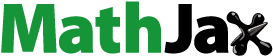
Abstract
Previous studies have designed educational methods to cultivate digital citizenship behavior and support the construction of knowledge. However, these methods have not well incorporated personalized feedback mechanisms for enhancing digital citizenship knowledge. Therefore, this study proposed an algorithm that combines concept-effect propagation, fuzzy logic, and decision tree methods to address this drawback and create a personalized, contextual gaming experience. This personalization ensures an engaging and contextually relevant learning experience, addressing learning challenges related to digital citizenship scales. The game was tailored to individual learning experiences and decision-making patterns, with fuzzy logic interpreting nuanced student responses and decision trees guiding learning paths. A digital citizenship knowledge test and an affection questionnaire measured the game’s impact. Moreover, eye tracking was used to ensure attention in the experimental group. Therefore, a quasi-experimental design was conducted to evaluate the influence of a digital citizenship game on 110 students. ANCOVA and the Chi-square tests were performed to analyze students’ knowledge of digital citizenship. Moreover, eye-tracking metrics were used to gain deeper insights into students’ visual attention and engagement. The experimental results reveal that the proposed game enhanced the students’ digital citizenship achievement and promoted their perceptions. Additionally, eye-tracking data showed that the proposed gaming environment positively influenced students’ engagement. Findings indicate that using fuzzy logic and decision trees in educational games significantly promotes affection and alters attention in learning digital citizenship. This study contributes to educational technology by showcasing the potential benefits of personalized educational experiences. The insights gained are valuable for educators and educational game developers focused on digital citizenship education.
Introduction
Digital citizenship education, vital for equipping individuals with the skills for ethical technology use, has incorporated competencies such as online safety and media literacy. The consensus in literature underscored the need for a multifaceted approach, advocating for customization to meet diverse learner needs and challenges (Ali et al., Citation2023; Duran, Citation2022). Emphasizing integration, Li et al. (Citation2023) suggested embedding digital citizenship across disciplines to foster holistic understanding. Law et al. (Citation2018) suggested updating norms to reflect modern digital realities essential for ethical engagement with technology. Christensen et al. (Citation2021) explored civic engagement through social media as a means of societal contribution, highlighting the empowering potential of digital citizenship. Mattson and Curran (Citation2017) recommended ongoing refinement in teaching methods to keep pace with digital advancements, ensuring continued relevance and efficacy in digital citizenship education. Moreover, various resources were available for teaching digital citizenship, such as Be Internet Awesome by Google, Book Creator, BrainPOP, Common Sense Media, and Nearpod, each offering specific lessons and activities focused on different aspects of digital citizenship. There has been a call for more research on digital citizenship frameworks that specifically address the gaps between theory and practice in education. This suggested that current models may not be adequately grounded in practical, classroom-based realities or may not effectively translate theoretical concepts into actionable teaching strategies (Vajen et al., Citation2023). Prior research in this area has primarily focused on knowledge construction and cultivating digital citizenship behaviors in educational settings. However, these approaches often lack individualized feedback mechanisms, limiting their effectiveness in addressing diverse learning needs. Therefore, incorporating activities and tools that promote independent learning and engagement in digital citizenship is crucial. This could make the learning experience more authentic and meaningful.
Recently, while digital games could be a valuable tool in engaging students, these potential challenges needed to be carefully considered and addressed to ensure they were a beneficial component of digital citizenship education. That is to say, educational games offered a practical way for students to learn about digital citizenship, allowing them to experiment and learn by doing. However, this approach might require supplementary educational materials and mentorship to fully grasp digital citizenship’s complexities. It also indicated a gap in many digital citizenship curricula, where the focus on technical skills and safety might not adequately prepare students for active, meaningful participation in the digital world. Tapingkae et al. (Citation2020) study focusing on a formative assessment-based contextual gaming approach in digital citizenship education revealed challenges associated with integrating digital games in teaching complex and dynamic concepts as digital citizenship. The effectiveness of the gaming approach could vary significantly based on factors like age, cultural background, previous gaming experience, and individual learning characteristics. However, the point of formative assessments in gaming contexts may depend on how well the game adapts to individual learning paths corresponding to decision-tree storytelling. The educational value could be compromised if the adaptation mechanisms were not sophisticated or responsive enough. With the benefits of the Fuzzy rule, several studies applied its elements to developing online learning systems (Hwang et al., Citation2020; Ingkavara et al., Citation2022; Panjaburee et al., Citation2022; Wanichsan et al., Citation2021; Wongwatkit et al., Citation2017). That is to say, one of the key advantages of using the Fuzzy rule is its ability to provide personalized feedback. The online learning systems could analyze a student’s performance and learning status to offer tailored advice or resources, enhancing the learning experience. It could help in creating adaptive learning paths for students. Based on a student’s learning status diagnosis, the system can modify the gaming environment or the instructional content to suit the individual’s learning needs (Komalawardhana et al., Citation2021). Therefore, Tapingkae et al. (Citation2020) have suggested enhancing the decision tree-based gaming approach for teaching digital citizenship by incorporating additional mechanisms. Specifically, integrating Fuzzy logic into the decision tree-based gaming environment could improve its effectiveness by offering more personalized and meaningful feedback. This adjustment aims to enrich students’ learning experiences and outcomes by making the gaming approach more responsive to their needs and contexts.
Additionally, online learning systems have been investigated by scholars that they could potentially improve student engagement and motivation through immediate and personalized feedback. For example, Clark et al. (Citation2016) highlight the educational effectiveness of digital games, noting the importance of feedback mechanisms. Similarly, Van der Kleij et al. (Citation2015) and Plass et al. (Citation2015) emphasize the significance of timely and personalized feedback in improving student performance and sustaining interest. Hamari et al. (Citation2016) further confirm the motivational benefits of educational games, attributing a crucial role to personalized feedback in engaging students. In addition, scholars have recognized that using eye-tracking technology could provide a comprehensive and nuanced understanding of students’ behaviors, perceptions, and cognitive processes during interacting with digital materials (Cheng et al., Citation2024; Yang & Wang, Citation2023). Therefore, the combination of eye-tracking technology with self-report surveys offers a robust method for gathering objective (eye movement data) and subjective (self-reported perceptions and attitudes) data, leading to a more comprehensive understanding of participant behavior and attitudes in research studies.
Accordingly, this is the first empirical into using an AI-driven gaming environment, employing a concept-effect propagation-oriented method, fuzzy logic, and decision trees to diagnose students’ digital citizenship behavior status and provide personalized feedback. It attempted to address the gaps in digital citizenship education by enhancing the learning experience through personalization and engagement tailored to individual learning paths. Specifically, the study aimed to answer the following three research questions:
Do students who receive the fuzzy logic and decision tree-based personalized gaming approach have better knowledge of digital citizenship than those who receive a decision tree-based gaming approach?
How did the fuzzy logic and decision tree-based personalized gaming approach impact students’ affections compared to those who received a decision tree-based gaming approach?
How does a fuzzy logic and decision tree-based personalized gaming environment influence students’ visual attention?
The investigation-worthy research questions were to empirically examine the efficacy of the proposed AI-driven gaming approach in enhancing digital citizenship knowledge, affecting student affection, and influencing visual attention. Moreover, this study’s contributions are improving digital citizenship knowledge, positively affecting student affection, and enhancing visual attention. That is to say, it contributes to the body of knowledge on educational technology and digital citizenship, offering valuable insights for educators and researchers interested in leveraging technology to improve educational outcomes.
Related works
Digital citizenship education
Digital citizenship, vital in modern education, lacks a universally agreed-upon definition but is broadly explored across disciplines due to the growth of digital technology (Chen et al., Citation2021). It is variously understood as promoting online respectful behavior and civic engagement, aligning with broader citizenship goals to foster responsible, participatory behavior in digital spaces (Tadlaoui-Brahmi et al., Citation2022). Additionally, digital citizenship involves proactive engagement in virtual environments, emphasizing active participation and empowerment in the digital realm (Ribble, Citation2015). It is also seen as encompassing competencies like critical thinking and safe, responsible digital world participation relevant in education for framing teachable skills and attitudes (Lauricella et al., Citation2020). Furthermore, digital citizenship encompasses the safe, legal, ethical, and responsible use of computer and communication technologies (Ribble, Citation2011). Educationally, it aims to foster proper technology use and prevent cyberbullying and harassment. The importance of teaching appropriate online communication and cultivating good online behaviors from a young age is highlighted, focusing on reducing cyberbullying and increasing responsible online interaction (Jones & Mitchell, Citation2016). Addressing cyberbullying in children and secondary school students is critical (Sittichai, Citation2013; Tokunaga, Citation2010), along with enhancing digital literacy and citizenship for meaningful technology use (Ng, Citation2012). Therefore, in education, these definitions underscore the importance of teaching students how to use digital technology and how to do so responsibly, ethically, and effectively as part of a larger digital society. This includes instilling values of respect, responsibility, and active participation in the digital world and developing competencies that enable them to navigate and contribute positively to the digital landscape.
Achieving digital citizenship involves diverse teaching and learning methods. Competence-focused approaches center on digital skills and norms, while participation-focused methods emphasize social and political engagement through digital means (Chen et al., Citation2021). Various models and resources have been proposed for teaching digital citizenship (Bolkan, Citation2014; Common Sense Media, Citation2016; d‘Haenens et al., Citation2007; Fredrick, Citation2013; Hollandsworth et al., Citation2017; James et al., Citation2009; Jones & Mitchell, Citation2016; Prensky, Citation2004; Ribble et al., Citation2004; Sanders et al., Citation2016; Searson et al., Citation2015; Thompson, Citation2013; Verma et al., Citation2016), including digital games for promoting learning and engagement (Blevins et al., Citation2014; Hill, Citation2015). The CRISS H2020 platform is used in Europe for developing and certifying digital competencies through ICT-supported scenarios (Maina et al., Citation2020). Addressing gender issues in social media is also crucial for digital citizenship (Wachs et al., Citation2016). Although Tapingkae et al. (Citation2020) study demonstrated the success of using the formative assessment-based contextual gaming approach to promote good digital citizenship behavior. It is still questioning about creating appropriate instruction for individual students through the gaming approach (Komalawardhana et al., Citation2021).
Personalized learning through gaming
Digital game-based learning, using games for educational purposes, enhances students’ learning performance, cognitive abilities, and attitudes (Cohen, Citation2014; Hsiao et al., Citation2014; Papadakis & Kalogiannakis, Citation2019; Schaaf, Citation2012; Yang et al., Citation2022). It boosts learning motivation and engagement (Khan et al., Citation2017; Ronimus et al., Citation2014; Yu et al., Citation2021) and uses in-game analytics for assessment (Kiili et al., Citation2018; Kim & Shute, Citation2015; Yang & Lu, Citation2021). These games, implemented across subjects and education levels (Chang & Hwang, Citation2019), facilitate interactive learning and prosocial behavior (Fang et al., Citation2022; Saleme et al., Citation2021). However, effectiveness varies with individual factors, such as gender and prior knowledge (Tsai et al., Citation2012; Tsai, Citation2017; Yang & Chen, Citation2020), suggesting a need for AI-driven adaptability and personalization in game design.
Decision trees, a data mining approach with a flowchart-like structure, effectively classify variables into a visual model, mirroring human cognitive processes (Patel & Prajapati, Citation2018). They were favored in AI for their accuracy and simplicity. Applied in various fields like stock trading (Wu et al., Citation2006), medicine (Podgorelec et al., Citation2002), and policy-making during COVID-19 (Karnon, Citation2020), decision trees are also widespread in education. They helped learn analytics and understand student patterns (Gomes & Almeida, Citation2017; Priyam et al., Citation2013; Rizvi et al., Citation2019). For instance, they have been used in physical education (Chen & Hung, Citation2010) to analyze university enrolment trends (Paideya & Bengesai, Citation2021) and provide personalized learning (Kurilovas, Citation2019). and guide students in digital citizenship games (Tapingkae et al., Citation2020), effectively addressing individual learning needs.
Although decision trees, useful in classifying variables into visual models, can support this personalization in digital game-based learning. Their ability to analyze and understand student patterns can be integrated into educational games (Zhong, Citation2022); however, providing tailored feedback based on individual learning is still needed and challenging to create in the AI-driven gaming approach. In other words, decision trees have offered some potential in the context of personalized digital game-based learning. They can provide customized feedback based on individual student profiles and learning patterns by integrating their analytical capabilities into educational games. However, implementing this personalized approach in AI-driven educational games has presented challenges. The complexity of accurately capturing and responding to the nuances of individual learning preferences requires sophisticated algorithmic design and constant refinement to ensure that the games are engaging and educationally effective. This necessitates a deeper understanding of student behaviors, learning outcomes, and the dynamic nature of educational gaming environments to create more effectively personalized learning experiences.
Fuzzy logic and decision tree-based personalized gaming approach
The study was a 2D role-playing game for digital citizenship education. It incorporated various scenarios to enhance learning through decision-making elements. The game, with text, images, animation, and narration, was adapted to student’s understanding of digital citizenship conceptions. Moreover, this current study proposed a Fuzzy rule-based approach for immediately providing personalized feedback on digital citizenship behaviors, aiming to boost student engagement and motivation.
The fuzzy logic and decision tree-based personalized gaming system uses HTML5 and MySQL. It consists of a gaming module, a testing model, a learning status recording model, and an expert system module, as shown in . The gaming module allows students to play contextual games corresponding to storytelling and make decisions to reveal their good or bad decisions. The testing model is used to ensure individual students’ knowledge. The learning status recording model records the individual decision-making paths corresponding to the digital citizenship scale in the system logs for further analysis. The expert system module is used to analyze digital citizenship status and to determine the gaming scenario for individual students based on their knowledge and decision path.
With digital citizenship education by Common Sense Media (Citation2016) curriculum (ie cyberbully or be upstanding, safe online talk, reality of digital drama, and cyberbullying with crossing the line), the storytelling for each aspect was created to serve a well-crafted narrative, which could deeply immerse players in the game story, making them feel like they are a part of that story regarding digital citizenship, as shown in . This can be achieved through compelling characters, an engaging plot, and a richly detailed setting. Storytelling also allows players to form connections with the characters in the game, making the characters’ successes and failures more impactful for the player. Therefore, the contextual game’s decision tree corresponding to the storytelling was used to guide the students to make decisions during the gaming process. As shown in , the decision tree included the gaming scenarios (decision to be made), the decision (good or bad decision), and the gaming results corresponding to the player’s decision. Moreover, facial emotion was used to reflect decision results (ie a happy face emoji with a good decision and a sad face emoji with a good decision), as shown in . When the players make inadequate or bad decisions, the gaming system will direct them to corresponding scenarios to fix the problems and revise their decisions.
The fuzzy logic and decision tree-based personalized gaming method applied a concept-effect propagation approach to diagnose individual students’ problems of digital citizenship behaviors and provide corresponding behavioral status as follows.
Step 1: Applying a concept effect-oriented approach (Srisuwan & Panjaburee, Citation2020) to construct a digital citizenship concept-effect propagation table (CEPT) based on a concept-effect relationship diagram. The CEPT records all storytelling scenarios (SS) associated with each digital citizenship scale (DCS). Considering , CEPT (SS1, DCS1) = 1, meaning that SS1 is one of the storytelling scenarios associated with DCS1. Otherwise, CEPT (SS1, DCS2) = 0, meaning that SS1 is not storytelling scenario associated with DCS2. That is, SS1: the story of cyberbullying or being upstanding with DCS1: the online respectful behavior, but DCS2: the online civic engagement scale.
Table 1. An example of concept-effect propagation table of digital citizenship behavior (CEPT).
Step 2: For each gaming scenario, teachers constructed the test items covering the conceptions of each storytelling scenario. The table of test item- storytelling scenario relationships (TIRT) is constructed to represent the degree of association between the test item and storytelling scenario, in which 0 refers to no relationship, and 1 refers to a strong relationship, as shown in . In addition, an answer sheet table (AST) is used to record a value of answers given by individual students, in which 0 indicates that student Stdk answered test item Qn correctly, 1 indicates that student Stdk answered test item Qn incorrectly, as shown in .
Table 2. An example of associations between test items and storytelling scenarios (TIRT).
Table 3. An example of answers from individual students (AST).
Step 3: Diagnosing student digital citizenship problems with Matrix Composition is calculated by relationships among CEPT, TIRT, and AST data sets. The max-min composition method is adopted to derive the error degree for individual students regarding each digital citizenship scale. The calculation of error degree is error_degree(Stdk, DCSi) = AST(Stdk, Qn) ο TIRT(Qn, SSj) ο CEPT(SSj, DCSi), as flowing steps:
Step 3.1: The max-min composition method was adopted to elicit the error_degree of AST(Std1, Qn), where n = 1 to 4, and TIRT(Qn, SSj), where j = 1 to 4:
(1)
(1)
Step 3.2: Accordingly, the max-min composition method was repeated to calculate the error_degree of Equation(1)(1)
(1) and CEPT(SSj, DCS1), where j = 1 to 4:
Such that, for individual students, a vector is obtained to indicate the failure of correct answer degree for each digital citizenship scale: error_degree(Stdk, DCSi) based on the results of the max-min composition method as Equation(2)(2)
(2)
(2)
(2)
Step 4: Generating the status of the digital citizenship scale for individual students is provided by performing an error degree of each digital citizenship scale using fuzzy inference. The membership functions for LOW, AVERAGE, and HIGH error_degree are defined as follows (Ingkavara et al., Citation2022):
LOW (X = error_degree(Stdk, DCSi)), where k = Student 1 to n; i = DCS 1 to n:
AVERAGE (X = error_degree(Stdk, DCSi)), where k = Student 1 to n; i = DCS 1 to n:
HIGH (X = error_degree(Stdk, DCSi)), where k = Student 1 to n; i = DCS 1 to n:
Accordingly, the corresponding fuzzy rules for determining digital citizenship behavior_status are given as follows:
If error_degree(DCSi) is HIGH, then digital citizenship behavior_status(DCSi) is Poorly-performed;
If error_degree(DCSi) is AVERAGE, then digital citizenship behavior_status(DCSi) is Partially-performed;
If error_degree(DCSi) is LOW, then digital citizenship behavior_status(DCSi) is Well-performed.
Assuming an error_degree(Std1, DCS3) = 0.7, by using the fuzzification operations, the following fuzzy input values will be analyzed:
By applying the fuzzy implication operation, the outputs will be as follows:
digital citizenship behavior_status(Std1, DCS3) is Poorly-performed with degree 0.82;
digital citizenship behavior_status(Std1, DCS3) is Partially-performed with degree 0.68;
digital citizenship behavior_status(Std1, DCS3) is Well-performed with degree 0.18.
Consequently, the maximum membership defuzzification is used to interpret the final outcome, digital citizenship behavior_status(Std1, DCS3) is poorly performed with degree 0.82.
Step 5: Calculating the suitable digital citizenship diagnosis for individual students by obtaining the data of decision path logs in the gaming system. Two variables are employed for digital citizenship diagnosis for individuals: GBH refers to good decision-making count, and BBH refers to bad decision-making count. The results and comments of the digital citizenship diagnosis are shown in by integrating them with the digital citizenship behavior status.
Table 4. The results and comments of the digital citizenship diagnosis.
Such that, in this case, the student Std1 is Poorly-performed DCS3 with a degree of 0.82 and assuming that GBH-BBH < 0, then the student is guided to seriously repeat playing the games relevant to storytelling containing the digital citizenship DCS3 (ie SS1, SS2, SS3, SS4), as shown in the CEPT in , and ask the teacher to help you find out what caused you, as shown in . That is to say, the gaming system will automatically navigate the student to repeat playing in SS1, SS2, SS3, and SS4.
Research methodology
This study employed a quasi-experimental design. All experimental procedures performed in this study involving human participants followed the ethical standards of the committee for research ethics and with comparable ethical standards.
Participants and experimental procedure
A total of 110 eighth Thai-graders were recruited to participate in this study. The average age of the participants was 14. They were divided into two groups where the 57 students in the experimental group received the fuzzy logic and decision tree-based personalized gaming approach. The 53 students in the control group participated in the decision tree-based gaming approach without fuzzy logic and personalized learning manners. Regarding research ethics clearance guidelines, participants were explained on the investigation activities and provided informed consent, with assurance that their names and identifying information would remain confidential.
The students completed a 10-minute introduction to digital citizenship education and learning goals and activities. Afterward, for 4 d (a total of 160 min), the students in the experimental group learned digital citizenship with the fuzzy logic and decision tree-based personalized gaming approach as the in-class learning activity with the teacher as facilitator, while those in the control group learning digital citizenship with the conventional approach, that is the decision tree-based gaming approach without the fuzzy logic and personalized learning manners with the teacher playing the main role of providing learning guidance and feedback. Both groups completed a similar worksheet after the learning activities. The students then took a knowledge test of digital citizenship and filled out the affection questionnaire, including perceptions and learning motivation, which took 40 min. Moreover, the volunteered students in the experimental group were conducted with the eye-tracking instrument to ensure their visual attention during game playing.
Measuring tool
An affection questionnaire was used to investigate the students’ learning perceptions and motivations after participating in the learning approach. Regarding the Tapingkae et al. (Citation2020) study, the affection questionnaire had six psychological constructs. The questionnaire was adopted from technology acceptance perceptions (Teo et al., Citation2009) and the Science Motivation Questionnaire (Glynn & Koballa, Citation2006). It consists of 22 items with a 5-point Likert rating scale ranging from 1 (strongly disagree) to 5 (strongly agree). Perceived usefulness and perceived ease of use constructs reflect students’ beliefs about the learning approach’s impact on performance and ease of use. Attitude construct indicates their satisfaction with learning activities. Intention to use construct shows their willingness to use the approach in the future. The intrinsic motivation construct relates to undertaking tasks for internal rewards, and the self-efficacy construct describes their belief in their ability to succeed in specific situations or tasks. The Cronbach’s alpha value of the questionnaire in the Thai version was .94, showing acceptable reliability in the internal consistency.
Data analysis
Before performing the data analysis, this study cleaned and preprocessed the data to ensure consistency and handle missing values. This step was crucial for ensuring the accuracy of subsequent analyses. This study employed quantitative data analysis techniques tailored to the research questions. Data analyses were conducted using reputable statistical software such as IBM SPSS version 28. Statistical tests such as the one-way analysis of covariance (ANCOVA) and chi-square tests were used to examine the differences among variables.
ANCOVA was performed to investigate differences in digital citizenship knowledge between two groups concerning research question 1. The independent variable was the gaming approach, and the dependent variable was the post-test scores. The analysis included the pre-test scores as a covariate to control for baseline knowledge. A power analysis revealed that the study achieved an actual power of 0.835 for the ANCOVA, exceeding the conventional threshold of 0.8. It suggests that the study was sufficiently powered to detect the effects being tested. Additionally, a large effect size (Cohen’s f-squared) of 0.8 was determined, which indicates a strong capacity to identify meaningful differences between groups, provided that the estimated effect size was precise.
A Chi-square test was conducted to assess the differences in responses to the affection questionnaire among groups for research question 2. The association between two categorical variables was measured, revealing Phi coefficients varying from 0.314 to 0.487. This variation indicates a moderate to strong relationship between the variables across the different groups. The analysis incorporated 110 valid responses, and in all instances, the tests generated p-values below the conventional alpha level of .05. The statistical significance suggests a consistent and reliable association between the variables within the dataset.
Moreover, the temporal eye movement measures were followed by Yang and Wang (Citation2023) study to analyze students’ gaze positions and eye movements during game playing regarding research question 3. Additionally, reliability was assessed using Cronbach’s alpha for internal consistency of the measuring tools. Values above 0.7 were considered acceptable, indicating that the tools reliably measured the intended constructs. It could be ensured that the data analysis was methodologically sound and the study’s findings were reliable and valid, providing meaningful contributions to digital citizenship education.
Results
Impact of gaming approach on students’ knowledge of digital citizenship
To validate the conditions for conducting an ANCOVA test, Levene’s test for equality of variances was performed and found to be not significant (F(1, 108) = 0.646, p > .05), indicating that variances between groups were equal. Consequently, it was appropriate to proceed with the ANCOVA to compare the knowledge levels between the two groups. As shown in , the adjusted means for the experiment and control groups were 46.74 and 41.47. The different games significantly affected the students’ knowledge (F(1, 109) = 4.34, p < .05). The results indicated that the students who received the fuzzy logic and decision tree-based personalized game had significantly higher knowledge than those who received the decision tree-based gaming approach without the fuzzy logic and personalized learning manners. In response to research question 1, the fuzzy logic and decision tree-based personalized game enhanced students’ knowledge of digital citizenship.
Table 5. The one-way ANCOVA results of students’ knowledge.
Impact of gaming approach on students’ affections
As shown in to response research question 2, the Chi-square test results offer insights into the interventions’ impact on students’ affections. This test was chosen for its suitability with categorical data, and its ability to highlight significant differences between group responses revealed a statistically significant association between the different groups and their ratings of affection regarding various constructs: Perceived Usefulness (PU), Perceived Ease of Use (PEU), Attitude (AT), Intention to Use (IU), Intrinsic Motivation (IM), and Self-Efficacy (SEF). Out of the 22 items compared across all these constructs, the experimental group was more likely to rate their affections as 4 (‘agree’) to 5 (‘strongly agree’) in 18 items compared to those in the control group. However, in 4 items within the PEU, IM, and SEF constructs, the ratings from the experimental group were similar to those from the control group.
Table 6. Rating distribution comparisons between the experimental and control groups (In parenthesis: control group).
Visual attention with eye-tracking analysis
The eye movement records from the four volunteer students who received the fuzzy logic and decision tree-based personalized game were further analyzed. In this study, the Tobii eye trackers were used to monitor students’ gaze positions and eye movements during the game playing. Tobii Pro Lab software was also used to analyze eye movement matrices. This analysis focused on temporal eye movement measures (Yang & Wang, Citation2023): Time to First Fixation (TFF), Total Fixation Duration (TFD), Percentage of Fixation Duration (PFD), Average Fixation Duration (AFD), and Saccade Duration (SD). To analyze the eye movement data, areas of interest (AOIs) were created concerning each type of information involving the core elements of the games: textual representation (ie story-telling and decision-making) and graphical representation (ie facial emotion and personalized feedback). Therefore, TFF is when a viewer’s gaze fixates on a particular AOI after presenting an image or scene. Shorter times can indicate that an AOI is immediately noticeable or of high interest. TFD is the cumulative time that viewers have spent fixating on an AOI. Longer durations typically suggest greater interest or engagement with the AOI. PFD metric reflects the proportion of the total viewing time spent fixating on an AOI. A higher percentage can imply that the AOI is more relevant or appealing to the viewers. AFD is the average length of a single fixation within an AOI. Longer fixations can indicate deeper processing or difficulty in extracting information. Saccade Duration is rapid eye movements as it jumps from one fixation point to another. The duration here may refer to the average time it takes to make these jumps. Saccade characteristics can indicate how viewers take in the information and can vary with the difficulty or distance between AOIs.
Referring to research question 3, the analysis of students’ visual attention characteristics regarding the eye-tracking measures were shown in . It was found that the AI-driven gaming environment, namely the fuzzy logic and decision tree-based personalized game, effectively engages students at various cognitive and emotional levels, with storytelling drawing sustained attention, decision-making prompting immediate but concise engagement, facial emotions being processed rapidly to augment the experience, and personalized feedback capturing a balanced share of attention conducive to learning. The eye-tracking metrics for the AOIs can be comparatively indicated from various perspectives.
Table 7. Means of eye movement measures in different AOIs regarding the fuzzy logic and decision tree-based personalized game group.
The time to first fixation (TFF) data revealed that decision-making elements captured student attention quickly, showcasing the system’s usefulness in emphasizing key learning stages. Storytelling also gathered prompt attention, which was vital for engagement. Facial emotions and personalized feedback, with their higher TFFs, served as supplementary focal points rather than dominating the learning experience of the proposed game. The total fixation duration (TFD) data from the AI-driven gaming study indicated that story-telling commanded the most attention, suggesting high engagement and importance for learning. Decision-making had the shortest TFD, implying efficient processing, possibly due to straightforward or less complex choices. Facial emotion drew more focus than decision-making but less than story-telling, reflecting quicker recognition of emotional cues. Personalized feedback received moderate attention, likely due to its tailored nature necessitating deeper cognitive engagement from students.
When comparing the percentage of fixation time (PFD) across the four AOIs, they suggested a hierarchy of visual engagement. Story-telling elements are the most engaging. The personalized feedback element was four times higher than that for decision-making and facial emotion but lower than storytelling. This suggests personalized feedback captures more attention than the quick-processing elements of decision-making and facial emotion recognition. Both decision-making and facial emotion had the same low PFD, significantly lower than story-telling. This result could suggest that these elements, while important, were quickly processed or not the primary focus of the students during the gameplay. Decision-making might require less time to consider choices, and facial emotions might be easily recognized and thus require less time to process.
The average fixation duration (AFD) metrics suggest that the story-telling element was extremely short. That is, the students were either quickly scanning the storytelling text, or the content was highly efficient at conveying information. The decision-making element was longer AFD, implying that the students spent more time considering the decision-making content. The facial emotion element was moderate AFD, indicating that the students spent a reasonable amount of time processing facial emotions. While facial cues were recognized and considered, they did not require as prolonged attention as decision-making, fitting their role in providing emotional context and cues within the game. The personalized feedback element was shorter AFD than decision-making but longer than storytelling, implying that personalized feedback was noted and processed relatively quickly. This indicates effective communication of feedback where the content is significant enough to warrant attention but concise enough not to disrupt the game’s flow. Additionally, the saccade duration metrics indicate that students transitioned between storytelling elements most quickly, suggesting smoother cognitive flow. Decision-making had the longest saccade times, hinting at more complex thought processes. Facial emotion elicited similar saccade times to decision-making, suggesting substantial cognitive engagement. Personalized feedback had a moderate saccade duration, longer than storytelling but shorter than decision-making and facial emotions, indicating feedback was well-integrated and cognitively balanced within the game.
Discussions
This study significantly advances digital citizenship education by integrating an AI-driven gaming environment. This environment, leveraging a concept-effect propagation-oriented algorithm, fuzzy logic, and decision tree methods, has demonstrated a novel path for personalizing educational experiences in a digital game context. In other words, the game elements include textual representation (ie story-telling and decision-making) and graphical representation (ie facial emotion and personalized feedback). , relevant to research question 1, and , corresponding to research question 2, clearly indicate that fuzzy logic and decision tree-based personalized gaming approach significantly enhanced students’ digital citizenship knowledge and promoted their positive perceptions and altered learning motivations compared to the conventional gaming approach. That is to say, the students engaging with the fuzzy logic and decision-tree-based game had a more favorable response in most items, suggesting that the design elements of this game are more effective in positively influencing students’ affections. It also might be because the fuzzy logic and decision tree-based personalized gaming approach likely provided a more tailored gaming experience, which can enhance Perceived Usefulness (PU), Perceived Ease of Use (PEU), Attitude (AT), Intention to Use (IU), Intrinsic Motivation (IM), and Self-Efficacy (SEF). Specifically, personalization could make the game more relevant and engaging for the students, thereby positively impacting these constructs. In comparison, the conventional gaming approach, which consisted of the decision tree-based gaming approach without fuzzy logic and personalized learning manners, had less personalized feedback for engaging the students in learning digital citizenship. Concerning the psychological constructs, PU and PEU related to how students perceive the game in terms of its benefits and usability. A more personalized game experience can enhance these perceptions. AT and IU were influenced by how much students enjoyed the game and their willingness to use it in the future. Personalization and relevance are key factors here. IM is related to the game’s enjoyment and challenge, which can be enhanced through a well-designed decision tree and fuzzy logic system. Moreover, SEF was influenced by how competent students feel while playing the game; personalized feedback could greatly enhance self-efficacy. Students’ affections aligned with the study of Komalawardhana et al. (Citation2021), which focused on using fuzzy logic in the game. Such a game underscored personalization’s importance in enhancing learning experiences and psychological constructs. Oliveira et al. (Citation2022) also emphasized that personalized gamification positively affected students’ experiences. It aligns with the notion that fuzzy decision trees can create more tailored and responsive gaming environments, improving students’ learning experiences. Using fuzzy logic and decision trees in game-based learning can significantly enhance students’ engagement, learning motivations, and perceptions. Moreover, previous studies underscored the importance of affection elements in the design of intelligent game-based learning environments (Sun et al., Citation2023; Zhong et al., Citation2023). This aligns with the observation that personalization in gaming, which addressed individual learning differences, could significantly enhance student engagement and, consequently, learning performance.
Furthermore, eye-tracking technology provided a more objective and comprehensive visual attention analysis, overcoming the limitations of self-report questionnaires. This suggests that eye-tracking can offer valuable insights into students’ engagement with educational content. In the context of the study’s research question 3, it was found that storytelling elements in the personalized game were most effective in engaging students (). This indicates that storytelling can be a powerful tool in educational games, particularly when combined with sophisticated decision-making algorithms. Decision-making and facial emotion components required less visual attention from the students. It implies that while these elements are important, they do not capture student attention as much as storytelling does. The balance between attention and cognitive engagement was achieved through personalized feedback elements. This suggests that when done right, personalized feedback can enhance the learning experience without overwhelming the students. Additionally, the findings about cognitive engagement and learning outcomes highlight that storytelling and decision-making components, especially when presented textually, effectively capture and hold students’ attention, leading to cognitive engagement and reflection. This is considered crucial for achieving learning outcomes related to digital citizenship. For graphical representations and emotional engagement, the efficient processing of graphical representations, like facial emotions, indicates their potential to enhance emotional engagement. Moreover, they provide meaningful feedback without detracting from the core learning objectives of the game. That is to say, the fuzzy logic and decision tree-based personalized game, including storytelling, decision-making, facial emotion graphics, and personalized feedback, can support visual attention, particularly in learning digital citizenship. These findings align with the previous studies concerning eye-tracking technology, storytelling in educational games, decision-making algorithms, and personalized feedback in educational settings. That is to say, it is the growing interest and effectiveness of eye-tracking technology in educational settings. Scholars revealed that eye-tracking could capture learning behaviors and enhance the understanding of student attention and engagement in educational games (Gu et al., Citation2022; Olsen et al., Citation2022; Pattemore & Gilabert, Citation2023). On the other hand, the study of Campbell (Citation2012) reported that engagement in digital storytelling significantly increased task completion and the ability of students to stay on task. Although direct studies on personalized feedback in educational games using eye-tracking technology were not specifically found, the general trend of research in this field suggested using eye-tracking for games, a positive correlation between innovative teaching methods and enhanced student learning and engagement (Gu et al., Citation2022; Koenka & Anderman, Citation2019; Wang et al., Citation2020). Using eye-tracking data to explore the impact of game elements on visual patterns and attention levels further supported the idea that different components in educational games, such as storytelling and decision-making, have varying effects on student attention and engagement (Chen & Tsai, Citation2015; Chen & Tu, Citation2021). These studies emphasized personalized game elements in supporting visual attention and learning.
Contributions
The study contributes significantly to the field by demonstrating the effectiveness of a personalized, AI-driven gaming approach in digital citizenship education, underlining the importance of tailored learning experiences, and providing novel insights into student engagement and learning through advanced technological methods. That is to say, this study proposed a novel gaming environment, leveraging fuzzy logic and decision tree methodologies. This approach marked a significant advancement in personalizing educational experiences within a digital game context, catering to individual student needs and preferences. The personalized gaming experience positively impacted crucial psychological constructs such as perceived usefulness, perceived ease of use, attitude, intention to use, intrinsic motivation, and self-efficacy, making the game more relevant and engaging for students. Using eye-tracking technology, it was found that the balance between cognitive engagement and visual attention in students was established through personalized feedback elements within the game. Therefore, the study provides important insights into the design of intelligent game-based learning environments. It underscores the importance of including affection elements and personalized feedback in such environments. Moreover, this study contributes to the existing literature by aligning with and expanding upon previous research that emphasizes the importance of personalization in enhancing learning experiences and psychological constructs. It also adds to the body of knowledge on the use of eye-tracking technology in educational settings.
Practical implications
The findings of this study led to the practical implications of affecting the design and implementation of educational games, instructional strategies, and the overall approach to digital citizenship education in the modern and technology-driven educational landscape. Educators and game developers can use the insights from this study to design more effective educational games. Incorporating fuzzy logic and decision tree methodologies can lead to more personalized and engaging learning experiences (Papadimitriou et al., Citation2019). The personalized approach in game design can significantly enhance student engagement and motivation, leading to better learning outcomes, especially in areas like digital citizenship, where attention is crucial. The positive impact on psychological constructs suggests that educational games should be designed to enhance these aspects. This can lead to a more holistic educational experience beyond mere knowledge acquisition. Applying eye-tracking technology in educational settings can provide educators with objective and comprehensive insights into student engagement and learning behaviors, leading to better-informed instructional strategies and game design choices (Alemdag & Cagiltay, Citation2018; Lai et al., Citation2013). Companies and institutions involved in educational technology can leverage these insights for further research and development. This can lead to the creation of more advanced, effective, and user-friendly educational tools and resources.
Theoretical implications
The study’s findings on the AI-driven gaming environments in digital citizenship education also have theoretical implications. The effective integration of AI-driven gaming with fuzzy logic and decision tree methodologies supports and extends existing learning theories. It suggests that personalized, interactive experiences can enhance learning outcomes, aligning with constructivist theories emphasizing the importance of active engagement and personalization in learning (Zhong, Citation2022). The research extends the principles of multimedia learning theory by demonstrating the effectiveness of combining textual and graphical elements, such as storytelling and facial emotion graphics, in educational games. This highlights the importance of using multiple modalities to enhance learning (Brunken et al., Citation2003; Çeken & Taşkın, Citation2022; Zarifsanaiey et al., Citation2022). By employing eye-tracking technology, the study offers new insights into theories of attention and engagement in educational settings. It reveals how different game elements capture and maintain student attention, contributing to theories about visual attention and cognitive engagement. Moreover, the study’s focus on digital citizenship education added to the theoretical understanding of how digital literacy and responsible online behavior can be effectively taught through innovative technology. Therefore, the theoretical implications of this study are extending existing theories in educational psychology, learning, and technology and providing new perspectives on how AI-driven, personalized gaming environments can be utilized to enhance learning experiences and outcomes.
Limitations and future work
The fuzzy logic and decision tree-based personalized gaming approach impacted psychological constructs, such as perceived usefulness, perceived ease of use, attitude, intention to use, intrinsic motivation, self-efficacy, visual attention, and cognitive engagement. There are some limitations of the study. The study’s findings may be specific to the particular AI-driven gaming environment used, limiting the generalizability of the results to other educational contexts or different types of educational games. The study was conducted with a sample from a school; the results might not represent broader student populations, limiting the findings’ applicability to diverse educational settings. The short-term study leaves questions about the sustainability of the effects of using AI-driven gaming environments on students’ learning and motivation, leaving questions about the sustainability of these effects. By addressing these limitations and exploring these suggestions, future research could involve a more diverse and larger sample size to enhance the generalizability of the findings by conducting longitudinal studies to assess the long-term impacts of AI-driven gaming environments on learning and motivation would provide deeper insights. Researching how AI-driven gaming environments can be effectively integrated with traditional learning methods could provide a more holistic approach to education. Additionally, future studies could explore additional psychological constructs, such as social interaction, collaboration, and long-term knowledge retention.
Conclusions
This research proposed a gaming approach in digital citizenship education by integrating AI-powered gaming with algorithms like fuzzy logic and decision trees. The game provided personalized learning experiences through story-telling, decision-making, and graphical elements like facial emotions. The study revealed that this method significantly promoted students’ perceptions and motivations compared to the conventional gaming approach. Eye-tracking technology was employed for in-depth analysis, showing that storytelling captivates students more, while other game aspects demand less visual focus. This method achieved a balance between cognitive engagement and attention through personalized feedback. The findings underscored the effectiveness of incorporating affection and personalization in game-based learning, highlighting the positive impact of fuzzy logic and decision trees on student engagement and perceptions in digital citizenship education.
Authors contributions
Patcharin Panjaburee performed conceptualization, funding acquisition, project administration, formal analysis and writing- original draft preparation. Gwo-Jen Hwang, Ungsinun Intarakamhang, Niwat Srisawasdi performed conceptualization and writing- review and editing. Pawat Chaipidech conducted data curation. All authors read and approved the final manuscript.
Ethics statement
The research involving human participants followed the ethical standards of the committee for research ethics and with comparable ethical standards.
Data availability statement
The datasets generated during and analyzed during the current study are available from the corresponding author on request.
Disclosure statement
No potential conflict of interest was reported by the author(s).
Correction Statement
This article has been corrected with minor changes. These changes do not impact the academic content of the article.
Additional information
Funding
Notes on contributors
Patcharin Panjaburee
Patcharin Panjaburee is an Associate Professor at the Faculty of Education, Khon Kaen University, Thailand. She is interested in computer-assisted testing, adaptive learning, expert systems, digital material-supported learning, inquiry-based mobile learning, and web-based inquiry learning environment. She is the corresponding author of this paper.
Gwo-Jen Hwang
Gwo-Jen Hwang is a Chair professor at the Graduate Institute of Digital Learning and Education, National Taiwan University of Science and Technology, and Graduate Institute of Educational Information and Measurement, National Taichung University of Education, Taiwan. His research interests include mobile learning, digital game-based learning, flipped classrooms, and AI in education.
Ungsinun Intarakamhang
Ungsinun Intarakamhang is an Associate Professor at Behavioral Science Research Institute, Srinakharinwirot University, Thailand. Her research interests include health literacy, psychological social, and performance management.
Niwat Srisawasdi
Niwat Srisawasdi is an Assistant Professor of Science Education at the Division of Science, Mathematics, and Technology Education, Faculty of Education, Khon Kaen University, Thailand. He is interested in technology-enhanced science education and technological pedagogical and content knowledge for the science teacher.
Pawat Chaipidech
Pawat Chaipidech is a faculty member of Science Education at the Division of Science, Mathematics, and Technology Education, Faculty of Education, Khon Kaen University, Thailand. He is interested in mobile learning, technological pedagogical and content knowledge, STEM education, and technology-enhanced learning.
References
- Alemdag, E., & Cagiltay, K. (2018). A systematic review of eye tracking research on multimedia learning. Computers & Education, 125, 413–428. https://doi.org/10.1016/j.compedu.2018.06.023
- Ali, I., Butt, K., & Warraich, N. F. (2023). Factors affecting digital citizenship in the education sector: A systematic review and future direction. Education and Information Technologies. Education and Information Technologies, 28(12), 15789–15821. https://doi.org/10.1007/s10639-023-11811-8
- Blevins, B., LeCompte, K., & Wells, S. (2014). Citizenship education goes digital. The Journal of Social Studies Research, 38(1), 33–44. https://doi.org/10.1016/j.jssr.2013.12.003
- Bolkan, J. (2014). Resources to help you teach digital citizenship. The Journal, 41(12), 21–23.
- Brunken, R., Plass, J. L., & Leutner, D. (2003). Direct measurement of cognitive load in multimedia learning. Educational Psychologist, 38(1), 53–61. https://doi.org/10.1207/S15326985EP38017
- Campbell, T. A. (2012). Digital storytelling in an elementary classroom: Going beyond entertainment. Procedia - Social and Behavioral Sciences, 69, 385–393. https://doi.org/10.1016/j.sbspro.2012.11.424
- Çeken, B., & Taşkın, N. (2022). Multimedia learning principles in different learning environments: A systematic review. Smart Learning Environments, 9(1), 1–22. https://doi.org/10.1186/s40561-022-00200-2
- Chang, C. Y., & Hwang, G. J. (2019). Trends in digital game-based learning in the mobile era: a systematic review of journal publications from 2007 to 2016. International Journal of Mobile Learning and Organisation, 13(1), 68–90. https://doi.org/10.1504/IJMLO.2019.096468
- Chen, C.-C., & Tu, H.-Y. (2021). The effect of digital game-based learning on learning motivation and performance under social cognitive theory and entrepreneurial thinking. Frontiers in Psychology, 12, 750711. https://doi.org/10.3389/fpsyg.2021.750711
- Chen, L. L., Mirpuri, S., Rao, N., & Law, N. (2021). Conceptualization and measurement of digital citizenship across disciplines. Educational Research Review, 33, 100379. https://doi.org/10.1016/j.edurev.2021.100379
- Chen, Y. J., & Hung, Y. C. (2010). Using real-time acceleration data for exercise movement training with a decision tree approach. Expert Systems with Applications, 37(12), 7552–7556. https://doi.org/10.1016/j.eswa.2010.04.089
- Chen, Y., & Tsai, M.-J. (2015). Eye-hand coordination strategies during active video game playing: An eye-tracking study. Computers in Human Behavior, 51(A), 8–14. https://doi.org/10.1016/j.chb.2015.04.045
- Cheng, G., Zou, D., Xie, H., & Wang, F. L. (2024). Exploring differences in self-regulated learning strategy use between high- and low-performing students in introductory programming: An analysis of eye-tracking and retrospective think-aloud data from program comprehension. Computers & Education, 208, 104948. https://doi.org/10.1016/j.compedu.2023.104948
- Christensen, I. R., Biseth, H., & Huang, L. (2021). Developing digital citizenship and civic engagement through social media use in Nordic schools. In H. Biseth, B. Hoskins, & L. Huang (Eds.), Northern lights on civic and citizenship education IEA research for education (vol. 11). Springer. https://doi.org/10.1007/978-3-030-66788-7_4
- Clark, D. B., Tanner-Smith, E. E., & Killingsworth, S. S. (2016). Digital games, design, and learning: A systematic review and meta-analysis. Review of Educational Research, 86(1), 79–122. https://doi.org/10.3102/0034654315582065
- Cohen, E. L. (2014). What makes good games go viral? The role of technology use, efficacy, emotion and enjoyment in players’ decision to share a prosocial digital game. Computers in Human Behavior, 33, 321–329. https://doi.org/10.1016/j.chb.2013.07.013
- Common Sense Media. (2016). K-12 digital citizenship curriculum. https://www.commonsense.org/education/scope-and-sequence.
- d‘Haenens, L., Koeman, J., & Saeys, F. (2007). Digital citizenship among ethnic minority youths in the Netherlands and Flanders. New Media & Society, 9(2), 278–299. https://doi.org/10.1177/1461444807075013
- Duran, M. (2022). Digital citizenship. In M. Duran (Ed.), Learning Technologies. Springer. https://doi.org/10.1007/978-3-031-18111-5_8
- Fang, M., Tapalova, O., Zhiyenbayeva, N., & Kozlovskaya, S. (2022). Impact of digital game-based learning on the social competence and behavior of preschoolers. Education and Information Technologies, 27(3), 3065–3078. https://doi.org/10.21203/rs.3.rs-707659/v1
- Fredrick, K. (2013). Fostering digital citizenship. School Library Monthly, 29(4), 20–21.
- Glynn, S. M., & Koballa, T. R. (2006). Motivation to learn college science. In J. J. Mintzes & W. H. Leonard (Eds), Handbook of college science teaching (pp. 25–32). National Sciences Teachers Association Press.
- Gomes, C., & Almeida, L. S. (2017). Advocating the broad use of the decision tree method in education. Practical Assessment, Research, and Evaluation, 22(1), 10. https://doi.org/10.7275/y36w-hg55.
- Gu, C., Chen, J., Lin, J., Lin, S., Wu, W., Jiang, Q., Yang, C., & Wei, W. (2022). The impact of eye-tracking games as a training case on students’ learning interest and continuous learning intention in game design courses: Taking Flappy Bird as an example. Learning and Motivation, 78, 101808. https://doi.org/10.1016/j.lmot.2022.101808
- Hamari, J., Shernoff, D. J., Rowe, E., Coller, B., Asbell-Clarke, J., & Edwards, T. (2016). Challenging games help students learn: An empirical study on engagement, flow and immersion in game-based learning. Computers in Human Behavior, 54, 170–179. https://doi.org/10.1016/j.chb.2015.07.045
- Hill, V. (2015). Digital citizenship through game design in Minecraft. New Library World, 116(7/8), 369–382. https://doi.org/10.1108/NLW-09-2014-0112
- Hollandsworth, R., Donovan, J., & Welch, M. (2017). Digital citizenship: You can’t go home again. TechTrends, 61(6), 524–530. https://doi.org/10.1007/s11528-017-0190-4
- Hsiao, H. S., Chang, C. S., Lin, C. Y., & Hu, P. M. (2014). Development of children’s creativity and manual skills within digital game‐based learning environment. Journal of Computer Assisted Learning, 30(4), 377–395. https://doi.org/10.1111/jcal.12057
- Hwang, G.-J., Sung, H.-Y., Chang, S.-C., & Huang, X.-C. (2020). A fuzzy expert system-based adaptive learning approach to improving students’ learning performances by considering affective and cognitive factors. Computers and Education, 1, 100003. https://doi.org/10.1016/j.caeai.2020.100003
- Ingkavara, T., Panjaburee, P., Srisawasdi, N., & Sajjapanroj, S. (2022). The use of a personalized learning approach to implementing self-regulated online learning. Computers and Education, 3, 100086. https://doi.org/10.1016/j.caeai.2022.100086
- James, C., Davis, K., Flores, A., Francis, J. M., Pettingill, L., & Rundle, M. (2009). Young people, ethics, and the new digital media. Digital Media, 2(54), 127. https://doi.org/10.7551/mitpress/8520.001.0001
- Jones, L. M., & Mitchell, K. J. (2016). Defining and measuring youth digital citizenship. New Media & Society, 18(9), 2063–2079. https://doi.org/10.1177/1461444815577797
- Karnon, J. (2020). A simple decision analysis of a mandatory lockdown response to the COVID-19 pandemic. Applied Health Economics and Health Policy, 18(3), 329–331. https://doi.org/10.1007/s40258-020-00581-w
- Khan, A., Ahmad, F. H., & Malik, M. M. (2017). Use of digital game based learning and gamification in secondary school science: The effect on student engagement, learning and gender difference. Education and Information Technologies, 22(6), 2767–2804. https://doi.org/10.1007/s10639-017-9622-1
- Kiili, K., Moeller, K., & Ninaus, M. (2018). Evaluating the effectiveness of a game-based rational number training - In-game metrics as learning indicators. Computers & Education, 120, 13–28. https://doi.org/10.1016/j.compedu.2018.01.012
- Kim, Y. J., & Shute, V. J. (2015). The interplay of game elements with psychometric qualities, learning, and enjoyment in game-based assessment. Computers & Education, 87, 340–356. https://doi.org/10.1016/j.compedu.2015.07.009
- Koenka, A. C., & Anderman, E. M. (2019). Personalized feedback as a strategy for improving motivation and performance among middle school students. Middle School Journal, 50(5), 15–22. https://doi.org/10.1080/00940771.2019.1674768
- Komalawardhana, N., Panjaburee, P., & Srisawasdi, N. (2021). A mobile game-based learning system with personalised conceptual level and mastery learning approach to promoting students’ learning perceptions and achievements. International Journal of Mobile Learning and Organisation, 15(1), 29–49. https://doi.org/10.1504/IJMLO.2021.10032848
- Kurilovas, E. (2019). Advanced machine learning approaches to personalise learning: learning analytics and decision making. Behaviour & Information Technology, 38(4), 410–421. https://doi.org/10.1080/0144929X.2018.1539517
- Lai, M.-L., Tsai, M.-J., Yang, F.-Y., Hsu, C.-Y., Liu, T.-C., Lee, S. W.-Y., Lee, M.-H., Chiou, G.-L., Liang, J.-C., & Tsai, C.-C. (2013). A review of using eye-tracking technology in exploring learning from 2000 to 2012. Educational Research Review, 10, 90–115. https://doi.org/10.1016/j.edurev.2013.10.001
- Lauricella, A. R., Herdzina, J., & Robb, M. (2020). Early childhood educators’ teaching of digital citizenship competencies. Computers & Education, 158, 103989. https://doi.org/10.1016/j.compedu.2020.103989
- Law, N., Chow, S. L., & Fu, K. W. (2018). Digital citizenship and social media: A curriculum perspective. In J. Voogt, G. Knezek, R. Christensen, & K. W. Lai (Eds.), Second handbook of information technology in primary and secondary education. Springer. https://doi.org/10.1007/978-3-319-53803-7_3-2
- Li, Y., Cheung, S. K. S., Wang, F. L., Lu, A., & Kwok, L. F. (2023). Integrating digital citizenship into a primary school course “Ethics and the Rule of Law”: Necessity, strategies and a pilot study: Lessons learned and ways forward. Springer. https://doi.org/10.1007/978-3-031-35731-2_7
- Maina, M. F., Santos-Hermosa, G., Mancini, F., & Guàrdia Ortiz, L. (2020). Open educational practices (OEP) in the design of digital competence assessment. Distance Education, 41(2), 261–278. https://doi.org/10.1080/01587919.2020.1757407
- Mattson, K., & Curran, M. B. F. X. (2017). Digital citizenship education: Moving beyond personal responsibility. In B. S. De Abreu, P. Mihailidis, A. Y. Lee, J. Melki, & J. McDougall (Eds.), International handbook of media literacy education (1st ed., pp. 12). Routledge. https://doi.org/10.4324/9781315628110
- Ng, W. (2012). Can we teach digital natives digital literacy? Computers & Education, 59(3), 1065–1078. https://doi.org/10.1016/j.compedu.2012.04.016
- Oliveira, W., Hamari, J., Joaquim, S., Toda, A. M., Palomino, P. T., Vassileva, J., & Isotani, S. (2022). The effects of personalized gamification on students’ flow experience, motivation, and enjoyment. Smart Learning Environments, 9(1), 1–16. https://doi.org/10.1186/s40561-022-00194-x
- Olsen, J. K., Ozgur, A. G., Sharma, K., & Johal, W. (2022). Leveraging eye tracking to understand children’s attention during game-based, tangible robotics activities. International Journal of Child-Computer Interaction, 31, 100447. https://doi.org/10.1016/j.ijcci.2021.100447
- Paideya, V., & Bengesai, A. V. (2021). Predicting patterns of persistence at a South African university: A decision tree approach. International Journal of Educational Management, 35(6), 1245–1262. https://doi.org/10.1108/IJEM-04-2020-0184
- Panjaburee, P., Komalawardhana, N., & Ingkavara, T. (2022). Acceptance of personalized e-learning systems: A case study of concept-effect relationship approach on science, technology, and mathematics courses. Journal of Computers in Education, 9(4), 681–705. https://doi.org/10.1007/s40692-021-00216-6
- Papadakis, S., & Kalogiannakis, M. (2019). Evaluating the effectiveness of a game-based learning approach in modifying students’ behavioural outcomes and competence, in an introductory programming course. A case study in Greece. International Journal of Teaching and Case Studies, 10(3), 235–250. https://doi.org/10.1504/IJTCS.2019.102760
- Papadimitriou, S., Chrysafiadi, K., & Virvou, M. (2019). Evaluating the use of fuzzy logic in an educational game for offering adaptation. 2019 International Conference on Computer, Information and Telecommunication Systems (CITS) (pp. 1–5). IEEE. https://doi.org/10.1109/CITS.2019.8862064
- Patel, H. H., & Prajapati, P. (2018). Study and analysis of decision tree based classification algorithms. International Journal of Computer Sciences and Engineering, 6(10), 74–78. https://doi.org/10.26438/ijcse/v6i10.7478
- Pattemore, M., & Gilabert, R. (2023). Using eye-tracking to measure cognitive engagement with feedback in a digital literacy game. The Language Learning Journal, 51(4), 472–490. https://doi.org/10.1080/09571736.2023.2207582
- Plass, J. L., Homer, B. D., & Kinzer, C. K. (2015). Foundations of game-based learning. Educational Psychologist, 50(4), 258–283. https://doi.org/10.1080/00461520.2015.1122533
- Podgorelec, V., Kokol, P., Stiglic, B., & Rozman, I. (2002). Decision trees: an overview and their use in medicine. Journal of Medical Systems, 26(5), 445–463. https://doi.org/10.1023/a:1016409317640
- Prensky, M. (2004). The emerging online life of the digital native. https://www.bu.edu/ssw/files/pdf/Prensky-The_Emerging_Online_Life_of_the_Digital_Native-033.pdf.
- Priyam, A., Abhijeeta, G. R., Rathee, A., & Srivastava, S. (2013). Comparative analysis of decision tree classification algorithms. International Journal of Current Engineering and Technology, 3(2), 334–337.
- Ribble, M. (2011). The nine elements of digital citizenship. Digital Citizenship in Schools, 3777, 15–44. https://doi.org/10.1111/j.1467-8535.2012.01378_9.x
- Ribble, M. (2015). Digital citizenship in schools: Nine elements all students should know. International Society for Technology in Education.
- Ribble, M. S., Bailey, G. D., & Ross, T. W. (2004). Digital citizenship: Addressing appropriate technology behaviour. Learning and Leading with Technology, 32(1), 6. https://doi.org/10.1002/asi.20906
- Rizvi, S., Rienties, B., & Khoja, S. A. (2019). The role of demographics in online learning; A decision tree based approach. Computers & Education, 137, 32–47. https://doi.org/10.1016/j.compedu.2019.04.001
- Ronimus, M., Kujala, J., Tolvanen, A., & Lyytinen, H. (2014). Children’s engagement during digital game-based learning of reading: The effects of time, rewards, and challenge. Computers & Education, 71, 237–246. https://doi.org/10.1016/j.compedu.2013.10.008
- Saleme, P., Dietrich, T., Pang, B., & Parkinson, J. (2021). Design of a digital game intervention to promote socio-emotional skills and prosocial behavior in children. Multimodal Technologies and Interaction, 5(10), 58. https://doi.org/10.3390/mti5100058
- Sanders, M. J., Van Oss, T., & McGeary, S. (2016). ISTE standards. Journal of Experiential Education, 39(1), 73–88. https://doi.org/10.1177/1053825915608872
- Schaaf, R. (2012). Does digital game-based learning improve student time-on-task behavior and engagement in comparison to alternative instructional strategies? The Canadian Journal of Action Research, 13(1), 50–64. https://doi.org/10.33524/cjar.v13i1.30
- Searson, M., Hancock, M., Soheil, N., & Shepherd, G. (2015). Digital citizenship within global contexts. Education and Information Technologies, 20(4), 729–741. https://doi.org/10.1007/s10639-015-9426-0
- Sittichai, R. (2013). Bullying and cyberbullying in Thailand: A review. International Journal of Cyber Society and Education, 6(1), 31–44. https://doi.org/10.7903/ijcse.1032
- Srisuwan, C., & Panjaburee, P. (2020). Implementation of flipped classroom with personalised ubiquitous learning support system to promote the university student performance of information literacy. International Journal of Mobile Learning and Organisation, 14(3), 398. https://doi.org/10.1504/IJMLO.2020.108200
- Sun, L., Kangas, M., & Ruokamo, H. (2023). Game-based features in intelligent game-based learning environments: A systematic literature review. Interactive Learning Environments, 1–17. https://doi.org/10.1080/10494820.2023.2179638
- Tadlaoui-Brahmi, A., Çuko, K., & Alvarez, L. (2022). Digital citizenship in primary education: A systematic literature review describing how it is implemented. Social Sciences & Humanities Open, 6(1), 100348. https://doi.org/10.1016/j.ssaho.2022.100348
- Tapingkae, P., Panjaburee, P., Hwang, G. J., & Srisawasdi, N. (2020). Effects of a formative assessment-based contextual gaming approach on students’ digital citizenship behaviours, learning motivations, and perceptions. Computers & Education, 159, 103998. https://doi.org/10.1016/j.compedu.2020.103998
- Teo, T., Lee, C. B., Chai, C. S., & Wong, S. L. (2009). Assessing the intention to use technology among pre-service teachers in Singapore and Malaysia: A multigroup invariance analysis of the technology acceptance model (TAM). Computers & Education, 53(3), 1000–1009. https://doi.org/10.1016/j.compedu.2009.05.017
- Thompson, P. (2013). The digital natives as learners: Technology use patterns and approaches to learning. Computers & Education, 65, 12–33. https://doi.org/10.1016/j.compedu.2012.12.022
- Tokunaga, R. S. (2010). Following you home from school: A critical review and synthesis of research on cyberbullying victimization. Computers in Human Behavior, 26(3), 277–287. https://doi.org/10.1016/j.chb.2009.11.014
- Tsai, F. H. (2017). An investigation of gender differences in a game-based learning environment with different game modes. EURASIA Journal of Mathematics, Science and Technology Education, 13(7), 3209–3226. https://doi.org/10.12973/eurasia.2017.00713a
- Tsai, F. H., Yu, K. C., & Hsiao, H. S. (2012). Exploring the factors influencing learning effectiveness in digital game-based learning. Journal of Educational Technology & Society, 15(3), 240–250.
- Vajen, B., Kenner, S., & Reichert, F. (2023). Digital citizenship education – Teachers’ perspectives and practices in Germany and Hong Kong. Teaching and Teacher Education, 122, 103972. https://doi.org/10.1016/j.tate.2022.103972
- Van der Kleij, F. M., Feskens, R. C. W., & Eggen, T. J. H. M. (2015). Effects of feedback in a computer-based learning environment on students’ learning outcomes: A meta-analysis. Review of Educational Research, 85(4), 475–511. https://doi.org/10.3102/0034654314564881
- Verma, A., Jha, M., & Mitra, M. (2016). Digital awareness and internet usage by school students. Journal of Psychosocial Research, 11(2), 259–270. https://www.proquest.com/scholarly-journals/digital-awareness-internet-usage-school-students/docview/1868276902/se-2.
- Wachs, S., Jiskrova, G. K., Vazsonyi, A. T., Wolf, K. D., & Junger, M. (2016). A cross-national study of direct and indirect effects of cyberbullying on cybergrooming victimization via self-esteem. Psicología Educativa, 22(1), 61–70. https://doi.org/10.1016/j.pse.2016.01.002
- Wang, X., Lin, L., Han, M., & Spector, J. M. (2020). Impacts of cues on learning: Using eye-tracking technologies to examine the functions and designs of added cues in short instructional videos. Computers in Human Behavior, 107, 106279. https://doi.org/10.1016/j.chb.2020.106279
- Wanichsan, D., Panjaburee, P., & Chookaew, S. (2021). Enhancing knowledge integration from multiple experts to guiding personalized learning paths for testing and diagnostic systems. Computers and Education: Artificial Intelligence, 2, 100013. https://doi.org/10.1016/j.caeai.2021.100013
- Wongwatkit, C., Srisawasdi, N., Hwang, G. J., & Panjaburee, P. (2017). Influence of an integrated learning diagnosis and formative assessment-based personalized web learning approach on students learning performances and perceptions. Interactive Learning Environments, 25(7), 889–903. https://doi.org/10.1080/10494820.2016.1224255
- Wu, M. C., Lin, S. Y., & Lin, C. H. (2006). An effective application of decision tree to stock trading. Expert Systems with Applications, 31(2), 270–274. https://doi.org/10.1016/j.eswa.2005.09.026
- Yang, F.-Y., & Wang, H.-Y. (2023). Tracking visual attention during learning of complex science concepts with augmented 3D visualizations. Computers & Education, 193, 104659. https://doi.org/10.1016/j.compedu.2022.104659
- Yang, J. C., & Chen, S. Y. (2020). An investigation of game behavior in the context of digital game-based learning: an individual difference perspective. Computers in Human Behavior, 112, 106432. https://doi.org/10.1016/j.chb.2020.106432
- Yang, J. C., Chung, C. J., & Chen, M. S. (2022). Effects of performance goal orientations on learning performance and in‐game performance in digital game‐based learning. Journal of Computer Assisted Learning, 38(2), 422–439. https://doi.org/10.1111/jcal.12622
- Yang, K. H., & Lu, B. C. (2021). Towards the successful game-based learning: Detection and feedback to misconceptions is the key. Computers & Education, 160, 104033. https://doi.org/10.1016/j.compedu.2020.104033
- Yu, Z., Gao, M., & Wang, L. (2021). The effect of educational games on learning outcomes, student motivation, engagement and satisfaction. Journal of Educational Computing Research, 59(3), 522–546. https://doi.org/10.1177/0735633120969214
- Zarifsanaiey, N., Mehrabi, Z., Kashefian-Naeeini, S., & Mustapha, R. (2022). The effects of digital storytelling with group discussion on social and emotional intelligence among female elementary school students. Cogent Psychology, 9(1), 2004872. https://doi.org/10.1080/23311908.2021.2004872
- Zhong, L. (2022). Incorporating personalized learning in a role-playing game environment via SID model: A pilot study of impact on learning performance and cognitive load. Smart Learning Environments, 9(1), 1–18. https://doi.org/10.1186/s40561-022-00219-5
- Zhong, L., Xie, Y., & Xu, L. (2023). The impact of personalization feature on students’ engagement patterns in a role-playing game: A cultural perspective. Education and Information Technologies, 28(7), 8357–8375. https://doi.org/10.1007/s10639-022-11529-z