Abstract
Background. Although several candidate gene polymorphisms (SNPs) have been associated with increased risk of type 2 diabetes mellitus (T2DM), relatively few studies have assessed the ability of T2DM candidate genes to assess the risk of impaired fasting glucose (IFG), impaired glucose tolerance (IGT), and T2DM beyond the information provided by clinical risk factors.
Objective. To test whether the inclusion of genetic markers in a regression model provides a better assessment of the risk of IFG, IGT, and T2DM than a model based only on non-genetic risk factors commonly assessed in clinical settings. Methods. Subjects (n = 485; 213 parents, 272 offspring) from the Quebec Family Study, not known to haveT2DM, were measured for several risk factors and underwent an oral glucose tolerance test. Thirty-eight SNPs in 25 susceptibility/ candidate genes previously reported to be associated with T2DM were genotyped. In order to identify risk factors associated with IFG/IGT/T2DM, two logistic regression models were tested: a full model (FM) including age, sex, body mass index (BMI), systolic and diastolic blood pressure, smoking status, and the 38 SNPs; and a reduced model (RM), in which the SNPs were dropped, which allowed us to test the null-hypothesis that the markers are not associated with the risk of IFG/IGT/T2DM. Performances of the models were compared by using a likelihood ratio test and the receiver-operating characteristic curves (ROC).The area under the curve (AUC) was calculated from the ROC curve.
Results. The analyses showed that age (P < 0.0001), BMI (P < 0.0001), and six variants (IGF2BP2 rs4402960, P = 0.002; ADIPOQ+276 G>T, P = 0.004; UCP2Ala55Val, P = 0.01; CDKN2AI2B rs3731201, P = 0.02; rs495490, P = 0.02, and rsl 0811661, P = 0.03) were significantly associated with the risk of IFG/IGT/T2DM. Dropping genetic markers from the analysis significantly reduced the fit of the model to the data (chi-square = 38.98, P < 0.00001 contrasting RM to FM), suggesting that the genetic markers are significantly associated with the risk of IFG/IGT/T2DM. Furthermore, the AUC was higher for FM than for RM (0.85 (95% CI 0.81–0.89) versus 0.81 (95% CI 0.76–0.85), P = 0.004). Conclusion. Our results suggest that combining genetic markers with traditional clinical risk factors has the potential to improve our ability to assess the risk of complex diseases such as T2DM.
Key message
Combining genetic markers and traditional clinical risk factors has the potential to improve the risk assessment of pre-diabetes and diabetes.
Abbreviations | ||
ABCC8 | = | sulfonylurea receptor 1 |
ADRB2 | = | β2 adrenergic receptor |
ADRB3 | = | β3 adrenergic receptor |
ADIPOQ | = | adiponectin |
AUC | = | area under the curve |
BMI | = | body mass index |
CDKAL1 | = | CDK5 regulatory subunit-associated protein 1-like 1 |
CDKN2AI2B | = | cyclin-dependent kinase inhibitor 2A/2B |
CI | = | confidence interval |
FABP2 | = | fatty acid-binding protein 2 |
FM | = | full model |
FPG | = | fasting plasma glucose |
GWAS | = | genome-wide association studies |
GYS1 | = | glycogen synthase 1 |
HHEX/IDE | = | hematopoietically expressed homeo-box/insulin-degrading enzyme |
HNF1B | = | hepatocyte nuclear factor 1 β |
HNF4A | = | hepatocyte nuclear factor 4 α |
HWE | = | Hardy-Weinberg equilibrium |
IGF2BP2 | = | insulin-like growth factors 2 mRNA-binding protein 2 |
IFG | = | impaired fasting glucose |
IGT | = | impaired glucose tolerance |
IL6 | = | interleukin 6 |
IRS1 | = | insulin receptor substrate 1 |
KCNJ11 | = | potassium inwardly rectifying channel, subfamily J, member 11 |
LD | = | linkage disequilibrium |
LIPC | = | hepatic lipase |
LRT | = | likelihood ratio test |
OGTT | = | oral glucose tolerance test |
OPRM1 | = | opioid receptor mu 1 |
OR | = | odds ratio |
PPARGC1A | = | PPARG co-activator 1 |
PPARA | = | peroxisome proliferator-activated receptor α |
PPARG | = | peroxisome proliferator-activated receptor γ |
QFS | = | Quebec Family Study |
RM | = | reduced model |
ROC | = | receiver-operating characteristic curve |
SLC30A8 | = | solute carrier family 30 (zinc transporter), member 8 |
SNPs | = | single nucleotide polymorphisms |
T2DM | = | type 2 diabetes mellitus |
TCF7L2 | = | transcription factor 7-like 2 |
TNFA | = | tumor necrosis factor a |
UCP2 | = | uncoupling protein 2 |
WFS1 | = | wolfram syndrome 1 |
WHO | = | World Health Organization |
Introduction
Current data indicate that there are worldwide more than 300 million individuals with impaired glucose tolerance (IGT) and 246 million with type 2 diabetes mellitus (T2DM) (Citation1). These two conditions are frequently asymptomatic (Citation2) and associated with increased risk of cardiovascular disease and premature death (Citation3). Efforts directed at preventing or delaying the development of T2DM by intervening at the ‘pre-diabetes’ stage are therefore an important next step. Several studies have already demonstrated the potential of diet and exercise, with or without pharmacological therapy, to reduce progression from IGT to T2DM (Citation4–6). However, before being able to prevent the disease, it is essential to develop strategies allowing early identification of at-risk individuals who might benefit from these interventions. The question still remains: How can these individuals be best identified?
Several tools have been developed, notably questionnaires and risk scores, to allow health care professionals to identify individuals at risk for T2DM (Citation7). These screening tools are based on information that is ordinarily available in routine clinical settings, such as age, gender, ethnicity, body mass index, family history of T2DM, lifestyle habits, medication use, and glucose or lipid plasma levels. All of these factors have been reported to be associated withT2DM risk. A genetic susceptibility to T2DM is known to be present in large segments of the population, and several single nucleotide polymorphisms (SNPs) have been associated with increased risk of the disease (Citation8). From a clinical point of view, it is of great importance to investigate whether the use of the identified risk variants, in addition to traditional clinical risk factors, may help in the determination of an individual's risk of developing T2DM.
Previous empirical studies on the predictive value of genetic variants inT2DM, either alone or in addition to clinical risk factors, have been conducted. Weedon et al. (Citation9) reported that individuals with KCNJ11Glu23Lys, PPARGProl2Ala, and TCF7L2rs790146 risk alleles had a 5.71-fold (95% CI 1.15–28.3) increased risk of diabetes compared to those with no risk allele. They calculated the area under the receiver-operating characteristic (ROC) curves (AUC) for the three variants and obtained an AUC of 0.58. Lyssenko and al. (Citation10) showed an impressive 21.2-fold increased risk for T2DM in obese individuals with elevated fasting plasma glucose (FPG) and carrying the combined PPARG Prol2Pro and CAP10 SNP43/44 GG/TT risk genotypes compared to individuals who had none of these risk factors. However, when the AUC was calculated for body mass index (BMI) and FPG alone and for BMI, FPG, and genetic markers, an AUC of 0.68 was found for both models (Citation11), suggesting that adding genetic testing did not improve the prediction of T2DM beyond that provided by BMI and FPG. Similarly, Vaxillaire et al. (Citation12) investigated three SNPs (GCK -308 G>A, IL6-174 G>C, TCF7L2rs7903146) and found that the predictive value was quite similar with either clinical characteristics alone or with a combination of clinical characteristics and genetic information. Since 2007, genome-wide association studies (GWAS) have led to the discovery of novel variants predisposing to T2DM (Citation13–19), and several studies investigated whether adding these genetic variants to clinical characteristics may improve T2DM diagnostic accuracy (Citation20–24). All studies to some extent have come to the conclusion that the addition of genetic factors to those measured in clinic, i.e. age, sex, BMI, family history of diabetes, FPG, etc., albeit significant, is modest.
In the present study, we aimed to compare the performance of two regression models in assessing the risk of undiagnosed impaired fasting glucose/ impaired glucose tolerance/type 2 diabetes (IFG/ IGT/T2DM): a full model including genetic and clinical risk factors and a reduced model in which we dropped the genetic markers. Our goal was to evaluate whether the inclusion of genetic information significantly improves our ability to assess the risk of IFG/IGT/T2DM above and beyond the information provided by a model based only on risk factors routinely collected in clinical settings.
Methods
Study design
The Quebec Family Study (QFS) has been described in detail elsewhere (Citation25). Briefly, QFS is composed of French-Canadian families living in and around the Quebec City area. The QFS sample is composed of a mixture of randomly ascertained families (phase 1) and families ascertained through an obese (BMI > 30 kg/m2) proband.The total QFS sample includes 951 individuals from 223 nuclear families. The present is based on 485 adults from 164 nuclear families (213 parents, 272 offspring, aged 18–78 years) with a wide range of adiposity (BMI 17.0–65.0 kg/m2), not known to haveT2DM at their entry in the study and who underwent a 75-g oral glucose tolerance test (OGTT). The Ethics Committee of Laval University approved the protocol and all participants gave their written consent to participate in the study.
Phenotypes
Variables included in the regression models were chosen because they are recognized as risk factors for T2DM and are ordinarily available in a routine clinical setting. Height and weight were measured to the nearest 0.1 cm and 0.1 kg, respectively, with a stadiometer and a balance-beam scale, and BMI was calculated (kg/m2). Blood pressure was measured with a mercury sphygmomanometer as previously described (Citation26). Information on smoking habits was obtained from a self-administered questionnaire. The participants underwent an OGTT after an overnight fast. Blood glucose levels were measured at -15, 0, 15, 30, 45, 60, 120, 150, and 180 minutes after the glucose load and assayed as previously described (Citation27). Impaired fasting glucose, impaired glucose tolerance, and T2DM diagnosis were based on fasting and 2-h glucose plasma levels according to the World Health Organization (WHO) criteria (Citation28). Following the OGTT, 11 individuals had IFG (6.1 mmol/L < fasting glucose < 7.0 mmol/L, and 2-h glucose < 7.8 mmol/L), 68 had IGT (fasting glucose < 7.0 mmol/L, and 7.8 mmoL < 2-h glucose < 11.1 mmol/L), and 20 were newly diagnosed as diabetic (fasting glucose > 7.0 mmol/L, or 2-h glucose > 11.1 mmol/L).
Genotyping
presents the variants tested and their allelic frequencies. The candidate regions were selected on the basis of two criteria: they were associated with T2DM or related phenotypes in at least three studies or were significantly associated with diabetes-related phenotypes in prior QFS reports (see Supplementary Table I online for the list of studies that were used for SNPs selection—http://www.informahealthcare.com[DOI]). Genomic DNA was obtained from cultured lymphoblastoid cell lines by protei-nase K and phenol/chloroform extraction procedure. Variants in the following genes were genotyped using the polymerase chain reaction (PCR) (Citation29): adi-ponectin (ADIPOQ), glycogen synthase 1 (GYS1), insulin receptor substrate 1 (IRS1), peroxisome proliferator-activated receptor y (PPARG), PPARG co-activator 1 (PPARGC1A), peroxisome prolifera-tor- activated receptor a (PPARA), (32 adrenergic receptor (ADRB2), (33 adrenergic receptor (ADRB3), fatty acid-binding protein 2 (FABP2), hepatic lipase (LIPG), interleukin 6 (IL6), tumor necrosis factor a (TNFA), hepatocyte nuclear factor 4 a (HNF4A), opioid receptor mu 1 (OPRM1), sulfonylurea receptor 1 (ABCC8), and uncoupling protein 2 (UCP2). Variants in CDK5 regulatory subunit-associated protein 1-like 1 (CDKAL1); cyclin-dependent kinase inhibitor 2A/2B (CDKN2A/2B); hemato poietically expressed homeobox/insulin-degrading enzyme (HHEX/IDE); insulin-like growth factors 2 mRNA binding protein 2 (IGF2BP2); potassium inwardly rectifying channel, subfamily J, member 11 (KCNJ11); solute carrier family 30 (zinc transporter), member 8 (SLC30A8); hepatocyte nuclear factor 1 (3 {HNF1B); transcription factor 7-like 2 (TCF7L2); and Wolfram syndrome 1 (WFS1) were genotyped using the Illumina Golden Gate assay on the Illumina Bead Station platform (Illumina Inc., San Diego, CA) or the TaqMan methodology of Applied Biosystem Company (Citation30). All the genotyping results were stored in a local dBase IV database, GENEMARK, and Mendelian inheritance incompatibilities were verified using the Pedcheck software (Citation31). Subjects with Mendelian incompatibilities were excluded from the database automatically by GENEMARK and retyped completely.
Table I. Polymorphisms analyzed in the present study.
Statistical analysis
Deviation from Hardy-Weinberg equilibrium (HWE) and linkage disequilibrium (LD) among SNPs located in a given gene were tested in unrelated individuals (i.e. in the parents, n = 213) using the ALLELE procedure implemented in SAS (SAS Institute, Cary, NC, version 9.1.3).The pairwise LD among SNPs was assessed by r2 and D’ (Citation32).
Two multiple logistic regression models were tested to identify predictors of IFG/IGTT2DM. We first tested a ‘full model’ (FM) which included age, sex, BMI, systolic and diastolic blood pressure, smoking status, and the 38 variants as independent variables. The non-genetic clinical variables were forced into the model, while the stepwise selection method was applied to the SNPs in order to retain only those contributing significantly to the risk in the presence of the clinical variables chosen. A second ‘reduced model’ (RM), which included the same independent variables as in the full model, except the genetic variants, was then tested. Since the family structures and the potential intra-family correlations can influence risk estimation, we tested the familial resemblance for IFG/IGTT2DM before performing the regression analyses. An analysis of variance on IFG/IGT/T2DM revealed that the variance accounted for by family lines was not significant γ2 = 35%, P = 0.29). In the absence of familial resemblance, the relatedness among family members was not taken into account in the logistic regression analyses (LOGISTIC procedure implemented in SAS, version 9.13; SAS Institute, Cary, NC).
Comparison of the models
Predictive ability. The goodness of fit of the reduced model without the genetic markers was compared to the full model using a likelihood ratio test (LRT). Twice the difference in the log likelihood of the two models asymptotically yields a distribution of chi-square with degrees of freedom (df) equal to the number of constrained parameters. A significant chi-square statistic indicates that the reduced model is rejected, which suggest that the risk of IFG/IGTT2DM accounted for by a model including genetic markers fits the data better than a model without such data.
Discriminative ability. The ability of the two models to discriminate between subjects with and without IFG/IGT/T2DM was also investigated by plotting ROC curves. The ROC curves characterize the relationship between sensitivity and 1-specificity. The ROC curves can therefore be used to visualize how well the test correctly distinguishes subjects with IFG/IGT/T2DM from subjects without IFG/IGT/ T2DM.The closer the ROC curve is to the diagonal (see ), the less useful the model is at discriminating between cases and controls. This closeness to the diagonal can be quantified by calculating the AUC which can take values from 0.5 (no discrimination) to 1 (perfect discrimination); the larger the AUC, the better is the discriminative ability. The AUC were calculated by using the LOGISTIC procedure implemented in SAS (version 9.13; SAS Institute, Cary, NC).The 95% CIs of the AUCs and the statistical significance of difference in these areas were estimated by using the algorithm developed by DeLong and colleagues (Citation33).
Figure 1. Comparison of areas under the receiving-operating characteristic (ROC) curves. Area under the ROC curve for the full model (gray): 0.85 (95% CI 0.81–0.89). Area under the ROC curve for the reduced model (black): 0.81 (95% CI 0.76–0.85). P-value for the difference between the areas under the ROC curves of the two models: 0.004. (From DeLong et al. (33).)
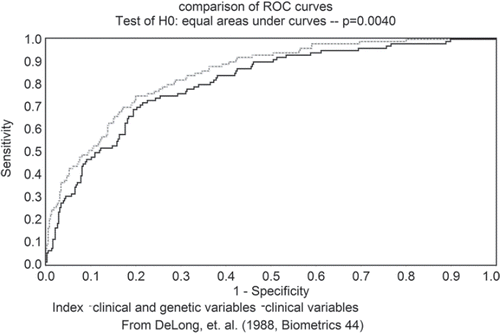
Power calculations performed in the Quebec Family Study cohort indicate that under a sample size of 500 subjects, we have 99% power at a = 0.05 and 99% at a = 0.01 to detect an association for a variant affecting 5% of the population and having a mean displacement between carriers and non-carriers of 2.0 standard deviation units.
Results
The genetic variants analyzed in our study are presented in . They were all in HWE (P > 0.05 from a chi-square test), and those within a given gene were not in complete LD (i.e. D’ < 1.0, and r2 < 1.0). The characteristics of the participants included in the study are presented in . As expected, compared to normal glucose-tolerant subjects, the IFG/IGT/T2DM subjects were older, presented greater adiposity, had higher systolic and diastolic blood pressure, and higher fasting and 2-h glucose levels P = 0.0001 for all).
Table II. Characteristics of the participants.
Results of logistic regression analyses () showed that age P = 0.0001), BMI P = 0.0001), and six variants (IGF2BP2 rs4402960, P = 0.002; ADIPOQ+276 G>T, P = 0.004; UCP2 Ala55Val, P = 0.01; CDKN2AI2B rs3731201, P = 0.02; rs495490, P = 0.02; and rsl0811661, P = 0.03) contributed significantly to the risk of IFG/IGT/ T2DM in the full model, whereas age P = 0.0001) and BMI (P < 0.0001) contributed significantly to the risk in the reduced model. Results from the full model indicate that, for each genetic marker, independently of the other variables in the model, carrying one of the risk alleles was associated with a 2.0–5.8-fold increased risk for undiagnosed IFG/ IGT/T2DM. The highest risk was associated with the CDKN2AI2B rsl0811661 polymorphism (OR = 5.8, 95% CI 1.47–39.79, P = 0.03). As shown in , the full model explains a greater amount of variance in the prediction of IFG/IGT/T2DM than the reduced model γ2 = 38.6% for FM versus R2 = 28.7% for RM). In line with this observation, the likelihood ratio test reveals that dropping the genetic markers from the full model decreased significantly the ability to predict undiagnosed IFG/IGT/T2DM (chi-square = 38.98, P < 0.00001 contrasting RM to FM). Moreover, the areas under the ROC curves presented in show that the two models are relatively good at discriminating between subjects with and without IFG/IGT/T2DM, but suggest that the model with the genetic markers performs better (farther form the diagonal). Indeed, the analysis revealed that the AUC was significantly higher for the full model (with the genetic markers) than for the reduced model (0.85 (95% CI 0.81–0.89) versus 0.81 (95% CI 0.76–0.85), P = 0.004).
Table III. Results of logistic regression analyses predicting undiagnosed IFG/IGT/T2DM.
Discussion
Clinical trials have demonstrated that high-risk individuals, defined as having IGT, can reduce their risk of T2DM by more than half by lifestyle modification programs and to a lesser extent with preventive pharmacological interventions (Citation4–6). It would therefore be useful to develop effective methods to identify people at high risk for T2DM who might benefit from such interventions. The objective of the present study was to evaluate whether the inclusion of genetic markers in a model has the ability to better assess the risk of undiagnosed IFG, IGT, and T2DM beyond the information provided by non-genetic measures commonly assessed in clinical settings. We developed two models for selecting subjects with an increased risk of having undiagnosed IFG, IGT, or T2DM. In the first model we included non-genetic and genetic information, whereas in the second model we dropped information provided by markers and evaluated whether this significantly reduced the fit to the data.
One of our principal findings is that individuals carrying one of the risk alleles ofIGF2BP2 rs44029 60, ADIPOQ+276 G>T, UCP2Ala55Val,CDKN2AI2B rs3731201, rs495490, or rsl0811661 have a 2.0–5.8-fold increased risk for undiagnosed IFG/IGT/ T2DM (). The contribution of these six variants to the risk of impaired glucose metabolism is not surprising since they have been previously reported to be associated with the disease. CDKN2AI2Brs 10811661, which was associated with the greatest risk (OR = 5.8, 95% CI 1.47–39.79),has been identified through GWAS (Citation14,Citation15,Citation19) and further confirmed as a strong diabetes variant (Citation34–38). The comparison of the two models tested in the present study by using the likelihood ratio test clearly shows that dropping genetic information from the full model decreased significantly its capacity to predict deterioration in glucose tolerance (). Indeed, the ‘-2 log likelihood’, which is a measure of the variance remaining unexplained when all the independent variables from the model are considered, was higher for the reduced model than for the full model. Moreover, the variance accounted for by the full model was higher than the one accounted for by the reduced model. Similarly, the comparison of the area under the ROC curve of the two models revealed a modest, albeit significant, difference in discriminative ability of the model with the genetic markers compared to the model without these markers (). Our results suggest that the combination of genetic and non-genetic information in a model assessing the risk of IFG/IGT/T2DM performs better than a model based only on clinical risk factors, which demonstrates the clinical potential to use genetic information in the assessment of the disease risk.
Previous studies have investigated the impact of genetic variants and/or clinical characteristics on risk of T2DM. An overview of these studies is presented in , which presents the area under the ROC curves for the various predictive models reported in these studies. Among those, the study by Cauchi et al. (Citation39) looked at the combined effect of 15 novel loci identified by GWAS and showed that individuals carrying 18–30 risk alleles (9.8% of the population) had an increased risk of T2DM (OR = 8.68, 95% CI 6.37–11.83) compared to the reference group (0–10 risk alleles, 7.5%). The estimated area under the ROC curve for clinical characteristics (age, sex, and BMI) and the 15 variants was 0.86. However, as suggested by Janssens et al. (Citation40), the usefulness of genetic markers for predicting T2DM should be evaluated by comparing the discriminatory accuracy of predictions based on models that do and do not include genetic markers in order to evaluate whether genetic testing will improve the prediction of T2DM in the presence of information on non-genetic factors.
Table VI. Overview of type 2 diabetes predictive models including genetic and/or clinical risk factors.a (P-values are for comparison between AUC for clinical characteristics and for genetic variants plus clinical characteristics.)
In recent months, five studies that investigated whether adding genetic information to clinical characteristics may improve T2DM diagnostic accuracy have been published (Citation20–24) (). The Genetics of Diabetes Audit and Reseach Tayside Study (GoDARTS) (Citation20) and the Rotterdam Study (Citation23) examined 18 SNPs (14 were identical in both studies). Carriers of more than 24 risk alleles had a prevalence ratio of 4.2 (95% CI 2.11–8.56) in the GoDARTS and 2.10 (95% CI 1.04–4.22) in the Rotterdam Study as compared with carriers of less than 12 risk alleles. In the GoDARTS, the AUCs were 0.60,0.78, and 0.80 for models containing the genetic variants, the clinical characteristics (age, sex and BMI), and the combination of genetic and clinical information, whereas in the Rotterdam Study, the AUCs were 0.60, 0.66, and 0.68, respectively. Although marginal, the increase in the AUC when genetic variants were combined with clinical characteristic was statistically significant in both studies (GoDARTS: P= 2.88 x 1012,and Rotterdam Study: P< 0.0001). Lyssenko et al. (Citation21) and van Meigs et al. (Citation22) have tested several T2DM-predictive models that include or do not include genetic information. The former reported that the addition of genetic information (16 variants) to clinical factors slightly improved the prediction of future T2DM, with the AUC increasing from 0.74 to 0.75 (P = 0.0001), whereas the latter demonstrated that when clinical risk factors were considered, the genetic information (18 genetic markers, of which 14 were studied by Lyssenko et al.) did not improve risk discrimination. Finally, Miyake et al. (Citation24) tested on a Japanese population a predictive model for T2DM containing risk alleles for 11 genes, as well as age, sex, and BMI.The discriminative capacity (AUC) of the model including either genetic factors, clinical factors, or the combination of both, was respectively 0.63, 0.68, and 0.72.
Our study raised several points that should be discussed. First, we should emphasize that established models to predict T2DM, such as the Framingham risk score, include fasting glucose, whereas the predictive model of the current paper does not. Our study therefore demonstrates that T2DM-predictive models may have good predictive and discriminative capacities without including fasting glucose levels. Furthermore, our predictive models were based on the assessment of the risk of impaired glucose metabolism while those of the other studies predicted the risk of T2DM. This difference in the outcome prediction could explain the stronger odds ratio associated with the variants and the higher AUC values that we have found. Variants may indeed have stronger effects on pre-diabetic phenotypes than on the final disease phenotype. In this respect, Lyssenko et al. (Citation21) demonstrated that genetic information may have the greatest yield before other risk factors have appeared, suggesting that assessment of genetic risk factors is clinically more meaningful the earlier in life they are measured, i.e. before the development of T2DM and its complications. Our study has some limitations, the main being its cross-sectional design that did not allow us to truly determine the predictive power of the variants. Moreover, the small number of participants could have resulted in false positive associations. However, the variants that contributed significantly to the model were previously reported to be strong susceptibility genes for T2DM. Of note, a large number of candidate regions selected in the current study were different from those investigated in the other studies. Importantly, we did not assess the effects of the most recently identified T2DM variants (Citation18). The discriminative accuracy of predictive genetic testing in complex diseases depending on the number of genes involved, the risk allele frequencies, and the size of the associated risks (Citation41), and the impact of several missing genes that are strongly associated with T2DM could have led to a decrease in the predictive power of our full model.
The present study and those of others illustrate that combining genetic information and non-genetic factors has the potential to improve prediction of pre-diabetes and diabetes. However, the discriminative ability conferred by the addition of genetic markers, albeit significant, is modest. Therefore, the use of genetic information as a screening tool is not clinically efficient at this time. It has been estimated that only about 20–25 common risk variants (allele frequencies > 10%) with small effect sizes (OR 1.2–1.5) would be needed to explain 50% of the inherited risk of T2DM (Citation42). With the rapid progress made in identifying T2DM susceptibility genes, we can expect that in the near future an array of markers will be defined with sufficient clinical relevance for the prediction of T2DM risk.
Supplementary Material
Download PDF (40.2 KB)Acknowledgements
The Quebec Family Study was supported over the years by multiple grants from the Medical Research Council of Canada and the Canadian Institutes for Health Research (PG-11811, MT-13960, and GR-15187) as well as other agencies. This work was supported by a grant from the Canadian Institutes of Health Research (CIHR)—New Emerging Team Program (NET) (#OHN-63276). S.-M. Ruchat is supported by a scholarship from the Canadian Diabetes Association. C. Bouchard is partially funded by the George A. Bray Chair in Nutrition. Thanks are expressed to Dr G. Theriault and to G. Fournier, L. Allard, M. Chagnon, and C. Leblanc for their contributions to the recruitment and data collection of QFS.
Declaration of interest: The authors report no conflicts of interest. The authors alone are responsible for the content and writing of the paper.
References
- International Diabetes Federation. Diabetes Atlas 2007. Available from: www.eatlas.idf.org (accessed 1 February 2007).
- Cowie CC, Rust KF, Byrd-Holt DD, Eberhardt MS, Flegal KM, Engelgau MM, . Prevalence of diabetes and impaired fasting glucose in adults in the U. S. population: National Health And Nutrition Examination Survey 1999–2002. Diabetes Care.2006;29:1263–8.
- Harris MI, Eastman RC. Early detection of undiagnosed diabetes mellitus: a US perspective. Diabetes Metab Res Rev.2000;16:230–6.
- Chiasson JL, Josse RG, Gomis R, Hanefeld M, Karasik A, Laakso M. Acarbose for prevention of type 2 diabetes mellitus: the STOP-NIDDM randomised trial. Lancet.2002;359:2072–7.
- Knowler WC, Barrett-Connor E, Fowler SE, Hamman RF, Lachin JM, Walker EA, . Reduction in the incidence of type 2 diabetes with lifestyle intervention or metformin. N Engl J Med.2002;346:393–403.
- Tuomilehto J, Lindstrom J, Eriksson JG, Valle TT, Hama-lainen H, Ilanne-Parikka P, . Prevention of type 2 diabetes mellitus by changes in lifestyle among subjects with impaired glucose tolerance. N Engl J Med.2001;344: 1343–50.
- Schwarz PE, Li J, Lindstrom J, Tuomilehto J. Tools for predicting the risk of type 2 diabetes in daily practice. Horm Metab Res.2009;41:86–97.
- Freeman H, Cox RD. Type-2 diabetes: A cocktail of genetic discovery. Hum Mol Genet. 2006; 15 Spec No 2:R202–9.
- Weedon MN, McCarthy MI, Hitman G, Walker M, Groves CJ, Zeggini E, . Combining information from common type 2 diabetes risk polymorphisms improves disease prediction. PLoS Med. 2006;3:e374.
- Lyssenko V, Almgren P, Anevski D, Orho-Melander M, Sjogren M, Saloranta C, . Genetic prediction of future type 2 diabetes. PLoS Med. 2005;2:e345.
- Janssens AC, Gwinn M, Khoury MJ, Subramonia-Iyer S. Does genetic testing really improve the prediction of future type 2 diabetes? PLoS Med. 2006;3:ell4; author reply e27.
- Vaxillaire M, Veslot J, Dina C, Proenca C, Cauchi S, Charpentier G, . Impact of common type 2 diabetes risk polymorphisms in the DESIR prospective study. Diabetes.2008;57:244–54.
- Gudmundsson J, Sulem P, Steinthorsdottir V, Bergthorsson JT, Thorleifsson G, Manolescu A, .Two variants on chromosome 17 confer prostate cancer risk, and the one inTCF2 protects against type 2 diabetes. Nat Genet.2007;39: 977–83.
- Saxena R, Voight BF, Lyssenko V, Burn NP, de Bakker PI, Chen H, . Genome-wide association analysis identifies loci for type 2 diabetes and triglyceride levels. Science.2007;316:1331–6.
- Scott LJ, Mohlke KL, Bonnycastle LL, Wilier CJ, Li Y, Duren WL, . A genome-wide association study of type 2 diabetes in Finns detects multiple susceptibility variants. Science.2007;316:1341–5.
- Sladek R, Rocheleau G, Rung J, Dina C, Shen L, Serre D, . A genome-wide association study identifies novel risk loci for type 2 diabetes. Nature.2007;445:881–5.
- Wellcome Trust Case Control Consortium. Genome-wide association study of 14,000 cases of seven common diseases and 3,000 shared controls. Nature.2007;447:661–78.
- Zeggini E, Scott LJ, Saxena R, Voight BF, Marchini JL, Hu T, . Meta-analysis of genome-wide association data and large-scale replication identifies additional susceptibility loci for type 2 diabetes. Nat Genet.2008;40:638–45.
- Zeggini E, Weedon MN, Lindgren CM, Frayling TM, Elliott KS, Lango H, . Replication of genome-wide association signals in UK samples reveals risk loci for type 2 diabetes. Science. 2007;316:1336–41.
- Lango H, Palmer CN, Morris AD, Zeggini E, Hattersley AT, McCarthy MI, . Assessing the combined impact of 18 common genetic variants of modest effect sizes on type 2 diabetes risk. Diabetes.2008;57:3129–35.
- Lyssenko V, Jonsson A, Almgren P, Pulizzi N, Isomaa B, Tuomi T, . Clinical risk factors, DNA variants, and the development of type 2 diabetes. N Engl J Med.2008;359: 2220–32.
- Meigs JB, Shrader P, Sullivan LM, McAteer JB, Fox CS, Dupuis J, . Genotype score in addition to common risk factors for prediction of type 2 diabetes. N Engl J Med.2008;359:2208–19.
- van Hoek M, Dehghan A, Witteman JC, van Duijn CM, Uitterlinden AG, Oostra BA, . Predicting type 2 diabetes based on polymorphisms from genome-wide association studies: a population-based study. Diabetes.2008;57: 3122–8.
- Myake K, Yang W, Hara K, Yasuda K, Horikawa Y, Osawa H, . Construction of a prediction model for type 2 diabetes mellitus in the Japanese population based on 11 genes with strong evidence of the association. J Hum Genet. 2009;54:236–1.
- Bouchard C. Genetic epidemiology, association and sib-pair linkage: results from the Quebec Family Study. Bray GA, Ryan DH, editors. Molecular and genetic aspects of obesity. Baton Rouge: Louisiana State University Press; 1996. p. 470–81.
- Perusse L, Rice T, Bouchard C, Vogler GP, Rao DC. Cardiovascular risk factors in a French-Canadian population: resolution of genetic and familial environmental effects on blood pressure by using extensive information on environmental correlates. Am J Hum Genet.1989;45: 240–51.
- Rice T, Nadeau A, Perusse L, Bouchard C, Rao DC. Familial correlations in the Quebec family study: cross-trait familial resemblance for body fat with plasma glucose and insulin. Diabetologia.1996;39:1357–64.
- World Health Organization. Definition, diagnosis and classification of diabetes mellitus and its complications. Geneva: World Health Organization; 1999.
- Chagnon YC, Borecki IB, Perusse L, Roy S, Lacaille M, Chagnon M, . Genome-wide search for genes related to the fat-free body mass in the Quebec family study. Metabolism.2000;49:203–7.
- Livak KJ. Allelic discrimination using fluorogenic probes and the 5’ nuclease assay. Genet Anal.1999;14:143–9.
- O’Connell JR, Weeks DE. PedCheck: a program for identification of genotype incompatibilities in linkage analysis. Am JHum Genet.1998;63:259–66.
- Devlin B, Risch N. A comparison of linkage disequilibrium measures for fine-scale mapping. Genomics.1995;29: 311–22.
- DeLong ER, DeLong DM, Clarke-Pearson DL. Comparing the areas under two or more correlated receiver operating characteristic curves: a nonparametric approach. Biometrics. 1988;44:837–45.
- Hertel JK, Johansson S, Raeder H, Midthjell K, Lyssenko V, Groop L, . Genetic analysis of recently identified type 2 diabetes loci in 1,638 unselected patients with type 2 diabetes and 1,858 control participants from a Norwegian population-based cohort (the HUNT study). Diabetologia.2008;51:971–7.
- Horikawa Y, Myake K, Yasuda K, Enya M, Hirota Y, Yamagata K, . Replication of genome-wide association studies of type 2 diabetes susceptibility in Japan. J Clin Endocrinol Metab.2008;93:3136–41.
- Lee YH, Rang ES, Kim SH, Han SJ, Kim CH, Kim HJ, . Association between polymorphisms in SLC30A8, HHEX, CDKN2A/B, IGF2BP2, FTO, WFS1, CDKAL1, KCNQ1 and type 2 diabetes in the Korean population. J Hum Genet.2008;53:991–8.
- Omori S, Tanaka Y, Takahashi A, Hirose H, Kashiwagi A, Kaku K, . Association of CDKAL1, IGF2BP2, CDKN2A/B, HHEX, SLC30A8, and KCNJ11 with susceptibility to type 2 diabetes in a Japanese population. Diabetes.2008;57:791–5.
- Tabara Y, Osawa H, Kawamoto R, Onuma H, Shimizu I, Mki T, . Replication study of candidate genes associated with type 2 diabetes based on genome-wide screening. Diabetes.2009;58:493–8.
- Cauchi S, Meyre D, Durand E, Proenca C, Marre M, Hadjadj S, . Post genome-wide association studies of novel genes associated with type 2 diabetes show gene-gene interaction and high predictive value. PLoS One. 2008; 3:e2031.
- Janssens AC, Pardo MC, Steyerberg EW, van Duijn CM. Revisiting the clinical validity of multiplex genetic testing in complex diseases. Am J Hum Genet.2004;74:585–8.
- Janssens AC, Aulchenko YS, Elefante S, Borsboom GJ, Steyerberg EW, van Duijn CM. Predictive testing for complex diseases using multiple genes: fact or fiction? Genet Med. 2006;8:395–400.
- Yang Q, Khoury MJ, Friedman J, Little J, Flanders WD. How many genes underlie the occurrence of common complex diseases in the population? Int J Epidemiol. 2005;34:1129–37.
- Lu Q, Elston RC. Using the optimal receiver operating characteristic curve to design a predictive genetic test, exemplified with type 2 diabetes. Am J Hum Genet.2008;82: 641–51.