Abstract
Background: Although technology-enhanced simulation is increasingly used in health professions education, features of effective simulation-based instructional design remain uncertain.
Aims: Evaluate the effectiveness of instructional design features through a systematic review of studies comparing different simulation-based interventions.
Methods: We systematically searched MEDLINE, EMBASE, CINAHL, ERIC, PsycINFO, Scopus, key journals, and previous review bibliographies through May 2011. We included original research studies that compared one simulation intervention with another and involved health professions learners. Working in duplicate, we evaluated study quality and abstracted information on learners, outcomes, and instructional design features. We pooled results using random effects meta-analysis.
Results: From a pool of 10 903 articles we identified 289 eligible studies enrolling 18 971 trainees, including 208 randomized trials. Inconsistency was usually large (I 2 > 50%). For skills outcomes, pooled effect sizes (positive numbers favoring the instructional design feature) were 0.68 for range of difficulty (20 studies; p < 0.001), 0.68 for repetitive practice (7 studies; p = 0.06), 0.66 for distributed practice (6 studies; p = 0.03), 0.65 for interactivity (89 studies; p < 0.001), 0.62 for multiple learning strategies (70 studies; p < 0.001), 0.52 for individualized learning (59 studies; p < 0.001), 0.45 for mastery learning (3 studies; p = 0.57), 0.44 for feedback (80 studies; p < 0.001), 0.34 for longer time (23 studies; p = 0.005), 0.20 for clinical variation (16 studies; p = 0.24), and −0.22 for group training (8 studies; p = 0.09).
Conclusions: These results confirm quantitatively the effectiveness of several instructional design features in simulation-based education.
Introduction
Technology-enhanced simulation permits educators to create learner experiences that encourage learning in an environment that does not compromise patient safety. We define technology-enhanced simulation as an educational tool or device with which the learner physically interacts to mimic an aspect of clinical care for the purpose of teaching or assessment. Previous reviews have confirmed that technology-enhanced simulation, in comparison with no intervention, is associated with large positive effects (Cook et al. Citation2011; McGaghie et al. Citation2011). However, the relative merits of different simulation interventions remain unknown. Since the advantages of one simulator over another are context-specific (i.e. a given simulator may be more or less effective depending on the instructional objectives and educational context), it makes sense to focus on the instructional design features that define effective simulation training—the active ingredients or mechanisms. A comprehensive synthesis of evidence would be timely and useful to educators.
One systematic review identified 10 key features based on prevalence in the literature, but did not examine the impact of these features on educational outcomes (Issenberg et al., Citation2005). Other reviews have found an association between longer training time and improved outcomes (McGaghie et al. Citation2006) and that simulation with deliberate practice has consistently positive effects (McGaghie et al. Citation2011). In a review of simulation in comparison with no intervention (Cook et al. Citation2011), subgroup meta-analyses provided weak evidence suggesting better outcomes when learning activities were distributed over >1 day and when learners were required to demonstrate mastery of the task. When comparing simulation with non-simulation instruction (Cook et al. Citation2012), subgroup meta-analyses suggested better outcomes when extraneous cognitive load was low, when learners worked in groups, and when feedback and learning time were greater.
However, such subgroup analyses represent an inefficient method of exploring the effectiveness of design features because they evaluate differences between studies, and between-study variation in learners, contexts, clinical topics, and outcome measures introduces error and confounds interpretations. Most of the subgroup interactions evaluated in these reviews (Cook et al. 2011, 2012) varied from outcome to outcome and most were statistically non-significant. The direct comparison of two instructional variations in a single study offers a less problematic approach, as it capitalizes on within-study (rather than between-study) design differences. For example, meta-analysis of head-to-head comparisons has been used to identify effective instructional design features in Internet-based instruction. (Cook et al. Citation2010b)
A comprehensive review of head-to-head comparisons of different simulation-based instructional interventions (i.e. comparative effectiveness studies) would fulfill two important needs in health professions education. First, a quantitative synthesis of evidence regarding specific instructional design features would immediately inform educational practice. Second, a thematic summary of the comparisons made and research questions addressed would inform future research by providing a list of common comparisons (indicating themes felt to be important and likely worthy of further study) and by highlighting evidence gaps. We sought to address both of these needs through a systematic review.
Methods
This review was planned, conducted, and reported in adherence to PRISMA standards of quality for reporting meta-analyses (Moher et al. Citation2009).
Questions
We sought to answer: what instructional design features are associated with improved outcomes in studies directly comparing one technology-enhanced simulation training approach with another, and what themes have been addressed in such comparisons? To answer the first question we selected eight instructional design features identified in the review by Issenberg et al. (Citation2005) and additional features of cognitive interactivity, distributing training across multiple sessions, group vs independent practice, and time spent learning (see for definitions). We hypothesized that outcomes would be higher with more of each feature.
Box 1 Definitions of terms*
Study eligibility
We included studies published in any language that investigated use of technology-enhanced simulation to teach health professions learners at any stage in training or practice, in comparison with another technology-enhanced simulation design or a variation or augmentation of the first, using outcomes (Kirkpatrick Citation1996) of reaction (satisfaction), learning (knowledge or skills in a test setting), behaviors (in practice), or effects on patients (see ). Technology-enhanced simulation encompasses diverse products including computer-based virtual reality simulators, high fidelity and static mannequins, plastic models, live animals, inert animal products, and human cadavers. Because they have been the subject of recent reviews, we excluded studies in which the only simulation activities involved computer-based virtual patients (Cook & Triola Citation2009; Cook et al. Citation2010a) and human patient actors (standardized patients) (Bokken et al. Citation2008; May et al. Citation2009).
Study identification
We searched MEDLINE, EMBASE, CINAHL, PsycINFO, ERIC, Web of Science, and Scopus using a search strategy developed by an experienced research librarian (PJE). The search included terms for the intervention (including simulator, simulation, manikin, cadaver, MIST, Harvey, and many others), topic (surgery, endoscopy, anesthesia, trauma, colonoscopy, etc.), and learners (education medical, education nursing, education professional, internship and residency, etc.). We used no beginning date cutoff, and the last date of search was May 11, 2011. In addition, we added all articles published in two journals devoted to health professions simulation (Simulation in Healthcare and Clinical Simulation in Nursing) since their inception, and the entire reference list from several published reviews of health professions simulation. Finally, we searched for additional studies in the reference lists of all included articles published before 1990 and a random sample of 160 included articles published in or after 1990. Our complete search strategy has been published previously (Cook et al. Citation2011).
Study selection
Working independently and in duplicate, we screened all titles and abstracts for inclusion. In the event of disagreement or insufficient information in the abstract we reviewed the full text of potential articles, again independently and in duplicate, resolving conflicts by consensus. Chance-adjusted interrater agreement for study inclusion, determined using intraclass correlation coefficient (ICC), was 0.69. Non-English articles were translated in full.
Data extraction
Using a data abstraction form we abstracted data independently and in duplicate for all variables where reviewer judgment was required, resolving conflicts by consensus. Interrater agreement was fair (ICC 0.2–0.4) or moderate (0.4–0.6) for most variables (Landis & Koch Citation1977). We identified the main theme of each comparison (research question, study hypothesis) using an inductive, iterative approach. We abstracted information on the training level of learners, clinical topic, training location (simulation center or clinical environment), study design, method of group assignment, outcomes, and methodological quality. We planned to abstract information on simulation fidelity but dropped this variable due to difficulty operationalizing it with acceptable reliability. We coded simulation features (see ) of:
clinical variation (present/absent; ICC, 0.46),
cognitive interactivity (high/low; ICC, 0.35),
curriculum integration (present/absent; ICC, 0.49),
distributed practice (training on 1 or >1 day; ICC, 0.73),
feedback (high/low; ICC, 0.46),
group vs independent practice (ICC, 0.71),
individualized learning (present/absent; ICC, 0.25, with raw agreement 85%),
mastery learning (Issenberg's “defined outcomes,” i.e. training to a predefined level of proficiency, present/absent; ICC, 0.53),
multiple learning strategies (high/low; ICC, 0.49),
range of task difficulty (present/absent; ICC, 0.30, with raw agreement 82%),
repetitive practice (number of repetitions; ICC, 0.60), and
time spent learning (ICC, 0.72).
Table 1. Description of included studies
Methodological quality was graded using the Medical Education Research Study Quality Instrument (Reed et al. Citation2007) and an adaptation of the Newcastle-Ottawa scale for cohort studies (Wells et al. 2007; Cook et al. Citation2008b) that evaluates representativeness of the intervention group (ICC, 0.68), selection of the comparison group (ICC, 0.26 with raw agreement 86%), comparability of cohorts (statistical adjustment for baseline characteristics in nonrandomized studies [ICC, 0.88], or randomization [ICC, 0.84] and allocation concealment for randomized studies [ICC, 0.63]), blinding of outcome assessment (ICC, 0.58), and completeness of follow-up (ICC, 0.36 with raw agreement 80%).
Since the results associated with simulation training may vary for different outcomes, we distinguished outcomes using Kirkpatrick's classification (Kirkpatrick Citation1996) and abstracted information separately for satisfaction, learning (knowledge and skills, with skills further classified as time to complete the task, process, and product [see for definitions]), behaviors with patients (time and process), and results (patient effects). Authors frequently reported multiple measures of a single outcome (e.g. multiple measures of process skill), in which case we selected, in order of priority, (1) the author-defined primary outcome, (2) a global or summary measure of effect, (3) the most clinically relevant measure, or (4) the average of the measures reported. We also prioritized skill outcomes assessed in a different setting (e.g. different simulator or clinical setting) over those assessed in the simulator used for training.
Data synthesis
For each reported outcome we calculated the standardized mean difference (Hedges’ g effect size) between each group using standard techniques (Borenstein Citation2009; Morris & DeShon Citation2002; Curtin et al. Citation2002; Hunter & Schmidt Citation2004) as we have detailed previously. (Cook et al. Citation2011) For studies reporting neither p values nor any measure of variance, we used the average standard deviation from all other studies reporting that outcome. If we could not calculate an effect size using reported data we requested additional information from authors via e-mail.
We used the I2 statistic (Higgins et al. Citation2003) to quantify inconsistency (heterogeneity) across studies. I2 estimates the percentage of variability across studies not due to chance, and values >50% indicate large inconsistency. Large inconsistency weakens the inferences that can be drawn, but does not preclude the pooling of studies sharing a common conceptual link.
We planned meta-analyses to evaluate the effectiveness of each instructional design feature, pooling the results of all studies for which that feature varied between two simulation-based interventions. For example, if one study group received high feedback and the other low feedback, this study would be included in the “Feedback” meta-analysis. If feedback were equal in both arms, it would be excluded from that analysis. To increase the power of these analyses, we merged process and product skills into a single outcome of “non-time skills,” and we also combined behaviors and patient effects. Because we found large inconsistency in most analyses, we used random effects models to pool weighted effect sizes. Many studies appear in >1 analysis (i.e. both feedback and repetitive practice) but no study appeared more than once per analysis. For studies with >2 groups (for example, three different simulation instructional designs), we selected for the main analysis the designs with the greatest between-group difference, and then performed sensitivity analyses substituting the other design(s). We also performed sensitivity analyses excluding low-quality studies (those with NOS and MERSQI scores below the median) and studies with imprecise effect size estimation (p value upper limits or imputed standard deviations).
We used SAS 9.2 (SAS Institute, Cary, NC) for all analyses. Statistical significance was defined by a two-sided alpha of 0.05. Determinations of educational significance emphasized Cohen's effect size classifications (<0.2 = negligible; 0.2–0.49 = small; 0.5–0.8 = moderate) (Cohen, Citation1988).
Results
Trial flow
We identified 10 297 articles using our search strategy and 606 from our review of reference lists and journal indices. From these we identified 295 studies comparing two or more simulation training interventions () of which 290 reported eligible outcomes. Two articles reported the same data and we selected the most detailed one for inclusion. We obtained additional outcomes data for 1 study from study authors. Ultimately, we included 289 studies enrolling 18 971 trainees. Twenty-six of these 289 were multi-arm studies that included a comparison with no-intervention, and the no-intervention results were reported previously (Cook et al. Citation2011). summarizes key study characteristics and Appendix 1 provides a complete listing of articles with additional information.
Study characteristics
Studies in our sample used technology-enhanced simulations to teach topics such as minimally invasive surgery, dentistry, intubation, physical examination, and teamwork. Nearly half the articles (N = 139) were published in or after 2008, and five were published in a language other than English. Learners included student and practicing physicians, nurses, emergency medicine technicians, dentists, chiropractors, and veterinarians, among others. summarizes the prevalence of instructional design features such as feedback (75 studies), repetitive practice (233 studies), and distributed practice (98 studies). Most studies reported learner skills, including 100 time, 197 process, and 56 product skill outcomes. Fifty-six studies reported satisfaction, 34 reported knowledge outcomes, 1 reported time behavior, 9 reported process behavior, and 8 reported patient effects.
Study quality
summarizes the methodological quality of included studies. The number of participants providing outcomes ranged from 4 to 817 with a median of 30 (interquartile range 20–53). Groups were randomly assigned in 208 studies (72%). Studies lost more than 25% of participants from time of enrollment or failed to report follow-up for 13 of 56 satisfaction outcomes (23%), 5 of 34 knowledge (15%), 31 of 100 time skill (31%), 62 of 197 process skill (31%), 18 of 56 product skill (32%), and 1 of 9 process behavior (11%) (time behavior and patient effect outcomes had complete follow-up). Assessors were blinded to group assignment for 309 of 461 outcome measures (67%). Most outcomes reflect objective measures (e.g. computer scoring, objective key, or human rater). All knowledge and time behavior outcomes were determined objectively, while trainee self-assessments comprised five process skill outcomes and one each of time skill, product skill, process behavior, and patient effect outcomes. The mean (SD) quality scores averaged 3.5 (1.3) for the Newcastle-Ottawa Scale (6 points indicating highest quality) and 12.3 (1.8) for the Medical Education Research Study Quality Instrument (maximum 18 points).
Table 2. Quality of included studies
Table 3. Research themes (comparisons) addressed by studies
Meta-analysis
For meta-analysis we merged process and product skills into a single outcome of “non-time skills,” and we likewise merged behaviors and patient effects. shows the pooled effect size for each instructional design feature, organized by outcome (panels A–E). For non-time skills we confirmed small to moderate positive effects favoring the presence of each proposed feature of effective simulation except group training, and most (7 of 11) effects were statistically significant. Results for other outcomes nearly always (35 of 38) favored the proposed feature, but results were usually not statistically significant.
Figure 2. Random effects meta-analysis Comparisons of simulation interventions varying in the specified key feature; positive numbers favor the intervention with more of that feature. If a feature is not present in the analysis for a given outcome, it is because no studies reporting that outcome had interventions that varied for that particular feature. Some feature comparisons had only 1 relevant study; these are included in the figures but we note that the effect size reflects only that 1 study (ie, not pooled).
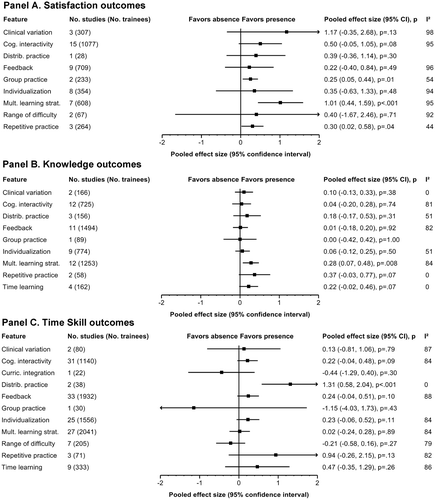
For example, for the non-time skill outcomes (, panel D), 20 studies reported a comparison in which one simulation design included tasks reflecting a range of difficulty and the other did not. Among these studies, designs offering a range of difficulty were associated with better outcomes than those of uniform difficulty, with pooled effect size (ES) 0.68 (95% confidence interval [CI], 0.30–1.06, p < 0.001). This difference is statistically significant, and moderate in magnitude using Cohen's classification. Further differences of small to moderate magnitude were found for instructional designs incorporating clinical variation (0.20), more interactivity (0.65), training over >1 day (0.66), more feedback (0.44), individualization (0.52), mastery learning (0.45), more learning strategies (0.62), repetition (ES 0.68), and longer time (0.34). Findings for knowledge, time, and behavior-patient effect outcomes were similarly favorable, but with smaller and usually statistically non-significant effects (see ). Inconsistency was large (I2 > 50%) in most analyses.
The exception to the predicted pattern was group training, which showed a small negative association with non-time skills (ES −0.22 [95% CI, −0.48 to 0.03], p = 0.09). Knowledge and time outcomes (one study each) showed similar results.
Several studies reporting non-time skills and behavior-patient effects had three simulation arms. Since we could only compare two groups at once, we first included the groups with the greatest between-design difference and then performed sensitivity analyses substituting the third group (see Appendix 2). Results changed almost imperceptibly for non-time skills: pooled effect sizes varied by <0.08 in all analyses, and statistical significance changed in only one instance (the group practice analysis was now statistically significant, p = 0.02). For behavior–patient effects the pooled ES for feedback dropped to 0.18.
Additional sensitivity analyses excluded low-quality studies. The direction of effect reversed only rarely (5 of 153 analyses), namely: mastery learning (non-time skill outcomes) when excluding imprecise effect size estimation or low NOS score (N = 4 studies remaining for each analysis); feedback (satisfaction outcomes) when excluding low MERSQI or NOS score (N = 2 for each); and interactivity (behavior–patient effects) when excluding low MERSQI scores (N = 2).
Research themes
Through an iterative process we identified six main research themes, with a much larger number of sub-themes (). Thirty-eight studies had two or three arms each, resulting in a total of 337 comparisons. The most prevalent main theme involved comparisons of different instructional design features such as the amount or method of feedback, the sequence of training activities, task variability, or repetition. Second most common were studies that compared two technology-enhanced simulation modalities, such as mannequin vs part-task model, mannequin vs virtual reality, or two different mannequins. Several studies evaluated the addition of another instructional modality (e.g. a lecture, computer-assisted instruction, or another simulation modality) to the standard simulation training. The remaining themes focused on the role of the instructor, sensory augmentation including haptics, and group composition. We initially identified a main theme of “fidelity,” but upon further reflection realized that all of the studies thus classified could be more appropriately classified using another theme, most often that of “modality comparison.”
Discussion
In 2005, Issenberg et al. proposed 10 features of effective simulation based on prevalence in the literature. Our synthesis of research provides empiric support for nearly all of these features and several others. Although the pooled effect sizes were often small and not statistically significant, and between-study inconsistency was high, the consistent direction of effect across outcomes suggests that the benefits are real. To put these findings in perspective, the effect size of 0.68 observed for “range of difficulty” using non-time skill outcomes would translate to a 5% improvement (out of 100%) on a typical skill assessment. Effect sizes were generally larger for skills than for knowledge, a tendency we also observed among studies comparing simulation with non-simulation instruction (Cook et al. Citation2012). Of the twelve features evaluated, only group instruction failed to demonstrate consistently positive effects. Interestingly, in the previous meta-analysis (Cook et al. Citation2012) of studies comparing simulation with non-simulation instruction, group instruction was associated with improved outcomes; this incongruity merits further study.
We also classified the research themes for 337 simulation–simulation comparisons. The most prevalent theme involved evaluating key features of instructional design. These studies, along with those exploring instructor roles and group composition, typically allowed generalizable conclusions. By contrast, another one-third of the themes focused on comparing different simulation modalities. While modality comparisons initially appear useful, we noted that the results varied widely as technologies changed and evolved, the educational context varied, and different implementations of the same technology employed different instructional designs. As a result, we suspect the findings from modality comparisons will have limited generalizability.
One design feature from Issenberg et al.'s review that we did not code was fidelity. We found fidelity difficult to code, during both the quantitative data abstraction and the thematic analysis. We found that “fidelity” encompasses a number of different facets related to the simulation activity, including the characteristics of the simulator that mediate sensory impressions (visual, auditory, olfactory, and tactile/haptic), the nature of the learning objectives and task demands, the environment, and other factors that might affect learner engagement and suspension of disbelief. Labeling a simulation as “high fidelity” conveys such diverse potential meanings that the term loses nearly all usefulness. Based on our experiences during this review, we suggest that researchers and educators employ more specific terminology when discussing the physical and contextual attributes of simulation training.
Limitations and strengths
In order to present a comprehensive thematic overview of the field and achieve adequate statistical power for meta-analyses, we used intentionally broad inclusion criteria. However, in so doing we included studies reflecting diverse training topics, instructional designs, and outcome measures. These differences likely contributed to the large between-study inconsistency. This inconsistency tempers our inferences, but does not preclude meta-analytic pooling (Montori et al. Citation2003; Cook Citation2012b). Future original research and research syntheses might further clarify the importance of these instructional design features for specific topics, such as technical and non-technical tasks.
Literature reviews are necessarily constrained by the quantity and quality of available evidence. Among the included studies, sample sizes were relatively small, sample representativeness was rarely addressed, outcome validity evidence was infrequently presented, and many reports failed to clearly describe key features of the context, instructional design, or outcomes. Although we found numerous studies reporting skill outcomes and several reporting satisfaction and knowledge, we found few studies reporting higher-order outcomes of behavior and patient effects. However, more than 70% of the studies used randomization, and MERSQI scores were substantially higher than those found in previous reviews of medical education research (Reed et al. Citation2007, Citation2008).
Coding reproducibility was suboptimal for some instructional design features, likely due to both poor reporting and difficulty operationalizing coding criteria. However, we reached consensus on all codes prior to meta-analysis.
To increase statistical power and to reduce the number of independent meta-analyses, we combined process and product outcomes for assessments in both an education setting (skills) and with real patients (behaviors and patient effects). Analyzing these separately might have led to slightly different conclusions.
Our review has several additional strengths, including an extensive literature search led by a skilled librarian; no restriction based on time or language of publication; explicit inclusion criteria encompassing a broad range of learners, outcomes, and study designs; duplicate, independent, and reproducible data abstraction; rigorous coding of methodological quality; and hypothesis-driven analyses.
Comparison with previous reviews
The present review complements our recent meta-analysis showing that simulation training is associated with large positive effects in comparison with no intervention (Cook et al. Citation2011). Having established that simulation can be effective, the next step is to understand what makes it effective. Although several other reviews have addressed simulation in general (Issenberg et al. Citation2005; McGaghie et al. Citation2010) or in comparison with no intervention, (Gurusamy et al. Citation2008; McGaghie et al. Citation2011), we are not aware of previous reviews focused on comparisons of different technology-enhanced simulation interventions or instructional designs. By confirming the effectiveness of the design features proposed by Issenberg et al. (Citation2005), our comprehensive and quantitative synthesis represents a novel and important contribution to the field.
Our findings of small to moderate effects favoring theory-predicted instructional design features parallel the findings of a review of Internet-based instruction (Cook et al. Citation2008b). An association between longer time in practice and improved outcomes was also reported in a previous review of simulation-based education (McGaghie et al. Citation2006).
Implications
The features proposed by Issenberg et al. (Citation2005) as central to effective simulation appear to work, as do the additional features we identified. We recommend that these be considered the current “best practices” for the field. In order of pooled effect size, these are: range of difficulty, repetitive practice, distributed practice, cognitive interactivity, multiple learning strategies, individualized learning, mastery learning, feedback, longer time, and clinical variation.
However, we simultaneously highlight the need for further research elucidating what works, for whom, under what circumstances. The large inconsistency observed in nearly all analyses indicates that the effect varies from study to study, and the relative contribution of multiple potentially influential variables (learners, environment, operational definition of interventions, outcomes, and other study methods) remains unclear. Besides meta-analysis, other synthesis methods such as realist review (Pawson et al. Citation2005) will help interpret existing evidence.
Going forward, we believe that a fundamental change in the conception and design of new research is required. To-date, the number of studies attempting to clarify the use of simulation by directly comparing different simulation-based interventions is small (N = 289) relative to the number of studies comparing simulation with no intervention or non-simulation instruction (N = 690 [see ]) and studies without any comparison (N = 864). Studies comparing simulation with simulation will do far more to advance the field than comparisons of simulation with non-simulation approaches. (Cook Citation2010) Yet not all simulation–simulation comparisons are equally useful, and studies evaluating modalities or instructional designs without a conceptual or theoretical rationale have limited generalizability.
The field thus needs research that goes beyond simple comparisons of presence/absence of key features (Weinger, Citation2010). For example, it appears that feedback improves outcomes—but we expect that much could yet be learned about the basis, timing, and delivery of feedback. This research will require progressively refined theories and conceptual frameworks that programmatically study carefully constructed questions (Bordage Citation2009; McGaghie et al. Citation2010). The themes identified in this review (see ) provide a starting point for such research programs. It will also be important to systematically account the costs of alternate instructional approaches (Levin Citation2001), and explore how costs can inform design decisions (Zendejas 2012).
Table A1. List of all included studies
Of course, such research will not be possible without adequate funding. Health professions education research is underfunded (Reed et al. Citation2005), even though funding is associated with higher quality work (Reed et al. Citation2007). Those responsible for funding decisions must recognize the importance of theory-building research that clarifies (Cook et al. Citation2008a) the modalities and features of simulation-based education that improve learner and patient outcomes with greatest effectiveness and at lowest cost.
Finally, we note that the effect sizes for these comparisons are much lower than those observed for comparisons with no intervention. This is not unexpected, as comparing training vs no training ought to result in greater improvement than comparing two active instructional interventions (Cook Citation2012a). However, we caution investigators that the small samples that proved sufficient to identify statistically significant differences in no-intervention-comparison studies will be inadequate for simulation–simulation research. Advance calculation of sample size, clear justification of educational significance, and use of confidence intervals when interpreting results will be essential. These, together with other research methods that minimize confounding, will facilitate studies that truly advance our understanding of how to improve healthcare through simulation-based education.
Practice points
Evidence supports the following as best practices for simulation-based education: range of difficulty, repetitive practice, distributed practice, cognitive interactivity, multiple learning strategies, individualized learning, mastery learning, feedback, longer time, and clinical variation.
Future research should clarify the mechanisms of effective simulation-based education: what works, for whom, in what contexts?
Direct comparisons of alternate simulation-based education instructional designs can clarify these mechanisms.
Acknowledgements
Portions of this work were presented at the 2012 International Meeting on Simulation in Healthcare, in San Diego, California.
Declaration of interest: This work was supported by intramural funds, including an award from the Division of General Internal Medicine, Mayo Clinic. The authors report no conflicts of interest. The authors alone are responsible for the content and writing of the article.
References
- Bokken L, Rethans JJ, Scherpbier AJ, Van Der Vleuten CP. Strengths and weaknesses of simulated and real patients in the teaching of skills to medical students: A review. Simul Healthc 2008; 3: 161–169
- Bordage G. Conceptual frameworks to illuminate and magnify. Med Educ 2009; 43: 312–319
- Borenstein M. Effect sizes for continuous data. The Handbook of Research Synthesis2nd, H Cooper, LV Hedges, JC Valentine. Russell Sage Foundation, New York 2009; 221–235
- Cohen J. Statistical Power Analysis for the Behavioral Sciences. Lawrence Erlbaum, Hillsdale, NJ 1988
- Cook DA, 2012a. If you teach them, they will learn: Why medical education needs comparative effectiveness research, Advances in Health Sciences Education, 17:305–310
- Cook DA, 2012b. Randomized controlled trials and meta-analysis in medical education: What role do they play?, Medical Teacher, In press
- Cook DA. One drop at a time: Research to advance the science of simulation. Simul Healthc 2010c; 5: 1–4
- Cook DA, Bordage G, Schmidt HG. Description, justification, and clarification: A framework for classifying the purposes of research in medical education. Medl Educ 2008a; 42: 128–133
- Cook DA, Brydges R, Hamstra S, Zendejas B, Szostek JH, Wang AT, Erwin P, Hatala R. Comparative effectiveness of technology-enhanced simulation vs other instructional methods: A systematic review and meta-analysis. Simul Healthc 2012, In press
- Cook DA, Erwin PJ, Triola MM. Computerized virtual patients in health professions education: A systematic review and meta-analysis. Acad Med 2010a; 85: 1589–1602
- Cook DA, Hatala R, Brydges R, Zendejas B, Szostek JH, Wang AT, Erwin P, Hamstra S. Technology-Enhanced simulation for health professions education: A systematic review and meta-analysis. J Am Med Assoc 2011; 306: 978–988
- Cook DA, Levinson AJ, Garside S, Dupras DM, Erwin PJ, Montori VM. Internet-Based Learning in the Health Professions: A Meta-Analysis. JAMA 2008b; 300: 1181–1196
- Cook DA, Levinson AJ, Garside S, Dupras DM, Erwin PJ, Montori VM. instructional design variations in internet-based learning for health professions education: A systematic review and meta-analysis. Acad Med 2010b; 85: 909–922
- Cook DA, Triola MM. Virtual patients: A critical literature review and proposed next steps. Medical Education 2009; 43: 303–311
- Curtin F, Altman DG, Elbourne D. Meta-analysis combining parallel and cross-over clinical trials. I: Continuous outcomes. Stat Med 2002; 21: 2131–2144
- Gurusamy K, Aggarwal R, Palanivelu L, Davidson BR. Systematic review of randomized controlled trials on the effectiveness of virtual reality training for laparoscopic surgery. Br J Surg 2008; 95: 1088–1097
- Higgins JPT, Thompson SG, Deeks JJ, Altman DG. Measuring inconsistency in meta-analyses. Br Med J 2003; 327: 557–560
- Hunter JE, Schmidt FL. Methods of Meta-Analysis: Correcting Error and Bias in Research Findings. Sage, Thousand Oaks, CA 2004
- Issenberg SB, Mcgaghie WC, Petrusa ER, Lee Gordon D, Scalese RJ. Features and uses of high-fidelity medical simulations that lead to effective learning: A BEME systematic review. Med Teac 2005; 27: 10–28
- Kirkpatrick D. Revisiting Kirkpatrick's four-level model. Training Dev 1996; 50(1)54–59
- Landis JR, Koch GG. The measurement of observer agreement for categorical data. Biometrics 1977; 33: 159–174
- Levin HM. Waiting for Godot: Cost-effectiveness analysis in education. New Directions for Evaluation 2001; 90: 55–68
- May W, Park JH, Lee JP. A ten-year review of the literature on the use of standardized patients in teaching and learning: 1996–2005. Med Teach 2009; 31: 487–492
- Mcgaghie WC, Issenberg SB, Cohen ER, Barsuk JH, Wayne DB. Does simulation-based medical education with deliberate practice yield better results than traditional clinical education? A meta-analytic comparative review of the evidence. Acad Med 2011; 86: 706–711
- Mcgaghie WC, Issenberg SB, Petrusa ER, Scalese RJ. Effect of practice on standardised learning outcomes in simulation-based medical education. Med Educ 2006; 40: 792–797
- Mcgaghie WC, Issenberg SB, Petrusa ER, Scalese RJ. A critical review of simulation-based medical education research: 2003–2009. Med Educ 2010; 44: 50–63
- Moher D, Liberati A, Tetzlaff J, Altman DG. Preferred reporting items for systematic reviews and meta-analyses: The PRISMA statement. Ann Intern Med 2009; 151: 264–269
- Montori VM, Swiontkowski MF, Cook DJ. Methodologic issues in systematic reviews and meta-analyses. Clin Orthop Relat Res 2003; 413: 43–54
- Morris SB, Deshon RP. Combining effect size estimates in meta-analysis with repeated measures and independent-groups designs. Psychol Meth 2002; 7: 105–125
- Pawson R, Greenhalgh T, Harvey G, Walshe K. Realist review – a new method of systematic review designed for complex policy interventions. J Health Serv Res Policy 2005; 10(Suppl 1)21–34
- Reed DA, Beckman TJ, Wright SM, Levine RB, Kern DE, Cook DA. Predictive validity evidence for medical education research study quality instrument scores: Quality of submissions to JGIM's Medical Education Special Issue. J Gen Intern Med 2008; 23: 903–907
- Reed DA, Cook DA, Beckman TJ, Levine RB, Kern DE, Wright SM. Association between funding and quality of published medical education research. J Am Med Assoc 2007; 298: 1002–1009
- Reed DA, Kern DE, Levine RB, Wright SM. Costs and funding for published medical education research. J Am Med Assoc 2005; 294: 1052–1057
- Weinger MB. The pharmacology of simulation: A conceptual framework to inform progress in simulation research. Simul Healthc 2010; 5: 8–15
- Wells GA, Shea B, O’connell D, Peterson J, Welch V, Losos M, Tugwell P, The Newcastle-Ottawa Scale (NOS) for assessing the quality of nonrandomised studies in meta-analyses. [Accessed 29 February, 2012] Available from http://www.ohri.ca/programs/clinical_epidemiology/oxford.htm
- Zendejas B, Wang AT, Brydges R, Hamstra SJ, Cook DA, 2012. Cost: The missing outcome in simulation-based medical education research: A systematic review. Surgery; Online early doi 10.1016/j.surg.2012.06.025
Appendix
Table A2. Results of sensitivity analyses