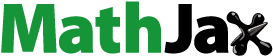
ABSTRACT
Anti-government protests emerged globally in response to COVID-19 countermeasures. What are the key drivers of these pandemic-related protests, and to what extent do they differ from the drivers of non-COVID protests? We examine these questions in the context of Israel, which faced a growing political crisis at the start of the pandemic, effectively blurring the distinction between different causes of protest. Our data features 1,922 protests across 189 Israeli localities for the period between March and July 2022. Using a machine learning approach, we find that all protests, regardless of whether they were directly related to the pandemic or not, were motivated by the same set of key indicators – albeit with the ranking of drivers for COVID-related protests inverted for non-COVID protests. Local infection rates and government responses were more pronounced for the former, whereas differences in residential and commercial property taxes, access to affordable housing, quality of education and demography were among the most important drivers for the latter. Our analysis underscores the role that local governments played in managing the pandemic, and demonstrates that variation in socioeconomic conditions had an important effect on the incidence of protests across Israel.
KEYWORDS:
Introduction
Israel's ‘double crisis’ – the onset of the pandemic coupled with a deepening political crisis – led to the emergence of multiple protest movements with distinct goals (Hitman Citation2022; Maor, Sulitzeanu-Kenan, and Chinitz Citation2020). In Tel Aviv, the anti-Netanyahu Black Flags movement called on the Prime Minister to resign over corruption charges. In Jerusalem, self-employed workers and members of Histadrut, the General Federation of Labour in Israel, lamented the government's failure to protect jobs and businesses, demanding compensation for financial losses. And in localities across Israel, protests against pandemic-related restrictions occurred, albeit with little or no coordination (Hitman Citation2021). To what extent, then is it possible to identify common drivers across the seemingly disparate landscape of political and pandemic-related protests in Israel?
We employ machine learning methods – specifically, a random forest classifier – as a complement to conventional statistical modelling techniques in an effort identify the drivers of different types of protest activity. Quantitative studies typically rely upon regression analysis, OLS (Kriesi and Oana Citation2022) or negative binomial models (Neumayer, Pfaff, and Plümper Citation2023; Plümper, Neumayer, and Pfaff Citation2021; van der Zwet et al. Citation2022) in particular, albeit with more recent work that adopts a systems dynamics approach (van der Zwet et al. Citation2022) to analyze protest activity. Our approach permits a systematic exploration of the relative importance and interdependence of societal, political, economic and health-related conditions, accounting for the onset of political and pandemic-related protests across localities. Our sample consists of 189 localities in which 1,922 protests were recorded between March 2020 and July 2022 (ACLED Citation2020a).
The paper is organized as follows. In Section ‘Literature review’, we provide an overview of protest dynamics in Israel as well as prior work that our analysis builds on. In Section ‘Data sources and processing’, we describe the study period, data sources and processing, and the machine learning approach and analysis in Section ‘Methods’. We present our results in Section ‘Results’ and discuss the robustness and limitations of the analysis and provide concluding remarks in Section ‘Discussion’.
Literature review
Protests are by no means new to Israel, with movements spanning issue areas, social groups and time periods (Fleischmann Citation2021). In the early seventies, the Israeli ‘Black Panthers Movement’ staged demonstrations in Jerusalem to protest grievances felt by Mizrahi Jews – namely immigration policies that favoured the Ashkenazi (Frankel Citation2020). More recently, acute shortages of affordable housing for low- to moderate-income households resulted in ‘Tent Protests’ expressing dissatisfaction with Netanyahu's neo-liberal policies (Hananel and Nachmany Citation2021). The Tent Protests further underscore the salience of structure or the built environment – the encampments on Rothschild Boulevard in central Tel Aviv effectively increasing visibility and enabling the protests to spread across the country (Marom Citation2013). Like structure, opportunity-based factors – utility driven calculations shaped by the political environment, coordination and turnout, framing and alignment – have also played a key role (Alimi Citation2012). Growing international criticism of the ‘separation wall’ in 2002 provided a window of opportunity for the ‘Machsom Watch’ movement in an otherwise closed political environment (Kaufman Citation2008).Footnote1 Unlike opportunity, coercion serves as a constraint on protest, though it may also serve a motivating factor. In 2020, religious Jews protested against the implementation of Daylight Savings Time by the government, viewing the proposed change as an infringement on their rituals, calendars and every-day routines (Barak Citation2020).
It follows that social protest may be driven by four distinct mechanisms: (i) grievances (Berkowitz Citation1989; Cederman, Gleditsch, and Buhaug Citation2013; Collier and Hoeffler Citation2004; Kurer et al. Citation2019); (ii) structure (Al-Sayed and Hanna Citation2013; Castells Citation1983; Goldberg Citation2010; Gould Citation1991; Hillier, Yang, and Turner Citation2012); (iii) coercion (Borland and Sutton Citation2007; Carey Citation2006; Davenport and Inman Citation2012; Gupta, Singh, and Sprague Citation1993; Lichbach Citation1987; Trentmann Citation2009) and (iv) opportunity (Cederman Citation2005; Eisinger Citation1973; Granovetter Citation1978; Kuran Citation1991; Noelle-Neumann Citation1974; Tilly Citation1978; Warhurst, Tilly, and Gatta Citation2017; Wimmer Citation2008). We use a total of 60 indicators, –
, to assess the relative importance of these drivers, which notably share a significant degree of overlap.
Economic and material grievances have typically played a prominent role in generating protests (Hermann Citation1996). Grievance related indicators comprise inequality, relative deprivation or exclusion as sources of protest. Examples include ethnic or religious discrimination (Cederman, Wimmer, and Min Citation2010) (–
) and socio-economic inequalities (
–
) or economic instability (
–
), poverty (
–
) and decreased life expectancy (
), as well as levels of locality development (
), all of which increase existing grievances. Demographic (
–
) and structural factors also serve as key determinants of protest. Examples include municipality characteristics (
–
), infrastructure and access to basic services, including education (
–
) and healthcare (
–
), and the built environment or the spatial layout of a city (
–
), which in turn provides both opportunities and constraints for protest (Parkinson Citation2012; Routledge Citation2003). Examples of opportunity-based factors include population size and density (
–
), high COVID-19 infection rates and related dynamics (
–
), as well as electoral turnout (
–
). Unlike opportunity, coercion serves as a constraint on protest, though it may also serve a motivating factor. Examples include repressive policies and measures taken by the Israeli government to curtail freedom of movement or disrupt everyday routines in response to the pandemic, such as curfews and lockdowns (
–
).
Our application of a machine learning approach makes no a priori assumptions about the relative importance of the four mechanisms for COVID-19 (henceforth COVID) and non-COVID protests – those not directly related to the pandemic.
Data sources and processing
To compare the drivers of COVID and non-COVID protest, we employ a novel dataset on protest dynamics in Israel that spans the period from March 2020 to July 2022 – accounting for the peak of the pandemic in Israel. Our data include granular information on weekly protest events for 189 Israeli localities and 60 potential indicators drawn from a variety of publicly-available sources. We provide the full list of covariates and sources, together with descriptions of data structure, pre-processing and aggregation rules in Supplementary Table S1 and S2 of the Online Appendix.
Locality-level covariates. Israel has one sub-national level of government, composed of directly elected local authorities that have administrative and governmental power in their locality (CBS Citation2023). Annual survey data on various locality characteristics, specifically population, ethnicity, religiosity, demography, education, health, housing prices, taxation and voter turnout are provided by the Israeli Central Bureau for Statistics (CBS) (CBS Citation2020) and combined with daily information on local COVID dynamics. Daily information on the number of COVID infections and tests per locality is available from the Israeli Ministry of Health (Israel Citation2023) and included to track the evolution of the pandemic over time.
State-level covariates. At the state-level, we use daily information on national government responses to the pandemic, relying on the Oxford COVID-19 Government Response Tracker (OxCGRT) (Oxford COVID-19 Government Response Tracker Citation2023). Following Hale et al. (Citation2021) and van der Zwet et al. (Citation2022), we use four indexes calculated daily from a total of 19 indicators. These indices capture the all government responses (GRI), stringency of containment efforts, and measures taken to ameliorate health care (CHI) and enhance economic support (ESI).
Protest events
Daily protest data is sourced from the Armed Conflict Location & Event Data Project (ACLED, Raleigh et al. Citation2010). To effectively disentangle direct and indirect effects of the pandemic on protest patterns, we combine incident information from ACLED's regular Disorder Events in Israel and Palestine (ACLED Citation2023b) with COVID-19 Disorder Events (ACLED Citation2023a). All events featured in the ACLED's curated data file are directly related to the global pandemic. Following ACLED's coding, we identify protests as directly related to COVID, if ‘coronavirus’ or ‘COVID-19’ are mentioned in the explanatory notes column.Footnote2 This tag is used only if an incident report explicitly states that coronavirus motivated the event (ACLED Citation2020a) – for instance, protests as direct responses to government (mis)management of the pandemic or demands for economic support to compensate losses caused by containment policies or lockdowns.
‘On 19 October 2021, tour guides demonstrated in Ben Gurion International Airport (HaMerkaz, Petah Tiqwa) against the continuation of the coronavirus restrictions that forbade tourists from entering Israel, leaving them unemployed. The protesters tried to block the entrance to the airport's departure gate and were removed by a police force’.
Yet, this distinction is not always clear, as protest incidents with mixed motives, as illustrated by the example below, are also considered to be COVID-related.
‘On 1 August 2020, a protest was held in Sderot as part of the nationwide Black Flag Movement, demanding the resignation of Prime Minister Netanyahu over corruption charges and the mishandling of the coronavirus pandemic. Three Netanyahu supporters splashed unidentified fluid on protesters, and were later arrested by the police’.
The example that follows, of a non-COVID protest, was motivated by the ongoing political crisis, albeit with no direct relation to the pandemic.
‘On 24 September 2021, Jewish and Arab protesters, affiliated with “Standing Together” and other Israeli pro-peace organizations held a demonstration rally in Hanton (HaZafon, Akko), demanding the Israeli government to revive the Israeli-Palestinian peace process’.
Our sample includes 1,922 protests, of which 597 are directly related to COVID. illustrates reported protests in the 189 Israeli localities featured in this study (in pink). During the 125-week study period, protest activity was reported in 110 localities with an average of 9.56 protests per locality. Jerusalem, the capital city of Israel, had the highest incidence (308 protests), followed by Tel Aviv (303 protests) and Haifa (123 protests). illustrates that the number of protests was low in the early stages of the pandemic (from March to April 2020) with protest activity peaking between December 2020 and March 2021 (precisely when most people received their first vaccination) with a pronounced dip in non-COVID protest activity.
Data pre-processing and aggregation
Our unit of analysis is the locality-week over a period that corresponds to the information available in the COVID-19 Disorder Tracker (ACLED Citation2023a). The first reported incident of protest against COVID-19 measures took place in March 2020 (calendar week 10) and we cover the following 125 consecutive weeks. To generate a model landscape that closely reflects changes in protest patterns across regions in Israel, all observations are geo-coded at the locality-level, drawing on geographical information systems (GIS) and shape files from the Central Bureau of Statistics (CBS) in Israel (CBS Citation2020). Protests are assigned to localities when the point coordinates of daily protest events overlap with a municipality polygon area. Locality characteristics are slow-moving and hence considered as fixed in the analysis. COVID dynamics and government measures are aggregated to their weekly means. In addition, missing values are imputed using k-nearest neighbour imputation and standardized, i.e. centered around zero and with roughly unit variance. Additional details, including summary statistics of the 60 indicators are provided in Supplementary Section A3 in the Online Appendix.
Methods
We begin with the premise that all four mechanisms, and associated covariates, comprise plausible predictors for protest occurrence. The data data-driven strategy we adopt to identify key predictors effectively allows us to discriminate between more and less plausible explanations for the protest dynamics. This approach also enables us to compare key drivers across protest types – COVID protests and non-COVID protests, the latter not directly related to the pandemic.
The main model specifications predict protest occurrence – the number of protests in a given week – using two modelling techniques: (i) a Poisson regression model which serves as a baseline model; and (ii) a random forest classifier as our main machine learning algorithm. The regression framework serves as the closest approximation to methods used in related studies of COVID protests (Hunger, Hutter, and Kanol Citation2023; Kriesi and Oana Citation2022; Neumayer, Pfaff, and Plümper Citation2023; Sørensen and Christiansen Citation2022) whereas a key feature of random forest classifiers is selection – the ability to identify a minimal set of key predictors, thereby improving predictive accuracy and performance classification (Chen et al. Citation2020).
We report results from the random forest classifier given its overall superior performance relative to the baseline Poisson regression model, although both models consistently identify the same key dimensions. Baseline model results are relegated to Section B1 in the Online Appendix, and referenced, where appropriate, in the main text. We rely on standard best practices for machine learning in this setting, including k-fold cross-validation, regularization and tests of out-of-sample predictive power. Given the panel structure of our data, we sample training and test data to ensure a consistent temporal structure. The next section provides more details of the machine learning approach we employ.
Machine learning approach
As a first step, we prepare the data for the prediction task and train our model with all available covariates. This allows us to identify covariates with the greatest importance and specify a minimal predictive model that use a smaller set of key predictors. We do this both for COVID and non-COVID protests. In a second step, we run additional robustness checks, including cross-predictions (see Supplementary Section B in the Online Appendix), out-of-sample prediction tests on a temporally split-sample (see Supplementary Section C1 in the Online Appendix) and an assessment of classification accuracy, relative to the baseline model performance, for binary protest outcomes (see Supplementary Section C2 in the Online Appendix).
Data preparation. Data preparation entails a number of subtle but important methodological choices which we highlight here. The machine learning approach requires us to split the data in two non-overlapping sets, the train and test set. The train set – as the name suggests – is used to train the model in-sample and identify the most influential predictors. The test set is then used to evaluate model performance out-of-sample on data the model has not been trained on. We use a standard 80% to 20% split for the train vs. test data. We therefore adopt a standard machine learning setup, albeit one that is attentive to the panel structure of our data – weekly data for each of the 189 localities over 125 consecutive weeks.
In a non-panel setting, the standard approach for split samples would randomly allocate observations to each of the two sets. Given that we have time-ordered observations per locality, the model could be trained on observations later in our study period to predict prior observations – events that occurred at an earlier time – thus, violating the causal ordering of events. In addition, the standard split-sample approach could artificially separate observations from the same locality that may be temporally auto-correlated – localities that already experienced protests are more likely to experience protests again. To overcome these limitations, we implement a custom splitting algorithm that randomly samples at the locality – level while ensuring that the (aggregate) protest occurrence is balanced across splits. We then build the train and test set by allocating all observations to the set a locality was randomly assigned to.
Two additional steps involve the preparation of covariate data for the machine learning approach: (i) For categorical data we employ standard one-hot encoding (Lantz Citation2019) – a technique that re-codes categorical variables as a series of binary variables representing whether or not a given level of the categorical variable is present;Footnote3 and (ii) We use k-nearest neighbours imputation (Wilson and Martinez Citation2000) for missing values on any of the covariate dimensions, a robust and well-established pre-processing step that ensures no missing values on any of the covariate dimension.Footnote4
Full model. In training the full model, we follow standard best practices used in machine learning. The spatial nature of our data raises the concern that protest occurrence in adjacent localities may be correlated. We consequently train the model using repeated k-fold cross-validation. That is, we repeat the training for randomly reshuffled observations within the train set. Throughout the study, we set k = 10 and repeat the process five times to obtain less noisy estimates per fold. This yields reliable mean estimates and confidence bounds for the model's in-sample performance.
The random forest model uses the standard implementation of the randomForest function of the package with the same name in the statistical programming language R (Liaw and Wiener Citation2002). To train the model, we use k-fold cross-validation, and to avoid over-fitting we regularize the models using the number of predictors sampled for splitting at each node (see Mentch and Zhou Citation2020). We use the caret-package (Kuhn et al. Citation2020) to implement a systematic grid search to identify the optimal number of trees (Kuhn et al. Citation2013) and do not restrict the maximum number of terminal nodes, instead allowing trees to grow to their maximum depth. Supplementary Section B2 in the Online Appendix illustrates the grid search results for the full and minimal random forest models. Out-of-sample accuracy was assessed on the separate test dataset, featuring the remaining 20% of observations. For the baseline model, we train a generalized linear model (GLM) with Poisson distribution, provided by the glm function of the stats-package in R (R Core Team Citation2013). The model is again trained on the train set using k-fold cross validation, after which we evaluate its performance on the test set.
Minimal Model. The minimal predictive models follow directly from the results of the full model using the 20 most influential covariates identified in the previous step. The models are trained and evaluated in exactly same way. The rationale for specifying minimal models is two-fold. Whereas feature importance for the random forest classifier does not have the same straightforward interpretation as the baseline logistic regression model, the performance or predictive power of two equally sized minimal models can be directly compared, including whether the models use a similar or different set of key predictors. And model performance may actually increase for smaller set of predictors, while at the same time simplifying interpretability. Standard variable importance following the permutation principle – the ‘mean decrease in accuracy’ if a covariate was left out of the model – was computed using the party-package (Hothorn et al. Citation2015).Footnote5
Robustness Checks. Whereas model performance is evaluated on locality-split data that preserves the temporal structure of observations, we assess the robustness of our results by implementing a temporal split of the data, training the models on the first 95 consecutive weeks and evaluating their performance on the last 30 weeks across all local authorities (see Supplementary Section C1 in the Online Appendix). We also test the performance of our machine learning algorithm across prediction tasks. As such, we use cross-predictions to assess the accuracy of random forest models trained on COVID protests to predict non-COVID protests and vice-versa (see Supplementary Section B in the Online Appendix). In addition, we calculate classification accuracy of binary protest occurrence per locality-week for both models, and interpret it relative to model performance on the regression tasks (see Supplementary Section C2 in the Online Appendix). While the random forest is suited for both binary and count prediction tasks, we implement a standard binomial logistic regression model as the binary counterpart to a Poisson model in the GLM family and use this as a baseline.
Results
Model performance
summarizes quantitative model fit for combinations of sampling techniques (locality vs. temporal split), models (GLM vs. random forest) and covariates (full vs. minimal models) used to predict the number of COVID and non-COVID protests based on the training data. Our aim is to identify the best model for each protest outcome in terms of maximum in-sample fit. While Poisson regression is representative of the widely-used family of Generalized Linear Models (GLMs) and especially well-suited to count data, the non-linear random forest (RF) models outperform this baseline in terms of model fit, regardless of protest type: the baseline GLM captures between 21% and 23% of variation ( for the full model); the minimal random forest model explains 71% of in-sample variability of COVID protest occurrence (
).
Table 1. Overview of quantitative model fit.
Given the stronger performance of the random forest models, we focus on those outcomes for the remainder of the manuscript and relegate details that pertain to the baseline Poisson count model to Supplementary Section B1 in the Online Appendix. The minimal random forest model, which uses the top-20 predictors identified in the full model, has the smallest overall error () while capturing 71% of in-sample variability of COVID protest occurrence (
). The full random forest model, with all 60 indicators, performs less well in terms of error, with significantly lower explanatory power (
). For non-COVID protests, the in-sample performance of the full random forest model is comparable to the baseline Poisson count regression model, while the relative explanatory power almost doubles in the minimal model (
), results that remain largely unchanged for the temporal split-samples.
With respect to the out-of-sample results (see Supplementary Table S4), the random forest classifier consistently performs well on the test set when using the spatial, locality-based split. The overall predictive performance is relatively lower but comparable to the in-sample performance on the train set – the full COVID model explains about 47% of out-of-sample variability (,
). For the temporal split – predicting the last 9 months out-of-sample – the random forest model performs well for non-COVID protests but, given the lower number of COVID protests in that period, the performance drops as expected. Supplementary Section C in the Online Appendix details the validation results for all sub-models. Together, these tests suggest that our main findings are robust to the specification of our dependent variable.
The overall predictive power of the random forest model underscores the value added of our machine-learning approach, given the multi-faceted nature of protests, complexity of protest dynamics and interdependence of key protest drivers. Given its ability to capture non-linear relationships, model fit remains consistently high regardless of protest outcomes, spatial or temporal splits. Feature selection, in particular, more consistently identifies key drivers and their relevance, consistent in both full and minimal models (not the case for the baseline linear model).
Key drivers of COVID-19 protests
Our machine learning approach permits data-driven identification of key drivers for the protest patterns. As noted, we have no strong theoretical priors as to which of the four mechanisms – grievance, structure, coercion and opportunity – contribute to driving protest dynamics in Israel. Hence, identifying key drivers associated with one or several of these mechanisms permits us to discriminate between different plausible explanations. lists the top-20 drivers of COVID (left) and non-COVID protests (right) identified by the random forest model, according to their permutation importance – that is, the relative decrease in accuracy (Mean-Standard-Error) if a given indicator was excluded from the model.
Figure 3. Key Drivers of COVID-19 (left) and non-COVID Protests (right).
Notes: The top-20 indicators were derived from the full set of 60 potential drivers using the cross-validated random forest models for each protest outcome. Variable importance is measured as permutation importance, i.e. the relative decrease in accuracy if an indicator was excluded from the model.

As illustrated in , local COVID protests in Israel are driven primarily by political opportunity and coercion. The top-7 drivers of COVID protests consist of local infection dynamics (measured by weekly tests, cases and hospitalizations) and government responses (measured as COVID-19 response indices). In sum, these seven indicators account for almost two thirds of overall variable importance (some 64%). If the three proxy measures for political opportunity are excluded, this results in a 34% relative decrease in model accuracy; the four coercion-based government response measures account for the remaining 30%. Note that population size, underpinning the basic assumption that protest is more likely to occur in localities with more inhabitants (Eisinger Citation1973), constitutes another opportunity-based indicator, albeit with little explanatory power in itself. Contrary to expectation, grievances are marginally significant as a predictor for COVID protests. While locality specific property taxes (for residential, commercial and industry buildings) drive protests, ethnic, religious or identity-based grievances are not amongst the key drivers. And finally, structure is the least important driver for the emergence of COVID protests. The percentage of people aged 65 years or older features amongst the top-20 indicators, suggesting this vulnerable segment was either more or less likely to protest – for better health care or due to physical constraints, respectively. Note that the logic of random forest classifiers does not lend itself to inferring the directionality of associations. That is, for more nuanced explorations on the association between COVID protests and the population aged 65 years and more, additional analysis would be required.
COVID-19 vs. non-COVID protests
To what extent, then, do the drivers of COVID protests overlap with those of non-COVID protests? Our data-driven approach lends itself to a direct, side-by-side comparison of relative influence. The comparison in suggests that the random forest models for each type of protest – with comparable performance – identify a similar set of key drivers. Indeed, 15 of 20 key indicators are the same across both protest types. That said, the ranking of mechanisms for non-COVID protest is more or less the inverse of the raking for COVID drivers. While protest directly related to the pandemic is driven largely by political opportunity and coercion, the relative influence of COVID on the occurrence of non-COVID protests during the pandemic period is far less pronounced. Indicators for COVID prevalence – cases, tests, hospitalizations and economic support – are not among the top-10 drivers for non-COVID protests.
For non-COVID protests, 10 of the 20 structural indicators account for approximately 40% of overall variable importance – pointing to the role that access to affordable housing, the quality of education and demography play – although their relative influence as driving factors is more pronounced here (the percentage of people aged 65 years or older does not feature amongst the top-20 drivers of non-COVID protest). Grievance is the second major underlying explanation for non-COVID protest, with property taxes, life expectancy, municipality budget and the number of child support beneficiaries accounting for approximately 38% of the relative decrease in model accuracy. Many local governments in Israel, facing acute fiscal crises (Carmeli Citation2008), were unable to ensure the provision of basic services during the pandemic (Thomas Citation2021). While most domains of public service provision, for instance education and health, are to some extent co-financed by the national government, revenue raised from residential and commercial property tax, the so-called ‘Arnona’, accounts for somewhere between 50 to 90% of annual municipality budgets (Carmeli Citation2008). During the pandemic, high inflation combined with high property taxes were likely to have sparked protests in localities across the country – suggesting that even non-COVID protests were indirectly affected by the pandemic.
Given a large degree of overlap between drivers of COVID and non-COVID protest – albeit with different degrees of importance – we assess whether the underlying mechanisms for COVID and non-COVID protests, as captured by the random forest classifier, are separable. We utilize cross-prediction for this task – training the random forest model on COVID protest incidents and using the model to predict non-COVID protest and vice versa (further details are provided in Supplementary Table S4 in the Online Appendix.).Footnote6 We find that the model trained on non-COVID protests has predictive power for COVID protests (Pearson's r = .66, ) suggesting that that the same set of structural drivers make localities vulnerable to both types of protest. In contrast, the model trained on COVID protests cannot predict non-COVID protest (Pearson's r = .03 and
). As such, the top-7 drivers directly related to the pandemic correspond to mechanisms that can be distinguished from those for non-COVID protest. It follows that despite a large overlap between the top-20 drivers for both types of protests, the cross-prediction suggests that COVID protests, in particular, were distinct.
Discussion
In March 2020, the pandemic effectively compounded the ongoing constitutional and electoral crisis (Hitman Citation2021; Maor, Sulitzeanu-Kenan, and Chinitz Citation2020). This ‘double-crisis’ (Hitman Citation2021) sparked waves of protest across the country, with some localities experiencing more protests against COVID-19 measures relative to others. Savyon, located East of Tel Aviv, is one of the wealthiest municipalities across the country. Its 4000 inhabitants were only mildly affected by the pandemic (approximately 3 out of 10 inhabitants) yet engaged in 11 protests against COVID-19 measures. The 2700 similarly affluent inhabitants of Rosh Pinna in the Upper Galilee, moderately affected by COVID-19 (approximately 5 out of 10 inhabitants) engaged in 10 protests, none of which directly related to the pandemic. The residential property tax in Rosh Pinna is 2,115 NIS/, almost twice that of Savyon. Jisr Az-Zarqa, located south of Haifa, is a poverty-stricken and densely populated Israeli Arab town with approximately 13,000 inhabitants and a residential property tax rate of 348 NIS/
. The town was heavily affected by the pandemic (approximately 7 out of 10 inhabitants) but did not experience any protests. Nor did Ghajar, a Syrian Alawite village in the Golan Heights, with 2700 inhabitants and the country-wide lowest residential property tax rate of 81 NIS/
. The village was least affected by COVID-19 (approximately 2 out of 10 inhabitants).
Why then did some of localities experience protests while others did not? And how do key drivers of COVID protests compare to those of non-COVID protests? In an effort to disentangle the underlying motives and mechanisms, we find that all protests during the COVID outbreak, regardless whether they were directly related to the pandemic or not, were largely motivated by the same set of key indicators. Local infection rates and government responses motivated all protests during the pandemic to a certain extent, although the relative importance of pandemic-related drivers was more pronounced for predicting COVID protests. Differences in residential and commercial property tax, access to affordable housing, the quality of education and demography are among the most important drivers of non-COVID protests.
Our analysis underscores the role that local governments played in the managing the pandemic. While the powers of local authorities are typically limited (Beeri Citation2021), the Israeli Cabinet adopted a four-tiered ‘Traffic Light Model’ to apply COVID-related measures according to locality-specific infection rates (Cohen-Almagor Citation2021). Yet, even as local governments were tasked to provide essential services and goods during phases of lockdown (Waitzberg et al. Citation2020), the ongoing emergency broadened the gap between revenue, from taxes for instance, and expenditure, with a significant effect on protest activity. Thus, while political opportunity and coercion explain COVID-related protest activity, slow-moving indicators for grievances and structure – while not insignificant for COVID protests – are better suited to explain non-COVID protests. This is evident when considering the role of property taxes. While local government effectively set taxes every year, these rates are remain fixed in the short to medium term. Arguably, locality specific characteristics also shape other grievance related indicators, such as ‘high schools’ and the number of new immigrants, albeit over the long term.
With respect to methodology, our key contribution lies in the application of machine learning approaches to an analysis of protest activity. The approach we adopt has distinct advantages over traditional statistical models as the complexity of protest patterns increases – that is when several plausible mechanisms and associated covariate dimensions co-exist – and when there are no strong theoretical priors to identify the mechanisms and dimensions that matter most. In such settings, the ability of machine learning approaches to identify key drivers in a data-driven fashion is clearly superior. That said, the random forest classifier does not lend itself to identifying the directionality of effects, in contrast to the GLM, which performs less well on the prediction task but permits a straightforward interpretation of directionality. We consequently advocate the use of multiple models given no a priori reason to select a particular approach, and in keeping with the notion that no single model is likely to capture all relevant aspects of a problem (Page Citation2018, Chapter 1). As such, we consider the machine learning approach we use as a complement to other, well-established approaches for the study of protest events.
Supplemental Material
Download PDF (1.2 MB)Disclosure statement
No potential conflict of interest was reported by the author(s).
Data Availability availability statement
All data used in this study draw on publicly available resources and we provide details on all variables used in the Online Appendix. Protest data combines direct COVID-19 disorder events with regular disorder events in Israel and Palestine, both available via www.acleddata.com. Annual survey data on various locality characteristics, are provided by the Israeli Central Bureau for Statistics (CBS) available at www.cbs.gov.il. Information on local COVID-19 dynamics is available from the Israeli Ministry of Health https://www.gov.il/he/Departments/General/database. Data on Israeli government restrictions in response to COVID-19 are obtained from the Oxford COVID-19 Government Response Tracker (Citation2023). All datasets and replication code are available via the Harvard Dataverse, a FAIR-compliant data repository: https://doi.org/10.7910/DVN/E6GXGZ.
Notes
1 Machsom is the Hebrew expression for ‘checkpoint’.
2 While ACLED researchers working on Israel and Palestine are provided with special training to better understand the regional conflict and media environment, and despite the extensive details on newspaper sources and coding procedures provided online (ACLED Citation2020b), ACLED still suffers from known reporting biases. For instance, we do not consider the number of participants in our analysis, due to inconsistencies in the reporting across different pro-Israeli and pro-Palestinian sources. In addition, municipality-level protests may be under-reported as the data include little coverage of sub-national newspaper outlets for many regions in Israel. In fact, ACLED's coding is mainly based on reports by national outlets that may not cover smaller protests or activities in more remote localities. We note, further, that the conceptualizations of key variables in our dataset may not fully reflect the actual complexity of local protest patterns in Israel. One example is our classification of localities according to the ethnicity of the majority population group. To validate this measure, we systematically compare whether the group indicated as protest actor in the ACLED data matches the majority group in the locality – Israeli vs. Palestinian. And, while we acknowledge that peaceful and violent forms of protest may not be driven by the same mechanisms (Geschwender Citation1968), an initial screening of the incident data indicated that approximately 90% of protests were peaceful and that only three events in the entire 125-week period reported the use of excessive force against protesters in Israel. We therefore do not differentiate protests according to whether they were violent or not in our analysis
3 A categorical variable with three levels is consequently coded as three binary variables, the advantage being that it correctly represents a categorical variable for which no ordered relationships exists.
4 Note that the initial fraction of missing covariate values is quite low with 5.9%.
5 We consistently identify the same key predictors using conditional variable importance (Strobl et al. Citation2008), which adjusts for correlations between indicators; see Section C4 in the Online Appendix for details.
6 We do not report results for the GLM models since they are characterized by significant over-fitting.
References
- ACLED. 2020a. “Coronavirus-Related Events in the Acled Dataset.” Armed Conflict Location & Event Data Project. Accessed February 10, 2023. https://acleddata.com/acleddatanew/wp-content/uploads/dlm_uploads/2020/04/ACLED_Direct-COVID19-Disorder_Methodology-Brief_4.2020.pdf.
- ACLED. 2020b. “Israel and Palestine Sourcing Profile.” Armed Conflict Location & Event Data Project. Accessed April 10, 2023. https://acleddata.com/acleddatanew/wp-content/uploads/2021/11/ACLED_Israel-Palestine-Sourcing-Profile_April-2020.pdf.
- ACLED. 2023a. “Direct Covid-19 Disorder Events.” Armed Conflict Location & Event Data Project. Accessed February 10, 2023. https://acleddata.com/analysis/covid-19-disorder-tracker.
- ACLED. 2023b. “Disorder Events in Israel and Palestine.” Armed Conflict Location & Event Data Project. Accessed February 10, 2023. https://acleddata.com/data-export-tool/.
- Al-Sayed, K., and S. Hanna. 2013. “How City Spaces Afford Opportunities for Riots.” In Proceedings of the Ninth International Space Syntax Symposium, edited by Y. O. Kim, H. T. Park, and K. W. Seo, 1–12. Seoul: Sejong University.
- Alimi, E. Y. 2012. “Occupy Israel: A Tale of Startling Success and Hopeful Failure.” Social Movement Studies 11 (3-4): 402–407. https://doi.org/10.1080/14742837.2012.708921.
- Barak, Y. 2020. “Israel's Daylight Saving Time Controversy.” Israel Affairs 26 (5): 698–715. https://doi.org/10.1080/13537121.2020.1806564.
- Beeri, I. 2021. “Lack of Reform in Israeli Local Government and Its Impact on Modern Developments in Public Management.” Public Management Review 23 (10): 1423–1435. https://doi.org/10.1080/14719037.2020.1823138.
- Berkowitz, L. 1989. “Frustration-Aggression Hypothesis: Examination and Reformulation.” Psychological Bulletin 106 (1): 59–73. https://doi.org/10.1037/0033-2909.106.1.59.
- Borland, E., and B. Sutton. 2007. “Quotidian Disruption and Women's Activism in Times of Crisis, Argentina 2002–2003.” Gender & Society 21 (5): 700–722. https://doi.org/10.1177/0891243207306383.
- Carey, S. C. 2006. “The Dynamic Relationship Between Protest and Repression.” Political Research Quarterly 59 (1): 1–11. https://doi.org/10.1177/106591290605900101.
- Carmeli, A. 2008. “The Fiscal Distress of Local Governments in Israel: Sources and Coping Strategies.” Administration & Society 39 (8): 984–1007. https://doi.org/10.1177/0095399707309358.
- Castells, M. 1983. The City and the Grassroots: A Cross-Cultural Theory of Urban Social Movements. Berkeley, CA: University of California Press.
- CBS. 2020. “Regional Statistics.” Israeli Central Bureau For Statistics. Accessed February 10, 2023. https://www.cbs.gov.il/en/settlements/Pages/default.aspx?mode=Yeshuv.
- CBS. 2023. “Glossary: Locality.” Israeli Central Bureau For Statistics. Accessed February 10, 2023. https://www.cbs.gov.il/en/Pages/All-Terms.aspx?k=Locality.
- Cederman, L. E. 2005. “Computational Models of Social Forms: Advancing Generative Process Theory.” American Journal of Sociology 110 (4): 864–893. https://doi.org/10.1086/426412.
- Cederman, L. E., K. S. Gleditsch, and H. Buhaug. 2013. Inequality, Grievances, and Civil War. Cambridge: Cambridge University Press.
- Cederman, L. E., A. Wimmer, and B. Min. 2010. “Why Do Ethnic Groups Rebel? New Data and Analysis.” World Politics 62 (1): 87–119. https://doi.org/10.1017/S0043887109990219.
- Chen, R. C., C. Dewi, S. W. Huang, and R. E. Caraka. 2020. “Selecting Critical Features for Data Classification Based on Machine Learning Methods.” Journal of Big Data 7 (1): 1–26. https://doi.org/10.1186/s40537-020-00327-4.
- Cohen-Almagor, R.. 2021. “Israel Facing Covid-19.” Public Governance, Administration and Finances Law Review 6 (1): 7–17. https://doi.org/10.53116/pgaflr.2021.1.2.
- Collier, P., and A. Hoeffler. 2004. “Greed and Grievance in Civil War.” Oxford Economic Papers 56 (4): 563–595. https://doi.org/10.1093/oep/gpf064.
- Davenport, C., and M. Inman. 2012. “The State of State Repression Research Since the 1990s.” Terrorism and Political Violence 24 (4): 619–634. https://doi.org/10.1080/09546553.2012.700619.
- Eisinger, P. K. 1973. “The Conditions of Protest Behavior in American Cities.” The American Political Science Review 67 (1): 11–28. https://doi.org/10.2307/1958525.
- Fleischmann, L.. 2021. “Protest Movements and Democracy in Israel.” In The Palgrave International Handbook of Israel, edited by P.R. Kumaraswamy, 1–15. London: Springer.
- Frankel, O.. 2020. “Blackness in Translation: The Israeli Black Panthers, 1971.” In Blackness in Israel, edited by U. Dorchin and G. Djerrahian, 91–112. New York, NY: Routledge.
- Geschwender, J. A. 1968. “Civil Rights Protest and Riots: A Disappearing Distinction.” Social Science Quarterly 49 (3): 474–484.
- Goldberg, A. 2010. “Life As Politics: How Ordinary People Change the Middle East.” Canadian Journal of Sociology (Online) 35 (3): 485. https://doi.org/10.29173/cjs8882.
- Gould, R. V. 1991. “Multiple Networks and Mobilization in the Paris Commune, 1871.” American Sociological Review 56 (6): 716–729. https://doi.org/10.2307/2096251.
- Granovetter, M. 1978. “Threshold Models of Collective Behavior.” American Journal of Sociology 83 (6): 1420–1443. https://doi.org/10.1086/226707.
- Gupta, D. K., H. Singh, and T. Sprague. 1993. “Government Coercion of Dissidents: Deterrence Or Provocation?” Journal of Conflict Resolution 37 (2): 301–339. https://doi.org/10.1177/0022002793037002004.
- Hale, T., N. Angrist, R. Goldszmidt, B. Kira, A. Petherick, T. Phillips, S. Webster, et al. 2021. “A Global Panel Database of Pandemic Policies (Oxford COVID-19 Government Response Tracker).” Nature Human Behaviour 5 (4): 529–538. https://doi.org/10.1038/s41562-021-01079-8.
- Hananel, R., and H. Nachmany. 2021. “Housing in the Face of Crisis: The Influence of Israel's 2011 Protest on Housing Policy.” Political Geography 90:102460. https://doi.org/10.1016/j.polgeo.2021.102460.
- Hermann, T. 1996. “Do they Have a Chance? Protest and Political Structure of Opportunities in Israel.” Israel Studies 1 (1): 144–170. https://doi.org/10.2979/isr.1996.1.issue-1.
- Hillier, W., T. Yang, and A. Turner. 2012. “Normalising Least Angle Choice in Depthmap-and How it Opens Up New Perspectives on the Global and Local Analysis of City Space.” Journal of Space Syntax 3 (2): 155–193. https://access.portico.org/stable?au=pjb405bhmh2.
- Hitman, G. 2021. “More Divided Than United: Israeli Social Protest During Covid-19 Pandemic of 2020.” Cogent Social Sciences 7 (1): 1994203. https://doi.org/10.1080/23311886.2021.1994203.
- Hitman, G. 2022. “Haredi Anti-Zionist Ideology As the Driving Force Behind the Ultraorthodox Protests in Israel.” Contemporary Review of the Middle East9 (4): 426–443. https://doi.org/10.1177/23477989221115293.
- Hothorn, T., K. Hornik, C. Strobl, A. Zeileis, and M. T. Hothorn. 2015. “Package ‘Party’.” https://cran.r-project.org/package=party.
- Hunger, S., S. Hutter, and E. Kanol. 2023. “The Mobilisation Potential of Anti-Containment Protests in Germany.” West European Politics 46 (4): 812–840. https://doi.org/10.1080/01402382.2023.2166728.
- Israel Ministry of Health. 2023. Covid-19 by Area Accessed February 10, 2023. https://data.gov.il/dataset/covid-19/resource/d07c0771-01a8-43b2-96cc-c6154e7fa9bd.
- Kaufman, I. 2008. “Resisting Occupation or Institutionalizing Control? Israeli Women and Protest in West Bank Checkpoints.” International Journal of Peace Studies 13 (1): 43–62.
- Kriesi, H., and I. E. Oana. 2022. “Protest in Unlikely Times: Dynamics of Collective Mobilization in Europe During the COVID-19 Crisis.” Journal of European Public Policy 30 (4): 740–765. https://doi.org/10.1080/13501763.2022.2140819.
- Kuhn, M., K. Johnson, M. Kuhn, and K. Johnson. 2013. “Classification Trees and Rule-Based Models.” Applied Predictive Modeling 369–413. https://doi.org/10.1007/978-1-4614-6849-3.
- Kuhn, M., J. Wing, S. Weston, A. Williams, C. Keefer, A. Engelhardt, and T. Cooper, et al. 2020. “Package ‘Caret’.” https://cran.r-project.org/package=caret.
- Kuran, T. 1991. “Now out of Never: The Element of Surprise in the East European Revolution of 1989.” World Politics 44 (1): 7–48. https://doi.org/10.2307/2010422.
- Kurer, T., S. Häusermann, B. Wüest, and M. Enggist. 2019. “Economic Grievances and Political Protest.” European Journal of Political Research 58 (3): 866–892. https://doi.org/10.1111/ejpr.2019.58.issue-3.
- Lantz, B. 2019. Machine Learning with R: Expert Techniques for Predictive Modeling. Birmingham: Packt publishing ltd.
- Liaw, A., and M. Wiener. 2002. “Package ‘Randomforest’.” https://cran.r-project.org/package=randomForest.
- Lichbach, M. I. 1987. “Deterrence Or Escalation?: The Puzzle of Aggregate Studies of Repression and Dissent.” Journal of Conflict Resolution 31 (2): 266–297. https://doi.org/10.1177/0022002787031002003.
- Maor, M., R. Sulitzeanu-Kenan, and D. Chinitz. 2020. “When COVID-19, Constitutional Crisis, and Political Deadlock Meet: The Israeli Case From a Disproportionate Policy Perspective.” Policy and Society 39 (3): 442–457. https://doi.org/10.1080/14494035.2020.1783792.
- Marom, N. 2013. “Activising Space: The Spatial Politics of the 2011 Protest Movement in Israel.” Urban Studies 50 (13): 2826–2841. https://doi.org/10.1177/0042098013477699.
- Mentch, L., and S. Zhou. 2020. “Randomization As Regularization: A Degrees of Freedom Explanation for Random Forest Success.” Journal of Machine Learning Research 21 (171): 1–36. http://jmlr.org/papers/v21/19-905.html
- Neumayer, E., K. G. Pfaff, and T. Plümper. 2023. “Protest Against Covid-19 Containment Policies in European Countries.” Journal of Peace Research (forthcoming). https://doi.org/10.1177/00223433221135335.
- Noelle-Neumann, E. 1974. “The Spiral of Silence: A Theory of Public Opinion.” Journal of Communication 24 (2): 43–51. https://doi.org/10.1111/j.1460-2466.1974.tb00367.x.
- Oxford COVID-19 Government Response Tracker. 2023. Blavatnik School of Government, University of Oxford. Accessed April 1, 2023. https://raw.githubusercontent.com/OxCGRT/covid-policy-tracker/master/.
- Page, S. E. 2018. The Model Thinker: What You Need to Know to Make Data Work for You. New York, NY, USA: Basic Books.
- Parkinson, J. 2012. Democracy and Public Space: The Physical Sites of Democratic Performance. Oxford: Oxford University Press.
- Plümper, T., E. Neumayer, and K. G. Pfaff. 2021. “The Strategy of Protest Against Covid-19 Containment Policies in Germany.” Social Science Quarterly 102 (5): 2236–2250. https://doi.org/10.1111/ssqu.v102.5.
- Raleigh, C., A. Linke, H. Hegre, and J. Karlsen. 2010. “Introducing ACLED: An Armed Conflict Location and Event Dataset: Special Data Feature.” Journal of Peace Research 47 (5): 651–660. https://doi.org/10.1177/0022343310378914.
- R Core Team. 2013. “R: A Language and Environment for Statistical Computing [Computer software manual].” Vienna, Austria: http://www.R-project.org/ (ISBN 3-900051-07-0).
- Routledge, P. 2003. “Convergence Space: Process Geographies of Grassroots Globalization Networks.” Transactions of the Institute of British Geographers 28 (3): 333–349. https://doi.org/10.1111/1475-5661.00096.
- Sørensen, J. F. L., and M. Christiansen. 2022. “The Role of Economic Stress, Health Concerns, and Institutional Trust in Supporting Public Protests Against COVID-19 Lockdown Measures in Denmark.” International Journal of Environmental Research and Public Health 20 (1): 148. https://doi.org/10.3390/ijerph20010148.
- Strobl, C., A. L. Boulesteix, T. Kneib, T. Augustin, and A. Zeileis. 2008. “Conditional Variable Importance for Random Forests.” BMC Bioinformatics 9:1–11. https://doi.org/10.1186/1471-2105-9-307.
- Thomas, A.. 2021. “Reforming the Taxation of Housing in Israel.” OECD Taxation Working Papers 53: 1–43.
- Tilly, C. 1978. From Mobilization to Revolution. New York, NY: McGraw-Hill.
- Trentmann, F.. 2009. “Disruption is Normal: Blackouts, Breakdowns and the Elasticity of Everyday Life.” In Time, Consumption and Everyday Life: Practice, Materiality and Culture, edited by E. Shove, F. Trentmann, and R. Wilk, 67–84. New York, NY: Routledge.
- van der Zwet, K., A. I. Barros, T. M. van Engers, and P. M. Sloot. 2022. “Emergence of Protests During the COVID-19 Pandemic: Quantitative Models to Explore the Contributions of Societal Conditions.” Humanities and Social Sciences Communications 9 (1). https://doi.org/10.1057/s41599-022-01082-y.
- Waitzberg, R., N. Davidovitch, G. Leibner, N. Penn, and S. Brammli-Greenberg. 2020. “Israel's Response to the COVID-19 Pandemic: Tailoring Measures for Vulnerable Cultural Minority Populations.” International Journal for Equity in Health 19 (1): 1–5. https://doi.org/10.1186/s12939-020-01191-7.
- Warhurst, C., C. Tilly, and M. Gatta. 2017. A New Social Construction of Skill. Oxford: Oxford University Press.
- Wilson, D. R., and T. R. Martinez. 2000. “Reduction Techniques for Instance-Based Learning Algorithms.” Machine Learning 38 (3): 257–286. https://doi.org/10.1023/A:1007626913721.
- Wimmer, A. 2008. “The Making and Unmaking of Ethnic Boundaries: A Multilevel Process Theory.” American Journal of Sociology 113 (4): 970–1022. https://doi.org/10.1086/522803.