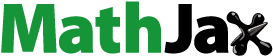
Abstract
This study delves into the multifaceted relationship between student satisfaction and academic performance in undergraduate economics. While prior research often overlooks the complex interplay of these factors with cognitive potential, prior knowledge, and gender, this investigation sheds light on their combined influence on student success. Data collected from 115 economics students at the University of Rwanda were analyzed using an econometric approach [both maximum likelihood estimator (MLE) and ordinary least squares (OLS)] and t-test. The findings reveal interesting gender differences: female students reported higher satisfaction, suggesting stronger self-efficacy and intrinsic motivation, while male students showed superior academic performance. Additionally, factors like level of study and personal laptop access impacted satisfaction, while higher cognitive abilities, deeper engagement with the field, and prior math background positively predicted academic achievement. Intriguingly, the study found that female students performed lower despite higher satisfaction levels, prompting further research into potential explanations. Additionally, older students exhibited lower performance, highlighting the need for understanding age-related factors influencing academic success. These findings advocate for a multifaceted approach to supporting student success in economics. By prioritizing student satisfaction, fostering individual cognitive potential, and delivering tailored support based on diverse needs (including gender and age), educational institutions can create the optimal conditions for all economics students to flourish.
REVIEWING EDITOR:
1. Introduction
In the dynamic and competitive landscape of higher education, understanding the factors that influence student satisfaction and academic performance is crucial for enhancing learning outcomes and institutional success (Kuh et al., Citation2006; Pascarella & Terenzini, Citation2005). The University of Rwanda (UR), as a leading academic institution in Rwanda, recognizes the importance of student satisfaction and academic performance in achieving its strategic goals. Therefore, investigating the relationship between satisfaction and academic performance among undergraduate economics students at UR is of paramount significance.
By examining the factors that contribute to student satisfaction, such as teaching quality, learning environment, and institutional resources, we can identify areas for improvement and enhance the overall student experience (Tinto, Citation2012). Additionally, understanding the linkages between satisfaction and academic performance will provide valuable insights into how positive student experiences can translate into improved learning outcomes (Pascarella & Terenzini, Citation2005). The research by Mihanović et al. (Citation2016) emphasizes the crucial role of faculty and teaching methods in shaping not only student learning but also their broader university experience and success. It highlights the need for universities to prioritize creating a positive learning environment and providing strong academic support to enhance student satisfaction and performance.
The theoretical framework for this study is drawn from two primary theories: Astin’s Input-Output Model (Chigbu & Nekhwevha, Citation2021) and Tinto’s Model of Student Departure (Tinto, Citation2012). Astin’s Input-Output Model posits that student satisfaction is influenced by a set of input variables, such as student characteristics, institutional characteristics, and environmental factors (Chigbu & Nekhwevha, Citation2021). These input variables, in turn, influence student outcomes, such as academic performance, persistence, and graduation rates (Chigbu & Nekhwevha, Citation2021). Tinto’s Model of Student Departure suggests that student satisfaction is crucial for student retention and academic success. When students are satisfied with their academic experiences, they are more likely to persist in their studies and achieve their educational goals (Tinto, Citation2012).
In the context of undergraduate economics students, these theories suggest that factors, such as teaching quality, learning environment, institutional resources, and peer interactions can influence student satisfaction, which in turn, can impact academic performance. Understanding the interplay between these factors is essential for developing effective interventions to enhance student satisfaction and academic success in undergraduate economics programs. In the same context, Galbraith and Merrill's (Citation2012) findings highlight the potential of peer observation as a tool for faculty development and improving student achievement. It encourages a move beyond traditional student ratings of teaching effectiveness (SETEs) to consider more nuanced measures of teaching effectiveness that capture the complex interactions between teacher behaviors, student engagement, and learning outcomes. Even though this research does not directly address the drivers of student satisfaction, it can be inferred that effective teaching practices (clear organization, active participation, etc.) could indirectly contribute to student satisfaction.
Several studies have investigated the relationship between student satisfaction and academic performance in higher education. These studies have consistently shown that there is a positive correlation between satisfaction and performance (Kuh et al., Citation2006; Pascarella & Terenzini, Citation2005). Additionally, studies have identified several factors that influence student satisfaction, including teaching quality, learning environment, institutional resources, and peer interactions (Chigbu & Nekhwevha, Citation2021; Tinto, Citation2012).
These empirical findings highlight the importance of understanding the factors that influence student satisfaction in undergraduate economics programs. By identifying and addressing these factors, institutions can enhance student satisfaction, promote academic performance, and produce graduates who are well-prepared for success in the field of economics.
The University of Rwanda (UR) was established in 2013 through the merger of several public institutions of higher learning, including the National University of Rwanda (NUR). The NUR, the predecessor to UR, was founded in 1963 as the first university in Rwanda. Economics has been a core discipline at UR since its inception, and the university’s Department of Economics is one of the most respected economics departments in the country. Over the years, the department has produced numerous graduates who have gone on to successful careers in academia, government, and the private sector. In recent years, UR has made significant strides in improving the quality of its education and enhancing the student experience. The university has invested heavily in infrastructure, faculty development, and student support services. As a result, UR has attracted a growing number of talented students and has become a leading institution of higher education in Rwanda.
Understanding the satisfaction and academic performance of undergraduate economics students at the University of Rwanda (UR) is crucial within the broader context of Rwanda’s higher education landscape and its economic development aspirations. Higher Education Institutions in Rwanda witnessed an 8.4% surge in student enrollment during the 2021/2022 academic year, welcoming a total of 95,863 students. This marks a significant increase from the previous academic year, which enrolled 88,448 students. For all education levels, over six out of ten individuals within the official school age range of 3–22 years are actively engaged in education, reflecting a participation rate of 63.0% (MINEDUC, Citation2023). This expansion might have been driven by the government investment in education (Okoye et al., Citation2022), the government’s recognition of the importance of education in fostering economic growth (Benos & Zotou, Citation2014; Ahmad et al., Citation2023), and reducing poverty (Liu et al., Citation2021). This could also be due to strong parental support for education, as well as a growing economy that creates demand for skilled workers.
Economics plays a critical role in Rwanda’s economic development strategy. The government has identified economics as a key area of focus for investment and has implemented policies to promote the development of skills in economics and related fields. These efforts are aimed at creating a skilled workforce that can support Rwanda’s transition to a knowledge-based economy (Government of Rwanda, Citation2017).
In this context, ensuring that undergraduate economics students at UR are satisfied with their academic experiences and achieve high levels of academic performance is essential for meeting the country’s economic development needs. By understanding the factors that influence student satisfaction and academic performance, UR can develop targeted interventions to enhance the quality of its economics programs and produce graduates who are equipped to contribute to Rwanda’s economic transformation.
Despite the growing body of research on student satisfaction and academic performance, there is a notable research gap in the context of undergraduate economics students in Rwanda. Existing studies have primarily focused on the general student population or students in other disciplines, leaving a gap in understanding the specific factors that influence the satisfaction and academic performance of economics students in Rwanda. This research aims to address this gap by providing a comprehensive analysis of the factors that contribute to satisfaction and academic performance among undergraduate economics students at UR. More specifically, this study aims to (1) investigate the key factors that contribute to the satisfaction of undergraduate economics students at UR; and (2) analyze the relationship between satisfaction with various academic services and the academic performance of undergraduate economics students at UR. Accordingly, this study attempts to answer two specific research questions: (1) What are the primary factors that contribute to the satisfaction of undergraduate economics students at UR? (2) How does the student satisfaction with the academic performance of undergraduate economics students affect their academic performance?
By addressing these research questions, this study will provide a valuable contribution to the understanding of student satisfaction and academic performance in the context of undergraduate economics education in Rwanda. The findings of this study will have practical implications for UR's College of Business and Economics, enabling the development of targeted interventions and strategies to enhance student satisfaction and academic performance. By fostering a supportive and engaging learning environment, UR can empower its economics students to reach their full potential and contribute to the future economic development of Rwanda.
2. Literature review
2.1. Theoretical literature review
This paper explores the intricate relationship between student satisfaction and academic performance, drawing upon various theoretical frameworks. Our analysis emphasizes how satisfaction contributes to positive learning environments, fostering motivation, engagement, and ultimately, academic success.
Student satisfaction and academic performance can essentially be backed by motivational theories, cognitive theories, socio-ecological theories, and specifically in the Rwandan context, ubuntu philosophy. Starting with motivational theories, the Self-Determination Theory (SDT) proposes that intrinsic motivation, fueled by the desire for personal growth and mastery, leads to higher academic achievement (Ryan & Deci, Citation2017). Student satisfaction can contribute to intrinsic motivation by fostering a positive learning environment and enhancing engagement with the subject matter (Deci & Ryan, Citation2000). For the Goal Setting Theory, it is suggested that specific, challenging, and attainable goals can guide and motivate students (Locke & Latham, Citation2002). When students are satisfied with their learning environment and resources, they may be more likely to set and pursue ambitious goals, ultimately leading to improved performance (Latham & Locke, Citation2018). As for the Expectancy-Value Theory, it posits that students are motivated by their expectations of success and the value they place on achieving specific outcomes (Eccles & Wigfield, Citation2002, Citation2020). A positive learning environment that fosters satisfaction can increase students’ confidence in their abilities and the perceived value of academic success, leading to improved performance (Hulleman et al., Citation2016).
In line with this study, Cognitive Theories encompass a set of two theories, namely cognitive load theory, and flow theory. Cognitive Load Theory suggests that complex learning tasks can overload students’ cognitive resources (Sweller, Citation2011). When students feel satisfied with their learning experience, they may be better able to manage their cognitive resources and focus on learning, leading to improved performance (Szulewski et al., Citation2023). As for the Flow Theory, it describes a state of optimal experience characterized by complete absorption in an activity (Heutte et al., Citation2021). When students are engaged and satisfied with their learning, they may be more likely to enter a flow state, facilitating deeper understanding and improved performance (Csikszentmihalhi, Citation2020).
Concerning Social-Ecological Theories, Social Cognitive Theory (SCT) and Bronfenbrenner’s Ecological Systems Theory are aligned with the current study. Social Cognitive Theory (SCT) emphasizes the role of social learning and individual agency in shaping behavior (Schunk & DiBenedetto, Citation2020). Peers, teachers, and the overall academic environment can influence students’ beliefs about their abilities and the value of academic achievement (Bandura, Citation2014b). A positive and supportive learning environment that fosters satisfaction can contribute to positive self-efficacy and academic engagement, leading to improved performance (Schunk, Citation2012). In the same vein, Bronfenbrenner’s Ecological Systems Theory posits that individuals are nested within a series of interconnected systems, such as family, school, and community (Bronfenbrenner, Citation2013). These systems can influence individual development and academic success. When students experience satisfaction within their various social systems, it can create a supportive network that promotes academic achievement (May-Varas et al., Citation2023).
In addition, the theoretical literature on student satisfaction and academic performance provides a valuable framework for understanding the complex factors that influence student outcomes in higher education. By identifying and addressing these factors, institutions can enhance student satisfaction, promote academic performance, and produce graduates who are well-prepared for success in their chosen fields.
As students progress through their academic journey, their perceptions and expectations evolve, influencing their overall satisfaction with their time at school. This dynamic nature of student satisfaction is highlighted in the research of Astin (Citation1999) and Pascarella and Terenzini (Citation2005), who found that satisfaction fluctuates throughout a student’s journey, influenced by factors, such as changing expectations and course-specific experiences. Students may enter with high expectations for challenging coursework and opportunities for personal growth, but if these expectations are not met, their satisfaction can decline. Conversely, positive course experiences, supportive teaching environments, and meaningful engagement opportunities can contribute to increased satisfaction levels. Institutions can foster a more satisfying academic experience by providing supportive teaching environments, encouraging positive interactions among students and faculty, offering meaningful engagement opportunities, identifying struggling students early on, creating a culture of feedback, and continuously gathering student input.
Student satisfaction is a crucial aspect of higher education, influencing academic performance, retention, and future career success. Research has demonstrated a clear link between high satisfaction and positive outcomes, including improved grades, increased graduation rates, and enhanced career prospects. Institutions can foster a positive and satisfying learning environment by providing quality instruction, offering opportunities for meaningful engagement, creating a supportive campus environment, providing timely and effective support services, and gathering regular feedback. By investing in student satisfaction, institutions can not only improve the quality of the student experience but also contribute to the overall success of their students. High satisfaction is linked to positive academic performance, graduation rates, and future career success (Tudor, Citation2018).
By employing these diverse theoretical perspectives, we gain a comprehensive understanding of how student satisfaction and academic performance are intricately linked. This framework provides a valuable foundation for further research and educational practice aimed at creating optimal learning environments that cultivate student satisfaction and drive academic success.
2.2. Empirical literature review
Student satisfaction is a crucial aspect of higher education, as it influences academic performance, retention, and overall well-being. In the context of undergraduate economics programs, understanding the factors that contribute to student satisfaction is essential for enhancing the learning experience and promoting academic success. This empirical literature review delves into the relationship between satisfaction and academic performance among undergraduate economics students at the University of Rwanda.
Numerous studies have identified a range of factors that influence student satisfaction with their academic experiences. These factors can be broadly categorized into three main areas. The first area concerns Teaching Quality, which is consistently identified as a significant factor in student satisfaction. Students are more satisfied when they perceive that their instructors are knowledgeable, engaging, and accessible (Chigbu & Nekhwevha, Citation2021; Pascarella & Terenzini, Citation2005). The second is the Learning Environment which also plays a crucial role in student satisfaction. Factors, such as the quality of library resources, technology access, and the availability of support services can contribute to student satisfaction (Kuh et al., Citation2006; Tinto, Citation2012). The third (and thus the last) area encompasses Institutional Factors: Institutional characteristics, such as the institution’s reputation, class size, and overall campus climate, can also influence student satisfaction. Students are more satisfied when they feel connected to their institution and perceive that it is a supportive and welcoming environment (Chigbu & Nekhwevha, Citation2021; Pascarella & Terenzini, Citation2005).
The positive relationship between student satisfaction and academic performance is evident in the literature. Studies have shown that students who are more satisfied with their academic experiences tend to have higher grade point averages (GPAs), better course grades, and are more likely to graduate (Kuh et al., Citation2006; Pascarella & Terenzini, Citation2005). This relationship is likely due to the fact that satisfaction can lead to increased motivation, engagement, and persistence in academic pursuits.
Understanding the factors that influence student satisfaction and academic performance is particularly relevant for undergraduate economics students in Rwanda. As Rwanda’s higher education sector continues to expand, ensuring that economics students are satisfied with their education and achieve high levels of performance is essential for meeting the country’s economic development needs.
Several studies have investigated the impact of teaching methods and faculty expertise on student satisfaction and academic performance in undergraduate economics courses. Roach’s (Citation2014) study provides evidence that flipped learning can be an effective instructional method for economics education. Students who experience flipped learning are likely to have positive perceptions of it and to experience positive learning outcomes. While examining the relationship between student engagement and academic performance, Hu et al. (Citation2014) findings are consistent with a growing body of research on the importance of student-faculty interaction in higher education. This interaction can help students to develop a sense of belonging, feel more connected to their studies, and receive personalized support from their faculty. As a result, student-faculty interaction is a valuable tool for promoting student engagement and achievement.
Peer support and networking opportunities have also been shown to play a significant role in student satisfaction and academic performance in undergraduate economics programs. A study by Okolie et al. (Citation2022) found that collaborative learning (CL) is a promising approach for enhancing student engagement in the acquisition of practical skills (SEPSA) in higher education. By fostering task value, mastery goal orientation, and using structured activities, educators can create CL environments that promote deeper engagement and better skill development in their students.
The effectiveness of institutional policies and resources in influencing student satisfaction and academic performance has also been explored in the literature. Pascarella and Terenzini (Citation2005) found that students were more satisfied with institutions that had clear academic policies, provided adequate academic support services, and fostered a supportive campus climate. These factors contributed to higher levels of academic engagement and, consequently, improved academic performance. Longitudinal studies that track student satisfaction and academic performance over time provide valuable insights into the long-term effects of interventions and the factors that contribute to sustainable student success. Studies by Kuh et al. (Citation2010) and Whitt et al. (Citation2013) followed a cohort of students over four years and found that those who were initially more satisfied with their college experience were more likely to persist, graduate, and achieve higher levels of post-graduation success. Follow-up research with graduates can also shed light on the impact of undergraduate economics education on career outcomes and professional achievements.
The empirical literature on student satisfaction and academic performance provides strong evidence that these two constructs are positively correlated. Undergraduate economics students who are more satisfied with their academic experiences tend to achieve higher levels of academic performance. The literature also identifies a range of factors that influence student satisfaction, including teaching methods, faculty expertise, peer support, institutional policies, and resources. Understanding these factors and implementing effective interventions can enhance student satisfaction, promote academic performance, and contribute to the success of undergraduate economics programs.
Building upon the reviewed literature and aligned with the research’s specific objectives, presents a conceptual framework that elucidates the relationships between the study variables pertaining to student satisfaction and academic performance. This framework serves as a roadmap for the investigation, guiding the analysis of how these variables interact and potentially influence each other.
3. Materials and methods
3.1. Sources of data
This section describes the research design, sources of data, sampling procedure, sample size, as well as the methods of data analysis used in this study. A representative sample of economics students enrolled in a 3-year undergraduate programme at the University of Rwanda. The target population consisted of all 311 enrolled students. To ensure representativeness and minimize selection bias, a stratified random sampling technique was employed. This method involves dividing the population into subgroups (strata) based on a relevant characteristic, in this case, year level. Proportional allocation was used to determine the sample size for each stratum. This ensures that each subgroup is represented in the sample according to its proportion in the population. Following this approach, 30 students were selected from Year 1, 28 students from Year 2, and 57 students from Year 3, resulting in a total sample size of 115 (Iliyasu & Etikan, Citation2021). To ensure unbiased selection within each year level (stratum), a simple random sampling technique, such as random number generation, was employed to select individual participants from the respective student lists (see Khadka, Citation2019; Sekaran & Bougie, Citation2016). Data was collected through a well-structured questionnaire in August–September 2021. A survey questionnaire was administered to gather data on student satisfaction levels with respect to various aspects of their academic experiences, including teaching quality (their active participation), learning environment, institutional resources, and overall satisfaction. The survey also collected demographic information and academic performance data, namely annual average academic scores.
3.2. Study variables and empirical model
Within the realm of scientific analysis, the meticulous selection and operationalization of variables, alongside the construction of a robust empirical model, constitute the cornerstone of meaningful research. By translating theoretical constructs into quantifiable entities, we collected and analyzed data with precision. For this study, the details of the study variables are summarized in .
Table 1. Definition and measurement of the study variables.
Following the established principles of scientific research, the empirical model for this study was meticulously crafted and designated. This empirical model embodies the theoretical framework underlying the investigation, explicitly outlining the relationships between the key variables and the anticipated outcomes. The specified model is described as per EquationEquation (1)(1)
(1) .
(1)
(1)
Where Y is the dependent variable, X is the vector of all independent variables selected for this study, α is the constant, β is the vector of coefficients of the independent variables X, and e is the error term. EquationEquation (1)(1)
(1) was utilized to estimate two distinct models. In the first model, the equation served to identify the factors driving the satisfaction of economics students. Subsequently, the second model employed the equation to investigate the impact of satisfaction on academic performance.
3.3. Methods of data analysis
Quantitative data from the survey were analyzed using a regression approach to identify patterns, and potential causal relationships between satisfaction, academic performance, and various factors. The student test (t-test) was also conducted to compare the annual mean scores between the groups of students with and without prior background in mathematics.
Aiming to identify the factors influencing the satisfaction of university economics students, EquationEquation (1)(1)
(1) adopts the form of a binomial logistic regression model. This choice is driven by the dichotomous nature of the dependent variable, which assumes two distinct values: 1 (indicating student satisfaction with academic services) or 0 (denoting dissatisfaction). In such circumstances, the data were analyzed utilizing the maximum likelihood estimator (see Agresti, Citation2018; Breen et al., Citation2018).
Given the continuous nature of the dependent variable, measuring academic performance, EquationEquation (1)(1)
(1) adopts a linear functional form. This choice is consistent with the established practice within the field of educational research, where linear regression models are commonly employed to analyze the relationship between student satisfaction and academic outcomes (e.g. Cheong & Ong, Citation2016; Hopland & Nyhus, Citation2015; Khan & Iqbal, Citation2016; Kirmizi, Citation2015). Consequently, the ordinary least squares (OLS) method was deemed the most appropriate statistical technique for estimating the parameters of the model. This approach minimizes the sum of squared errors between predicted and observed values, providing a robust framework for analyzing the association between student satisfaction and academic performance (Hair et al., Citation2010).
In intent to compare academic performance between student groups with and without prior mathematics background, a t-test was conducted. The comparison of mean scores for two independent samples, n1 and n2, originated from Student (Karch, Citation2021). It is a proficient and powerful analytical tool to compare the mean score of group1, and the mean score of the group2,
Following Van Elst (Citation2019), this is described by EquationEquation (2)
(2)
(2) .
(2)
(2)
where
stands for the Student statistic;
and
are the sample 1 and sample 2, respectively;
and
the estimated means of the sample 1 and sample 2, respectively;
the standard error [
where
is the estimated sample variance for the sample 1;
is the estimated sample variance of sample 2];
the Student distribution;
the degree of freedom;
and
This entails that the size of both reference and comparison groups should be bigger than or equal to 50 observations.
4. Results and discussion
4.1. Factors affecting student satisfaction
Results from the econometric analysis in reveal that gender, specifically being female, emerged as the sole variable with a statistically significant positive effect on student satisfaction. This implies that females may demonstrate stronger self-efficacy beliefs and intrinsic motivation for learning, leading to a more positive outlook on their academic experience. Besides, it seems that females may favor collaborative learning environments and communication-intensive approaches, which are often associated with higher levels of engagement and satisfaction.
Table 2. Binary logistic estimations of the drivers of the satisfaction of undergraduate economics.
Conversely, both the level of study and the challenge of not having a laptop exerted a significant negative influence on student satisfaction. Concerning the level of study, the results could entail that higher levels of study often demand greater time commitment, complex coursework, and intense assessments, potentially leading to stress and burnout. Another reason could be that advanced coursework might prioritize theoretical knowledge over practical application, leading to a sense of disconnect and dissatisfaction among students who prefer hands-on learning experiences.
4.2. Effect of student satisfaction on the academic performance of undergraduate economics students
The econometric analysis presented in reveals a strong and statistically significant positive association between student satisfaction and academic performance. This implies that students who are more satisfied with their educational experience tend to achieve higher academic outcomes. This finding underscores the importance of fostering a positive and engaging academic environment to optimize student learning and success.
Table 3. Ordinary least squares estimations of the effect of student satisfaction on the academic performance of undergraduate economics students.
Beyond student satisfaction, the analysis also identifies several other variables that exert a positive and significant influence on academic performance. Results indicate that the student’s score at secondary school completion (SECSCOR) is one of the primary drivers of academic performance: Students with higher cognitive abilities, as measured by the COBIN index, tend to perform better academically. This highlights the importance of individual cognitive potential in educational achievement. As for the level of study (LEVEL), results show that the students enrolled in higher levels of study tend to have higher academic performance, likely due to their prior academic preparation and deeper engagement with their chosen field.
Interestingly, the analysis also reveals variables with a negative impact on academic performance. For the sex of the student (SEX), results reveal that female students, in this sample, exhibit lower academic performance compared to their male counterparts. Further research is needed to explore the complex interplay of gender and academic achievement. Concerning the age (AGE), results show that older students, in this sample, demonstrate lower academic performance compared to younger students. This could be attributed to various factors, such as competing life demands or changes in learning styles with age.
Our cross-sectional model exhibits an R-squared value of 0.318. This value falls within the acceptable range for cross-sectional analyses, where an R-squared ≥0.20 is commonly considered sufficient (see Wooldridge, Citation2010).
It is important to note that these findings are based on a specific study and might not be generalizable to all populations. Nevertheless, they provide valuable insights into the factors that influence student performance and highlight the need for a multifaceted approach to educational success. By addressing factors like student satisfaction, cognitive abilities, and providing tailored support based on individual needs, educational institutions can create the optimal conditions for students to thrive.
4.3. Effect of mathematics background on academic performance of economics students
Complementing regression analysis, a Student test (t-test) was conducted to compare the academic performance between student groups with and without prior background in mathematics. Results in reveal a statistically significant difference between these two groups. Specifically, students possessing prior mathematics background demonstrated higher annual academic performance compared to their counterparts without such background. This finding provides robust evidence supporting the notion that prior knowledge and experience in mathematics serve as a significant predictor of subsequent academic success among undergraduate economics students.
Table 4. Comparison of academic performance between student groups with and without prior mathematics background.
4.4. Discussion of the main findings
The present study, through econometric analysis, sheds light on the factors influencing student satisfaction. Interestingly, gender emerged as the sole variable with a statistically significant positive effect. This study’s finding that female students exhibit higher satisfaction aligns with the theoretical perspectives presented in the literature review. Self-Determination Theory suggests females might possess stronger intrinsic motivation and self-efficacy beliefs (Ryan & Deci, Citation2017), potentially contributing to a more positive outlook on their learning experience. This finding also corroborates existing research suggesting that females may demonstrate stronger self-efficacy beliefs and intrinsic motivation for learning, leading to a more positive outlook on their academic experience (Bandura, Citation2014a). Additionally, females may favor collaborative learning environments and communication-intensive approaches, fostering higher levels of engagement and satisfaction (Brown & Palincsar, Citation2018).
Conversely, both the level of study and the challenge of not having a laptop exerted a significant negative influence on student satisfaction. The finding that higher levels of study negatively impact satisfaction aligns with Cognitive Load Theory (Sweller, Citation2011). Complex learning tasks in advanced coursework can overload students’ cognitive resources, leading to stress and diminishing satisfaction (Szulewski et al., Citation2023). This result also echoes aspects of Motivational Theories like Self-Determination Theory (SDT) and Expectancy-Value Theory (Eccles & Wigfield, Citation2020). High academic pressure and workload can undermine intrinsic motivation and the perceived value of academic success, negatively impacting satisfaction (Eccles & Wigfield, Citation2002; Ryan & Deci, Citation2017). Additionally, advanced coursework might prioritize theoretical knowledge over practical application, resulting in a sense of disconnect and dissatisfaction among students who prefer hands-on learning experiences (Kolb, Citation2014).
Furthermore, the identified negative impact of not having a laptop on student satisfaction underscores the crucial role of technology access in the contemporary learning environment. This echoes the concerns raised by scholars like Lythreatis et al. (Citation2022), Ritzhaupt et al. (Citation2020), and Van Deursen and Van Dijk (Citation2019), who highlight the potential for the digital divide to exacerbate existing social and economic disparities, hindering access to resources and contributing to lower levels of student satisfaction and academic success.
Concerning the academic performance, the findings of this study reveal a robust and statistically significant positive association between student satisfaction and academic performance. This implies that increased satisfaction likely fosters stronger intrinsic motivation, fueling a desire for personal growth and mastery, and leading to higher academic performance (Ryan & Deci, Citation2017). Positive learning environments contribute to intrinsic motivation by fostering engagement and meaningful connections to the subject matter (Deci & Ryan, Citation2000). This corroborates the notion that a nurturing positive and engaging academic environment plays a critical role in optimizing student learning and facilitating their success. Furthermore, these findings are congruent with the works of Chigbu and Nekhwevha (Citation2021) and Kuh (Citation2009) who emphasize the importance of fostering a holistic learning environment that nurtures intellectual engagement, facilitates positive peer interactions, and cultivates supportive relationships with faculty. When students are satisfied with their environment and resources, they may be more likely to set ambitious learning goals and persist in achieving them, ultimately leading to improved performance (Latham & Locke, Citation2018; Locke & Latham, Citation2002). Such environments have been demonstrably instrumental in promoting both academic achievement and personal development among college students.
Beyond student satisfaction, the analysis identifies additional variables that exert a statistically significant positive influence on academic performance. Notably, SECSCOR emerges as one of the primary drivers of academic achievement. As anticipated, students with higher levels of cognitive ability demonstrate a tendency towards enhanced academic outcomes. This finding underscores the critical role that individual cognitive potential plays in educational attainment (Donnelly et al., Citation2016; Hanushek & Woessmann, Citation2012). Moreover, results also show that students enrolled in higher levels of study tend to have higher academic performance. This is likely due to their prior academic preparation and deeper engagement with their chosen field (Pascarella & Terenzini, Citation2005), and it seems that these students are highly motivated and engaged in their studies (An, Citation2015).
Interestingly, the analysis extends beyond identifying positive influences to reveal variables with a demonstrably negative impact on academic performance, namely the sex (SEX) and age (AGE). Female students within this sample demonstrate statistically significant lower academic performance compared to their male counterparts. This finding likely stems from a complex interplay of social, cultural, and educational factors, necessitating further investigation to elucidate the underlying mechanisms (Buchmann & Park, Citation2009). Similarly, results reveal an inverse association between age and academic performance, with older students exhibiting lower outcomes compared to younger students. This observation could be attributed to a variety of factors, including competing life demands, changes in learning styles with age, or potentially reduced access to academic support services (Pascarella & Terenzini, Citation2005).
Building upon the insights gained through regression analysis, the findings from the t-test add further weight to the notion that prior knowledge and experience in mathematics serve as a robust predictor of subsequent academic success among undergraduate economics students. This robust evidence reinforces the critical role of strong foundational mathematics skills in navigating the complexities of economic theory and quantitative analysis.
This observed association aligns with established research highlighting the pivotal role of foundational knowledge in promoting deeper understanding and cognitive development (Bransford et al., Citation2000). A strong foundation in mathematics equips students with essential skills and concepts, enabling them to navigate more complex mathematical problems and grasp abstract mathematical principles with greater ease (Björklund et al., Citation2020). Students with a strong foundation are better equipped to set challenging and achievable goals in mathematics, leading to increased motivation and effort (Locke & Latham, Citation2002). Additionally, prior success in mathematics can foster positive self-efficacy beliefs and intrinsic motivation towards the subject, leading to a more engaged and productive learning experience (Bandura, Citation2014a).
These findings have significant implications for educational practice. Firstly, they underscore the importance of fostering strong foundational skills in mathematics during early educational stages. Implementing effective instructional strategies that promote conceptual understanding and problem-solving abilities can help build a solid foundation for future academic success (Kilpatrick & Swafford, Citation2002). Secondly, the results highlight the need for targeted interventions and support systems for students without prior mathematics background. Providing additional resources, personalized instruction, and peer-tutoring programs can help bridge the knowledge gap and equip these students with the necessary skills to succeed in their academic endeavors (Slavin, Citation2018). Furthermore, diversifying teaching approaches to cater to different learning styles and prior knowledge levels can further enhance student engagement and learning outcomes. This could involve incorporating diverse instructional materials, utilizing technology tools effectively, and creating a supportive classroom environment that encourages collaboration and inquiry-based learning (Hattie, Citation2008).
While not explicitly addressed, socioeconomic disparities likely intertwine with the observed factors influencing student satisfaction and academic performance. The identified negative impact of lacking a laptop aligns with concerns about the digital divide, potentially disadvantaging students from underprivileged backgrounds with limited technological access (Van De Werfhorst et al., Citation2022; Warschauer & Tate, Citation2017). Similarly, increased pressure and workload at higher levels could disproportionately affect students lacking strong support systems or adequate resources often associated with lower socioeconomic circumstances (Camacho-Morles et al., Citation2021). The observed gender disparity in performance warrants further investigation, as socioeconomic inequities in educational opportunities, cultural expectations, or gender biases in learning environments could be underlying factors (Ceci et al., Citation2014). Finally, the importance of a prior mathematics background highlights the potential disadvantage faced by students from underprivileged backgrounds who may lack access to quality pre-university education or resources like private tutoring, perpetuating existing socioeconomic disparities in academic outcomes (Artigue et al., Citation2020). Addressing these concerns through initiatives like targeted interventions, accessible technology, and inclusive learning environments will be crucial to creating a more equitable and successful educational experience for all students, regardless of their socioeconomic background.
It is important to note that these findings are based on a specific study and may not be generalizable to all populations. However, they provide valuable insights into the multi-faceted nature of student performance. Understanding these factors is crucial for educational institutions to develop interventions and strategies to optimize learning and success for all students. This includes fostering a positive learning environment, providing tailored support based on individual needs and cognitive abilities, addressing socioeconomic disparities, and further exploring the complex factors influencing gender differences in academic achievement.
5. Conclusion
The present research investigated the determinants of student satisfaction and academic performance among undergraduate economics students. Several key findings emerged from the analysis.
A significant gender disparity was observed in student satisfaction, with females exhibiting higher levels than males. This finding aligns with existing research suggesting that females may possess stronger self-efficacy beliefs, intrinsic motivation, and a preference for collaborative learning environments, all of which contribute to enhanced satisfaction within educational settings. Conversely, the level of study and access to a laptop were found to exert a negative influence on student satisfaction. This can be attributed to the increased workload, complex coursework, and theoretical emphasis encountered at higher levels, which can lead to stress and frustration. Additionally, the lack of a personal laptop hinders access to valuable learning resources and limits engagement with online learning activities, further contributing to dissatisfaction.
On the other side, a robust and statistically significant positive association was observed between student satisfaction and academic performance. This finding accentuates the critical role of fostering a conducive and engaging academic environment in promoting optimal learning and student success. Additional variables exhibiting statistically significant positive effects on academic performance were score at secondary school completion (SECSCOR) and level of study, while gender and age exhibited negative associations. SECSCOR, a proxy for cognitive abilities, exerted a positive influence on academic performance. This underscores the crucial role of individual cognitive potential in educational attainment. The level of study also demonstrated a positive impact on academic performance, likely attributable to prior academic preparation and deeper engagement with the chosen field. Conversely, gender and age displayed a negative association with academic performance. Female students and older individuals within the sample exhibited lower academic performance compared to their counterparts. Further research is necessary to elucidate the underlying factors driving these disparities.
Furthermore, a comparison of academic performance between student groups with and without prior mathematics background revealed a significant association between prior knowledge and academic success. Students possessing such background demonstrated demonstrably higher annual performance compared to their counterparts without prior knowledge. This finding underscores the critical role of prior knowledge and experience in subsequent academic achievement.
These findings offer valuable insights into factors influencing student success in undergraduate economics programs. Addressing factors that contribute to student satisfaction, such as providing a supportive and collaborative learning environment, can positively impact academic performance. Additionally, recognizing the importance of individual cognitive abilities and prior knowledge can inform the development of tailored support programs and interventions to promote academic success for all students.
These findings offer valuable insights for educational institutions aiming to enhance student satisfaction and foster a more equitable learning environment. Consequently, the university institutions could consider (1) providing accessible technology resources and digital literacy training: which could help bridge the digital divide and ensure all students have the tools they need to succeed in the digital age; (2) cultivating supportive learning communities and personalized support systems: creating a sense of belonging and community can help mitigate the stress and isolation associated with higher levels of study; (3) employing diverse pedagogical approaches that cater to different learning styles and preferences: this can help address the concerns of students who prefer hands-on learning experiences or favor collaborative learning environments; and (4) implementing strategies to reduce the academic pressures associated with higher levels of study: This could involve providing more flexible learning arrangements, offering support services for stress management and time management, and fostering a culture of mentorship and peer support.
By addressing these issues, institutions can create a more inclusive and effective learning environment that cultivates student satisfaction and success. Further research is needed to delve deeper into the causal mechanisms underlying these relationships and to develop targeted interventions that address the specific needs and challenges faced by diverse groups of students. It is important to acknowledge that these findings are based on a single study and might not be generalizable to all university students from all disciplines. Further research with larger and more diverse samples is needed to confirm and extend these findings. Additionally, exploring the underlying mechanisms through which these factors influence student outcomes would provide valuable insights for educational practice and policy.
Drawing on this study’s findings and the broader literature, future research should prioritize: (1) investigating the gender disparity through qualitative methods to uncover underlying social, cultural, and educational factors, while exploring interventions addressing implicit biases and fostering inclusive learning environments; (2) mitigating higher-level study pressure by analyzing workload and stress contributors (course content, teaching methods, assessment practices) to inform targeted interventions promoting student well-being; (3) examining the long-term impact of prior mathematics background on academic performance and career outcomes beyond undergraduate economics, using longitudinal studies and evaluating interventions like remedial courses and personalized tutoring; (4) addressing the interplay between socioeconomic factors and student satisfaction/performance, analyzing how socioeconomic background interacts with other factors (gender, age, prior knowledge) to inform interventions and policies promoting educational equity and inclusivity; and (5) continuing to explore the effectiveness of diverse teaching methods and pedagogical approaches in fostering student engagement, satisfaction, and academic success within undergraduate economics education. These avenues hold promise for deepening our understanding of the multifaceted factors influencing student success and developing effective strategies to create equitable and successful learning environments for all.
Consent statement of the participants
Before the commencement of the interview, all potential participants were apprised of the voluntary nature of their participation and the anonymized utilization of their data for research purposes. This information was communicated verbally to guarantee clarity and understanding. Subsequently, the first question posed to each individual was a dichotomous choice: ‘Do you agree to participate in this interview?’ Only those who provided an affirmative response to this initial inquiry were allowed to proceed with the interview. This approach ensured that all participants entered the study with complete autonomy and full awareness of the research objectives and data usage.
Disclosure statement
No potential conflict of interest was reported by the author(s).
Data availability statement
No data were generated during this study.
Additional information
Funding
Notes on contributors

Aristide Maniriho
Dr. Aristide Maniriho, a lecturer in the Department of Economics at the University of Rwanda, brings a multifaceted expertise to the field of agricultural and development economics. His PhD from the University of Liege—Gembloux Agro-Bio Tech (Belgium) in December 2021 solidified his core research focus, encompassing not only agricultural economics but also environmental and natural resource economics, and food economics. His secondary interests in university pedagogy of economics and international economics showcase a nuanced understanding of the interconnectedness of these factors in driving agricultural and rural development. Notably, Dr. Maniriho’s passion and his commitment to participatory learning and teaching methods infuse his research and pedagogical approaches, demonstrating a dedication to fostering sustainable and inclusive development in the agricultural sector and further enriching his multifaceted academic profile.
References
- Agresti, A. (2018). An introduction to categorical data analysis (Vol. 656). John Wiley & Sons.
- Ahmad, M. I. S., Idrus, M. I., & Rijal, S. (2023). The role of education in fostering entrepreneurial spirit in the young generation. Journal of Contemporary Administration and Management, 1(2), 93–100. https://doi.org/10.61100/adman.v1i2.28
- An, B. P. (2015). The role of academic motivation and engagement on the relationship between dual enrollment and academic performance. The Journal of Higher Education, 86(1), 98–126. https://doi.org/10.1080/00221546.2015.11777358
- Artigue, M., Bosch, M., Doorman, M., Juhász, P., Kvasz, L., & Maass, K. (2020). Inquiry based mathematics education and the development of learning trajectories. Teaching Mathematics and Computer Science, 18(3), 63–89. https://doi.org/10.5485/TMCS.2020.0505
- Astin, A. W. (1999). Student involvement: A developmental theory for higher education. Journal of College Student Development, 40(5), 518–529.
- Bandura, A. (2014a). Exercise of personal agency through the self-efficacy mechanism. In Self-efficacy (pp. 3–38). Taylor & Francis.
- Bandura, A. (2014b). Social cognitive theory of moral thought and action. In Handbook of moral behavior and development (pp. 69–128). Psychology Press.
- Benos, N., & Zotou, S. (2014). Education and economic growth: A meta-regression analysis. World Development, 64, 669–689. https://doi.org/10.1016/j.worlddev.2014.06.034
- Björklund, C., van den Heuvel-Panhuizen, M., & Kullberg, A. (2020). Research on early childhood mathematics teaching and learning. ZDM Mathematics Education, 52(4), 607–619. https://doi.org/10.1007/s11858-020-01177-3
- Bransford, J. D., Brown, A. L., & Cocking, R. R. (2000). How people learn (Vol. 11). National Academy Press.
- Breen, R., Karlson, K. B., & Holm, A. (2018). Interpreting and understanding logits, probits, and other nonlinear probability models. Annual Review of Sociology, 44(1), 39–54. https://doi.org/10.1146/annurev-soc-073117-041429
- Bronfenbrenner, U. (2013). Ecology of the family as a context for human development: Research perspectives. Adolescents and Their Families, 2013, 1–20.
- Brown, A. L., & Palincsar, A. S. (2018). Guided, cooperative learning and individual knowledge acquisition. In Knowing, learning, and instruction (pp. 393–451). Routledge.
- Buchmann, C., & Park, H. (2009). Stratification and the formation of expectations in highly differentiated educational systems. Research in Social Stratification and Mobility, 27(4), 245–267. https://doi.org/10.1016/j.rssm.2009.10.003
- Camacho-Morles, J., Slemp, G. R., Pekrun, R., Loderer, K., Hou, H., & Oades, L. G. (2021). Activity achievement emotions and academic performance: A meta-analysis. Educational Psychology Review, 33(3), 1051–1095. https://doi.org/10.1007/s10648-020-09585-3
- Ceci, S. J., Ginther, D. K., Kahn, S., & Williams, W. M. (2014). Women in academic science: A changing landscape. Psychological Science in the Public Interest, 15(3), 75–141. https://doi.org/10.1177/1529100614541236
- Cheong, K. C., & Ong, B. (2016). An evaluation of the relationship between student engagement, academic achievement, and satisfaction. In Assessment for Learning Within and Beyond the Classroom: Taylor’s 8th Teaching and Learning Conference 2015 Proceedings (pp. 409–416). Springer Singapore.
- Chigbu, B. I., & Nekhwevha, F. H. (2021). High school training outcome and academic performance of first-year tertiary institution learners-taking’ input-environment-outcomes model’ into account. Heliyon, 7(7), e07700. https://doi.org/10.1016/j.heliyon.2021.e07700
- Csikszentmihalhi, M. (2020). Finding flow: The psychology of engagement with everyday life. Hachette UK.
- Deci, E. L., & Ryan, R. M. (2000). The “what” and “why” of goal pursuits: Human needs and the self-determination of behavior. Psychological Inquiry, 11(4), 227–268. https://doi.org/10.1207/S15327965PLI1104_01
- Donnelly, J. E., Hillman, C. H., Castelli, D., Etnier, J. L., Lee, S., Tomporowski, P., Lambourne, K., & Szabo-Reed, A. N. (2016). Physical activity, fitness, cognitive function, and academic achievement in children: A systematic review. Medicine and Science in Sports and Exercise, 48(6), 1197–1222. https://doi.org/10.1249/MSS.0000000000000901
- Eccles, J. S., & Wigfield, A. (2002). Motivational beliefs, values, and goals. Annual Review of Psychology, 53(1), 109–132. https://doi.org/10.1146/annurev.psych.53.100901.135153
- Eccles, J. S., & Wigfield, A. (2020). From expectancy-value theory to situated expectancy-value theory: A developmental, social cognitive, and sociocultural perspective on motivation. Contemporary Educational Psychology, 61, 101859. https://doi.org/10.1016/j.cedpsych.2020.101859
- Galbraith, C. S., & Merrill, G. B. (2012). Predicting student achievement in university-level business and economics classes: Peer observation of classroom instruction and student ratings of teaching effectiveness. College Teaching, 60(2), 48–55. https://doi.org/10.1080/87567555.2011.627896
- Government of Rwanda (2017). National Strategy for Transformation (NST1) 2017–2024. Ministry of Finance and Economic Planning.
- Guskey, T. R., & Brookhart, S. M. (2019). What we know about grading: What works, what doesn’t, and what’s next. ASCD.
- Hair, J. F., Black, W. C., Babin, B. J., & Anderson, R. E. (2010). Multivariate data analysis (7th ed.). Prentice Hall.
- Hanushek, E. A., & Woessmann, L. (2012). Do better schools lead to more growth? Cognitive skills, economic outcomes, and causation. Journal of Economic Growth, 17(4), 267–321. https://doi.org/10.1007/s10887-012-9081-x
- Hattie, J. (2008). Visible learning: A synthesis of over 800 meta-analyses relating to achievement. Routledge.
- Heutte, J., Fenouillet, F., Martin-Krumm, C., Gute, G., Raes, A., Gute, D., Bachelet, R., & Csikszentmihalyi, M. (2021). Optimal experience in adult learning: conception and validation of the flow in education scale (EduFlow-2). Frontiers in Psychology, 12, 828027. https://doi.org/10.3389/fpsyg.2021.828027
- Hopland, A. O., & Nyhus, O. H. (2015). Does student satisfaction with school facilities affect exam results? An empirical investigation. Facilities, 33(13/14), 760–774. https://doi.org/10.1108/F-09-2014-0076
- Hu, Y. L., Hung, C. H., & Ching, G. S. (2014). Student-faculty interaction: Mediating between student engagement factors and educational outcome gains. International Journal of Research Studies in Education, 4(1), 43–53. https://doi.org/10.5861/ijrse.2014.800
- Hulleman, C. S., Barron, K. E., Kosovich, J. J., & Lazowski, R. A. (2016). Student motivation: Current theories, constructs, and interventions within an expectancy-value framework. In A. A. Lipnevich, F. Preckel, & R. D. Roberts (Eds.), Psychosocial skills and school systems in the 21st century: Theory, research, and practice (pp. 241–278). Springer.
- Iliyasu, R., & Etikan, I. (2021). Comparison of quota sampling and stratified random sampling. Biometrics & Biostatistics International Journal, 10(1), 24–27. https://doi.org/10.15406/bbij.2021.10.00326
- Karch, J. (2021). Choosing between the two-sample t-test and its alternatives: A practical guideline.
- Khadka, J. (2019). Sampling error in survey research. International Journal of Scientific Research, 8, 2214–2220.
- Khan, J., & Iqbal, M. J. (2016). Relationship between student satisfaction and academic achievement in distance education: A case study of AIOU Islamabad. FWU Journal of Social Sciences, 10(2), 137.
- Kilpatrick, J., & Swafford, J. (2002). Helping children learn mathematics. National Academies Press.
- Kirmizi, Ö. (2015). The influence of learner readiness on student satisfaction and academic achievement in an online program at higher education. Turkish Online Journal of Educational Technology, 14(1), 133–142. Retrieved from https://files.eric.ed.gov/fulltext/EJ1057353.pdf
- Kolb, D. A. (2014). Experiential learning: Experience as the source of learning and development. FT Press.
- Kuh, G. D. (2009). The national survey of student engagement: Conceptual and empirical foundations. New Directions for Institutional Research, 2009(141), 5–20. https://doi.org/10.1002/ir.283
- Kuh, G. D., Kinzie, J. L., Buckley, J. A., Bridges, B. K., & Hayek, J. C. (2006). What matters to student success: A review of the literature (Vol. 8). National Postsecondary Education Cooperative.
- Kuh, G. D., Kinzie, J., Schuh, J. H., & Whitt, E. J. (2010). Student success in college (includes new preface and epilogue): Creating conditions that matter. John Wiley & Sons.
- Latham, G. P., & Locke, E. A. (2018). Goal setting theory: Controversies and resolutions. In The SAGE handbook of industrial, work & organizational psychology: Organizational psychology (Vol. 2, pp. 145–166). Sage.
- Liu, F., Li, L., Zhang, Y., Ngo, Q. T., & Iqbal, W. (2021). Role of education in poverty reduction: Macroeconomic and social determinants form developing economies. Environmental Science and Pollution Research International, 28(44), 63163–63177. https://doi.org/10.1007/s11356-022-19563-7
- Locke, E. A., & Latham, G. P. (2002). Building a practically useful theory of goal setting and task motivation: A 35-year odyssey. The American Psychologist, 57(9), 705–717. https://doi.org/10.1037/0003-066X.57.9.705
- Lythreatis, S., Singh, S. K., & El-Kassar, A. N. (2022). The digital divide: A review and future research agenda. Technological Forecasting and Social Change, 175, 121359. https://doi.org/10.1016/j.techfore.2021.121359
- May-Varas, S., Margolis, J., & Mead, T. (2023). Bioecological model of human development. In J. Margolis, S. May-Varas, & T. Mead (Eds.), Educational learning theories. Education Open Textbooks.
- Mihanović, Z., Batinić, A. B., & Pavičić, J. (2016). The link between students’ satisfaction with faculty, overall students’ satisfaction with student life and student performances. Review of Innovation and Competitiveness, 2(1), 37–60. https://doi.org/10.32728/ric.2016.21/3
- MINEDUC (2023). 2021/22 Education statistical yearbook – School year ended in July 2023. Ministry of Education (Rwanda).
- Okolie, U. C., Mlanga, S., Oyerinde, D. O., Olaniyi, N. O., & Chucks, M. E. (2022). Collaborative learning and student engagement in practical skills acquisition. Innovations in Education and Teaching International, 59(6), 669–678. https://doi.org/10.1080/14703297.2021.1929395
- Okoye, K., Nganji, J. T., Escamilla, J., Fung, J. M., & Hosseini, S. (2022). Impact of global government investment on education and research development: A comparative analysis and demystifying the science, technology, innovation, and education conundrum. Global Transitions, 4, 11–27. https://doi.org/10.1016/j.glt.2022.10.001
- Pascarella, E. T., & Terenzini, P. T. (2005). How college affects students: A third decade of research (Vol. 2). Jossey-Bass Publishers.
- Ritzhaupt, A. D., Cheng, L., Luo, W., & Hohlfeld, T. N. (2020). The digital divide in formal educational settings: The past, present, and future relevance. In M.J. Bishop, E. Boling, J. Elen & V. Svihla. (Eds.), Handbook of research in educational communications and technology: Learning design (pp. 483–504). Cham: Springer https://doi.org/10.1007/978-3-030-36119-8_23
- Roach, T. (2014). Student perceptions toward flipped learning: New methods to increase interaction and active learning in economics. International Review of Economics Education, 17, 74–84. https://doi.org/10.1016/j.iree.2014.08.003
- Ryan, R. M., & Deci, E. L. (2017). Self-determination theory: Basic psychological needs in motivation, development, and wellness. Guilford Publications.
- Schunk, D. H. (2012). Learning theories: An educational perspective. Pearson Education, Inc.
- Schunk, D. H., & DiBenedetto, M. K. (2020). Motivation and social cognitive theory. Contemporary Educational Psychology, 60, 101832. https://doi.org/10.1016/j.cedpsych.2019.101832
- Sekaran, U., & Bougie, R. (2016). Research methods for business: A skill-building approach (7th ed.). John Wiley & Sons.
- Slavin, R. E. (2018). Educational psychology: Theory and practice (12th ed.). Pearson Education.
- Sweller, J. (2011). Cognitive load theory. In Psychology of learning and motivation (Vol. 55, pp. 37–76). Academic Press.
- Szulewski, A., van Gog, T., Paas, F., & Sweller, J. (2023). Cognitive load theory: Researching and planning teaching to maximise learning. In J. Cleland & S. J. Durning (Eds.), Researching medical education (2nd ed., pp. 303–314). John Wiley & Sons, Inc.
- Tinto, V. (2012). Leaving college: Rethinking the causes and cures of student attrition. University of Chicago Press.
- Tudor, T. R. (2018). Fully integrating academic advising with career coaching to increase student retention, graduation rates and future job satisfaction: An industry approach. Industry and Higher Education, 32(2), 73–79. https://doi.org/10.1177/0950422218759928
- Van De Werfhorst, H. G., Kessenich, E., & Geven, S. (2022). The digital divide in online education: Inequality in digital readiness of students and schools. Computers and Education Open, 3, 100100. https://doi.org/10.1016/j.caeo.2022.100100
- Van Deursen, A. J., & Van Dijk, J. A. (2019). The first-level digital divide shifts from inequalities in physical access to inequalities in material access. New Media & Society, 21(2), 354–375. https://doi.org/10.1177/1461444818797082
- Van Elst, H. (2019). Foundations of descriptive and inferential statistics. Lecture notes for a quantitative-methodological module at the bachelor degree (B.Sc) level. Köln: parcIT GmbH. E-Print: arXiv:1302.2525v4 [stat.AP].
- Warschauer, M., & Tate, T. (2017). Digital divides and social inclusion. In K. A. Mills, A. Stornaiuolo, A. Smith, & J. Z. Pandya (Eds.), Handbook of writing, literacies, and education in digital cultures (pp. 63–75). Routledge.
- Whitt, E. J., Schuh, J. H., Kinzie, J., & Kuh, G. D. (2013). Student success in college: Creating conditions that matter. Jossey-Bass.
- Wooldridge, J. M. (2010). Econometric analysis of cross section and panel data. MIT Press.