: Media Ilmiah Pendidikan Dan Pengajaran, 21(1), 10–20. https://doi.org/10.22373/jid.v21i1.4120
- Hariharasudan, A., & Kot, S. (2018). A scoping review on digital english and education 4.0 for industry 4.0. Social Sciences, 7(11), 227. https://doi.org/10.3390/socsci7110227
- Kirkland, M. C. (2016). The effects of tests on students and schools. Review of Educational Research, 41(4), 303–350.
- Kivunja, C. (2018). Distinguishing between Theory, Theoretical Framework, and Conceptual Framework: A Systematic Review of Lessons from the Field. International Journal of Higher Education, 7(6), 44. https://doi.org/10.5430/ijhe.v7n6p44
- Kivunja, C., & Kuyini, A. B. (2017). Understanding and applying research paradigms in educational contexts. International Journal of Higher Education, 6(5), 26–41. https://doi.org/10.5430/ijhe.v6n5p26
- Kowash, M., Hussein, I., Halabi, & M., Al. (2019). Evaluating the quality of multiple choice question in paediatric denistry postgraduate examinations. Sultan Qaboos University Medical Journal [SQUMJ], 19(2), 135–141. https://doi.org/10.18295/squmj.2019.19.02.009
- Kumar, S., Agarwal, M., & Agarwal, N. (2021). Defining And Measuring Academic Performance of Hei StudentsA Critical Review. Turkish Journal of Computer and Mathematics Education, 12(6), 3091–3105.
- Loeb, S., & Byun, E. (2019). Testing, accountability, and school improvement. The ANNALS of the American Academy of Political and Social Science, 683(1), 94–109. https://doi.org/10.1177/0002716219839929
- Malloy, T. E. (2018). Social relations modeling of behavior in dyads and groups. Academic Press.
- Marsevani, M. (2022). Item analysis of multiple-choice questions: An assessment of young learners. English Review: Journal of English Education, 10(2), 401–408. https://doi.org/10.25134/erjee.v10i2.6241
- Montero, L., Cabalin, C., & Brossi, L. (2018). Alto al Simce: The campaign against standardized testing in Chile. Postcolonial Directions in Education, 7(2), 174–175.
- Motuma Hirpassa, M. (2019). Content Validity of EFL Teacher-Made Assessment: The Case of Communicative English Skills Course at Ambo University. East African Journal of Social Sciences and Humanities, 3(1), 41–62.
- Narad, A., & Abdullah, B, Lovely Professional University, Phagwara. (2016). Academic performance of senior secondary school students: Influence of parental encouragement and school environment. Rupkatha Journal on Interdisciplinary Studies in Humanities, 8(2), 12–19. https://doi.org/10.21659/rupkatha.v8n2.02
- Naumann, A., Rieser, S., Musow, S., Hochweber, J., & Hartig, J. (2019). Sensitivity of test items to teaching quality. Learning and Instruction, 60(2019), 41–53. https://doi.org/10.1016/j.learninstruc.2018.11.002
- Nodoushan, M. A. S. (2022). Psychometrics revisited: Recapitulation of the major trends in TESOL.
- Obilor, E. I., & Miwari, G. U. (2022). Content Validity in Educational Assessment. International Journal of Innovative Education Research, 10(2), 57–69.
- Obon, A. M., & Rey, K. (2019). Analysis of multiple-choice questions (MCQs): Item and test statistics from the 2nd year nursing qualifying exam in a university in Cavite, Philippines. Abstract Proceedings International Scholars Conference, 7(1), 499–511. https://doi.org/10.35974/isc.v7i1.1128
- Olugboyega, O. (2022). Confirmatory sequential research design for theory building and testing: proposal for construction management research’s paradigm and methodology. Obafemi Awolowo University, Ile-Ife.
- Perez, A. (2019). Assessing quality in mixed methods research: A case study operationalizing the legitimation typology.
- Pietromonaco. (2021). The effects of standardized testing on students.
- Polat, M. (2020). Analysis of multiple-choice versus open-ended questions in language tests according to different cognitive domain levels. Novitas ROYAL (Research on Youth and Language), 14(2), 76–96.
- Polat, M. (2022). Comparison of performance measures obtained from foreign language tests according to item response theory vs classical test theory. International Online Journal of Education and Teaching (IOJET), 9(1), 471–485.
- Polikoff, M. S. (2010). Instructional sensitivity as a psychometric property of assessments. Educational Measurement: Issues and Practice, 29(4), 3–14. https://doi.org/10.1111/j.1745-3992.2010.00189.x
- Purwoko, M., & Mundijo, T. (2018). Evaluating the use of MCQ as an assessment method in a medical school for assessing medical students in the competence-based curriculum. Jurnal Pendidikan Kedokteran Indonesia: The Indonesian Journal of Medical Education, 7(1), 54. https://doi.org/10.22146/jpki.35544
- Quansah, F., Amoako, I., & Ankomah, F. (2019). Teachers’ test construction skills in Senior High Schools in Ghana: Document Analysis. International Journal of Assessment Tools in Education, 6(1), 1–8. https://doi.org/10.21449/ijate.481164
- Razmi, M. H., Khabir, M., & Tilwani, S. A. (2021). A meta-analysis on the predictive validity of Graduate Record Examination (GRE) General Test. Tabaran Institute of Higher Education. International Journal of Language Testing, 11(2)
- Rehman, A., Aslam, A., & Hassan, S. H. (2018). Item analysis of multiple choice questions in pharmacology. Pakistan Oral & Dental Journal, 38(2), 291–293. https://doi.org/10.52206/jsmc.2020.10.2.320
- Salmani Nodoushan, M. A. (2021). Wash back or backwash? Revisiting the status quo of wash back and test impact in EFL contexts. Studies in English Language and Education, 8(3), 869–884. https://doi.org/10.24815/siele.v8i3.21406
- Shimekit, T., & Oumer, J. (2021). Ethiopian Public Universities Graduates Employability Enhancement at the Labor Market: Policies, Strategies, and Actions in Place. Academy of Educational Leadership Journal, 25(7), 1–18.
- Singh, C. K. S., Singh, H. K. J., Singh, T. S. M., Moneyam, S., Abdullah, N. Y., & Zaini, M. F. (2022). ESL teachers’ assessment literacy in classroom: A review of past studies. Journal of Language and Linguistic Studies, 18(1), 01–17.
- Singh, S. P., Malik, S., & Singh, P. (2016). Research paper factors affecting academic performance of students. Indian Journal of Research, 5(4), 176–178.
- Subali, B., Kumaidi, K., & Aminah, N. S. Prof., Universitas Negeri Yogyakarta, Indonesia, [email protected]. (2021). The Comparison of item test characteristics viewed from classic and modern test theory. International Journal of Instruction, 14(1), 647–660. https://doi.org/10.29333/iji.2021.14139a
- Sukarno, S. (2020). Enhancing English language teaching and learning in Industrial Revolution 4.0 Era. Methods, Strategies and Assessments.
- Suppiah Shanmugam, S. K., Wong, V., & Rajoo, M. (2020). Examining the quality of English test items using psychometric and linguistic characteristics among grade six pupils. Malaysian Journal of Learning and Instruction, 17(2), 63–101. https://doi.org/10.32890/mjli2020.17.2.3
- Tan, D. A., & Cordova, C. C. (2019). Development of Valid and Reliable Teacher-Made Tests for Grade 10 Mathematics. International Journal of English and Education, 8(1)
- Tekle, E. (2018). Teachers induction practices in secondary schools of Ethiopia.
- Ugwu, N.-G. (2019). Ensuring Quality in Education: Validity of Teacher-made Language Tests in Secondary Schools in Ebonyi State. American Journal of Educational Research, 7(7), 518–523.
- Yibrah, M. (2017). Assessing Content Validity of the EGSEC English Examinations. Haramaya University.
- York, T. T., Gibson, C., & Rankin, S. (2015). Defining and measuring academic success. Practical Assessment, Research, and Evaluation, 20(1), 5.
- Yousuf, M. I., Imran, M., Sarwar, M., & Ranjha, A. N. (2011). A study of non-cognitive variables of academic achievement at higher education: Nominal group study. Asian Social Science, 7(7), 53. https://doi.org/10.5539/ass.v7n7p53
- Yusoff, M. S. B, Department of Medical Education, School of Medical Sciences, Universiti Sains Malaysia, MALAYSIA. (2019). ABC of content validation and content validity index calculation. Education in Medicine Journal, 11(2), 49–54. https://doi.org/10.21315/eimj2019.11.2.6
- Zatul, T. (2020). Investigating reliability and validity of student performance assessment in Higher Education using Rasch Model. Journal of Physics: Conference Series, 1529, 042088.