Abstract
Daily treatment plan selection from a plan library is a major adaptive radiotherapy strategy to account for individual internal anatomy variations. This strategy depends on the initial input images being representative for the variations observed later in the treatment course. Focusing on locally advanced prostate cancer, our aim was to evaluate if residual motion of the prostate (CTV-p) and the elective targets (CTV-sv, CTV-ln) can be prospectively accounted for with a statistical deformable model based on images acquired in the initial part of treatment. Methods. Thirteen patients with locally advanced prostate cancer, each with 9–10 repeat CT scans, were included. Displacement vectors fields (DVF) obtained from contour-based deformable registration of delineations in the repeat- and planning CT scans were used to create patient-specific statistical motion models using principal component analysis (PCA). For each patient and CTV, four PCA-models were created: one with all 9–10 DVF as input in addition to models with only four, five or six DVFs as input. Simulations of target shapes from each PCA-model were used to calculate iso-coverage levels, which were converted to contours. The levels were analyzed for sensitivity and precision. Results. A union of the simulated shapes was able to cover at least 97%, 97% and 95% of the volumes of the evaluated CTV shapes for PCA-models using six, five and four DVFs as input, respectively. There was a decrease in sensitivity with higher iso-coverage levels, with a sharper decline for greater target movements. Apart from having the steepest decline in sensitivity, CTV-sv also displayed the greatest influence on the number of geometries used in the PCA-model. Conclusions. PCA-based simulations of residual motion derived from four to six DVFs as input could account for the majority of the target shapes present during the latter part of the treatment. CTV-sv displayed the greatest range in both sensitivity and precision.
Independent target motion is a challenge in radiotherapy (RT) when multiple targets are treated. For locally advanced prostate cancer residual motion after positioning based on the primary target (the prostate) stems from independent motion of the pelvic lymph nodes as well as residual motion caused by both independent motion and deformations of the seminal vesicles [Citation1–7]. We and others have shown large individual variations for margins required to cover this residual motion, in particular for the seminal vesicles [Citation1,Citation2,Citation4,Citation8]. For intra-prostatic fiducial setup, the suggested margin expansions for the seminal vesicles have ranged from 7 to 16 mm [Citation4,Citation8–11], while for the pelvic lymph nodes the suggested margins have been smaller, in the range 5–10 mm [Citation8–10]. These large expansions might be challenging in RT due the implied risk of toxicity [Citation12].
Adaptive RT refers to the concept where the treatment is altered to the variations observed for the individual patient [Citation13]. In RT of bladder cancer, adaptively selecting treatment plans according to the daily size and shape of the bladder target volume has presented encouraging results [Citation14]. In gynecological RT, modeling the deformable target of the uterus based on a bladder volume model has enabled a reduction of the treated volume [Citation15]. However, in RT of prostate cancer, the motion of the involved targets are induced both by changes in bladder as well as in the rectum [Citation13–18]. The modeling of target motion in locally advanced prostate cancer involving multiple targets is further complicated by the different motion patterns, e.g. seminal vesicles presenting large deformations while the prostate and the pelvic lymph nodes are assumed move more rigidly [Citation1–3,Citation7,Citation11]. For the purpose of calculating these motion patterns, we previously established a statistical deformable model of residual target motion [Citation8] based on principal component analysis (PCA) [Citation19,Citation20]. In the present study we will explore the PCA-model further for adaptation to patient-specific target volumes based on residual motion following image-guidance on intra-prostatic fiducial makers. In this setting, it is of importance to evaluate whether such motion patterns can be predicted and if the model can be used to prospectively account for subsequent variations in target shape. The current study therefore had the aim of individualizing target volumes and evaluating if the residual motion patterns for the primary target (CTV-p) and the two elective targets (CTV-sv and CTV-ln) could be modeled with a limited number of deformation vector fields (DVFs) as input.
Material and methods
Patient material
Thirteen patients previously treated with intensity-modulated RT (IMRT) for locally advanced prostate cancer were included in the study [Citation21]. Each patient had an image set consisting of a planning CT in addition to repeat CTs (9–10) evenly acquired during the treatment course. Details of slice thickness, patient positioning and definitions of each clinical target volume (CTV) have been previously presented [Citation8,Citation22]. In short, for each CT scan the three CTVs – CTV of prostate (CTV-p), CTV of seminal vesicles (CTV-sv) and CTV of the pelvic lymph nodes (CTV-ln) – were delineated by an experienced radiation oncologist [Citation22]. The CTV-ln delineations followed the RTOG guidelines [Citation6] except for the pre-sacral nodes which were omitted.
Generation of individualized statistical motion models
This study focused on the residual geometric uncertainties present after setup based on the intra- prostatic fiducial markers. Therefore, all residual motion, rigid as well as non-rigid, was derived relative to that of the intra-prostatic fiducial markers. In a previous study we developed a statistical deformable model of residual target motion based on PCA; all details of the model have been presented elsewhere [Citation8] but for completeness the most important parts are presented in the following.
Setup based on intra-prostatic fiducials was simulated through a rigid co-registration (translations only) on the markers in the repeat CTs to the markers in the planning CT. Hence, each target surface is defined in a common reference system defined by the fiducial markers in the planning CT. The target shape vector p consisted of M points (M = [1500;229 700]) distributed with an isotropic resolution of 1 mm on the target surface. Point correspondence of the target shapes in the planning CT and the target shapes in the repeat CT scans were calculated using an inverse-consistent version of the Thin-Plate Spline Robust-Point Matching (TPS-RPM) algorithm for non-rigid registrations [Citation23,Citation24]. From each deformable registration a DVF of all target points was obtained. Subsequently, the average target shape vector, and the covariance matrix, C were calculated according to:
for all, N target shape vectors, pi. In Equation 2, (…)T denotes the transposed vector and (…)×(…)T denotes the outer product.
Following the method introduced by Söhn et al. [Citation19,Citation20] these two moments of the shape vectors served as input to the PCA:
where the eigenvectors ql are normalized orthogonal vectors. In PCA these eigenvectors are ordered by the largest eigenvalues λl quantifying the variability that is accounted for by each component, l = 1...L,. The linear combinations of the components in Equation 3 describe the superposition of the eigenmodes. Since, in PCA the weight, cl is assumed to be normally distributed with an average of zero and a variance defined by λl, new displacement vectors can be constructed by sampling of cl [Citation19,Citation25]. In our simulations new displacement vectors were sampled using Equation 3 and considering all eigenmodes. Furthermore, a separate PCA-model was constructed for each target in every patient.
Evaluation of individualized statistical motion models
Four different individual PCA-models were evaluated, one with the DVFs from registration of all repeat scans as input, a second based on four input DVFs, a third based on five DVFs and a fourth using six. From each PCA-model, simulation of between 5000 and 10 000 shapes for each target were used to generate coverage probability maps. Fourteen iso-coverage levels varying from the union (denoted 0) of all the samples to the intersection (denoted 1) were evaluated. For each level the sensitivity and the precision was calculated as:
where the true-positive fraction was denoted TP, the false-negative fraction was denoted FN and the false-positive fraction denoted FP. Here, TP was the intersection of the volume enclosed by each iso-coverage level and the manually delineated structure volumes used for evaluation. FN was volume not considered target by the iso-coverage levels but enclosed by the manually delineated structures in contrast to FP, which was considered target by the iso-coverage levels but not enclosed by the manually delineated structures. For the PCA-model based on all geometries as input the same shapes were used for both input and evaluation. For the PCA-models based on a reduced number of geometries as input the remaining (3–4) delineated geometries not included in the model were used for evaluation of all models. In addition, to assess the influence of iso-coverage levels on the evaluation parameters for patient experiencing small versus large target motion we divided the patients into two groups based on the relative volume of the union of simulated shapes from the PCA-model with all geometries as compared to the volume of the planning CTV.
Results
Eigenvalue spectra
The eigenvalue spectra for each target with the all DVF models and separated for small and large movers, are displayed in . To account for at least 90% of the variance in CTV-ln five modes were necessary for patients considered as ‘small movers’ as compared to four modes for ‘large movers’. The large movers were defined as those patients where the volume confined to a union of the simulated target shapes from the PCA-model using all DVFs as input was at least twice as large as the volume of the CTV in the planning CT. This definition was applied to both CTV-ln and CTV-p, whereas for CTV-sv the volume increase as compared to the planning CTV had to be at least 4.5 to be considered a large mover. For CTV-sv 90% of the variance was accounted for by the first four modes for both large and small movers. Although CTV-p had the slowest decline as a function of modes, identical number of modes as for the CTV-p was needed to account for > 90% of the modeled variance for small and large movers.
Prospectively accounting for motion patterns deduced from the PCA-model
The sensitivity and precision for all targets with varying number of DVFs for input is displayed in and , respectively. The sensitivity measure () was more influenced by the iso- coverage level than the precision. The inter-patient variation in sensitivity also increased at higher iso-coverage levels (). Based on the PCA-model with all DVFs as input, a union of the simulated target shapes (corresponding to an iso-coverage level of 0) accounted for all variation. The patient-average relative volume increase (± 1 standard deviation) of this volume as compared to the volume of the delineated CTV in the planning CT was 2.0 ± 0.4 for CTV-p, 5.4 ± 2.4 for CTV-sv and 1.9 ± 0.2 for CTV-ln. With a reduced number of DVFs included in the PCA-model a union (iso-coverage level = 0) of the simulated shapes was still able to cover at least 95%, 97% and 97% of the volumes of the CTV shapes not included in the model when four, five and six DVFs were used as input for the model, respectively. In contrast, a large decrease in precision resulted when limiting the number of input geometries as compared to including all DVFs in the model (). However, the difference in average precision from a PCA-model based on six versus four DVFs was less than 0.04 across all targets and iso-coverage levels.
Figure 2. Sensitivity as a function of iso-coverage level for a PCA-model based on all DVFs as input (solid line), with six DVFs as input (dashed line), with five DVFs as input (dashed-dotted lines) and with four DVFs as input (dotted lines). The target of CTV-p is in red, CTV-sv (green) and CTV-ln (blue). All data is displayed as average values with ± 1 SD in error bars.
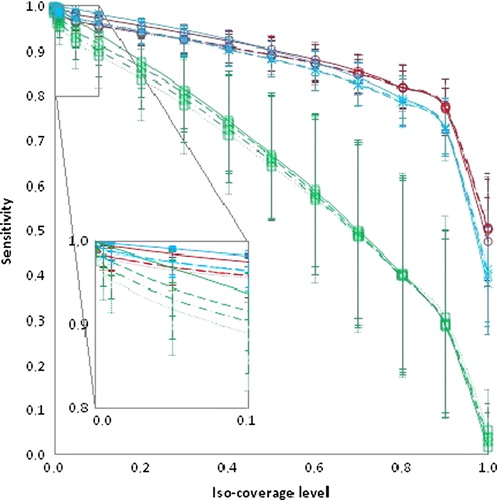
Figure 3. Precision as a function of iso-coverage level for a PCA-model based on all DVFs as input (solid line), with six DVFs as input (dashed line), with five DVFs as input (dashed-dotted lines) and with four DVFs as input (dotted lines). The target of CTV-p is in red, CTV-sv (green) and CTV-ln (blue). All data is displayed as average values with ± 1 SD in error bars.
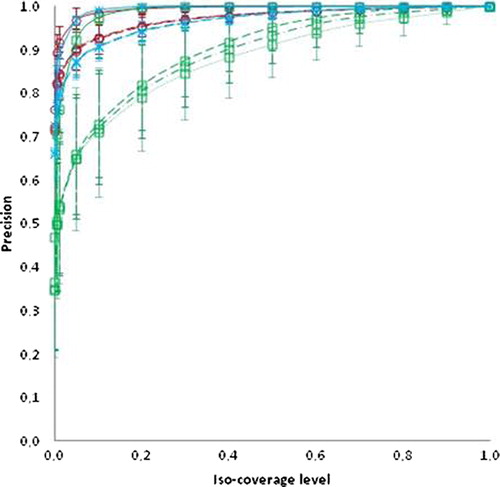
Large movers as compared to small movers displayed both reduced sensitivity and precision for each iso-coverage level, most pronounced for the elective targets. This finding was independent of the number of geometries used to build the PCA-models (Supplementary Figures 1 and 2, available online at http://informahealthcare.com/doi/abs/10.3109/0284186X.2013.818249). Comparing small and large movers, the difference in sensitivity for each iso-coverage level increased for higher levels but was relatively insensitive to the number of DVFs for the models (Supplementary Figure 1, available online at http://informahealthcare.com/doi/abs/10.3109/0284186X.2013.818249). However, it was the target with the largest relative volume of motion as compared to the delineated planning CTV (CTV-sv) that displayed the greatest influence of the number of geometries used in the PCA-model (). For the sensitivity, this was most pronounced for the lower iso-coverage levels whereas for the precision this dependency was greater for higher iso-coverage levels. The decline in sensitivity for CTV-sv and larger movers with increasing iso- coverage levels were almost linear (R > 0.99), irrespective of the number of DVFs used in the PCA-model (Supplementary Figure 1, available online at http://informahealthcare.com/doi/abs/10.3109/0284186X.2013.818249). Both CTV-p and CTV-ln displayed similar patterns in both sensitivity and precision. For iso-coverage levels lower than 0.2 both CTV-p and CTV-ln were equally successful in accounting for shape variations of the CTVs used for evaluation but for iso-levels larger than 0.2, CTV-p displayed higher sensitivity (, and Supplementary Figure 1, available online at http://informahealthcare.com/doi/abs/10.3109/0284186X.2013.818249). The iso-coverage levels where CTV-p is superior to CTV-ln in capturing the variations of the evaluated target shapes was slightly lower for larger movers (0.05–0.2) as compared to small movers (0.2–0.4) (Supplementary Figure 1, available online at http://informahealthcare.com/doi/abs/10.3109/0284186X.2013.818249) across PCA-models based on 4–6 DVFs for input. For the precision these differences between large and small movers were less pronounced (Supplementary Figure 2, available online at http://informahealthcare.com/doi/abs/10.3109/0284186X.2013.818249).
Discussion
In this study we have investigated whether a statistical motion model with a limited number of input geometries can account for subsequent target shapes excluded from the model building. This evaluation has been conducted for targets displaying different motion patterns in relation to intra-prostatic fiducial markers. We showed that a union of all simulated shapes successfully enclosed > 97% of the volumes in the target shapes used for evaluation with PCA- models based of 5–6 DVFs as input. However, this volume had the lowest precision. Ideally, the volume used to account for geometric uncertainties should have both a high sensitivity and precision. In the current study this would firstly recquire that the variations seen in the initial part of the treatment are representative for the motion during the latter part and secondly that the applied model can account for the motion. For large motion a greater trade-off between sensitivity and precision is to be expected, which was observed both regarding targets and regarding individual patients where CTV-sv and large movers had the largest range in both sensitivity and precision.
For uncertainties caused by target motion; the sensitivity can be argued to be the most important parameter. In that context the common derivation of a planning target volume (PTV) can also be considered the most robust method to handle geometric uncertainties but we and others have shown that, in particular, for the seminal vesicles large isotropic expansions are needed to cover the target motion [Citation1,Citation4,Citation8–10]. However, treatment plans with these large expansions might not be feasible to deliver due to normal tissue toxicity. Instead we aimed at adapting to the patient-specific motion patterns of the targets. Using the patient-specific union derived from the PCA-models and comparing it to the volume derived from a 5 mm isotropic expansion in the planning CT, resulted in slightly smaller volumes (expressed in relation to the delineated structures in the planning CT) of 1.9 as compared to 2.3 for CTV-p and 1.8 as compared to 2.0 for CTV-ln. Considering CTV-sv a union, i.e. iso-coverage = 0 would result in a slightly larger relative volume expansion of 5.6 as compared to 4.1 with a 5 mm isotropic expansion. However, several studies investigating seminal vesicle motion and margin requirements have found expansion larger than 5 mm to be needed [Citation4,Citation8–11]. For comparison, margins using the formalism described by van Herk et al. [Citation26] derived from rigid shift between the intra-prostatic fiducial markers and the CTV-sv would have yielded margins with an average sensitivity and precision of 0.9 and 0.6, respectively. For CTV-ln deriving the margins from rigid shifts between the markers and bony anatomy would have resulted in an average sensitivity of 1.0 and an average precision of 0.5 (detailed data not shown). For CTV-sv an average sensitivity of 0.9 and an average precision of 0.6 for PCA-models generated with 4–6 DVFs was obtained for iso-coverage levels between 0.05 and 0.1. For CTV-ln iso-coverage levels between 0.05 and 0.1 resulted in an average sensitivity 1.0 and an average precision of 0.9.
Using patient-specific PCA-models for various treatment sites, 3–6 measurements have been reported to account for > 90% of the variations, which is in agreement with our findings [Citation19,Citation20,Citation27,Citation28]. We also found a larger number of modes needed to account for 90% of the variations for small movers compared to large movers. This can be explained by large movers having a distinct and dominating motion as compared to small movers. In addition, this can explain the slower decline of the first modes for CTV-p as compared to both CTV-sv and CTV-ln () since the residual motion after setup based on intra-prostatic markers can be assumed to be more random/noisy. From these results mathematically at least 4–7 input geometries would be needed to model the motion patterns. For lung cancer Badawi et al. evaluated the impact of reducing the number of input geometries (4–7) in PCA-models and found the results to be comparable to that when all geometries (12–21) were used to build the model [Citation27].
Besides being labor intensive, manual delineations in multiple CTs may suffer from delineation uncertainties [Citation29–32]. To limit these uncertainties, the current study used delineations from one oncologist who made the segmentations in a short time interval. However, it cannot be excluded that part of the motion modeled in the study is caused by difficulties in differentiating the borders of the targets. For a clinical implementation, other deformable image registration algorithms not requiring manual delineations can be considered as well as improvements of image quality or using images from other image modalities, e.g. MR with better soft-tissue contrast [Citation29–32]. This could reduce the work-load for clinician as well as reducing the contribution of delineation uncertainties when deriving motion patterns for the proposed method.
The CTV-sv was the target where the sensitivity and precision was most influenced by the number of DVFs included in the PCA-model ( and ), with an increasing number of input geometries leading to more reliable estimates of subsequent target shapes excluded from the model. This is to be expected since larger movements causing wider probability distribution and wider distribution in turn require more samples to accurately estimate the mean value. However, even for CTV-sv the differences between four and six DVFs in the model were small for iso-coverage levels below 0.1. In addition, the steep dependency on iso-coverage levels as a function of sensitivity makes the choice of level susceptible to loss in geometric coverage. For both CTV-p and CTV-ln this effect was less pronounced and a slightly reduced sensitivity can be weighed against a gain in precision if needed, e.g. to adaptively solve a conflict with dose to an organ at risk. Whether other methods of generating coverage probabilities, e.g. not accounting for voxel- correspondence would yield similar results and comparison with other adaptive methods is part of future investigations.
In general, the sensitivity evaluation showed the decrease in sensitivity as a function of iso-coverage levels to be dependent on the motion displayed by the targets for the individual patients, with an increased steepness for the larger movers ( and Supplementary Figure 1, available online at http://informahealthcare.com/doi/abs/10.3109/0284186X.2013.818249). To evaluate if such a relationship existed was indeed the purpose behind dividing the patients into two groups for every target. The thresholds used for classifying patients into small and large movers are a bit arbitrary and different thresholds might lead to slightly different results. If applying the same threshold for CTV-sv as for the other two targets, i.e. twice the volume of the planning CTV, every CTV-sv would have been selected as large movers. Of note is that with the current applied thresholds the majority of the patients considered small movers were patients requiring margins less or equal to 3 mm in a previous study [Citation8].
The strength of our method for adaptations is that the statistical inter- and extrapolation in the multidimensional space described by the target shapes is not limited to rigid motion. Another advantage is that a large number of iso-coverage levels can be derived through these inter- and extrapolations. This would allow for ‘tuning’ of the iso-coverage levels and assessing the trade-off between a possible target miss in a defined region and a reduced dose to an organ at risk. A methodological weakness of the study is the potential dependency on the number of simulations used to create the coverage probability maps. Another weakness is the dependency of the number and choice of input shapes used both to build the PCA-model and those used for evaluation. The fluctuations of the results depending on the number of simulations were reduced by simulating 10 000 geometries for CTV-p and CTV-sv. However, due to computational limitations this number had to be limited to 5000 for CTV-ln. A more robust method for expansions based on the PCA-model might be to expand the mean geometry with certain fractions of the standard deviations. In addition, different combination of input geometries for the mode building versus for evaluation might alter the results. The motivation of our choice of using the initial scans for deriving PCA-models for a reduced number of inputs was that this reflects what would be available in an adaptive setting. To reduce the sensitivity of input selection a boot-strap method could be applied and used to derive a ‘soft-margin’ to account for variations in input sampling as similar to the method proposed by Hysing et al. [Citation33].
In conclusion, this study showed that using PCA-based simulations of residual motion derived from four to six DVFs as input could account for the majority of the volume enclosed by the target shapes excluded from building the model. Hence, it seems feasible to use PCA-model in a prospective manner within an adaptive RT strategy for locally advanced prostate cancer. The target most sensitive to the number of input geometries used was the seminal vesicles, where a model of six geometries was found superior. We also showed the decrease in sensitivity with higher iso-coverage levels was steeper with larger movement.
Supplementary Figures 1 and 2
Download PDF (1.7 MB)Declaration of interest: The authors report no conflicts of interest. The authors alone are responsible for the content and writing of the paper.
This work has been supported by research and travel grants from CIRRO – The Lundbeck Foundation Center for Interventional Research in Radiation Oncology.
References
- Smithmans MHP, de Bois J, Sonke JJ, Catton CN, Jaffray DA, Lebesque JV, et al. Residual seminal vesicle displacement in marker-based image-guided radiotherapy for prostate cancer and the impact on margin design. Int J Radiat Oncol Biol Phys 2011;80:590–6.
- Van der Wielen G, Mutanga TF, Incrocci L, Kirkels WJ, Vasquez Osorio EM, Hoogeman MS, et al. Deformation of prostate and seminal vesicles relative to intraprostatic fiducial markers,Int J Radiat Oncol Biol Phys 2008;72:1604–11.
- Liang J, Wu Q, Yan D. The role of seminal vesicle motion in target margin assessment for online image-guided radiotherapy for prostate cancer. Int J Radiat Oncol Biol Phys 2009; 73:935–43.
- Mutanga T, de Boer H, van der Wielen G, Hoogeman M, Incrocci L, Heijmen B. Margin evaluation in the presence of deformation, rotation, and translation in prostate and entire seminal vesicle irradiation with daily marker-based setup corrections. Int J Radiat Oncol Biol Phys 2011;81: 1160–7.
- Rossi PJ, Schreibmann E, Jani AB, Master VA, Johnstone PAS. Boost first, eliminate systematic error, and individualize CTV to PTV margin when treating lymph nodes in high-risk prostate cancer. Radiother Oncol 2009; 90:353–8.
- Lawton CAF, Michalski J, El-Naqa I, Buyyounouski MK, Lee R, Menard C, et al. RTOG GU radiation oncology specialist reach consensus on pelvic lymph node volumes for high-risk prostate cancer. Int J Radiat Oncol Biol Phys 2009;74:383–7.
- Hsu A, Pawlicki T, Luxton G, Hara W, King CR. A study of image-guided intensity-modulated radiotherapy with fiducials for localized prostate cancer including pelvic lymph nodes. Int J Radiat Oncol Biol Phys 2007;68:898–902.
- Thörnqvist S, Hysing LB, Zolnay AG, Söhn M, Hoogeman MS, Muren LP, et al. Evaluation of PTV margins in radiotherapy of primary and elective targets using a statistical deformable motion model and applied to locally advanced prostate cancer. Radiother Oncol (submitted).
- Mak D, Gill S, Roxby P, Stillie A, Haworth A, Kron T, et al. Seminal vesicle interfraction displacement and margins in image guided radiotherapy for prostate cancer. Radiat Oncol 2012;7:139.
- Wang Z, Wang K, Lerma FA, Liu B, Amin B, Yi B, et al. Planning margins to CTV for image-guided whole pelvis prostate cancer intensity-modulated radiotherapy. IJMPCERO 2012;1:23–31.
- Meijer GJ, de Klerk J, Bzdusek K, van den Berg H, Janssen R, Kaus MR, et al. What CTV-to-PTV margins should be applied for prostate irradiation?Four-dimensional quantitative assessment using model-based deformable image registration techniques. Int J Radiat Oncol Biol Phys 2008;72:1416–25.
- Harmenberg U, Hamdy FC, Widmark A, Lennernäs B, Nilsson S. Curative radiation therapy in prostate cancer. Acta Oncol 2011;50(Suppl 1):98–103.
- Yan D, Vicini F, Wong J, Martinez A. Adaptive radiation therapy. Phys Med Biol 1997;42:123–32.
- Vestergaard A, Søndergaard J, Petersen JB, Høyer M, Muren LP. A comparison of three different adaptive strategies in image-guided radiotherapy of bladder cancer. Acta Oncol 2010;49:1069–76.
- Bondar L, Hoogeman M, Mens JW, Dhawtal G, de Pree I, Ahmad R, et al. Toward an individualized target motion management for IMRT of cervical cancer based on model-predicted cervix-uterus shape and position. Radiother Oncol 2011;99:240–5.
- Thörnqvist S, Bentzen L, Petersen JBB, Hysing LB, Muren LP. Plan robustness of simultaneous integrated boost radiotherapy of prostate and lymph nodes for different image-guidance and delivery techniques. Acta Oncol 2011;50:926–34.
- Nakamura N, Shikama N, Takahashi O, Sekiguchi K, Hama Y, Akahane K, et al. The relationship between the bladder volume and optimal treatment planning in definitive radiotherapy for localized prostate cancer. Acta Oncol 2012;51:730–4.
- Onishi H, Kuriyama K, Komiyama T, Marino K, Araya M, Saito R, et al. Large prostate motion produced by anal contraction. Radiother Oncol 2012;104:390–4.
- Söhn M, Birkner M, Yan D, Alber M. Modelling individual geometric variation based on dominant eigenmodes of organ deformation: Implementation and evaluation. Phys Med Biol 2005;50:5893–908.
- Söhn M, Sobotta B, Alber M. Dosimetric treatment course simulation based on a statistical model of deformable organ motion. Phys Med Biol 2012;57:3693–709.
- Muren LP, Wasbø E, Helle SI, Hysing LB, Karlsdottir A, Odland OH, et al. Intensity-modulated radiotherapy of pelvic lymph nodes in locally advanced prostate cancer: Planning procedures and early experiences. Int J Radiat Oncol Biol Phys 2008;71:1034–41.
- Thörnqvist S, Petersen JBB, Høyer M, Bentzen L, Muren LP. Propagation of target and organ at risk contours in radiotherapy of prostate cancer using deformable image registration. Acta Oncol 2010;49:1023–32.
- Vásquez Osorio EM, Hoogeman MS, Bondar L, Levendag PC, Heijmen BJM. A novel flexible framework with automatic feature correspondence optimization for nonrigid registration in radiotherapy. Med Phys 2009; 36:2848–59.
- Bondar L, Hoogeman MS, Vásquez Osorio EM, Heijmen BJM. A symmetric nonrigid registration method to handle large organ deformations in cervical cancer patients. Med Phys 2010;37:3760–72.
- Budiartho E, Keijzer M, Storchi PR, Hoogeman MS, Bondar L, Mutanga TF, et al. A population-based model to describe geometrical uncertainties in radiotherapy: Applied to prostate cases. Phys Med Biol 2011;56:1045–61.
- Van Herk M, Remeijer P, Rasch C, Lebesque JV. The probability of correct target dosage: Dose-population histograms for deriving treatment margins in radiotherapy. Int J Radiat Oncol Biol Phys 2000;47:1121–35.
- Badawi AM, Weiss E, Sleeman WC. Optimizing principal component models for representing interfraction variations in lung cancer radiotherapy. Med Phys 2010;37:5080–91.
- Fontenla E, Pelizzari CA, Roeske JC, Chen GT. Numerical analysis of a model of organ motion using serial imaging measurements from prostate radiotherapy. Phys Med Biol 2001;46:2337–58.
- Choi HJ, Kim YS, Lee SH, Lee YS, Park G, Jung JH, et al. Inter- and intra-observer variability in contouring of the prostate gland on planning computed tomography and cone beam computed tomography. Acta Oncol 2011; 50:539–46.
- Gambacorta MA, Valentini C, Dinapoli N, Boldrini L, Caria N, Barba MC, et al. Clinical validation of atlas-based auto-segmentation of pelvic volumes and normal tissue in rectal tumors using auto-segmentation computed system. Acta Oncol 2013 (in press).
- De Brabandere M, Hoskin P, Haustermans K, Van den Heuvel F, Siebert FA. Prostate post-implant dosimetry: Interobserver variability in seed localisation, contouring and fusion. Radiother Oncol 2012;104:192–8.
- Lütgendorf-Caucig C, Fotina I, Stock M, Pötter R, Goldner G, Georg D. Feasibility of CBCT-based target and normal structure delineation in prostate cancer radiotherapy: Multi-observer and image multi-modality study. Radiother Oncol 2011;98:154–61.
- Hysing LB, Söhn M, Muren LP, Alber M. A coverage probability based method to estimate patient-specific small bowel planning volumes for use in radiotherapy. Radiother Oncol 2011;100:407–11.