Abstract
Objective: We attempt to explore the pathogenesis and specific genes with aberrant expression in diabetic nephropathy (DN). Methods: The gene expression profile of GSE1009 was downloaded from Gene Expression Omnibus database, including 3 normal function glomeruli and DN glomeruli from cadaveric donor kidneys. The differentially expressed genes (DEGs) were analyzed and the aberrant gene-related functions were predicted by informatics methods. The protein–protein interaction (PPI) networks for DEGs were constructed and the functional sub-network was screened. Results: A total of 416 DEGs were found to be differentially expressed in DN samples comparing with normal controls, including 404 up-regulated genes and 12 down-regulated genes. DEGs were involved in the process of combination to saccharides and the decline of tissue repairing ability of the organisms. The genes of VEGFA, ACTG1, HSP90AA1 had high degree in the PPI network. The main biological process of genes in the sub-network was related with cell proliferation and signal transmitting of cell membrane receptor. Conclusion: Significant nodes in PPI network provide new insights to understand the mechanism of DN. VEGFA, ACTG1 and HSP90AA1 may be the potential targets in the DN treatment.
Introduction
Diabetic nephropathy (DN), is the most common complication in patients with diabetes.Citation1 It affects 15–40% patients with type I diabetes.Citation2,Citation3 and the prevalence of DN in type 2 diabetes patients is around 5–20%Citation4 DN can result in renal failure, hypertension, arteriosclerosis and proteinuria. Thus, DN is one of the risk factors contributing to the high cardiovascular disease mortality.Citation5 DN is a progressive disease and can cause death within two or three years of lesion. DN is a major medical problem, but this disease is not well-controlled yet.
Previous evidence shows that good control of hyperglycemia and hypertension is the effective way to prevent the development of DN.Citation6 In the diabetic renal complications, hyperglycemia could induce the damage of glomerular and tubular locations at a cellular level. Hyperglycemia in DN patients may induce the increased flux through the polyol pathway, excessive formation of advanced glycation end-products, oxidative stress, and activation of the protein kinase C (PKC) pathway.Citation7 Besides, with the development of microarray technology, it provides a novel insight to understand the mechanism of DN. Previous microarray analysis of the diabetic glomeruli and control showed that the aberrant expression of genes was related with vascular damage, mesangial matrix expansion.Citation8 In addition, the tissue repair capability was found to be decreased in the progression of DN. Besides, miRNA-25, 29, 124 and 21 might be therapeutic target for DN.Citation9 However, the interactions of specific genes in the DN progression have not been clarified sufficiently.
In the current work, we downloaded the microarray data to perform bioinformatic analysis. The genes with aberrant expression were explored and the protein–protein interaction (PPI) network was constructed for these genes. We attempted to further explore the potential mechanism and key genes in DN progression.
Methods
Microarray data and preprocessing
The freely disseminated microarray data (accession ID: GSE1009) was downloaded from publicly available GEO repository, which were contributed by Baelde et al.Citation8 The dataset was developed from 3 pairs of normal function glomeruli and diabetic glomeruli samples from cadaveric donor kidneys.Citation8 DEGs were screened in DN samples compared with controls.
The raw data were normalized by Affy package in R language (version 1.44.0, Fred Hutchinson, Seattle, WA). The CEL data were converted to the expression measures matrix and the probe-level data were transferred to expression measures based on the platform of Affymetrix Human Genome U95 Version 2 Array (Affymetrix, Inc., Santa Clara, CA). If a single gene corresponds to multiple probes (many expression values), the average expression value was calculated for this specific gene.
DEGs screening and function analysis
DEGs were identified by using Limma (linear models for microarray data) package.Citation10 Only the genes with p ≤ 0.01 and |logFC| > 1 were selected as the DEGs for further analysis. GO (gene ontology) function and KEGG (Kyoto Encyclopedia of Genes and Genomes) pathway enrichment analysis were performed using DAVID (Database for Annotation, Visualization and Integrated Discovery) (Clinical services Program, SAIC-Frederick, Inc., Frederick, MD).Citation11 p-Value < 0.05 was considered as the cutoff value.
PPI network analysis
The PPI network is able to reveal the functions of proteins at the molecular level and help discover the rules of cellular activities including growth, development, metabolism, differentiation and apoptosis.Citation12,Citation13 Identification of protein interactions in a genome-wide scale is important for the interpretation of the cellular control mechanisms.Citation10 In this study, the protein pairs corresponding to DEGs were collected from STRING (Search Tool for the Retrieval of Interacting Genes) online datasets.Citation14 The PPI network was constructed by the Cytoscape software (National Institute of General Medical Sciences (NIGMS), Bethesda, MD).Citation15 Besides, sub-networks were screened and the node degrees were calculated using MCODE (Molecular Complex Detection) plugin.Citation16 Besides, the sub-network functions were analyzed with the function of MCODE plugin.
Results
Microarray data analysis between normal sample and DN samples
After pre-processing, expression values of 8657 genes in 6 glomeruli samples were obtained. The expression values of these genes were scaled to a standard level after data normalization (). Total 416 DEGs were screened out, including 404 up-regulated genes and 12 down-regulated genes in the glomerulus of DN patients (Supplementary material).
Figure 1. Box figure of expression value before (A) and after normalization (B) (the horizontal axis represents samples, while the vertical axis represents expression value). The black line in the box was the median of every group of data, which can tell the extent of normalization. As can be seen from the figure, the black lines were almost on the same straight line, indicating a high level of normalization.
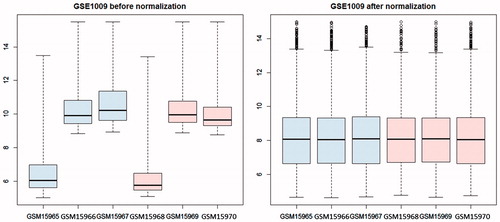
The results of GO function and pathway enrichment analysis for DEGs were shown in . The DEGs were enriched in 3 different GO categories, including biological process (BP), molecular function (MF) and cellar component (CC). The down-regulated genes were mainly enriched in response to toxin related BP, sugar binding related MF. The significant pathway involved with down-regulated DEGs was steroid hormone biosynthesis (hsa00140). Besides, the overrepresented GO terms of up-regulated genes contained intracellular signaling cascade (BP), regulation of cell proliferation (BP), intracellular non-membrane-bounded organelle (CC), non-membrane-bounded organelle (CC), cytoskeletal protein binding (MF) and calcium ion binding (MF). Furthermore, significantly enriched pathways included regulation of actin cytoskeleton (hsa04810), focal adhesion (hsa04510), and tight junction pathway (hsa04530).
Table 1. The GO terms which DEGs in PPI network were enriched in.
PPI network of DEGs
The PPI network was constructed by STRING online tool (). The sub-network 1 with nodes >10 in PPI network was selected (). Hub proteins with connective degree >10 were also selected, such as VEGFA, ACTG1, HSP90AA1, YWHAQ, VIM (). Among the hub nodes, VEGFA had the highest connective degree (degree = 27). Then, network 2 with VEGFA and its direct interaction proteins were constructed (). The function analysis showed that genes in sub-network 1 were mainly related with regulation of cell proliferation and cell surface receptor linked signal transduction. The main functions of genes in sub-network 2 were regulation of cell proliferation, positive regulation of cell proliferation ().
Figure 2. PPI network construction in diabetic nephropathy. The nodes stand for DEGs (differentially expressed genes) and the lines stand for the interactions between two proteins.
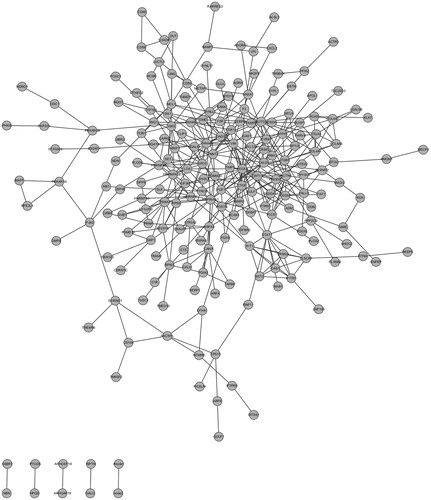
Figure 3. Subnetwork 1 was obtained by MCODE while subnetwork 2 was established based on the first nodes of hub proteins. The nodes stand for DEGs and the lines stand for the interactions between two proteins. (A) Subnetwork 1; (B) Subnetwork 2.
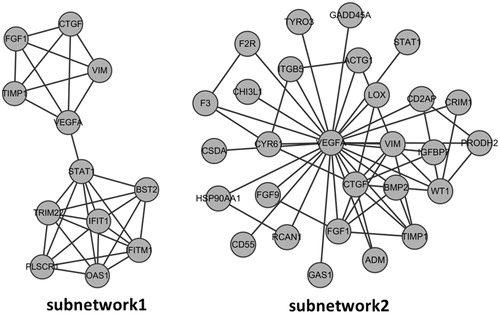
Table 2. A list of genes with connective degree than 10 in the PPI network.
Table 3. Main biological processes where DEGs in the sub-networks are involved.
Discussion
DN is a major cause of death in patients with diabetes mellitus.Citation17 A good understanding of DN pathogenesis is accessible to improving the prevention strategies. In the present study, we screened the genes with aberrant expression and explored the function and interrelationship of these genes. Bioinformatic analysis showed that 404 genes were overexpressed and 14 genes were down-regulated in diabetic glomeruli tissues. The number of up-regulated genes was higher than that of the down-regulated gene, which was corresponding to the originally published report.Citation8 The up-regulated genes were significantly enriched in intracellular signaling cascade, regulation of cell proliferation and regulation of apoptosis. So, the disordered cell activation is a common event in DN progression. The PPI network revealed that the genes of VEGFA (vascular endothelial growth factor A), ACTG1 (Actin, gamma 1), HSP90AA1 (Heat shock protein 90 kDa alpha (cytosolic), member A1) had high connective degrees with others, suggesting that these genes might play important roles in the occurrence and development of DN.
Among the hub nodes, VEGFA has the highest degree (degree = 27) and is a member of the vascular endothelial growth factor (VEGF) family. The endothelial dysfunction is a clinical hallmark in DN patients, which is characterized by increased endothelial permeability and proliferation.Citation18 Previous evidence suggests that the expression of VEGF is induced by hyperglycemia, hypoxia and increased blood pressure.Citation19,Citation20 VEGF level is found to be elevated in the early stage of DN in men and VEGF plays a predictable role in DN initiation,Citation21 which is in agreement with our findings that VEGFA was overexpressed in diabetic glomeruli by bioinformatic analysis.
Considerable research has focused on the critical role of VEGFA in kidney. VEGFA and epidermal growth factor (EGF) were decreased in mRNA level in DN, which was associated with a reduction in peritubular capillary densities.Citation1 The expression of VEGFA was inversely correlated with proteinuria level, but was positively related with hypoxia-inducible factor-1α in mRNA level.Citation1 Recently, it is found that VEGFA produced by tubular epithelial cells is critical in maintaining the peritubular capillary network in kidney.Citation22 VEGFA isoform VEGF-A165b is proved to have the vasculoprotective effects against DN.Citation23 Besides, sub-network 2 with VEGFA as the centre node was screened in our paper. Function analysis showed that sub-network 2 was closely related with cell proliferation regulation related biological process. It is in accordance with the recorded functions of VEGFA as mediator in endothelial cell growth, promoting cell migration, and inhibiting apoptosis. Therefore, VEGFA plays a key role in DN progression and good control of the VEGFA expression may be effective in DN prevention.
Gene ACTG-1 (degree = 18) is another hub node in PPI network. ACTG-1 encoding Actin, gamma 1 is a major component of cytoskeleton. According to AceView, ACTG-1 protein encoded by this gene are expected to have molecular functions (ATP binding, nucleotide binding) and localize in various compartments (cytoplasm, cytoskeleton, nucleus).Citation24 It is reported that ACTG-1 is expressed at very high level in DN and has been tested to be associated with various diseases, such as neoplasms, hearing loss and kidney disease.Citation25 In our work, ACTG-1 was found to be involved in pathways such as adherens junction, bacterial invasion of epithelial cells, dilated cardiomyopathy, which indicated the possible relations between ACTG-1 gene and the disease occurrence.
In addition, HSP90AA1 (degree = 17) is an inducible molecular chaperone,Citation26 which promotes the structural maintenance, maturation and proper regulation of specific target proteins involved in signal transduction and cell cycle controlCitation27 HSP90AA1 undergoes a functional cycle that is linked to its ATPase activity, which induces conformational changes in the client proteins. HSP90AA1 could interact dynamically with various co-chaperones to modulate its substrate recognition.Citation28 However, there is no report about the correlation between HSP90AA1 and DN. Further analysis of HSP90AA1 may provide new evidence for the molecular mechanism of DN.
In conclusion, the up-regulated genes play a major role in DN progression. Genes of VEGFA, ACTG1 and HSP90AA1 as significant nodes in PPI network are closely related with the molecular mechanism of DN. The significant nodes in PPI network provide new insights to understand the development of DN. VEGFA, ACTG1 and HSP90AA1 may be potential targets in the DN treatment. However, our findings are in urgent need of a large number of experimental validations in the future.
Supplementary Material available online
Supplementary Figures S1-S4.
Supplementary Table
Download MS Excel (50.9 KB)Declaration of interest
The authors report no conflicts of interest. The authors alone are responsible for the content and writing of the paper.
References
- Lindenmeyer MT, Kretzler M, Boucherot A, et al. Interstitial vascular rarefaction and reduced VEGF-A expression in human diabetic nephropathy. J Am Soc Nephrol. 2007;18:1765–1776
- Hovind P, Tarnow L, Rossing P, et al. Predictors for the development of microalbuminuria and macroalbuminuria in patients with type 1 diabetes: Inception cohort study. BMJ. 2004;328:1105
- Wong TY, Shankar A, Klein R, Klein BE. Retinal vessel diameters and the incidence of gross proteinuria and renal insufficiency in people with type 1 diabetes. Diabetes. 2004;53:179–184
- Adler AI, Stevens RJ, Manley SE, et al. Development and progression of nephropathy in type 2 diabetes: The United Kingdom Prospective Diabetes Study (UKPDS 64). Kidney Int. 2003;63:225–232
- Valmadrid CT, Klein R, Moss SE, Klein BE. The risk of cardiovascular disease mortality associated with microalbuminuria and gross proteinuria in persons with older-onset diabetes mellitus. Arch Inter Med. 2000;160:1093–1100
- Wolf G, Ziyadeh FN. Cellular and molecular mechanisms of proteinuria in diabetic nephropathy. Nephron Physiol. 2007;106:26–31
- Ohshiro Y, Takasu N. Molecular mechanism of diabetic nephropathy. Nihon Rinsho (Jpn J Clin Med). 2006;64:997--1003
- Baelde HJ, Eikmans M, Doran PP, et al. Gene expression profiling in glomeruli from human kidneys with diabetic nephropathy. Am J Kidney Dis. 2004;43:636–650
- Wu T, Li Q, Liu H. Identification of biological targets of therapeutic intervention for diabetic nephropathy with bioinformatics approach. Exp Clinic End Diab. 2014;122(10):587–591
- Smyth GK. Limma: Linear models for microarray data. In: Gentleman R, Carey VJ, Huber W, Irizarry RA, Dudoit S, eds. Bioinformatics and Computational Biology Solutions Using R and Bioconductor. New York: Springer; 2005:397–420
- Dennis Jr G, Sherman BT, Hosack DA, et al. DAVID: Database for annotation, visualization, and integrated discovery. Genome Biol. 2003;4:P3
- Xenarios I, Salwinski L, Duan XJ, et al. DIP, the Database of interacting proteins: A research tool for studying cellular networks of protein interactions. Nucleic Acids Res. 2002;30:303–305
- Fujita A, Sato J, Rodrigues L, Ferreira C, Sogayar M. Evaluating different methods of microarray data normalization. BMC Bioinformatics. 2006;7:469
- Von Mering C, Jensen LJ, Snel B, et al. STRING: Known and predicted protein–protein associations, integrated and transferred across organisms. Nucleic Acids Res. 2005;33:D433–D437
- Shannon P, Markiel A, Ozier O, et al. Cytoscape: A software environment for integrated models of biomolecular interaction networks. Genome Res. 2003;13:2498–2504
- Bader GD, Hogue CW. An automated method for finding molecular complexes in large protein interaction networks. BMC Bioinform. 2003;4:2
- John L, Kirubakaran MG, Shastry J. Diabetic nephropathy: A clinical study of 498 patients. J Diabet Complic. 1987;1:87–90
- Stehouwer CD, Lambert J, Donker A, van Hinsbergh VW. Endothelial dysfunction and pathogenesis of diabetic angiopathy. Cardiovas Res. 1997;34:55–68
- Williams B, Gallacher B, Patel H, Orme C. Glucose-induced protein kinase C activation regulates vascular permeability factor mRNA expression and peptide production by human vascular smooth muscle cells in vitro. Diabetes. 1997;46:1497–1503
- Takagi H, King GL, Ferrara N, Aiello LP. Hypoxia regulates vascular endothelial growth factor receptor KDR/Flk gene expression through adenosine A2 receptors in retinal capillary endothelial cells. Invest Ophth Vis Sci. 1996;37:1311–1321
- Hovind P, Tarnow L, Oestergaard PB, Parving H-H. Elevated vascular endothelial growth factor in type 1 diabetic patients with diabetic nephropathy. Kidney Int. 2000;57:S56–S61
- Dimke H, Sparks MA, Thomson BR, et al. Tubulovascular cross-talk by vascular endothelial growth factor A maintains peritubular microvasculature in kidney. J Am Soc Nephrol. 2015;26(5):1027--1038
- Oltean S, Qiu Y, Ferguson JK, et al. Vascular endothelial growth factor-A165b is protective and restores endothelial glycocalyx in diabetic nephropathy. J Am Soc Nephrol. 2014. [Epub ahead of print]. doi: 10.1681/ASN.2014040350
- Thierry-Mieg D, Thierry-Mieg J. AceView: A comprehensive cDNA-supported gene and transcripts. Genome Biol. 2006;7:S12
- Van Wijk E, Krieger E, Kemperman M, et al. A mutation in the gamma actin 1 (ACTG1) gene causes autosomal dominant hearing loss (DFNA20/26). J Med Genet. 2003;40:879–884
- Hendrick JP, Hartl F. Molecular chaperone functions of heat-shock proteins. Annu Rev Biochem. 1993;62:349–384
- Horwitz J. Alpha-crystallin can function as a molecular chaperone. Proc Natl Acad Sci USA. 1992;89:10449–10453
- Gano JJ, Simon JA. A proteomic investigation of ligand-dependent HSP90 complexes reveals CHORDC1 as a novel ADP-dependent HSP90-interacting protein. Mol Cell Proteomics. 2010;9:255–270