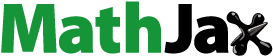
Abstract
Background
Effectiveness of heart rate variability (HRV) biofeedback is dependent on the relationship between the measured respiratory-heart rate coherence (CHR) and the resonant frequency (RFB) breathing. CHR is driven by the central autonomic network which consists of the interplay between the components of the autonomic nervous system, brainstem modulatory nuclei and cortical function. The extent to which heart rate is in coherence with respiratory frequency and the function of the central autonomic network in major depressive disorder (MDD) and suicidal ideation (SI) has not been investigated. Method: Sixty-one patients provided informed consent to participate and were divided into no MDD (CONT), MDD and an MDD plus SI (MDDSI) group. HRV activity was determined by multi lag tone-entropy (T-E) and respiratory-CHR at rest was characterized by the relationship between respiratory rate and heart rate. Results: CONT had the highest entropy compared to the MDDSI group, which had the lowest entropy and highest tone (p < 0.05). Autonomic parasympathetic function was also significantly lower in the MDDSI compared to the MDD group (p < 0.05). CHR indicated a significant phase desynchronization (decrease in coherence) between MDDSI, MDD and CONT (p < 0.05), with MDDSI having the lowest coherence and CONT the highest. Conclusion: T-E analysis indicated that HRV was significantly different in patients with SI. CHR as measured by our proposed synchronization index provides a novel feature to adapt HRV biofeedback to individual psychiatric profiles and may provide better clinical outcomes for this patient group.
Introduction
Major depressive disorder with or without suicidal ideation (SI) is on the rise worldwide [Citation1]. The etiology of major depressive disorder (MDD) is multifactorial with both genetic and environmental factors playing a large part. However, central and peripheral nervous system dysfunction are also associated with MDD and include changes in sleeping patterns, fatigue and weight changes suggesting autonomic nervous system involvement. Central nervous system involvement includes changes in neural network connectivity and associated neurotransmitter regulation involved in cortical and limbic system functionality, which manifest not only in emotion dysregulation but also loss of higher cognitive function leading to SI in this clinical group [Citation2]. Previous research has indicated that emotional dysregulation may precede or is certainly a causative or associative factor to suicidality [Citation3]. Current interventions concentrate on psychotherapy and medication [Citation4,Citation5]. However around 30% of this clinical group does not respond to either behavioral therapy or anti-depressive medication having led to the search for alternative therapies including repetitive transcranial magnetic stimulation (rTMS), transcranial direct current Sstimulation (tDCS) and vagal nerve stimulation (VNS) [Citation6,Citation7]. Many of these are in the experimental stage or invasive and have not provided clear positive outcomes [Citation8,Citation9].
Mental and emotional self-regulation often characterizes stress, anxiety and depression, which is linked to the activity of the vagus nerve and implicated in emotion regulation, reduction in stress and improvement in social interaction. Efferent and afferent branches of the vagus nerve modulate heart and respiratory system function, which in turn is linked with subcortical and cortical areas via brainstem modulatory systems making up a central autonomic network. Porges [Citation10] and Thayer ET AL. [Citation11] have both suggested models that include the central autonomic network (CAN) and its connection between heart rate and respiratory rate synchronization, brainstem nuclei and their connection to subcortical and cortical areas associated with mental and physical health. Coherence or synchronization can be assessed by measuring the changes in the correlation between changes in the heart rate and respiration. On this basis heart rate variability (HRV) biofeedback has been implemented for diverse mental health conditions [Citation12,Citation13].
HRV as a marker for depression and suicide risk
Physiological evidence-based diagnostic methods and interventions need to be implemented at a population, group and individual level to reduce mental illness and prevent suicide. Alternative automated diagnostic tools can be used in conjunction with a psychiatric evaluation or as a stand-alone screening, as part of a community health initiative to provide additional diagnostic tools.
Biological indicators of suicide risk, although useful are often invasive and time consuming. Heart rate changes over time HRV can be quantified as HRV by determining the standard deviation as a temporal measure or the frequency distribution of heart rate from a short heart rate or electrocardiogram (ECG) recording. HRV has been used as an indicator of central autonomic network function [Citation14,Citation15]. In particularly respiratory sinus arrhythmia (RSA), the high frequency component of HRV, has been linked to cardiac vagal tone in studies using autonomic blockade [Citation16] and recognized as an index of vagal-cardiac nerve activity [Citation17]. Decreased HRV and RSA are signs of lower vagal activity and MDD [Citation18–20]. Specifically, vagal tone has been associated with emotion regulation and social engagement, both important in mental health and reduced in MDD [Citation20]. Improvement in emotion regulation can be achieved by breathing in the low frequency domain range around 0.1 Hz or six breaths per minute. This breathing frequency leads to an overlap between heart rate increasing with inspiration and heart rate decreasing with expiration in the majority of the population with a predominance of vagal activity. Cardiovagal activity can be explained by the respiratory gate theory. Phasic vagal activity or vagal tone is associated with HRV and the basis why RSA is primarily a vagal phenomenon [Citation21]. Increases in vagal tone and vagal afferent signaling to brainstem nuclei and to subcortical and cortical areas is associated with better resilience, less stress and less depressive symptomatology [Citation22–24].
The analysis of phase synchronization between RSA and inspiration-expiration cycling may provide a new index for evaluating the psychological state of patients with MDD and guide HRV-based biofeedback. Phase synchronization among hemodynamic variables and respiration during a mental challenge has been reported [Citation25], yet quantitative analysis for the phase relationship between RSA and respiration in depressed patients has not been determined in conjunction with HRV measures. Heart rate variability and the heart rate - respiratory rate synchronization may provide a new measure for identifying MDD and suicidal ideation.
Methods
To determine the association between HRV, respiration and depression we first investigated the phase relationship between our multiscale HRV measure and a MDD cohort that included those with and without SI followed by determining the relationship between HRV and respiration in a subgroup of depressed patients. All participants were recruited and attended the Abu Dhabi American Centre for Psychiatry and Neurology in the UAE. Any participants with known cardiovascular disease or found to have abnormal respiration was excluded from this research. Twenty-nine non-depressed subjects and 32 patients with a primary diagnosis of MDD were included in this study. The study was approved by Al Ain District Ethics Committee and all participants provided written informed consent.
Participants
Diagnosis of depression severity and SI was made by the consultant psychiatrist using the mini-international neuropsychiatric interview (M.I.N.I.) [Citation26] and the Hamilton Depression Rating Scale (HAM-D) [Citation27]. The M.I.N.I. was designed as a structured diagnostic interview based on the major psychiatric disorders which are included in the DSM-5 and ICD-10. The standard version of the M.I.N.I. includes items to assess MDD and suicidality. The HAM-D is a multiple item questionnaire used to provide an indication of depression and as a guide to evaluate recovery.
In the current study, participants had to be un-medicated and speak Arabic or English fluently (more details are provided in [Citation28]). The C module of the M.I.N.I., which was made up of nine questions, was used to estimate SI among participants. Exclusions included significant cognitive impairment, other primary psychiatric diagnosis, current chronic disease within the preceding two years. The control group was interviewed by the psychiatrist for screening of mental illnesses and deciding whether further psychiatric tests were required to confirm the diagnoses. In this study, none of the participants in the healthy control group were identified with mental health issues and therefore did not complete the M.I.N.I. interview and the other questionnaires. All participants except the control group also completed the PHQ-9, GAD-7 and BDI. summarizes the demographics of participants for the three groups.
Table 1. Patient demographics and psychiatric scores.
HRV analysis and phase coherence
A 10 min ECG recording was obtained from all participants (Powerlab, AD Instruments,
Australia), using Lab Chart 7.1 and a sampling rate of 1000 Hz with a notch filter set at 50 Hz. High-frequency noise was removed with a 45 Hz low-pass filter and wondering baseline adjusted with a 0.5 Hz high-pass filter. The heart rate interbeat intervals (RR intervals) were obtained using the Pan and Tompkins algorithm [Citation29] and ectopic beats removed manually including heart rate less than 50 or greater than 200 beats/min.
Tone and entropy were determined by first defining the RR interval time series as given below. A RR interval or period is defined as the time difference between two consecutive R peaks of the ECG signal:
(1)
(1)
where, N is the number RR intervals. Heart rate acceleration and deceleration can be determined from the difference of consecutive RR intervals. If RRi+1 become shorter than RRi then it is an acceleration of heart rate. Therefore, acceleration of the heart rate is expressed as a positive difference and inhibition as a negative difference of RR intervals. However, to reduce the impact of heart rate variation over a wide range of possible recoding times during the day and different subjects, a normalized variation in RR interval is preferred to monitor the variability. In conventional tone-entropy (T-E) analysis, percentile changes of the successive RR intervals with respect to the previous RR interval are expressed as the percentage index (PI):
(2)
(2)
Tone is defined as a first order moment (arithmetic average) of this PI time series as:
(3)
(3)
Tone is the balance between accelerations (PI > 0) and inhibitions (PI < 0) of the heart rate and represents the sympatho-vagal balance [Citation30,Citation31]. Entropy is defined from the probability distribution of PI by using Shannon’s formula [Citation32]:
(4)
(4)
where,
is the probability of PI having values in the range
where
is an integer and
is the number of bins where,
The entropy evaluates total acceleration-inhibition activities, or total heart period variations.
For multi-lag T-E analysis, we have introduced the in EquationEquation (2)
(2)
(2) , used to derive the PI time series from the RR time series signal. Lag refers to the number of beats included in the analysis hence a lag or ‘m’ of two indicates two beats, m = 3 is a lag of three beats… Hence, in the multi-lag T-E analysis PI is expressed as the percent change of adding additional beats such that there are the second, third, fourth, fifth, etc. (i-th) beat and (i + m)-th RR intervals with respect to the i-th RR interval and is defined as:
(5)
(5)
where, m = 1 represents the conventional T-E analysis. Tone represents the autonomic balance by showing the net effect of combining sympathetic and parasympathetic nervous system activities. Because a heartbeat influences not only the beat immediately following it, but also up to 6–10 beats downstream [Citation33], tone at higher lags (m) could represent more sympathetic nervous system status in terms of autonomic balance. On the other hand, entropy reflects the gross variance of autonomic activities [Citation30,Citation31]. We analyzed the variation of tone and entropy values with a range of lags (
).
Several time domain HRV parameters were included in the analysis: mean RR, standard deviation of normal RR data (SDNN) and the square root of the mean squared difference of the successive RR data (RMSSD). The latter is an indicator of vagal activity, whereas SDNN is a more global indicator of heart rate variability. Spectral analysis was performed on linearly resampled (4 Hz) time series using Welch’s method [Citation34]. The 256-point fast Fourier transform was repeatedly computed with 50% overlap between adjacent segments. Then the spectral power of each segment was computed and averaged. Hanning window was applied to avoid spectral leakage. Subsequently, spectral powers in the low frequency (LF) band (0.04–0.15 Hz) and high frequency (HF) band (0.15–0.40 Hz) were obtained by integration [Citation35]. The power in the very lower frequency (VLF) band was set at ≤0.04 Hz. For frequency analysis, the HF power also indicates vagal function, the LF power sympathovagal influence on heart rate and VLF indicates long term modulation of heart rate possibly via the endocrine system.
Both RRI and respiration were resampled at 10 Hz and band passed filtered (0.10–0.4 Hz). Hilbert transform was applied to extract instantaneous phases of the RSA [] and respiration [
]. Then time dependent phase coherence (λ) between RSA and respiration was obtained by the following equation:
(6)
(6)
N denotes number of data samples. The λ value ranges from 0(lowest)∼1(highest) coupling.
Statistics
A nonparametric Mann–Whitney U test was performed to determine whether any significant differences in multi-lag T-E are present between healthy (CONT) versus MDDSI patients, CONT versus MDD patients and MDDSI patients versus MDD patients. Values of p < 0.05 were considered significant.
Results
The demographics characteristics of the three groups are shown in . The MDDSI group had significantly higher body mass index (BMI) and waist circumference (WC) and was older compared to the CONT group. The MDDSI group also had a significantly larger WC compared to MDD in addition to the significantly different suicidality score. However, no significant differences for BMI, and age were seen between MDDSI and MDD. A significant difference in age was found between CONT and MDD and MDDSI. Although a significant age difference was found between MDDSI and CONT, the average age difference was nine years with a high age overlap. Taking the work of Agelink ET AL. [Citation36], who investigated changes in HRV associated with aging, there are no significant differences in HRV for ages 26–35 and 36–45 and therefore differences seen in our features are associated with psychopathology.
Tone-entropy at lag 10 indicated a significantly higher tone (p < 0.05) in the MDDSI group whereas entropy significantly decreased (p < 0.05). shows the tone-entropy distribution for lag 10 ().
Table 2. Tone-entropy at different lags (1 to 10).
Averaged data of the three groups are shown by rectangles (mean ± SE). Three rectangles were arranged on the curvilinear relation. Mean entropy value was higher for lag 10 in the CONT group compared to MDDSI. In contrast, the mean entropy value of the MDD group was lower than that of the CONT at lag 10. Interestingly, there are no significant difference in the mean entropy value between the MDDSI and MDD groups ().
summarizes results for T-E analysis and conventional time and frequency domain results. Mean ± SD values of HRV features with p values from Mann Whitney U Test. * indicates statistical differences.
Table 3. Traditional HRV markers for MDDSI, MDD and control.
Time domain parameters decreased significantly (p < 0.05) in the MDDSI group compared to the control (CONT) group. However, only RMSSD was significantly lower in the MDDSI compared to the MDD group (p < 0.05). Frequency domain parameters (LF, HF) also decreased between the MDDSI and CONT group but was not statistically significant.
Heart rate to the respiratory-heart rate coherence
shows three examples of R-R intervals (RRI), Respiratory movement (Resp), Instantaneous phases for breathing and RRI (ϕ) and phase coupling coefficient (λ) between the three groups.
Figure 3. RR interval data and respiratory movement, instantaneous phases for breathing and phase coupling coefficient between MDDSI, MDD and control. R-R intervals (RRI), respiratory movement (Resp), instantaneous phases for breathing and RRI (ϕ) and phase coupling coefficient (λ) from examples of (A) control group (B) MDDSI − group and (C) MDDSI + group.
A higher breathing frequency (fR) was found for the MDD group with suicidal ideation (MDDSI), compared to MDD and CONT but the lowest amplitude of RSA (ARSA) compared to MDD and CONT. The latter having the highest RSA as is expected. The phase coupling coefficient (λ) and HF power were also lowest in the MDDSI group, with a slight increase in the MDD group and highest coefficient in the CONT group ().
Table 4. Cardiorespiratory and HRV variables.
Entropy is a global indicator of autonomic nervous system function, whereas tone indicates the sympathovagal balance. As lag increases more of the sympathetic influence is expected to be present. In the influence of the parasympathetic and sympathetic component can be observed with reference to the phase synchronization between heart and respiratory function. Significant correlations were found between entropy and control/MDD but not with MDDSI at lower lag, whereas at higher lag significant correlation was found between entropy and MDDSI suggesting changes in the function of the predominantly vagal innervation of both the heart and respiratory system.
Table 5. Correlation coefficients (r) of time domain and multi-lag T-E features with Phase synchronization parameter (λ).
Discussion
Understanding of the neurobiological mechanisms of suicidal behavior and associated biological markers is still limited. No currently available single biological marker can reliably predict suicidality, and biological tests for inflammatory cytokines or ECG analysis are not in routine clinical use. Suicidal ideation and self-harm behavior are considered possible complications of untreated depressive illness in combination with other risk factors because suicidal thoughts and behaviorr can be symptoms of moderate to severe depression [Citation37]. Previous studies using HRV analysis of patients with depression have shown significant differences to control groups. However, the current study showed that applying a multi-lag method improved classification especially by differentiating between MDD with suicidal ideation versus MDD without suicidal ideation. The reason why T-E performs better than traditional HRV features could be due to including the normalization process of beat-to-beat heart rate changes by the previous heart beat in calculating T-E values, which removes the baseline biases caused by other underlying factors such as age, BMI or WC.
Neural networks involved in depression and suicidality include the fronto-striatal pathways that link the dorsolateral prefrontal cortex, orbitofrontal cortex, anterior cingulate and dorsal cingulate. Other components of limbic circuitry, in particular the hippocampus and amygdala, have been implicated in depression [Citation38]. These cortical and subcortical areas link to brainstem nuclei and hence allow both a top-down and bottom-up regulation of cognitive and emotional function [Citation25,Citation39]. A link between the two may be the vagus nerve and its connections via the nucleus ambiguus and solitary nucleus in the brainstem with higher cortical areas. Decreased HRV has previously been shown by several researchers to be associated with vagal withdrawal and associated with major depressive disorder as well as in patients with variants including MDD with general anxiety disorder, melancholia or post-traumatic stress disorder or with suicidal ideation [Citation28,Citation40,Citation41]. Chronic disease such as diabetes, which leads to dysregulation of heart rate by affecting vagal function has also been shown to decrease HRV [Citation42]. In line with the bottom-up approach of vagal nerve connectivity is vagus nerve stimulation, which has been successful in addressing treatment-resistant depression [Citation43]. However, a less invasive and a more self-regulatory technique is to use HRV biofeedback. RSA is an important component of HRV biofeedback as is the synchronization or coherence between heart rate and respiration, which optimizes vagal function [Citation44–Citation46]. During HRV biofeedback, based on resonance frequency breathing, the respiratory sinus arrhythmia or the difference between maximum and minimum heart rate oscillations increases and becomes more sinusoidal compared to rest [Citation45]. The effectiveness of HRV biofeedback is primarily due to the phase relationship between heart rate and respiration becoming more coherent or in synchrony at resonant frequency breathing in association with the baroreceptor feedback loop [Citation47].
The present study demonstrated that HRV measured by multi lag T-E is significantly lower in patients with MDD and suicidal ideation compared to patients with MDD only or control participants confirming previous work that the central autonomic network suggested by Porges [Citation10] and Thayer ET AL. [Citation11] provides a link between the cortical networks associated with MDD and brainstem regulation of respiration and heart rate. Further the interaction of respiration and heart rate varies in phase depending on function and mental health, with phase-lag variations between RSA and respiration in MDDSI patients being significantly lower. Disease progression from control to MDD and MDDSI indicate that there is a loss of phase synchronization with increasing lag in the control and MDD group with a concomitant increase in the MDDSI group with entropy. The findings mirror current understanding of the autonomic nervous system innervation pattern of the heart and lungs and the effect of depression on heart rate variability [Citation40,Citation42].
The phase coupling coefficient (λ) showed significant positive correlation with autonomic vagal activity indexes of ARSA and the HF component of HRV, suggesting a possibility that λ may provide information about the extent of parasympathetic dysfunction in depression and possibly a treatment index for HRV biofeedback efficacy to revert the phase synchronization index between RSA and respiratory rate back to a normal level.
A thorough literature search has not revealed any work applying a combination of heart rate variability and respiration rate as a strong indicator of suicidality in patients with depression. However, a strong link between emotion dysregulation and suicidality has been reported being linked to respiratory sinus arrhythmia [Citation3]. Our work has extended the findings of Tsypes ET AL. [Citation48] and indicated a connection between respiration rate and heart rate variability, often referred to as heart rate coherence (CHR), which has been suggested to lead to changes in cortical function, especially in the prefrontal areas that are associated with MDD [Citation49–51]. To bring the current findings into clinical use we are continuing to recruit patients to obtain a larger cohort and over time to obtain a longitudinal database to analyze risk of suicide based on our recommended factors also. In addition, we will include a comprehensive test battery, which provides information on comorbidity such as anxiety and melancholia as well as presence of heart disease.
In conclusion our current work indicates that a reduction in suicidal ideation by HRV biofeedback-guided respiration - CHR may be possible based on the reduction of cardiac and respiratory function and importantly that the heart-respiratory synchronization is much less in the MDD with suicidal ideation group. HRV biofeedback has been shown to improve vagal function and central autonomic network connectivity leading to reduced MDD symptomatology.
Acknowledgments
The authors thank the psychiatric nurses of American Center for Psychiatry and Neurology for providing assistance with patient recruitment and experimental data collection from MDD patients.
Disclosure statement
No potential conflict of interest was reported by the author(s).
Data availability
Data is available on request from the corresponding author.
Additional information
Funding
Notes on contributors
Herbert F. Jelinek
Herbert Jelinek is an associate professor in the Department of Biomedical Engineering, Khalifa University. Dr. Jelinek contributed to the study design, data interpretation and writing of the manuscript.
Ahsan H. Khandoker
Ahsan Khandoker is an associate professor in the Department of Biomedical Engineering, Khalifa University. Dr. Khandoker contributed to the study design, data collection and interpretation and manuscript preparation.
References
- Kessler RC, Bromet EJ. The epidemiology of depression across cultures. Annu Rev Public Health. 2013;34:119–138.
- Maletic V, Robinson M, Oakes T, ET AL. Neurobiology of depression: an integrated view of key findings. Int J Clin Pract. 2007;61:2030–2040.
- Tsypes A, James KM, Woody ML, ET AL. Resting respiratory sinus arrhythmia in suicide attempters. Psychophysiology. 2018;55:e12978.
- Khan A, Faucett J, Lichtenberg P, ET AL. A systematic review of comparative efficacy of treatments and controls for depression. PLoS ONE. 2012;7:e41778.
- Nemeroff CB. The search for treatments for veterans with major depression: of paramount importance, yet still elusive. JAMA Psychiatry. 2018;75:877.
- Al-Harbi KS. Treatment-resistant depression: therapeutic trends, challenges, and future directions. Patient Prefer Adherence. 2012;6:369–388.
- To WT, De Ridder D, Hart Jr J, ET AL. Changing brain networks through non-invasive neuromodulation. Front Human Neurosci. 2018;12:128.
- Yesavage JA, Fairchild JK, Mi Z, ET AL. Effect of repetitive transcranial magnetic stimulation on treatment-resistant major depression in US veterans: a randomized clinical trial. JAMA Psychiatry. 2018;75:884.
- Dell’osso B, Camuri G, Castellano F, ET AL. Meta-review of metanalytic studies with repetitive transcranial magnetic stimulation (RTMS) for the treatment of major depression. Clin Pract Epidemiol Ment Health. 2011;7:167–177.
- Thayer JF, Hansen AL, Saus-Rose E, ET AL. Heart rate variability, prefrontal neural function, and cognitive performance: the neurovisceral integration perspective on self-regulation, adaptation and health. Ann Behav Med. 2009;37:141–153.
- Porges SW. Social engagement and attachment: a phylogenetic perspective. Ann N Y Acad Sci. 2003;1008:31–47.
- Jester DJ, Rozek EK, McKelley RA. Heart rate variability biofeedback: implications for cognitive and psychiatric effects in older adults. Aging Ment Health. 2019;23:574–580.
- Caldwell YT, Steffen PR. Adding HRV biofeedback to psychotherapy increases heart rate variability and improves the treatment of major depressive disorder. Int J Psychophysiol. 2018;131:96–101.
- Yeragani VK, Pohl R, Balon R, ET AL. Heart rate variability in patients with major depression. Psychiatry Res. 1991;37:35–46.
- Stein PK, Bosner MS, Kleiger RE, ET AL. Heart rate variability: a measure of cardiac autonomic tone. Am Heart J. 1994;127:1376–1381.
- Akselrod S, Gordon D, Ubel FA, ET AL. Power spectrum analysis of heart rate fluctuation: a quantitative probe of beat-to-beat cardiovascular control. Science. 1981;213:220–222.
- Grossman P, Wilhelm FH, Spoerle M. Respiratory sinus arrhythmia, cardiac vagal control, and daily activity. Am J Physiol Heart Circ Physiol. 2004;287:H728–34.
- Khandoker AH, Luthra V, Abouallaban Y, ET AL. Suicidal ideation is associated with altered variability of fingertip photo-plethysmogram signal in depressed patients. Front Physiol. 2017;8:501.
- Bernardi L, Wdowczyk-Szulc J, Valenti C, ET AL. Effects of controlled breathing, mental activity and mental stress with or without verbalization on heart rate variability. J Am Coll Cardiol. 2000;35:1462–1469.
- Geisler FCM, Kubiak T, Siewert K, ET AL. Cardiac vagal tone is associated with social engagement and self-regulation. Bio Psychol. 2013;93:279–286.
- Yasuma F, Hayano J. Respiratory sinus arrhythmia: why does the heartbeat synchronize with respiratory rhythm? Chest. 2004;125:683–690.
- Beauchaine TP. Respiratory sinus arrhythmia: a transdiagnostic biomarker of emotion dysregulation and psychopathology. Curr Opinion Psychol. 2015;3:43–47.
- McLaughlin KA, Rith-Najarian L, Dirks MA, ET AL. Low vagal tone magnifies the association between psychosocial stress exposure and internalizing psychopathology in adolescents. J Clin Child Adolesc Psychol. 2015;44:314–328.
- Servant D, Logier R, Mouster Y, ET AL. Heart rate variability. Applications in psychiatry. Encephale. 2009;35:423–428.
- Lackner HK, Papousek I, Batzel JJ, ET AL. Phase synchronization of hemodynamic variables and respiration during mental challenge. Int J Psychophysiol. 2011;79:401–409.
- Sheehan DV, Lecrubier Y, Sheehan KH, ET AL. The Mini-International Neuropsychiatric Interview (M.I.N.I.): the development and validation of a structured diagnostic psychiatric interview for DSM-IV and ICD-10. J Clin Psychiatry. 1998;59:22–33. quiz 34–57.
- Williams JB. A structured interview guide for the Hamilton Depression Rating Scale. Arch Gen Psychiatry. 1988;45:742–747.
- Khandoker AH, Luthra V, Abouallaban Y, ET AL. Predicting depressed patients with suicidal ideation from ECG recordings. Med Biol Eng Comput. 2017;55:793–805.
- Pan J, Tompkins WJ. A real-time QRS detection algorithm. IEEE Trans Biomed Eng. 1985;32:230–236.
- Khandoker AH, Jelinek HF, Moritani T, ET AL. Association of cardiac autonomic neuropathy with alteration of sympatho-vagal balance through heart rate variability analysis. Med Eng Phys. 2010;32:161–167.
- Oida E, Kannagi T, Moritani T, ET AL. Physiological significance of absolute heart rate variability in postural change. Acta Physiol Scand. 1999;165:421–422.
- Shannon CE. The mathematical theory of communication. MD Comput. 1997;14:306–317.
- Lerma C, Infante O, Perez-Grovas H, ET AL. Poincaré plot indexes of heart rate variability capture dynamic adaptations after haemodialysis in chronic renal failure patients. Clin Physiol Funct Imaging. 2003;23:72–80.
- Pagani M, Guzzetti S, Sandrone G, ET AL. Power spectral analysis as a clinical tool. In: Lown B, Malliani A, Prosdocimi M, editors. Neural mechanisms and cardiovascular disease. Vol. 5. Padova: Liviana Press; 1986. pp. 265–270.
- Heart rate variability: standards of measurement, physiological interpretation and clinical use. Task Force of the European Society of Cardiology and the North American Society of Pacing and Electrophysiology. Circulation. 1996;93:1043–1065.
- Agelink MW, Malessa R, Baumann B, ET AL. Standardized tests of heart rate variability: normal ranges obtained from 309 healthy humans, and effects of age, gender and heart rate. Clinical Autonomic Research. 2001;11:99–108.
- Pan LA, Hassel S, Segreti AM, ET AL. Differential patterns of activity and functional connectivity in emotion processing neural circuitry to angry and happy faces in adolescents with and without suicide attempt. Psychol Med. 2013;43:2129–2142.
- Bonelli RM, Cummings JL. Frontal-subcortical circuitry and behavior. Dialogues Clin Neurosci. 2007;9:141–151.
- Hugdahl K. Lateralization of cognitive processes in the brain. Acta Psychol (Amst). 2000;105:211–235.
- Kemp AH, Quintana DS, Felmingham KL, ET AL. Heart rate variability in unmedicated depressed patients without comorbid cardiovascular disease. PLoS ONE. 2012;7:e30777.
- Adolph D, Teismann T, Forkmann T, ET AL. High frequency heart rate variability: evidence for a transdiagnostic association with suicide ideation. Bio Psychol. 2018;138:165–171.
- Jelinek HF, Khandoker A, Quintana D, ET AL. Complex Correlation Measure as a sensitive indicator of risk for sudden cardiac death in patients with depression. Proceedings of the 2011 Computing in Cardiology; 2011 Sep 18–21; Hangzhou, China. Vol. 38. IEEE Press; 2011. p. 809–812.
- Aaronson ST, Sears P, Ruvuna F, ET AL. A 5-year observational study of patients with treatment-resistant depression treated with vagus nerve stimulation or treatment as usual: comparison of response, remission, and suicidality. Am J Psychiatry. 2017;174:640–648.
- McCraty R, Shaffer F. Heart rate variability: new perspectives on physiological mechanisms, assessment of self-regulatory capacity, and health risk. Glob Adv Health Med. 2015;4:46–61.
- McCraty R, Zayas MA. Cardiac coherence, self-regulation, autonomic stability, and psychosocial well-being. Front Psychol. 2014;5:1090.
- Keissar K, Davrath LR, Akselrod S. Coherence analysis between respiration and heart rate variability using continuous wavelet transform. Phil Trans Royal Soc Mathematical, Phys Eng Sci. 2009;367:1393–1406.
- Lehrer PM, Gevirtz R. Heart rate variability biofeedback: how and why does it work? Front Psychol. 2014;5:756.
- Wilson ST, Chesin M, Fertuck E, ET AL. Heart rate variability and suicidal behavior. Psychiatry Res. 2016;240:241–247.
- Salvadore G, Nugent AC, Lemaitre H, ET AL. Prefrontal cortical abnormalities in currently depressed versus currently remitted patients with major depressive disorder. NeuroImage. 2011;54:2643–2651.
- Vaccarino V, Lampert R, Bremner JD, ET AL. Depressive symptoms and heart rate variability: evidence for a shared genetic substrate in a study of twins. Psychosom Med. 2008;70:628–636.
- Carney RM, Freedland KE. Depression and heart rate variability in patients with coronary artery disease. Cleveland Clin J Med. 2009;76:S13–S17.