Abstract
The constantly evolving science of risk assessment is currently faced with many challenges, not only from the interpretation of the volume of data being generated with new innovative technologies, but also in attempting to quantitatively incorporate this information into understanding potential risk of adverse events in human populations. The objective of the case study described was to use the more recent data for di-(2-ethylhexyl)phthalate (DEHP) to investigate the impact of innovative quantitative approaches on the risk assessment of a compound, specifically as it can be used to move towards the new vision of risk assessment involving the integration of the available toxicological data to understand underlying biological processes. What emerged were several outcomes that demonstrated clearly the importance of the integration of the toxicological data, specifically to understand the biological processes being impacted, because standard statistical modeling approaches may not be adequate to describe the dose–response relationships observed. Alternative approaches demonstrate that a definitive mode of action is not needed to justify the shape of the low-dose region or a threshold, when the integration of the available data assist risk assessors in understanding the shape of the dose–response curve for both noncancer and cancer endpoints. Many of the challenges described as part of this case study would likely be encountered with compounds other than DEHP, especially other receptor-mediated compounds or compounds that “perturb” biological pathways, such as endocrine disruptors. This case study also highlights the importance of communication between risk assessors and the research community to focus on the generation of data most relevant for assessing the potential for chemicals to impact biological systems in the human.
Introduction
Since the first formal guidelines for risk assessment were developed (NAS, Citation1983; USEPA, Citation1986), many advances have been made not only in the process itself, but also in the types of toxicological data that are available to be considered as part of the process. The advancement of analytical techniques has resulted in a wealth of data on the pharmacokinetics of numerous compounds, as well as the impact of chemical exposure at the gene level. The need to consider these data in human health risk assessment, not only from a qualitative perspective but also quantitatively has resulted in the development of innovative approaches. These new approaches have mainly been incorporated into the current guidelines, while maintaining the basic steps in the conduct of a risk assessment as described in the “Red Book” (NAS, Citation1983). In the United States, toxicity assessments are continuing to evolve from a basically statistical treatment of cancer and noncancer data (i.e. selecting the endpoint that was statistically significantly increased leading to the selection of the endpoint that was presumed to be the most sensitive endpoint in the most sensitive species based on those statistical analyses), to an emphasis on consideration of the relevance of data to predict human health outcomes (NAS, Citation2009; USEPA, Citation2005). However, in general, these methods still rely upon the statistical evaluation of individual endpoints from individual studies.
While not explicitly characterized in the latest USEPA (Citation2005) cancer guidelines, these guidelines suggest an expansion of the data that should be considered as part of the risk assessment process. These types of recommendations have also been made in recent documents developed by the National Academy of Sciences (Ito et al., Citation2007; NAS, Citation2009). The intent of these documents is to outline a new vision of toxicity testing with a focus on the development of computational models combined with in vitro screens to potentially decrease animal testing. In addition, the NAS made recommendations on improving the technical analyses and utility of risk assessment. This involves expanding the use of scientific knowledge and information to improve risk assessment, as well as making risk assessment results more relevant and useful for risk management decisions.
The main challenge to the risk assessment community in attempting to address these recommendations is to develop novel qualitative and quantitative approaches that use and integrate the emerging toxicity data. The types of data that now need to be considered in the individual steps of the risk assessment process are not just the observation of adverse endpoints in animals or humans but now include:
understanding of the basic physiological processes in the animal model, i.e. mode of action, and how a chemical may be affecting those processes resulting in the adverse effect observed in the animal study; and an evaluation of the existence of the same biological processes in humans as part of the Hazard Assessment;
use of precursor data, genomic data, or other data indicative of the underlying biological processes based on the mode of action; the use of pharmacokinetic models (PBPK) for route and species extrapolation; and, the use of a suite of mathematical models and/or biological models based on the understanding of the mode of action for use in Dose Response Assessments;
use of biomonitoring data to refine Exposure Assessments; and,
use of probabilistic methods to address uncertainty and variability in the Risk Characterization.
The use of these types of data in risk assessment also needs to be expanded to not only characterize adverse effects, but to extend the use of these data to understand the potential impact to biological pathways in the low-dose regions that are most likely representative of chemical exposure in the general population. The development of formal qualitative frameworks to address the relevance to human of both cancer (Boobis et al., Citation2006; Cohen, Citation2004; Cohen et al., Citation2003; IPCS, Citation2005; Meek et al., Citation2003) and noncancer effects (Boobis et al., Citation2008; Seed et al., Citation2005) in animals have expanded the hazard assessment step, to not just focus on hazard potential, but also to integrate the available toxicological data to consider the potential biological perturbations and pharmacokinetics in evaluating differences between animals and humans.
The objective of this study was to use the database for di-(2-ethylhexyl)phthalate (DEHP) as a case study to investigate the impact of innovative quantitative approaches on the risk assessment of a compound. This case study is also an attempt to investigate how these innovative approaches can be used to move towards the new vision of risk assessment that involves the integration of the available toxicological data. It also provides an opportunity to investigate the types of innovative approaches that can be used to capture and characterize the impact of a chemical on biological pathways and the uncertainty involved in that characterization.
This investigation was never intended to include a comprehensive review of the toxicity of the chemical selected for the case study nor was it intended to conduct a human health risk assessment for the selected chemical. The case study was conducted to demonstrate the application of various quantitative approaches, both new and existing, to illustrate the use of these approaches not only in developing toxicity values but also in describing the types of data that are needed to successfully apply different approaches in a risk assessment. The use of newer tools, such as enhanced applications of PBPK models, probabilistic techniques (Bayesian and Monte Carlo), and the use of precursor and/or genomics analyses were explored to make use of more of the available data as part of the risk assessment process. The focus was not only to consider the application of innovative tools to quantify dose–response relationships for the types of endpoints observed in the animal studies, but also to investigate how to use those tools to reconcile seemingly different targets and time- and dose–response considerations within a gender, across genders, and across routes. Essentially, the focus was an attempt to apply new approaches to quantify kinetic and dynamic differences within and between species to investigate how these new approaches could improve the use of animal data in human health assessments.
The use of the database for DEHP as the focus of the case study also allowed the incorporation of innovative approaches into a risk assessment for a chemical whose prime mode of action likely involves the activation of one or more important physiological receptors. The question of whether or not the activation of single or multiple receptors may be involved in both the observed noncancer and cancer endpoints addresses the current attempts to harmonize the risk assessment of these endpoints. The resulting analyses also provided an opportunity to extend the existing Human Relevance Frameworks to address an integrated approach for compounds whose proposed modes of action for both noncancer and cancer effects may involve the same obligatory precursor step(s) (i.e. the activation of the peroxisome proliferator-activated receptor (PPAR) family of receptors). Noncancer and cancer information were analyzed both separately and collectively, evaluating the possibility for harmonization of both noncancer and cancer endpoints and, therefore, harmonization of the risk assessments. This case study also provided an opportunity to demonstrate the challenges encountered by risk assessors in evaluating complex information, especially with reproductive/development endpoints which provide unique issues to be considered.
The case study was conducted following the standard risk assessment paradigm that includes the hazard identification, dose–response modeling, exposure characterization, and risk characterization. This provided an opportunity to investigate various innovative quantitative approaches and techniques that could be applied in each step, as well as focus on critical decision points facing risk assessors.
Evaluating the available database for a chemical
The first step in conducting a risk assessment involves the critical review of the available database for a chemical to determine not only the potential for hazard, but also the potential modes of action by which the observed effects may occur. This review is critical, because the conclusions reached in this step of the risk assessment process can have a significant impact on whether or not quantitative analyses are conducted and the manner in which the dose–response assessment is conducted (i.e. linear versus nonlinear dose–response modeling).
The database available for DEHP is “data rich” and the available toxicity studies demonstrate potential human health concerns for both cancer and noncancer effects. The effects resulting from exposure to this compound has been well characterized and the potential modes of action for some endpoints have been hypothesized. The selection of a chemical with these criteria allows for the incorporation of advanced methods into the risk assessment paradigm without significantly increasing uncertainty.
Several extensive reviews are available for DEHP (ATSDR, Citation2002; Kavlock et al., Citation2006; Klaunig et al., Citation2003) that serve as a starting point for identifying those references that would likely provide the basis for a hazard assessment for DEHP, as well as provide the basis for conducting a dose–response assessment. Additional literature searches and review of recent literature (2002 to the present) was conducted not only to identify studies that may be used as the critical study in the dose–response assessment, but also to focus on those studies that provided the greatest number of opportunities for the demonstration of the use of innovative methods in risk assessment. A list of the studies and endpoints that were relied upon as part of this investigation are provided in and , with brief summaries of the results provided in the following sections.
Table 1. Selected noncancer studies for DEHP−reproductive/developmental effects considered for case study.
Table 2. Selected cancer studies for DEHP considered for case study.
Noncancer effects
The data suggest that reproductive/developmental effects following exposure to DEHP are the more sensitive of the noncancer effects (ATSDR, Citation2002; Kavlock et al., Citation2006). Several reproductive/development studies conducted with DEHP (Akingbemi et al., Citation2004; Akingbemi et al., Citation2001; Andrade et al., Citation2006c; Andrade et al., Citation2006b; Andrade et al., Citation2006a; Ge et al., Citation2007a; Grande et al., Citation2006; Grande et al., Citation2007; NTP, Citation2004) are available in the recent literature that allow for the comparison of effects across:
a wide range of doses, in particular in the low-dose range;
a wide range of exposure periods to include gestational, lactational and post-natal;
routes of exposure; and
generations using data provided in multigenerational studies.
These studies also provide a wide range of dose–response information for observational endpoints, as well as changes in events that could be key events in the biological cascade of events related to the mode of action for DEHP reproductive/developmental toxicity. These included for consideration changes in testosterone levels, or changes associated with the hypothesized mode of action, such as expressions of genomic/proteomic changes. Of particular interest were those data that could be used to conduct a comparison of the shape of the dose response curves for these reproductive/developmental effects. A brief discussion of these studies is provided.
Andrade and colleagues
Effects in male and female offspring of Wistar rats were evaluated following exposure in utero and during lactation to a wide range of concentrations of DEHP by Andrade and colleagues (Andrade et al., Citation2006c; Andrade et al., Citation2006b; Andrade et al., Citation2006a; Grande et al., Citation2006; Grande et al., Citation2007). Pregnant dams were administered DEHP by gavage from gestation day (GD) 6 to post-natal day (PND) 21 at doses of 0, 0.015, 0.45, 0.135, 0.405, or 1.215 mg/kg/day, termed the “low dose” range or at doses of 5, 15, 45, 135, or 405 mg/kg/day termed the “high dose” range resulting in indirect exposure to the fetus in utero and to the pups during lactation. Pups were not dosed following weaning, and were sacrificed at various times after delivery. Overall, no effect of DEHP administration on the production of the F1 generation was reported in these studies. In addition, no effect was reported on any index of reproductive function (e.g. litter sizes, implantation sites, post-implantation loss, pup birth weight, viability index).
Andrade et al., (Citation2006a) reported the effect of DEHP on sexual development of male offspring (). Effects indicative of changes in androgenic functioning were the more sensitive endpoints and included a significant delay in the onset of puberty (preputial separation) at doses of 15 mg/kg/day and higher. When measured on PND 22, testes weight was significantly increased at 5, 15, 45, and 135 mg/kg/day but not in the highest dose group, 405 mg/kg/day. However, the changes in testes weight did not increase monotonically with dose over an 80-fold increase in DEHP dose, when based on the amount administered to the dam. Other indications of anti-androgenic effects, including nipple retention and reduced anogenital distance (AGD), were reported only in the highest dose group (405 mg/kg/day). A significant increase in AGD was also reported in the lowest dose group, which the authors indicated is probably unrelated to treatment as no similar changes were observed at any other low doses tested. However, they also noted that they cannot rule out the possibility that this effect occurs at even lower doses that those currently evaluated. There was no effect reported on the age of testis descent at any DEHP dose given, another endpoint influenced by testosterone levels (see Section 2.4.1.1.1 for a discussion). Histopathological changes in the testes of newborn and weanling rats indicative of abnormal Sertoli cell function and disruption of Sertoli-germ cells interaction were noted in the two highest dose groups. On PND 1, the severity of bi- and multinucleated (enlarged) gonocytes were increased in the two highest dose groups; and on PND 22, there was an increase in severity of signs of reduced germ cell differentiation. However, there was no increase in epididymis weight or in the diameter of seminiferous tubules when measured on PND 22. While not significantly different from control, the change in diameter of seminiferous tubules followed the same pattern as that observed for testes weight.
Table 3. Effects on androgenic status, developmental landmarks and testicular histology in male offspring rats reported by Andrade et al. (Citation2006a).
Grande et al., (Citation2006) reported on the effects of DEHP on female Wistar rat reproductive development following the same protocol described above. Comparable to the delay in preputial opening in male offspring, there was a significant delay in the mean age at vaginal opening (~2 days) in the 15 mg/kg/day dose group and higher (). These data were reported as categorical data that is the number of animals with time to vaginal opening of >37 day, 35 to 37 days, and 33 to 34 days. A definite trend toward an increase in the number of animals in the >37 day category was reported, but no clear dose–response trend was apparent. There was also a trend for a delay (~2 days) of the age at first estrus in the two highest dose groups. These two endpoints are dependent on increasing levels of estradiol during puberty and a delay suggests antiestrogenic or androgenic activity for DEHP.
Table 4. Effects on female rat reproductive development following administration of DEHP reported by Grande et al. (Citation2006).
Andrade et al., (Citation2006b) investigated the possible long-term effects of developmental DEHP exposure on male reproductive tract structure and function. The focus of the study was on the potential effects on reproductive organ weights, testicular function, hormonal status, and sexual behavior and fertility. Selected males exposed both in utero and via lactation were followed for up to PND 144 without any direct DEHP exposure (Andrade et al., Citation2006b). Evidence of an impact on testicular function was observed at PND 144 (), but no effects on fertility or sexual behavior was seen at any dose. Specifically, there was no effect on testis, epididymis, or prostate weight, but seminal vesicle weight was significantly decreased in the high dose group. Serum testosterone concentrations fluctuated over the entire dose-range and were significantly increased in 0.045, 0.405, and 405 mg/kg/day groups only without any dose-related pattern. While the testosterone levels in the high dose group were approximately twice that in the control group, the increase across the other dose groups was non-monotonic and not proportional to the DEHP dose. According to the authors, the daily sperm production for the concurrent control was significantly higher than the historical rate for this strain in this laboratory. When compared to the historical control rates, statistical significance was only reached in males in the 1.215 mg/kg/day dosed group and again did not decrease in a dose-related manner. No effects on testicular morphometry indicative of alterations in Sertoli function were reported. Further, reproductive tract malformations were infrequently seen and spread across dose groups. Despite these changes, DEHP exposure did not produce changes in time to mating, or in mating and pregnancy indices (). No effects were observed on litter size, fetal weight, and the number of implantation sites, resorptions, and viable fetuses or any other indices of reproductive function or sexual behavior.
Table 5. Reproductive effects reported by Andrade et al. (Citation2006b) adult male rat offspring following administration of DEHP.
Similarly, to the evaluation of reproductive function in male offspring, reproductive effects in adult female offspring exposed to DEHP in utero and via lactation were reported by Grande et al., (Citation2007). No effects on reproductive organ weights, estrous cyclicity, concentrations of serum estradiol or progesterone, morphometric changes in the uterus or vagina luminal epithelial cell height were seen in any dose group. The only change was an increase in the number of ovarian tertiary follicles undergoing atresia seen in females in the high dose group ().
Table 6. Reproductive effects reported adult female offspring rats following administration of DEHP (Grande et al.,2007).
Andrade et al., (Citation2006c) investigated the effect of DEHP on estrogen metabolism that in addition to its anti-androgenic effects may be mediated through suppression of aromatase enzyme activity. In groups of male and female offspring of dams treated as noted above, levels of aromatase in the hypothalamic/preoptic area (HPOA) of the brain were measured on PND 1 and 22. Sex and age-related differences in HPOA aromatase activity compared to control rats were seen (). An interesting biphasic response was seen in males with low dose inhibition and high dose stimulation. On PND 1, aromatase activity in the HPOA showed a trend toward decreased aromatase activity that was significantly decreased in the 0.135 and 0.405 dose groups compared to male controls. A trend toward greater activity began in the 1.215 dose group that was statistically significantly increased at 15, 45, and 405 mg/kg/day. Again, however, the increase was non-monotonic and unrelated to dose. No significant changes compared to control were seen in females evaluated on PND1. On PND 22, brain aromatase activity was not elevated in males but was significantly different from controls in treated female offspring at doses beginning at 0.015 mg/kg/day. Again, no dose–response patterns were seen and the activity was not significantly increased in all dose groups. No consistent dose-related increases in aromatase activity were seen. According to the authors, the effects noted in both males (PND1) and females (PND 22) corresponded to time points when testes and ovaries were functionally active or initiating activity, respectively.
Table 7. Effects on rat brain aromatase activity following gavage administration of DEHP (Andrade et al.,2006c).
Akingbemi and colleagues
Another group of scientists studied the effects of DEHP on Leydig cell androgen and estradiol biosynthesis in male rats exposed at various gestational, lactational, and postnatal time points (Akingbemi et al., Citation2004; Akingbemi et al., Citation2001; Ge et al., Citation2007a). Akingbemi et al., (Citation2004) and Ge et al., (Citation2007a) administered prepubertal male rats with doses ranging from 10 to 750 mg/kg/day for various time intervals from PND 21 to 120.
In Akingbemi et al., (Citation2001), the potential effects of DEHP exposure on male reproductive development and function was evaluated. For prenatal exposure, pregnant (GD 12 to 21) and lactating (PND 1 to 21) Long Evans female rats received 0 or 100 mg/kg/day DEHP by gavage and male offspring were evaluated on PNDs 21, 35 and 90. For prepubertal exposure, male rats received 0, 1, 10, 100 or 200 mg/kg/day on PNDs 21 to 34, 35 to 48, or 21 to 48 also by gavage. Separate groups of young adult male rats were also exposed to DEHP by gavage at the same doses in the 1 to 200 mg/kg/day range from PND 62–89. Following in utero exposure, statistically significant decreases in serum testosterone and leutenizing hormone (LH) concentrations were reported in males exposed to DEHP at 100 mg/kg/day when measured on PNDs 21 and 35, but not at PND 90 (). No changes in serum testosterone or LH concentrations were reported in male offspring exposed only during lactation (PNDs 1 to 21) or at any time period postnatally (PNDs 21, 35, or 90). These results suggest that DEHP modulates Leydig cell function and steroidogenesis by both dose and the stage of development when treatment occurred. The data indicated that DEHP altered levels of enzymes involved in testosterone biosynthesis and levels of serum LH.
Table 8. Changes in serum testosterone and LH levels following gavage administration of DEHP (Akingbemi et al.,2001).
In male rats exposed postnatally, there were no decreases in serum testosterone or LH levels or in testis and seminal vesicle weights (). There was a significant decrease in Leydig cell testosterone production and LH-stimulated testosterone production at 100 mg/kg/day and higher when dosed on PNDs 21 to 34 and at 10 mg/kg/day and higher when dosed on PND 35 to 48. Measurement of steroidogenic enzyme activity following postnatal exposure of rats from PND 35 to 48 showed a significant decrease in 17-β hydroxysteroid dehydrogenase (17B-HSD) at 10 mg/kg/day or greater, decreased P450 cholesterol side-chain cleavage enzyme and 3β-HSD at 100 mg/kg/day or greater and decreased Cytochrome P450 17α-hydroxylase at 200 mg/kg/day. In males dosed for 28 days, a dose-dependent increase in serum concentration of LH and testosterone and testosterone levels in the testicular interstitial fluid at doses of 10 mg/kg/day and higher were seen. Treatment of young adult male rats (PND 62 to 89) did not produce similar changes. Histological evaluation of the testis presented no evidence of Leydig cell hyperplasia or other histopathological alterations in any group tested.
Table 9. Change in reproductive parameters reported in male rats following gavage administration of DEHP (Akingbemi et al.,2001).
Akingbemi et al., (Citation2004) investigated further the effects of postnatal exposure of DEHP on testicular function. Male Long Evans rats were administered 0, 10, or 100 mg/kg/day DEHP starting on PND 21 and continuing until PND 48, 90, or 120. Treatment of male rats (0, 10, or 100 mg/g/day) from PND 21 to 90 resulted in significant increases in serum LH and testosterone levels, and an increase in Leydig cell proliferation at both dose levels (). Evidence of Leydig cell proliferation was indicated by significant increases in mRNA for PCNA, cyclin D3, p53, and cyclin G1 (PNDs 21 to 90) and thymidine incorporation by Leydig cells (PNDs 21–120). Significant decreases in basal Leydig cell testosterone production and LH-stimulated testosterone production were also reported at both doses tested on PNDs 21 to 90. Similar patterns of increases and decreases in the same endpoints were seen in male rats treated from PND 21 to 120; however, significant changes were only reported in the high dose group, 100 mg/kg/day.
Table 10. Changes in Reproductive Parameters reported by Akingbemi et al. (Citation2004) in male rats following gavage exposure to DEHP.
When treatment with DEHP (0, 10, or 100 mg/kg/day by gavage) occurred on PNDs 21 to 48, serum estradiol levels and LH-stimulated Leydig cell estradiol levels were significantly elevated in both dose groups, while mRNA for aromatase and Basal Leydig cell estradiol production were significantly elevated in the males in the high dose group only. The authors concluded that the increased serum estradiol levels were the result of enhanced estradiol biosynthesis as indicated by the elevated levels of basal and LH-stimulated estradiol production by Leydig cells and the increases in aromatase activity in the Leydig cells. However, by PND 90, serum estradiol levels were comparable to controls, perhaps reflecting the increased numbers of Leydig cells in treated rats, as mentioned previously.
Ge et al., (Citation2007a) extended these investigations to assess the timing of puberty, as indicated by the time to preputial separation and reported a dose-dependent biphasic effect. In male rats administered DEHP by gavage at doses of 0, 10, 500 or 750 mg/kg/day from PND 21 to 49, an advanced onset of puberty (based on day of preputial separation) which was accompanied by significant increases in seminal vesicle weight and elevated serum testosterone levels in the low and high dose groups (10 or 750 mg/kg/day), with no significant changes reported in the mid-dose group of 500 mg/kg/day (). At the high dose of 750 mg/kg/day, the preputial separation was delayed, rather than advanced, and there were significant decreases reported in testicular weight, prostate weight, and serum testosterone. Ge et al., (Citation2007a) suggested that the low doses of DEHP induced serum testosterone levels and advanced the onset of puberty in male rats, while higher doses had the opposite effect.
Table 11. Biphasic effects of reported in male rats following postnatal exposure to DEHP for 28 days (PND 21 to 48)+ (Ge et al.,2007a).
National Toxicology Program (NTP)
The NTP conducted a complex three generation continuous breeding study in Sprague–Dawley rats (NTP, Citation2004). The F0 generation consisted of a control group (1.5 ppm - background in the diet) and seven treatment groups ranging from 10 to 10,000 ppm in the diet. Animals from the F0 generation were bred to produce the F1 generation, the F1 adults bred to produce the F2 generation, and the F2 adults bred to produce the F3 generation. Based on measured feed consumption, mg/kg daily doses were estimated to be 0.12, 0.78, 2.4, 7.9, 23, 77, 592, and 775 mg/kg/day in the F0 generation, 0.09, 0.48, 1.4, 1.9, 14, 48, 391, and 543 mg/kg/day in the F1 generation, and 0.1, 0.47, 1.4, 48, 14, 46, and 359 mg/kg/day in the F2 generation. The high concentration animals (10,000 ppm) only completed the F1 generation and were sacrificed because of the inability to produce an F2 generation.
Following 1 week of premating exposure to DEHP, animals were cohabitated for 9 weeks. The first two litters produced during the cohabitation period by the F0 generation (F1a, F1b) were counted, weighed and anogenital distance measured on PND 1, then sacrificed. The third litter born (F1c) was reared without culling until weaning on PND 21. On PND 1, 4, 7, 14, and 21, the number of pups was counted, each pup weighed and AGD (PND 1 only) measured. Litters were counted and weighed on PND 1, 4, and 21, with pups sacrificed in PND 21. On PND 12 and 13, male pups were examined for retained nipples. On PND 16, five males and two female offspring were randomly selected from each litter. For the females, one was selected for necropsy on PND 60–74 and the other was selected for cohabitation to produce the next generation. For the males, one was assigned for cohabitation, three were selected to be maintained until ~2 weeks prior to necropsy of the mating males for evaluation of testicular descent and preputial separation, and one was selected for necropsy on PND 63–64. The males and females necropsied on PND 60–74 had sexual development parameters measured and were then sacrificed.
Methods used for the mating of the F1 generation and the examination of the offspring (F2a, F2b, F2c) were similar, with the exception of on PND 16, up to 22 male pups were selected for the F2 mating trial from each dose group. Of these, 17 were assigned to cohabitation, with 3 males per litter per group selected for non-mating reproductive evaluation (testicular descent and preputial separation). Up to two females from each litter were selected to comprise the 17 that were assigned to the F2 mating trial. For the F2 generation, methods similar to those used for the F0 generation were applied, with no pups retained for eventual mating.
The authors reported that effects on reproduction, organ weight, and body weight were mainly noted in the 7500 and 10,000 ppm groups (). In the F1 generation, when all litters were combined, significant decreases in the number of live males per litter and total live pups per litter were noted in the 7500 ppm group only and decreased proportion of pups born alive and live pup weights (male, female and combined) were noted in the 10,000 ppm group only. It is important to note that dam food consumption during lactation was decreased in these two dose groups and may contribute to the decreased pup weight observed.
Table 12. Summary of results from the multigenerational reproductive assessment by continuous breeding conducted by NTP (Citation2004) in Sprague-Dawley rats in which DEHP was administered in the diet (NTP Citation2004).
In the F2 generation, when all litters were combined, decreases in live pup weights (adjusted for litter size for male, female and both) were reported in the 7500 ppm groups (no offspring produced by the 10,000 ppm group in the F1 generation). In the F3 generation, when all litters were combined, the only significant effect reported was a decrease in the average litters per pair.
In evaluating sexual development in the offspring, one unusual effect was observed in the F2 males (). A statistically significant delay in the day of preputial separation was noted in all dose groups tested and a significant delay in the day of testicular descent was noted in males administered 30 ppm or greater. Significant delays in these endpoints were only observed in the 7500 ppm groups of the F1 and F3 generations. No explanations for these observations were provided by the authors.
Table 13. Summary of sexual development data from NTP (Citation2004).
Noncancer studies by the inhalation route of exposure
Inhalation studies that could be considered for the estimation of a POD were limited to two studies conducted by a single group of investigators (Kurahashi et al., Citation2005; Ma et al., Citation2006). In these two studies, the protocols were similar, with one study focused on prepubertal effects in male rats (Kurahashi et al., Citation2005) and the other focused on effects in female rats (Ma et al., Citation2006). The concentrations administered were the same in both studies (0, 5, or 25 mg/m3), with time of exposure being similar as well. In the male studies, exposure was initiated at 28 days of age and continued for either 4 (PND 56) or 8 weeks (PND 84). In the females, exposure was initiated at 22 days of age and continued until postnatal day 41 (Experiment 2) or 84 (Experiment 1). The objective of each study was not only to investigate the effects of DEHP inhalation in prepubertal animals, but also whether there is a difference between routes of exposure.
In male rats, significant dose-related increases in seminal vesicle weight and plasma testosterone were observed only following 8 weeks of exposure (Kurahashi et al., Citation2005) (). This is consistent with changes in testosterone observed following oral exposure to DEHP in male rats from PND 21 to 90 (Akingbemi et al., Citation2004). No other dose-related changes were reported. In the female rats, a significant decrease in the age of vaginal opening and age of first estrous cycle were reported at both concentrations (Ma et al., Citation2006) (). A significant increase in the number of females with irregular estrous cyclicity was also observed in females receiving 25 mg/m3 on PND 49 to 84. Increases in estradiol and LH were noted in female rats at the high dose at PND 42 (Experiment 2). In addition, significantly increased cholesterol levels were noted at both doses on PND 42 (Experiment 2); however, the levels were significantly decreased at both doses on PND 84 (Experiment 1). Levels of aromatase, a rate-limiting enzyme responsible for the conversion of testosterone to estradiol, was elevated at the high dose on PND 20. In conclusion, the results reported in the female rats indicate that inhaled DEHP advanced the onset of puberty and altered post pubertal reproductive functions (Ma et al., Citation2006).
Table 14. The effects of subacute inhalation of DEHP on selected endpoints in prepubertal Wistar rats (Kurahashi et al., 2005).
Table 15. Reproductive effects reported in prepubertal female rats following inhalation exposure to DEHP (Ma et al.,2006).
Cancer effects
Five chronic oral studies in animals have been identified that evaluated the carcinogenic effects of DEHP in rats and mice (David et al., Citation1999; David et al., Citation2000a; David et al., Citation2000b; NTP, Citation1982; Voss et al., Citation2005). Voss et al., (Citation2005) fed male and female Sprague-Dawley rats a diet containing DEHP at concentrations of 0, 30, 95, or 300 mg/kg/day for 159 weeks. Statistically significant increases in tumors were reported in the liver and testes of sacrificed animals in the high dose group (). For the liver, the incidence of neoplasms evaluated included all benign and malignant neoplasia. In addition to being significant compared to control incidence, there was a significant positive dose-related trend over all three dose groups for all hepatocellular neoplasia. Significant increases in the incidence of Leydig cell tumors were also observed in the high-dose group, with significant dose-related trends also reported. The authors conducted time-to-tumor analyses and results indicated that the Leydig cell tumors occurred with a significantly higher incidence in the second time period (750–950 days), which was earlier than the period in which most of the hepatocellular tumors were reported. Although the authors did not report it as statistically significantly increased, the reported incidence of pancreatic acinar cell adenomas in the high-dose group was significantly increased compared to controls (p < 0.001).
Table 16. Incidence of tumors reported in male Sprague-Dawley rats following lifetime dietary administration of DEHP (Voss et al.,2005).
David et al., (Citation1999; Citation2000a; Citation2000b) studied the effects of chronic dietary exposure of DEHP in male and female B6C3F1 mice and Fischer 344 rats. In these studies, mice were fed a diet containing DEHP resulting in doses of 0, 30, 95, or 300 mg/kg/day for 159 weeks (David et al., Citation1999; Citation2000b), while rats received concentrations of 0, 100, 500, 2500, or 12,500 ppm for 104 weeks (David et al., Citation2000a; Citation2000b). Additional groups of rats and mice received the highest concentrations for 78 weeks and then the control diet for an additional 26 weeks (recovery group). Animals were sacrificed at 79 and 105 weeks for histopathological examination. Results indicated statistically significant increases in the incidence of hepatocellular adenomas and carcinomas in male mice at concentrations of 500 ppm and higher and in female mice at concentrations of 1500 ppm and higher (). In rats, statistically significant increases in the incidence of total hepatocellular tumors were observed in males at 2500 and 12,500 ppm and in females at 12500 ppm (). Statistically significant increases in the incidence of pancreatic acinar cell adenomas were also reported in male rats at dietary concentrations 12,500 ppm ().
Table 17. Incidences of hepatocellular neoplasms in fischer 334 rats following lifetime administration of DEHP (David et al.,1999).
Table 18. Incidences of histopathological lesions reported in male fischer 334 rats administered DEHP in the diet (David et al.,2000a).
Finally, NTP (NTP, Citation1982) examined the effects of DEHP in both male and female rats and mice. The mice were fed diets containing 0, 3000, or 6000 ppm DEHP and the rats were fed diets containing 0, 6000 or 12,000 ppm DEHP for 103 weeks. In female rats there were significant increases in the incidence of hepatocellular carcinoma and hepatocellular neoplastic nodules in the high dose group (). When the incidence of carcinomas and neoplastic nodules was combined it was significant in both the low and high dose groups of female rats and in the high dose group of male rats. In male and female mice, the incidence of hepatocellular carcinoma was significantly increased in the high dose group and the combined incidence of hepatocellular carcinoma or adenoma was significantly increased in both dose groups.
Table 19. Incidence of tumors reported in male and female rats following administration of DEHP (NTP Citation1982).
Toxicokinetics
DEHP is one of a class of phthalate esters that consist of paired ester groups on a cyclohexatriene ring (benzene-dicarboxylic acid) (Kluwe, Citation1982). This class of compounds is synthesized commercially by condensation of appropriate alcohols with phthalic anhydride. Members of this class with short alkyl groups, such as di-n-butyl phthalate (DBP), are relatively soluble in water, while other compounds, such as DEHP, are relatively insoluble in water, due to their lipophilic structures.
Humans can be exposed to DEHP by all routes of exposure, including intravenously through medical practices such as in dialysis or blood transfusions where the source of DEHP is the plastics used in medical treatment devices or storage bags. The focus of this review, however, is on those animal studies conducted by the oral and inhalation routes of exposure. A brief discussion of the pharmacokinetics of DEHP following exposure by these two routes is provided.
Absorption
In humans, measurement of DEHP metabolites in the urine indicates absorption by both the oral and inhalation routes of exposure (ATSDR, Citation2002). Following inhalation exposure, no quantitative estimate of the amount absorbed in humans or animals is available; however, identification of DEHP or its metabolites in the urine or in tissue samples indicate that absorption has occurred. In addition, due to the lipophilic nature of DEHP, it would be expected to be readily absorbed from the lungs into the circulation (Kluwe, Citation1982). Based on urinary excretion of metabolites, rats absorb greater amounts of DEHP than humans, with absorption of greater than 90% of the amount found in foods with DEHP concentrations ranging from 10 to 2000 ppm (Kluwe, Citation1982).
Results from animal studies suggest that at low exposure concentrations, most of the ingested DEHP is hydrolyzed in the small intestines and absorbed as mono(2-ethylhexyl)phthalate (MEHP) or 2-ethylhexanol (Albro, Citation1986; Albro et al., Citation1982). Larger percentages of smaller doses are expected to be absorbed, as intestinal transport of MEHP and DEHP can be saturated at high doses (Short et al., Citation1987).
Distribution
DEHP, whether administered orally or parenterally, is rapidly cleared from the body within 1–5 days (Kluwe, Citation1982). There is little or no evidence of tissue accumulation or prolonged tissue retention. Because it is lipophilic, if accumulation occurs, DEHP or its metabolites will be present in the adipose tissue, absorptive organs (i.e. gastrointestinal tract) and excretory organs (i.e. liver, kidney and gastrointestinal tract) (ATSDR, Citation2002; Kluwe, Citation1982). Tissue concentrations of DEHP from accident victims ranged from 0.3 to 1.0 ppm in adipose tissue (Mes et al., Citation1974). DEHP has also been isolated in the kidneys of autopsied patients (Overturf et al., Citation1979). However, since DEHP can easily contaminate biological samples during laboratory processing operations, the presence of DEHP in tissues may be an artifact.
Biomonitoring studies have measured concentrations of DEHP or its metabolites in the urine, blood and breast milk of humans, such as the studies conducted by the National Health and Nutrition Examination Surveys (NHANES) conducted by the Centers for Disease Control (CDC). The results of these biomonitoring studies will be discussed further below (Biomonitoring/Exposure Assessment).
Metabolism
The metabolism of DEHP in animals and humans consists of a complex series of reactions involving 30 or more metabolites (ATSDR, Citation2002). The first step of the process occurs rapidly in the intestines and involves the hydrolytic cleavage of DEHP to form MEHP and 2-ethylhexanol. This reaction occurs through interaction with lipases that are most abundant in the pancreas and intestinal mucosa, but also found in other organs, such as the liver, kidneys, lungs, skin and plasma (Albro Citation1986). Due to the differences in tissue enzyme activities, the amount of DEHP converted to MEHP and 2-ethylhexanol following oral exposure is greater than when DEHP is absorbed following inhalation or dermal exposure. Once converted to MEHP and 2-ethylhexanol, MEHP can be conjugated with glucuronic acid for excretion (ATSDR, Citation2002) or metabolized through oxidative pathways (Cytochromes P450). 2-Ethylhexanol is also metabolized through oxidative pathways and excreted in the urine as 2-ethylhexanoic acid and keto acid derivatives following β-oxidation.
In studies in humans exposed to DEHP via the oral route, 8 metabolites were identified in the urine with MEHP making up 6 to 12% of the metabolites (ATSDR, Citation2002). When hydrolyzed urine was compared to unhydrolyzed urine, 65% of the metabolites were glucuronide conjugates each being a product of oxidation of different carbons in the 2-ethylhexyl substituent. In humans exposed intravenously, DEHP was converted to MEHP with levels of DEHP being higher initially, followed by a rapid decline in DEHP and an increase in MEHP levels until the level eventually became almost equal (CALEPA, Citation1997). The urinary metabolites identified in humans are similar to those seen in laboratory animals; however, relative proportions differ by species, dose, and time (ATSDR, Citation2002).
No data are available that characterize metabolites of DEHP following inhalation (ATSDR, Citation2002). It can be assumed that the metabolic pathway will be similar to that following oral exposure. Lipases are present in the lungs and epidermis (Albro Citation1986). However, conversion of DEHP to MEHP and 2-ethylhexanol will likely occur at a slower rate because the activities of these lipases are about 0.25% of that in the pancreas (Albro Citation1986). Ng et al., (Citation1992) provided data on DEHP metabolism after dermal application, suggesting that ~70% was absorbed based on measurement of MEHP.
Excretion
DEHP and its metabolites are primarily eliminated in the urine and feces in both animals and humans (ATSDR, Citation2002; CALEPA, Citation1997; Kluwe, Citation1982). Fecal elimination of DEHP following oral absorption may be supplemented by a biliary contribution, with biliary excretion rates in animals ranging from 5 to 20%, which could confound total absorption estimates (CALEPA, Citation1997). DEHP metabolites are excreted in bile to an unknown extent, reabsorbed in the intestine, and then eliminated in the urine (Kluwe, Citation1982).
In humans receiving a single oral dose of 30 mg of DEHP, 11 to 15% of the dose was excreted as metabolites in the urine within 48 h (ATSDR, Citation2002). Rats excreted 32 to 70% of the orally administered dose of DEHP (50–300 mg/kg) in the urine as metabolites, and 20 to 25% of the absorbed dose was excreted with the bile in the fecal matter. Approximately 85 to 90% of the radiolabeled DEHP administered orally to rats and mice was excreted in the first 24 h following exposure. Excretion rates in the first 24 h following exposure were lower in dogs (67%), monkeys (50–80%), and miniature pigs (37%).
Proposed Modes of Actions for Effects Observed
When evaluated, the mode of action (MOA) for cancer and noncancer effects, typically, is considered separately. It has long been assumed that, more often than not, these adverse effects result from distinct and independent biological events affecting different cellular processes. In this review, MOA(s) that have been proposed for DEHP for both the production of tumors and reproductive/developmental toxicity were considered to determine if these effects may have an initiating key event(s) or an obligatory precursor event in common.
The intent of this review was not to put forth a compelling, definitive hypothesis for the molecular or mechanism of action of DEHP in the production of both cancer and noncancer effects. Rather, the intent was to test a framework for the evaluation of a chemical, in particular the dose–response evaluation, when either the obligatory precursor step or the penultimate step for cancer and noncancer effects is hypothesized to be the same. For example in male rats, the production of Leydig cell tumors in aging male rats and the developmental effects in male rats exposed during the perinatal period (in utero and lactational) could both be due to the reduction in testosterone levels when DEHP is administered in critical windows of physiological development, i.e. the aging rat with regard to testicular tumors or perinatal exposure of male rats at critical times in testosterone production and sexual development. Stated differently, there may be multiple pathways, either as a result of the same initiating key event, such as receptor-mediated interactions with resulting biological cascades of events, or to different critical initiating key events that result in the same physiological change, such as a decrease in testosterone. Such a change could be considered the common denominator and the necessary biological change (the obligatory precursor event) for induction of both tumors and the observed reproductive/developmental effects, even if the initiating key event(s) was different.
This review was intended to identify potential obligatory precursor steps that could be used in refining a PBPK model and/or in dose–response modeling, either by applying these precursor data into the quantitative assessment or, in the absence of specific quantitative data, exploring the application of chemical-specific adjustment factors or other approaches. Any evaluation of the MOA(s) for these effects should consider:
The basic biology of that organ system along with physiological controls, such as feedback loops, that explains normal functioning;
The key steps in that biological/physiological flow of normal functioning that could be impacted by either changes due to aging that are exacerbated by chemical exposure resulting in changes in that cell or organ system’s homeostasis; and,
The key step or obligatory precursor event that presumably provides the underlying stimulus to “push” a normally functioning organ or cell to an adverse change, thereby, producing the observed adverse effect.
This review of DEHP MOA focused on the last consideration—the key step and/or obligatory precursor events(s) that may be associated with the reproductive/developmental effects and tumors of the liver, Leydig cells and pancreas discussed in previous sections. The proposed MOA of DEHP in the production of cancer in rodents has been generally accepted as PPARα-mediated. The MOA(s) for the reproductive/developmental effects in male and female rodents are not as well-characterized as that for the cancer endpoints but it has been suggested that underlying processes involve PPARr-mediation (indicating that one or more PPAR subtypes may be involved). While the proposed receptor-mediated hypotheses for reproductive/developmental effects are not limited to PPARr activation, there is evidence that some if not all of the observed reproductive/developmental responses may be mediated by members of the PPAR family of receptors (i.e. PPARα, PPARγ, or PPARβ).
Proposed mode(s) of action for noncancer effects
The effects of DEHP on indicators of reproductive/developmental toxicity in male and female rats given DEHP at different ages from gestation to young adults, at different doses ranging from 0.015 mg/kg/day to 750 mg/kg/day or higher and by different routes of exposure, i.e. oral (gavage and diet) and inhalation were discussed in previous sections (Akingbemi et al., Citation2004; Akingbemi et al., Citation2001; Andrade et al., Citation2006c; Andrade et al., Citation2006b; Andrade et al., Citation2006a; Ge et al., Citation2007a; Grande et al., Citation2006; Grande et al., Citation2007; Kurahashi et al., Citation2005; Ma et al., Citation2006; NTP, Citation2004). A complete review of reproductive /development effects in humans and animals from exposure to DEHP was conducted by the NTP-Center for the Evaluation of Risks to Human Reproduction Expert Panel (Kavlock et al., Citation2006). Collectively, the key findings of these studies in male offspring dosed perinatally included those related to: 1) an antiandrogenic effect manifested as delays in preputial separation (onset of puberty), increased testis weight, nipple retention, reduced AGD, decreased testicular testosterone production, and undescended testes (Cryptorchidism); and, 2) changes indicative of abnormal Sertoli function and disruption of Sertoli-germ cell interaction leading to bi- and multinucleated gonocytes and decreased sperm production. In female offspring of rats exposed perinatally, a significant delay in vaginal opening was noted (Grande et al., Citation2006; Ma et al., Citation2006). Delayed vaginal opening is comparable to the delay in preputial separation seen in male offspring (David, Citation2006).
These effects are characteristic of some phthalates, namely phthalate esters of straight chain (C4-C6) alcohols to include DEHP (a branched C6 alcohol), DBP, and butyl benzyl phthalate, which share a common active metabolite with DBP (David, Citation2006). Microarray analyses have shown that DBP and DEHP produce a similar pattern of gene expression changes, indicating that these phthalates target the same pathways, at least in male development (Borch et al., Citation2006; Liu et al., Citation2004). While potency may differ, it is thought that these phthalates have a MOA in common; therefore, evidence for key events with DBP could be used to qualitatively address data gaps (David, Citation2006).
The overall conclusion from the data is that the effects of DEHP are not only dose-dependent but also time-dependent, i.e. the stage of the sexual maturation process in both males and females during which DEHP is administered (David Citation2006 among others). The Andrade group demonstrated bi-phasic responses in a number of parameters indicative of androgenic activity with stimulation of testosterone production, for example, in the low dose region, and inhibition of same in the higher dose groups. Further, some effects seen with perinatal or in utero exposure were not seen with only lactational exposure or when administered to older rats (Corton & Lapinskas, Citation2005).
In males, the observed reproductive effects have been proposed to be the result of chemical insult to either the primordial Sertoli cells and/or the primordial Leydig cells of the testes as the targets (Corton & Lapinskas, Citation2005). It is unknown if the effects occur in both types of cells simultaneously or have a primary effect on one specific cell type (Martino-Andrade & Chahoud, Citation2010). Given the complex paracrine relationships of the two cell types, effects in Leydig cells are likely to affect Sertoli cells and visa versa (Martino-Andrade & Chahoud, Citation2010). The effects seen on each cell type may be different and one cell type may be the primary target, while the other is the secondary target depending on the dose and age at which exposure to phthalates occurs (David, Citation2006). For this review, therefore, the potential MOAs for effects in male rats on Leydig cells and Sertoli cells are discussed separately and will focus on perinatal exposure. In females, the target cells are likely the granulosa cells in the ovaries (Lovekamp-Swan and Davis, Citation2003; Martino-Andrade & Chahoud, Citation2010). In this review, the proposed MOA following perinatal exposure only in male and female rats is discussed because these are the endpoints considered quantitatively in this investigation.
Effects in male rats
Effects on Leydig cell function. The potential MOAs for these reproductive/developmental effects in males has been reviewed by a number of investigators to include: Borch et al. (Citation2006), David (Citation2006), Martino-Andrade & Chahoud (Citation2010), Rider et al. (Citation2009), Ge et al. (Citation2007b), Howdeshell et al. (Citation2008), Corton & Lapinskas (Citation2005), Kurahashi et al. (Citation2005), and Foster (Citation2006) among others. In the normal development of the structure and then function of the male rat reproductive system, there are a number of points in the biosynthesis of testosterone that could be targets for DEHP.
Testosterone concentrations in peripheral blood change dramatically throughout the life cycle of male rats and is directly related to Leydig cell development (Cook et al., Citation1999). In the rat, Leydig cells appear in the fetal gonad by day 16 of gestation (GD16) arising exclusively by differentiation of mesenchymal cells shortly after a basement membrane forms around seminiferous cords containing Sertoli cell precursors and germ cells. A postnatal rise in testosterone also occurs but ceases within a few hours after birth and represents not only the increase in testosterone within the testes but also decreased clearance of testosterone. A third and final peak occurs at puberty. In the rat, this rise in testosterone occurs due to the production of a new generation of Leydig cells recruited from mesenchymal-like cells between 2 and 4 weeks of age.
Some of the effects seen by Andrade and colleagues and by Akingbemi and colleagues were consistent with alterations in testosterone biosynthesis. These changes could be occurring: 1) at the level of the hypothalamic-pituitary pathway, i.e. a decrease in LH that results in a decrease in either Leydig cell number or production of testosterone by the Leydig cell; 2) alterations in testosterone metabolism to estradiol, i.e. induction in key enzymes, such as aromatase that would increase the conversion of testosterone to estradiol; 3) changes in brain aromatase activity that would affect masculinization; and/or, 4) alterations in testosterone biosynthesis by the testes due to decreases in one or more of the enzymes in the testosterone biosynthetic chain that begins with cholesterol (Borch et al., Citation2006; Corton & Lapinskas, Citation2005; David, Citation2006; Foster, Citation2006; Ge et al., Citation2007b; Howdeshell et al., Citation2008; Martino-Andrade & Chahoud, Citation2010; Rider et al., Citation2009). While DEHP and other structurally similar phthalates act as antiandrogens in male rodents, these compounds do not bind to the androgen receptor (AR) and are not androgen receptor agonists or antagonists (Foster et al., Citation2001; Mylchreest et al., Citation1999; Parks et al., Citation2000). Also, phthalates were uniformly negative for estrogenicity when tested in vivo in a number of assays including the uterotrophic assay (Corton & Lapinskas, Citation2005).
Critical to the induction of some of the observed effects (i.e multinucleated gonocytes, lower sperm numbers) in the male rat exposed to high doses of DEHP is the reduction in fetal testicular testosterone production (Foster, Citation2006). The decrease in testosterone is mediated by changes in gene expression in a number of enzymes and transport proteins involved in normal testosterone biosynthesis in the fetal and neonatal rat (Borch et al., Citation2006; Foster, Citation2006). Another Leydig cell product, insulin-like factor-3 (Insl-3), is also significantly down-regulated (Borch et al., Citation2006; Foster, Citation2006) and is likely responsible for the cryptorchidism seen at high doses with these phthalates. Lowered testosterone levels also impact the dihydrotestosterone-induced development of the prostate and external genitalia (Foster, Citation2006).
The first step in the MOA of DEHP is the rapid hydrolysis of the diester to the monoester, mono-(ethyl-hexylphthalate) (MEHP) in the gut or shortly after absorption (David, Citation2006). Once absorbed and hydrolyzed by the dam, these monoesters can cross the placenta (Calafat et al., Citation2006; Fennell et al., Citation2004). Once absorbed, MEHP and other monoesters of active phthalates can interact with the proposed target cells (Corton & Lapinskas, Citation2005).
Testosterone levels are dependent on the uptake of circulating cholesterol or the de novo production of cholesterol in the testes. A role for PPARs and another member of the nuclear family of receptors, steroidogenic factor-1 (SF-1), in the interference with normal cholesterol catabolism by DEHP has been suggested by Borch et al. (Citation2006) and others. Corton & Lapinskas (Citation2005) noted that most phthalates that produce adverse effects in the testes are strong PPAR activators and, therefore, a role for PPAR activation by DEHP may be speculated.
In the scheme outlined by Borch et al. (Citation2006) and other reviews, it is suggested that MEHP binds to a PPARα receptor in the liver, thereby, affecting cholesterol production and the availability of serum cholesterol, and/or to one of the PPAR receptors on the Leydig cell membrane resulting in a decrease in cholesterol uptake into the Leydig cell by downregulation of scavenger receptor B-1. This MEHP:PPAR interaction is also proposed to result in the downregulation of peripheral benzodiazepine receptor (PBR), which along with downregulation of steroidogenic acute regulatory gene (StAR) reduces the transport of cytosolic cholesterol into mitochondria where cholesterol is metabolized to pregnenolone. MEHP is also thought to downregulate the gene SF-1 resulting in the downregulation of StAR and the steroid converting enzyme, P450 side chain (P450scc), in mitochondria either directly or indirectly by way of PPARs mediation. Both StAR and PBR cooperate in the transport of cholesterol into mitochondria, where P450ssc in mitochondria converts cholesterol to pregnenolone under the influence of SF-1. Downregulation of SF-1 results in the inhibition of cytosolic enzymes that convert pregnenolone to testosterone by downregulation of 3βHSD, CYP17, 17-OH, 7βHSD and P450c17, which sequentially convert pregnenolone to progesterone, 17-OH progesterone, androstenedione, testosterone, and ultimately, dihydrotestosterone. Sufficient disruption of testosterone biosynthesis would be associated with the antiandrogenic effects seen in rats exposed.
Also attributed to the direct or indirect interaction with SF-1 is a decline in the production of another Leydig cell product, Insl-3, which was also significantly down-regulated by DEHP (Borch et al., Citation2006; Foster, Citation2006). Insl-3 induces the gubernacular cords to differentiate and mature thereby initiating the first phase in testes descent from the kidney area to the inguinal region during fetal life (Howdeshell et al., Citation2008). Decreases in Insl-3 results in gubernacular cord underdevelopment in which these cords become elongated or absent entirely (Howdeshell et al., Citation2008). The absence of or decrements in Insl-3 is thought to be the underlying MOA for cryptoorchidism (Borch et al., Citation2006; Howdeshell et al., Citation2008).
The role of PPAR activation has been questioned based on data in PPAR-null mice; however, these data are not entirely contradictory. Focal tubular degeneration was seen in the testes of PPARα-null mice and wild-type mice given 12,000 ppm DEHP in the diet; however, these effects were noted at an earlier time point, 8 weeks, in wild-type mice than in PPARα-null, where the effects were seen at 24 weeks (Ward et al., Citation1998). Gazouli et al. (Citation2002) noted decreased testosterone levels in wild-type but not PPARα-null mice. Because the PPAR subtype that may be involved in developmental toxicity in rodents is not known with certainty, testicular toxicity could be a function of either of the two other identified PPAR agonists (Borch et al., Citation2006). Alterations in PPARγ levels were significantly altered in rats administered DEHP and may, along with direct or indirect suppression of SF-1, be involved in the downregulation of steroidogenic factors and testosterone levels resulting in adverse impacts in the male (Borch et al., Citation2006).
Effects on sertoli cell function
Sertoli cells are the other major cell type in the testes and function in the normal development and progression of germ cells to functioning sperm cells. In fact, Sertoli cells were the first cell type in the male reproductive system identified as a target cell for phthalates, is indicated by the reduction in sperm production in male rat offspring exposed during the prenatal period (Andrade et al., Citation2006b). The MOA for effects on Sertoli cells is not definitively understood, and may involve interactions with effects on Leydig cells, as noted above (Corton & Lapinskas, Citation2005; David, Citation2006; Foster, Citation2006; Howdeshell et al., Citation2008).
Atanassova et al., (Citation2005) reported decreased numbers of Sertoli cells and decreased volume per cell when androgen levels or other hormones, such as follicle stimulating hormones (FSH) were reduced. Interference with steroidogenesis in the Leydig cell leading to decreases in testosterone levels could indirectly affect Sertoli number. A direct effect of phthalates on Sertoli cells in culture was reported by Li et al., (Citation2000) where exposure to DEHP resulted in a decreased cyclin D2 expression and decreased Sertoli cell proliferation (Li et al., Citation2000). Thus, there may be two potential, but not exclusive pathways that lead to reduced Sertoli numbers (David, Citation2006). A reduction in the number of Sertoli cells leads to fewer gonocytes, (Atanassova et al., Citation2005; Kleymenova et al., Citation2005), and ultimately fewer mature sperm (David, Citation2006; Howdeshell et al., Citation2008).
Another potential MOA for the effects of DEHP and other phthalates is impaired communication between Sertoli cells and gonocytes, leading to the inability of gonocytes to divide (David, Citation2006; Howdeshell et al., Citation2008). Meachem et al. (Citation2001) suggested that a reduced number and/or function of Sertoli cells could result in an impaired ability of genocytes to divide because of a lack of intercellular signals, with the result being bi- and multinucleated gonocytes (David, Citation2006; Howdeshell et al., Citation2008). Exposure to DBP altered the cytoskeleton in Sertoli cells and interfered with communication between Sertoli cells and gonocytes (Kleymenova et al., Citation2005). Further, exposure to DEHP resulted in a decrease in gap junctional intercellular communication (GJIC) by Sertoli cells (Kang et al., Citation2002). Klaunig et al. (Citation2003) noted that inhibition of GJIC is a common associative event with PPAR-active compounds. Consequently, interference with intracellular communication could lead to dysgenesis of gonocytes, which has been noted following exposure to phthalates (Fisher et al., Citation2003; Mylchreest et al., Citation2002).
Relevance to humans. It is suspected that some environmental chemicals, such as phthalate esters, contribute to human testicular dysgenesis syndrome (Borch et al., Citation2006; Howdeshell et al., Citation2008). Swan et al., (Citation2005) reported an increase in altered reproductive development in infant sons of mothers exposed to phthalates during pregnancy. Based on current understanding of the potential role of phthalates in male reproductive/developmental processes, the actions of DEHP and other phthalates can reasonably be expected to be relevant to human health reproductive outcomes.
Effects in female rats
The effects of DEHP on reproductive/developmental systems have been evaluated in female rats administered DEHP by the oral route (Grande et al., Citation2006; Grande et al., Citation2007; Lovekamp-Swan and Davis, Citation2003; NTP, Citation2004) and the inhalation route (Ma et al., Citation2006). These effects included: 1) a significant delay in days to vaginal opening (Grande et al., Citation2006; Ma et al., Citation2006); 2) an increase in the number of ovarian tertiary follicles undergoing atresia (Grande et al., Citation2007); 3) decreases in the age of first estrous cycle, and estradiol, LH and aromatase levels (Ma et al., Citation2006); and, 4) an increase in the number of females with irregular estrus cyclicity (Ma et al., Citation2006). These effects on the female rat reproductive system have been reviewed by Lovekamp-Swan and Davis (Citation2003), Howdeshell et al. (Citation2008), and Martion-Andrade and Chahoud (Citation2010). Lovekamp-Swan and Davis (Citation2003) proposed the hypothesis that MEHP acts on the granulosa cell in the ovary by decreasing cAMP stimulated by FSH and by activating PPARs leading to decreased aromatase transcription. The sequence of events that has been hypothesized in these reviews includes: 1) actions on the granulosa membrane to inhibit FSH-stimulated cAMP, possibly through activation of an inhibitory G-protein; 2) activation of PPARs once inside the granulosa cell; and, 3) decreased transcription of aromatase by PPARα and PPARγ leading to decreased estradiol synthesis and increased estradiol catabolism. Lovekamp-Swan and Davis (Citation2003) have also hypothesized that activation of PPARγ disrupts the critical timing of growth and differentiation of ovarian follicles. Further, increased conversion of estradiol to estrone in both the liver and granulosa cell, with contributions from Theca cells, could result in decreased serum estradiol levels after DEHP treatment (Lovekamp-Swan and Davis, Citation2003).
Relevance to humans. Ovarian hormone production pathways are similar in rodents and humans (Lovekamp-Swan and Davis, Citation2003). PPARγ is expressed in both the human and rodent ovary, and MEHP has been shown to stimulate the transcriptional activity of both human and rodent PPARγ (Lovekamp-Swan and Davis, Citation2003). Therefore, DEHP, through its metabolite, MEHP, could suppress aromatase in the human ovary leading to adverse reproductive/developmental effects.
Proposed mode(s) of action for cancer effects
Chronic bioassays have been conducted in rats to evaluate the carcinogenicity of DEHP (David et al., Citation1999; David et al., Citation2000a; David et al., Citation2000b; NTP, Citation1982; Voss et al., Citation2005). Statistically significant increases in liver adenomas and carcinomas and pancreatic acinar tumors were reported in male or female rats, and Leydig cell tumors in male rats. The possible MOAs for the induction of liver, Leydig cell, and pancreatic acinar cell tumors in rats administered DEHP, liver) and other PPARα active compounds (all three tumor types) have been reviewed by a number of investigators (David et al., Citation1999; Klaunig et al., Citation2003; Rusyn et al., Citation2006; Rusyn et al., Citation2000; Seo et al., Citation2004). The MOA for the induction of liver, Leydig cell, and acinar pancreatic tumors is thought to be PPAR-α mediated (Klaunig et al., Citation2003). However, direct experimental evidence for this MOA specifically with DEHP for Leydig cell and pancreatic acinar cell tumors is lacking. Consequently, in order to identify potential obligatory precursor events for which there may be DEHP-specific data, proposed MOAs for other PPARα-active compounds are discussed for the latter tumor types. This review is limited to MOA data based on tumors in rats as these are the endpoints selected for consideration in this investigation, and further, Leydig cell and pancreatic acinar cell tumors have not been produced in mice (Klaunig et al., Citation2003).
Liver tumors in male and female rats
MOA for liver tumors
It is generally accepted that the tumors in rodents are caused by a PPARα-mediated MOA (Foster, Citation2006; Klaunig et al., Citation2003). Activation of the PPARα receptor is a well-characterized, nongenotoxic MOA causally related to the induction of rodent liver tumors and thought to be specific to rodent livers (Ashby et al., Citation1994; Bentley et al., Citation1993; Cattley et al., Citation1998; Chevalier and Roberts, Citation1998; Klaunig et al., Citation2003; USEPA, Citation2003; USEPA, Citation2005) PPARα is a member of the nuclear hormone receptor superfamily of ligand-activated transcription factors that is activated by PPARα agonists (Klaunig et al., Citation2003). The following sequence has been proposed for PPARα active compounds: 1) formation of a heterodimer with retinoid X-receptor (RXR) in the cytoplasm; 2) transport of the ligand/RXR to the nucleus where it binds to a particular sequence within the promoter region on target genes at specific peroxisome proliferators response elements and, 3) producing changes in gene expression (Shaban et al., Citation2005).
A well-characterized set of biochemical and cellular events has been identified in susceptible rodent strains that include the following: 1) PPARα activation; 2) sustained increase in the transcription of genes involved in peroxisome proliferation, cell cycle/apoptosis, and lipid metabolism; 3) increases in fatty acid β-oxidation leading to oxidative stress; 4) sustained hepatomegaly; 5) stimulation of non-parenchymal cells and inhibition of gap junction intercellular communication, both of which could contribute to the induction of cell proliferation; and, 6) increased cell proliferation and decreased apoptosis leading to proliferation of DNA-damaged cells resulting in hyperplasia and hepatic tumors (Cattley, Citation2004; Cattley et al., Citation1998; Klaunig et al., Citation2003; USEPA, Citation2003). The experimental data provided evidence that DEHP is active in altering all of these key events (Klaunig et al., Citation2003) and that the primary MOA of DEHP in the production of hepatic tumors is by activation of the PPARα receptor.
Guyton et al. (Citation2009) have suggested a PPARα-independent MOA for the production of liver tumors in rodents. DEHP has recently been shown to produce and increase in adenomas but not carcinomas in Sv/129 PPARα null-mice; however, in PPARα competent mice, DEHP did not cause a significant increase in either adenomas or carcinomas (Ito et al., Citation2007). In contrast, in PPARα competent B6C3F1 mice, DEHP produced a significant increase in both adenomas and carcinomas (David et al., Citation1999). Ito et al. (Citation2007) stated that their results suggested the existence of a PPARα-independent pathway. An alternative explanation is the over-expression of PPARγ in the null mice. According to Klaunig et al. (Citation2003), there is considerable “cross-talk” among the subtype PPAR receptors, and according to DeLuca et al. (Citation2000), agents that have some affinity for PPARγ could induce some, if not all, of the pleiotropic effects of peroxisomal proliferation in mice lacking functional PPARα receptors.
Relevance to humans. Both experimental and epidemiological data indicate that the early key events associated with PPARα activation in rodents are possible in humans but there is a lack of concordance between rodents and humans for the downstream events associated with the development of liver tumors in rodents due to species differences in both pharmacokinetics and pharmacodynamics (Rodricks et al., Citation2010). Epidemiological studies of humans chronically exposed to hypolipidemic drugs (Bentley et al., Citation1993; Cattley et al., Citation1998; IARC, Citation1995) and cancer studies in non-human primates (Tucker & Orton, Citation1995) suggest that PPARα agonists do not induce liver tumors in humans. Analyses of liver biopsies of patients treated with therapeutic doses of hypolipidemic drugs showed only slight or no increases in peroxisome numbers or volume density (Bentley et al., Citation1993; Hanefeld et al., Citation1983; Hinton et al., Citation1986). It is generally agreed that development of liver tumors in rodents is not relevant to human health (Klaunig et al., Citation2003; USEPA, Citation2003).
Pancreatic acinar tumors in male and female rats
Proposed MOA for pancreatic acinar tumors
Several PPARα activating compounds have been shown to induce pancreatic acinar cell hyperplasia and neoplasia in rats (Biegel et al., Citation2001; Obourn et al., Citation1997a). PPAR-induced cholestasis in rats has been associated with increased levels of cholecystokinin (CCK), and intestinal growth factors that stimulates release of digestive enzymes from pancreatic acinar cells, and also induce pancreatic acinar cell growth and proliferation (Longnecker, Citation1987; Obourn et al., Citation1997a; Obourn et al., Citation1997b; Tangoku et al., Citation1993). In studies where bile flow was diverted by cannulation of the bile duct, plasma CCK levels were elevated (Li et al., Citation1995; Louie et al., Citation1986). Studies have shown in rats that PPARα activation in the liver resulted in alteration of bile acid biosynthesis, secretion, and bile composition (Hunt et al., Citation2000; Post et al., Citation2001). Obourn et al. (Citation1997a) observed increased total bile acids, serum adenine phosphotase and bilirubin in serum, and decreased concentrations of total bile acids and bile flow relative to liver weight, changes consistent with cholestasis, in male rats receiving ammonium perfluorooctanoate (PFOA) for 6 months. Plasma CCK levels and mean pancreatic weights were also significantly increased. Based on these findings, Obourn et al. (Citation1997a) suggested that the increase in pancreatic weights observed in WY 14,643 (WY)-treated rats were the result of elevated levels of CCK that occurred in response to cholestasis, and speculated that sustained low-level increases in CCK might result in pancreatic acinar cell hyperplasia, focal proliferation within the pancreas, and eventually formation of pancreatic acinar cell tumors.
Available data support the following working hypothesis for pancreatic acinar cell hyperplasia and neoplasia in male rats: (1) activation of PPARα in hepatocytes results in the down-regulation of enzymes that are involved in the synthesis of bile acids and cholestasis; (2) cholestasis serves as a chronic signal to the intestinal mucosal cells to release CCK into the systemic circulation; and, (3) the prolonged increase in CCK levels causes cell proliferation and eventually acinar cell adenoma formation.
Relevance to humans. In contrast, the control of growth of human pancreatic acinar cells does not appear to be mediated by CCK (Ji et al., Citation2001; Ji et al., Citation2002). Rather, in the human pancreatic cells, cellular proliferation appears to be mediated via the activation of a mitogen activated protein kinase pathway in response to a cholinergic signal (2001). Moreover, neither CCK nor gastrin receptors were expressed in human acinar cells (Ji et al., Citation2001). Based on these results, Ji et al. (2001; 2002) concluded that increases in CCK or gastrin would not be expected to stimulate pancreatic acinar cell growth in humans, and that agents that increase the levels of these hormones would not result in altered pancreatic function or size. The results of monitoring of PFO-exposed workers indicated no evidence of cholestasis, increases in CCK levels, or acinar cell proliferation (Olsen et al., Citation2000).
Because activation of PPARα appears to be an obligatory precursor event for the formation of pancreatic acinar tumors by PPs in the rat, this process is assumed to occur via a nonlinear or threshold mode of action. However, the lack of CCK receptors and absence of a proliferative response to CCK in human pancreatic acinar cells suggest that this mechanism is not operative in humans. Consequently, the mechanism(s) by which PPs induce pancreatic acinar cell tumors in rats may not be relevant to human health. However, the USEPA PPAR Working Group (USEPA, Citation2003) maintains that the relevance of these tumors to human health is unknown.
Leydig cell tumors
MOA for Leydig cell tumors
Several PPAR active compounds have been shown to induce Leydig cell hyperplasia and neoplasia in aged male rats (Ashby et al., Citation1994; Bentley et al., Citation1993; Biegel et al., Citation2001; Biegel et al., Citation1995; Cook et al., Citation1992; Mennear, Citation1988; Sibinski, Citation1988). Leydig cell tumors in rats are frequently associated with conditions that increase LH levels, presumably due to the trophic hormone’s mitogenic effects (Clegg et al., Citation1997; Cook et al., Citation1999). However, this common mechanism appears not to be responsible for PPAR-induced Leydig cell tumorigenesis, as LH levels were not significantly altered in rats treated with PFOA or the WY (Biegel et al., Citation2001; Cook et al., Citation1992).
Two pathways have been hypothesized by which PPARα agonists can induce Leydig cell tumors. One proposed pathway, Pathway A, is indirect in that PPARα activation occurs in the liver leading to an induction of the aromatase gene and increased CYP19A2 activity, increased production of aromatase with the subsequent increase in conversion of testosterone to 17-β estradiol and sustained elevation of serum and testicular estradiol levels (Cook et al., Citation1999; Cook et al., Citation1992; Klaunig et al., Citation2003). Increased levels of estradiol were seen following administration of ~13.6 mg PFOA/kg/day for 2 years (Biegel et al., Citation1995). Inhibition of testosterone steroidogenesis in rats has been shown to result in an increased expression of mitogenic growth factors, such as transforming growth factor α (TGFα), in the testis (Biegel et al., Citation2001; Cook et al., Citation1999; Gazouli et al., Citation2002; Klaunig et al., Citation2003). Aging-related increases in serum estradiol is temporally associated with spontaneous development of Leydig cell hyperplasia and neoplasia in Fischer 344 rats (Rao and SubbaRao, Citation1995; Turek and Desjardins, Citation1979) and Wistar rats (Herath et al., Citation2001) is consistent with a causal relationship between the two. PFOA affected sex hormone production and/or release in isolated Leydig cells in vitro (Biegel et al., Citation1995; Liu et al., Citation1996). As noted in the section on developmental toxicity, the working hypothesis for Pathway A would be: 1) PPARs modulate the activities of several major hepatic sex steroid-metabolizing enzymes, including induction of cytochrome P450 19A1 (CYP19A1; aromatase) and repression of cytochrome P450 2C11 (CYP2C11), via a PPARα-dependent mechanism(s) in the liver; 2) altered hepatic enzyme activities result in sustained elevation of serum and testicular estradiol levels; 3) increased estradiol levels in the testis stimulate secretion of TGFα, a Leydig cell mitogen; and, 4) TGFα causes Leydig cell hyperplasia and adenoma formation (Biegel et al., Citation2001; Biegel et al., Citation1995; Cook et al., Citation1999; Cook et al., Citation1992; Klaunig et al., Citation2003). The mechanism(s) by which elevated levels of estradiol and TGFα (and/or other growth factors) might result in PPAR-induced Leydig cell hyperplasia and neoplasia have not been fully elucidated, and results of studies with phthalates suggest that complex interactions among multiple signaling pathways are involved (Akingbemi et al., Citation2004). However, available data are consistent with the hypothesis that rat-specific, PPARα-dependent hepatic effects are prerequisite for PFOA-induced Leydig cell hyperplasia and neoplasia.
The second pathway, Pathway B, involves direct inhibition of testosterone biosynthesis at the level of the testes (Klaunig et al., Citation2003). The biological events by which a PPAR active compound can affect steroidogenesis have been discussed in the section (Effects on Sertoli cell function section) for the hypothesized MOA reproductive/developmental effects beginning with the inhibition of PBR with the resulting cascade of events. Decreased testosterone levels can then result in the upregulation of LH. The sustained signal of LH on the Leydig cell from LH stimulation would then result in Leydig cell proliferation and the production of Leydig cell tumors.
Relevance to humans. Leydig cell tumors are very common in rodents but extremely rare in humans, accounting for about 0.01% of all cancers in human males, with an estimated age-adjusted incidence of only 0.00004% (Bosland, Citation1996; Clegg et al., Citation1997; Cook et al., Citation1999). Quantitative and qualitative differences documented between rat and human hepatic PPARα indicate that the human response to PPARs is both more limited in scope and less intense than that of rats. Consequently, the downstream consequences of the activation of PPARα (e.g. increases in estradiol) would also be quantitatively and qualitatively different. Mice and monkeys, species considered to better reflect human biology in this regard, do not develop Leydig cell adenomas in response to PPARs.
No specific chemical exposures have been associated with testicular cancer risk in humans (Bosland, Citation1996); the known risk factors are (1) heritable disorders of the endocrine system such as congenital adrenal hyperplasia and androgen insensitivity syndrome, (2) Klinefelter’s syndrome, and (3) cryptorchidism, conditions that are not exacerbated by exposures to PPARs. Despite widespread human exposure to agents known to induce Leydig cell hyperplasia and neoplasia in rats (including nicotine, lactose, 1,3-butadiene, cadmium, trichloroethylene, MTBE, fibrate hypolipidemic drugs and other PPARs such as PFO), the incidence of clinically apparent Leydig cell adenomas has not increased (Bosland, Citation1996; Clegg et al., Citation1997; Cook et al., Citation1999). Workers exposed to relatively high concentrations of PFO for multiple years have not experienced either alterations in reproductive hormone levels or increased incidence of testicular tumors (Alexander, Citation2001; Alexander et al., Citation2003; Gilliland and Mandel, Citation1993; Olsen et al., Citation1998; Ubel et al., Citation1980; Walrath, Citation1996). Similarly, there were no changes in reproductive hormone levels or Leydig cell proliferation in monkeys treated with PFOA (Butenhoff et al., Citation2002; Goldenthal et al., Citation1978; Thomford, Citation2000). Thus, the biochemical cascade of events that resulted in increased estradiol and/or decreased testosterone levels and Leydig cell tumors in rats does not appear to occur in monkeys or humans exposed to other PPAR active compounds.
Based on species differences in endocrine regulation and Leydig cell tumor biology, as well as differences in hepatic (and possibly testicular) effects mediated by PPARs, the events associated with tumorigenesis in rats and humans are likely to be mechanistically distinct; therefore, PPAR active compounds are unlikely to cause Leydig cell hyperplasia or neoplasia in humans. Because workers and hypolipidemic drug patients receive relatively high and prolonged levels of exposure to PPARs compared to those likely to be experienced by the general public without signs of Leydig cell effects that would be indicative of potential tumorigenesis in Leydig cells, it is not expected that the general population would experience PPAR-induced effects on Leydig cells.
Traditional/conventional approaches for characterizing dose–response
Typically, dose–response assessments have been conducted for noncancer and cancer endpoints separately because of the assumptions about the unspecified but presumed underlying modes of action for these types of toxicity. It has traditionally been assumed that noncancer endpoints had a threshold below which the adverse effect was not expected to occur. In the assessment of cancer endpoints, however, it was assumed that development of cancer occurred by biological processes that were linear in the low-dose region and did not have a threshold; that is, any amount of exposure, no matter how small, was assumed to have some probability of causing cancer, even if that probability was vanishingly small.
In either case, one element in common with both noncancer and cancer dose–response assessment was to rely on the endpoint assumed to be the most sensitive endpoint in the most sensitive species, based largely on statistical considerations. The challenge with noncancer risk assessments was to identify a No Observed Adverse Effect Level (NOAEL), which was sometimes indistinguishable from the No Observed Effect Level usually because of the lack of definitive data. Once the critical NOAEL is identified, this value is then divided by a series of Safety or Uncertainty Factors (UFs), typically factors of 10, to usually address three elements of uncertainty: 1) extrapolation across and within species; 2) adjustment for duration of exposure (subchronic to chronic); and, 3) consideration of database limitations. The result is an “acceptable” exposure level, that is, a Reference Dose (RfD) or Reference Concentration (RfC) for noncancer endpoints by the oral and inhalation routes, respectively.
While noncancer dose–response assessments have traditionally relied upon the selection of a dose from the critical study, because of the assumption of a linear, non-threshold dose–response for cancer endpoints, mathematical models, in particular the Linear Multistage model, which is a statistical treatment of the assumed linear biological processes leading to cancer, have been applied (USEPA, Citation1986; USEPA, Citation1999; USEPA, Citation2005). The resulting output is a Cancer Slope Factor (CSF) or Unit Risk (UR) estimate which is defined as the probability of developing cancer in a million persons exposed similarly to the chemical of interest for an entire lifetime at a dose of 1 mg/kg/day or 1 mg/m3. Rather than the application of UFs, in a traditional cancer dose–response assessment, extrapolation across species was assumed to be accomplished by converting the administered dose in the animal study to a human equivalent dose by scaling initially by comparative surface area (body weight2/3). In the last decade, this scaling factor has been revised to be comparative by body weight3/4. Footnote1 Low dose extrapolation and consideration of uncertainty were addressed by the linear extrapolation outside of the observable range to specified risk levels (policy derived) and by selecting the statistical upper bound (95th percentile upper confidence limit) on the most likely estimate of dose at the specified risk level, respectively. As with noncancer endpoints, uncertainty was not explicitly quantified.
With the recent attempts to harmonize noncancer and cancer risk assessment, both approaches have focused on two major steps in the quantitative characterization of potential health effects. The first is the use of the data in the observable region of the dose–response curve to derive a point of departure (POD),Footnote2 which can be derived two ways− use of the NOAEL/LOAEL approach and/or the application of mathematical modeling, in particular Benchmark Dose modeling. In either case, extrapolation to lower exposures and consideration of study design and potential uncertainties in the data are assumed to be accomplished by the use of uncertainty factors (UFs).
The following sections provide a demonstration of the application of these traditional approaches in the derivation of a POD for both cancer and noncancer endpoints for DEHP. These traditional assessments will provide a “baseline” range of PODs for comparison to PODs developed following the incorporation of alternative approaches into dose–response assessments.
Estimation of a POD for noncancer endpoints using a NOAEL/LOAEL approach
After a review of the data for a compound, one study and one data set in that study is typically relied upon to estimate a POD for noncancer endpoints. In this case study, several studies and endpoints that could potentially serve as the basis for the POD were identified and evaluated to illustrate the impact that the use of different studies or endpoints and different decisions made when using these data can have on characterizing the dose–response for a compound. For this initial “baseline” approach, the recent reproductive/developmental data for DEHP were focused on to determine an oral and an inhalation NOAEL/LOAEL that could serve as the basis for a POD.
Determination of an Oral NOAEL/LOAEL
Following oral exposure to DEHP, the available toxicological data suggest that the most sensitive effects and those most likely to provide the basis for a POD have been reproductive/developmental effects (ATSDR, Citation2002; Kavlock et al., Citation2006). As noted in the previous sections, various developmental effects have been observed in both male and female animals over similar dose ranges. The effects noted range from observational effects, such as a change in anogenital distance in male offspring, to dose-related changes in hormone levels, such as increases in serum testosterone. When relying upon reproductive/developmental effects as the basis for the derivation of a POD, changes in normal endocrine balance in the developing organism can have a significant impact on the development and eventual capabilities and function of the reproductive system. In conducting noncancer dose–response assessments for other organ systems in the adult, such subtle changes in clinical chemistry are not typically relied upon, as the mechanisms to maintain homeostasis are more developed in the adult.
A wide range of NOAELs/LOAELS can be identified for DEHP noncancer effects (), depending on the endpoint, with the more sensitive endpoints and the lowest doses tested reported by Andrade and coworkers (Citation2006c; Citation2006b; Citation2006a) in male and female rats following in utero/lactational exposure. Evaluation of the multiple endpoints in these studies in a traditional manner when determining a POD would focus on the most sensitive endpoint in the most sensitive species in a single study from a statistical perspective. This type of approach may or may not consider the differences in impact on reproductive/developmental parameters based on window of exposure and differences in time of observation of an endpoint, which are critical in the assessment of reproductive/developmental effects and their potential relevance to human health. The focus of this section will be the POD selected if decisions were made strictly based on statistical results with little consideration of biological underpinnings and whether or not an endpoint should be considered “adverse”.
Table 20. NOAELs/LOAELs for reproductive/development endpoints based on evaluation of statistical results.
In the Andrade et al. (Citation2006c; Citation2006b; Citation2006a) studies (, , ), the dose associated with the lowest statistically significant change in response is 0.015 mg/kg/day reported by Andrade et al. (Citation2006a). The change reported at this dose level is an increase in anogenital distance in male rats at postnatal day (PND) 22, following in utero/lactational exposure to DEHP. While statistically significantly different from controls, it is an increase, in contrast to decreases in distance anticipated based on the proposed antiandrogenic effects of phthalates reported in the literature, with no significant changes observed in this endpoint in mid doses (0.045 to 135 mg/kg/day). A significant decrease in this parameter was reported only at the highest dose tested (405 mg/kg/day). These results suggest that additional information outside of the results provided in the study would be needed to determine the potential relevance of this result and whether the POD should be 0.015 versus 405 mg/kg/day.
No other statistically significant change was reported by Andrade et al., (Citation2006a) in male or female rats at the 0.015 mg/kg/day dose level. At the next highest dose (0.045 mg/kg/day), a significant increase in serum testosterone and a significant decrease in sperm production were reported at PND 144 by Andrade et al. (Citation2006c). A comparison of sperm production in the treated males with the concurrent control animals indicated a significant decrease (<25%) in sperm production in all treated groups administered 0.045 mg/kg/day and greater. However, a comparison to historical controls indicated a statistically significant difference at doses of 1.215, 15, 45, 135, and 405 mg/kg/day. The use of either of these endpoints to estimate the POD would suggest a NOAEL of 0.015 or 0.405 mg/kg/day. It must be considered, however, when relying upon an endpoint such as changes in sperm production whether this change would actually result in an adverse effect.
The authors noted that the reduction in sperm production up to 5 mg/kg/day was within the variability of recent historical controls, and therefore not biologically significant. The authors also suggested that a reduction of 20% or greater in sperm production (seen at doses of 15, 135 and 405 mg/kg/day) should be used as a cut-off value for biologically relevant effects as this change in production could result in subfertility (increased time to conception) in humans. However, no impact on reproductive performance was noted in the male rats and the authors noted that a reduction of up to 90% of sperm production in the rat may not adversely affect fertility. This would suggest species differences that may be critical in the estimation of the POD based on this endpoint, but the data to confirm this difference may not be available. However, depending upon the acceptance of the arguments provided by the authors, a POD of 5 mg/kg/day could be justified. It could also be concluded that because there was no impact on reproductive performance in these male rats that the decrease in sperm production observed does not represent an adverse effect, resulting in the highest dose tested being identified as a NOAEL (405 mg/kg/day) for this endpoint.
For the remainder of the endpoints evaluated by Andrade and co-workers, a consistent dose-related pattern of change in endpoints was not observed until doses of 15 mg/kg/day were administered. At this dose level, significant changes in the age at vaginal opening were observed in female rats and the day of preputial separation in the male rat, both of which are an indicator of the onset of puberty, respectively. A significant delay in onset is noted in both sexes at doses of 15 mg/kg/day and above, supporting a NOAEL or POD of 5 mg/kg/day.
Using only the results of statistical analyses to make decisions regarding the POD could result in the selection of NOAELs ranging from 0.015 to 405 mg/kg/day, depending upon the rationale applied. Additional data and approaches need to be incorporated to refine the doses that may be representative of a threshold for the effects observed.
Determination of an inhalation NOAEC/LOAEC
Without the incorporation of PBPK modeling, the traditional approach has considered only those studies by the inhalation route of exposure for the derivation of a POD to serve as the basis for a Reference Concentration (RfC). In the case of DEHP, few inhalation studies have been conducted.
The most recent studies that could be considered in the derivation of a traditional NOAEC/LOAEC or POD are those conducted by Kurahashi et al. (Citation2005) and Ma et al. (Citation2006). These studies were conducted by a single group of investigators and focused on the evaluation of the impact of inhalation exposure of DEHP to male and female Wistar rats. As detailed previously, Kurahashi et al. (Citation2005) focused on the effects in male rats, while Ma et al. (Citation2006) focused on the effects in female rats following exposure to 0, 5 or 25 mg/m3 DEHP for 6 h/day, 5 days/week.
In contrast to the results following oral exposure in rats, female rats appeared to be more sensitive to inhalation of DEHP. A statistically significant concentration-related decrease in the age of vaginal opening and age at first estrous was observed in female rats following inhalation exposure to 5 or 25 mg/m3 DEHP on PND 22 to 41 or 22 to 84 (). Statistically significant concentration-related changes were also observed in male rats, including increases in seminal vesicle weight and serum testosterone levels. However, these effects were only observed following 8 weeks of exposure, while significant effects were observed in females following 4 weeks of exposure. This may be a difference in duration of exposure, but could also be attributed to exposure during critical windows of development. Regardless of whether the results in males or females are relied upon, a LOAEC of 5 mg/m3 would be the POD to derive an RfC, with no NOAEC identified.
Consideration of mathematical modeling in the determination of a POD for noncancer
In the last decade, advances have been made in both noncancer and cancer dose–response assessments. While always recommended in the determination of an acceptable toxicity value or a POD for cancer endpoints, mathematical models (i.e. Benchmark Dose (BMD) modeling) are now recommended for use in noncancer dose–response assessments, when the appropriate data are available, to estimate the POD then used in the derivation of an RfD or RfC rather than a NOAEL/LOAEL. The value typically used as the POD when BMD modeling is applied is the statistical lower confidence bound on the BMD, termed the BMDL. The BMDL is the value estimated to result in a specified increase in risk or change in response; the specified risk or response level is termed the Benchmark Response (BMR) level, and doses corresponding to that level. The BMD approach has several advantages over the NOAEL approach:
The BMD approach, unlike the NOAEL, takes into account the dose–response information (i.e. the shape of the dose–response curve);
The BMD approach does not involve sometimes argumentative “all or nothing” decisions, such as determining whether or not a NOAEL was defined at a particular dose;
The value of the lower confidence limit appropriately reflects the uncertainty associated with the sample size of the study (smaller studies have lower confidence and thus tend to result in wider confidence limitsand lower BMDs, whereas the opposite is true for NOAELs); and,
A benchmark dose can be determined even when a NOAEL has not been identified in a study.
The BMD approach has been recommended as the basis for the derivation of toxicity values because it is an approach without some of the limitations inherent in the NOAEL approach, and, therefore, recommended as a means to improve noncancer toxicity assessment (Barnes et al., Citation1995). When deriving an RfC or RfD based on the BMDL, the same sets of UFs would be applied to the POD as were used in the NOAEL/LOAEL approach. This approach is also similar to that used in cancer dose–response modeling, with the use of different terminology (i.e. BMDL10 versus LED10), but a similar concept.
Model Selection
In the application of the BMD approach, various models are available to choose from using the USEPA program BMDS (USEPA, Citation2000a). These models enable the risk assessor to conduct dose–response modeling for both quantal (e.g. incidence data) and continuous (e.g. changes in serum testosterone) endpoints. A brief description of the available models is provided in Appendix A. The model relied upon most often is the Multistage model, which is the standard model applied for cancer dose–response modeling as well. Several additional models are available and can be applied to attempt to better characterize the dose–response curve for individual endpoints.
In making a final determination of the model to be relied upon for estimating the BMDL or POD, there are many considerations. The fit of the model to the data is usually a determining factor in the selection of the dataset to be relied upon in the selection of the POD. The BMDS program provides two statistical values, the Akaike Information Criterion (AIC) (Akaike Citation1974) and the goodness-of-fit p-value, which provide an estimate of the fit of the model to the data. In conducting BMD modeling for risk assessment purposes, the USEPA prefers data sets with model goodness-of-fit p-values of 0.1 or greater (USEPA, Citation2000a). If the model fit value is less than this, the model in question does not adequately reflect the shape of the dose–response curve for the endpoint of interest; therefore, the results of the BMD analysis for that dataset are deemed unacceptable. Characterization of the relative fit between selected models for a particular dataset is assessed by the AIC. In comparing across model results for a single endpoint, the application of the model that results in the smallest AIC value indicates a better fit.
Among the various models available for continuous endpoints, the Hill model often appears to be the most problematic. It is a model that postulates an asymptotic level of response (the maximum or minimum response level that is approached as dose gets very large); when the data being analyzed do not suggest a value for, or even the existence of, such an asymptote, then the model may fit the data in non-intuitive ways.
Moreover, if the data demonstrate responses in a low-dose region (i.e. for some of the lowest experimental doses) that are not substantially different from the control response, with one or more high dose responses demonstrating some higher responses at roughly the same level (i.e. suggesting an asymptotic response level), or if there is only a control response before those high, apparently asymptotic, responses at the higher dose levels, with no experimental observations in between, then the model has no information about where intermediate responses should occur. In such cases, the modeling may result in estimating a dose–response that rapidly increases up to or close to the maximum response. This may be associated with a large estimated value of the power parameter n, in which case the low-dose response pattern would be predicted to be very flat followed by a steep increase, or it may be associated with an n value of 1 (if that was set as the lower bound on n) and a small value of k, in which case the curve will increase steeply right from zero dose. In any of these cases, the model fit may well be the best available (it is indeed the curve that gives the maximum likelihood) but the information available for fitting the model may not be adequate to constrain it to what might be considered reasonable values.
There is another issue to be aware of with respect to the Hill model or any model that postulates an asymptote. In some cases the requested Benchmark Response (BMR) may be outside the range of responses estimated by the model. If the BMR is a one standard deviation change in the mean (a common default choice), but the maximum (or minimum) response is less than 1 standard deviation away from the control mean, then there will be no dose that corresponds to the requested BMR (i.e. no BMD). The BMD will not be estimated by the model in such cases. If the endpoint is a rare or subtle endpoint that is toxicologically relevant, an alternative definition of the BMR may be needed in these instances because, in all likelihood, the model may be fitting the data adequately and appropriately.
In some instances, the polynomial model, which is also a continuous model, may exhibit idiosyncratic fitting properties, where the fit for one choice of the degree of the polynomial does not match the fit for the choice of another degree, even when the fits estimated by those two models should be the same (e.g. when they both result in a linear model, which is a special case of both of them). The reasons for such behavior are not well understood; the user is advised to try several choices for the degree of the polynomial if and when suspect model fits are observed. In all cases, the visual and statistical assessment of fit should guide the user to identify potentially problematic cases which should then be referred to a statistician.
All models are potentially susceptible to failures in BMD (ED) or BMDL (LED) estimation, especially the latter, because optimization routines are not infallible. When such failures occur, the user should seek statistical advice (e.g. with respect to initial values that might improve optimization) and might be best served by relying on other software. This is true for the models fit to dichotomous or quantal data, as well as those fit to continuous data.
Estimation of an Oral POD for noncancer
To determine the impact of using a mathematical model to determine a POD versus the use of a NOAEL/LOAEL for DEHP, the datasets that provided the lowest NOAELs/LOAELs from in utero/lactational exposure were modeled. These included anogenital distance in male rats at PND 22 (Andrade et al., Citation2006a), decreased sperm production in male rats (Andrade et al., Citation2006b), changes in the age of vaginal opening in female rats (Grande et al., Citation2006) and the age of preputial separation in male rats (Andrade et al., Citation2006a).
All of the available and applicable models (i.e. continuous models for continuous data) in BMDS were applied to each dataset using the information from all dose groups (). However, because of the nonmonotonic nature of the responses (, , and ), an adequate fit of the models to the data (p>0.05) could not be achieved for any of the datasets that would appear to be the basis of the most sensitive NOAEL/LOAELs (i.e. anogenital distance, sperm production).
Figure 1. Anogenital distance of male offspring rats (PND 22) exposed in utero and during lactation to peanut oil (vehicle control) or DEHP (Andrade et al. Citation2006a). Bars indicate mean±S.E.
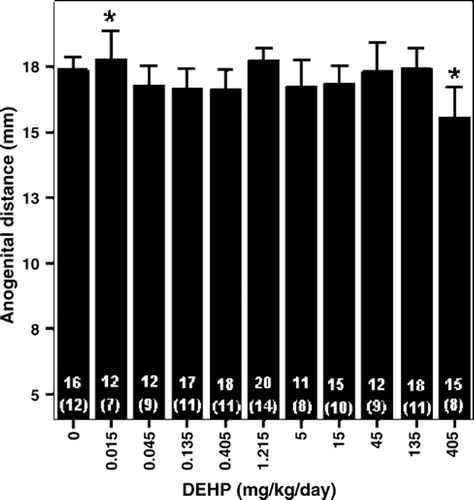
Figure 2. Effects of in utero and lactational DEHP exposure on daily sperm production in adult male offspring rats (Andrade et al. Citation2006). This figure shows the absolute and relative proportion of animals with sperm production above and below 34 million sperm/testis. Number of animals is indicated inside bars. *Significantly different from concurrent control. §Significantly different from historical control.
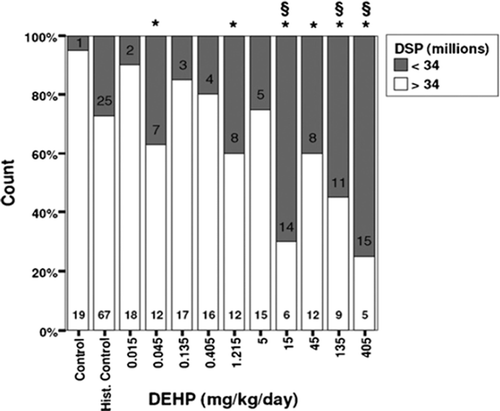
Figure 3. Biphasic effect of di(2-ethylhexyl)phthalate (DEHP) exposures on puberty (Ge et al. Citation2007a). Pubertal onset was assessed by preputial separation. Prepubertal rats were gavaged with DEHP (0, 10, 500, and 750 mg/kg/d). The time course of the accumulative frequency of rats with preputial separation was fitted by sigmoidal nonlinear regression. Average age was calculated as the intercept at 50% accumulative frequency, shown as the dotted line.
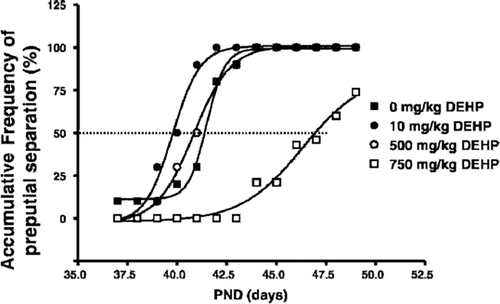
Table 21. BMD Modeling results based on selected reproductive endpoints using external administered doses as the relevant dose metric. (Data relied upon provided in and ).
Although statistically significant increases in the incidence of bi- and multinucleated gonocytes in male rats on PND1 (Andrade et al., Citation2006a) and tertiary aretic follicles in female rats (Grande et al., Citation2007) were also observed, these endpoints only achieved statistical significance at much higher doses (135 and 405 mg/kg/day) than those endpoints previously considered as the potential basis for the NOAEL/LOAEL. However, an attempt was made to model these endpoints as well to determine if any of the available mathematical models could successfully fit the results from the multi-dose in utero/lactational exposure study. While an acceptable fit could be achieved for these endpoints (), these are not likely the more sensitive endpoints and are the result of high-dose administration. The results of the BMD modeling using the incidence of bi- and multinucleated gonocytes in the male rats provided estimates of the lowest BMDL10 or POD of approximately 30 mg/kg/day using the Multistage model and a value of approximately 135 mg/kg/day based on the incidence of tertiary aretic follicles in female rats using the Linear model. While these endpoints are possibly not the most sensitive, based on the evaluation of potential NOAELs/LOAELs, the results demonstrated that these endpoints increased in a standard monotonic dose-related manner. Incorporation of the underlying MOAs for these two endpoints should be considered to determine how the other endpoints observed at lower doses in the Andrade and co-workers studies may be used in combination with these endpoints to estimate the most scientifically defensible POD.
Table 22. BMD Modeling results using external maternal doses (mg/kg/day) as the relevant dose metric. (Data relied upon provided in and ).
It has been common practice in the past for the dose–response modeling of cancer endpoints, when a response initially increases with dose and then reaches maximum response, high dose groups may be dropped from consideration for dose–response modeling. The justification for dropping high doses is that the low-dose region is the region of the dose–response curve that is typically critical for assessing potential risk of adverse effects in humans. The issue with the shape of the dose–response curves for the endpoints that may serve as the basis of the POD, based on the NOAEL/LOAEL approach, is that no dose-related statistically significant change is observed in the low range of concentrations (0.015−1.215 mg/kg/day) with a sustained significant difference from control starting at 15 mg/kg/day for selected endpoints including the age at vaginal opening in females (Grande et al., Citation2006) and the day of preputial separation in male rats (Andrade et al., Citation2006a). This may suggest that the sporadic statistically significant changes observed in the lower dose groups are the result of biological variability and not treatment-related. If this were the case, it suggests a threshold for effects around 5 mg/kg/day. In the application of models, an approach was tested to determine if an acceptable fit could be achieved by dropping low doses, rather than high doses as is typically done in cancer assessment, under the assumption that the changes observed in the lower dose groups are not biologically significant.
This was initially tested with the data on the change in the age of vaginal opening reported in female rats by Grande et al. (Citation2006) and the day of preputial separation in male rats reported by Andrade et al. (Citation2006a). These endpoints are associated with the potential antiandrogenic effects of DEHP. The age at vaginal opening in the female rat was reported as both a continuous and a dichotomous response (Grande et al., Citation2007). The results were provided as the mean (± SE) age at vaginal opening or by classifying the animals into one of three groups (age of vaginal opening on PND 33–34; PND35–37, or > PND37). While a similar endpoint (change in age of preputial separation) was reported in male rats, the results were only reported as a dichotomous response by classifying animals into one of three groups (age of preputial separation on PND 35–36, PND 37–38, or >PND38). For each of the datasets in which the animals were categorized into a range of days to vaginal opening or preputial separation days, the data were assessed with a single division (e.g. day of vaginal opening separated into ≤ 34 or > 34) to create a dichotomous dataset. To initially attempt to derive a POD using BMD modeling, the age of vaginal opening reported as the mean ±SE was relied upon because it is provided as the mean as well as the variability (SE) in the response per group.
Using the age of vaginal opening in female rats in the control and top seven dose groups (0.405− 405 mg/kg/day), an adequate fit was achieved with the Polynomial model (p = 0.07) (). The resulting POD or BMDL10 was approximately 120 mg/kg/day. While the fit of the model to this many dose groups was adequate (p ≤ 0.05), additional low-dose groups were dropped to attempt to improve fit. The use of the top 5 doses groups plus control did not result in an improved fit of the models to the data, with the exception of the Hill model, which could not estimate a lower bound. Dropping doses further to control plus 3 treated groups (45− 405 mg/kg/day), the minimum number of groups typically recommended to characterize the dose–response for a compound, resulted in an improvement of fit with the Polynomial model (p = 0.33) and an estimated BMDL10 of approximately 65 mg/kg/day.
Table 23. BMD Modeling Results considering age at vaginal opening in Wistar rats () as a continuous endpoint as reported by Grande et al., (Citation2006) Study and using external maternal doses (mg/kg/day) as the relevant dose metric.
Using the dichotomous results for the age of vaginal opening (dividing the animals into day of opening ≤ 34 or > 34 or ≤ 37 or > 37), no adequate fit of the models to the data could be achieved (). Even reducing the treatment groups for consideration to the control and the top three dose groups (45− 405 mg/kg/day) did not result in an adequate fit of the models to the data.
Table 24. BMD Modeling Results considering age at vaginal opening in Wistar rats () as a dichotomous endpoint as reported by Grande et al., (Citation2006) Study and using external maternal doses (mg/kg/day) as the relevant dose metric.
Because of the lack of a conventional monotonic dose–response curve for the majority of the endpoints in the Andrade et al. (Citation2006c; Citation2006b; Citation2006a) papers, an additional approach was attempted to achieve an acceptable fit for a selected dataset. Rather than dropping dose groups from the low end or the upper end of the dose–response curve as has been traditionally conducted, selected dose groups were modeled that appeared to capture the overall shape of the dose–response. This approach or “smoothing” of the dose response curve was attempted with two endpoints in male rats: the day of preputial opening and decreased sperm production (Andrade et al., Citation2006a), using the standard default dose–response model, the Multistage model.
Days to preputial separation was evaluated two ways. The results were categorized into three groups: age of preputial separation on PND 35–36, PND 37–38, or >PND38. Since the data had to be modeled as a dichotomous endpoint, the results were modeled under one of two assumptions: 1) assuming a delay of greater than day 36 would be adverse and 2) assuming a delay of greater than day 38 would be adverse. This endpoint is mainly dependent on increasing levels of estradiol and/or decreasing levels of testosterone during the perinatal period, and a delay in these processes, as well as the observation of delayed vaginal opening in the female rat, may suggest estrogenic or antiandrogenic activity (Ge et al. 2007b; Borch et al. Citation2006).
In “smoothing” the dose–response information, the modeler should be aware of the potential impact on the model estimates and should make sure that the results are not inconsistent with the observed data. For example, using the information assuming a delay of greater than day 38 would be adverse (), depending upon which portion of the dose response curve is considered the most important (i.e. dose groups 1, 10 and 11), results in PODs differing by orders of magnitude. Relying upon the low-dose region of the curve (groups 1-4) results in a POD of 0.03 mg/kg/day, while relying upon only the high-dose region of the curve (groups 1, 10 and 11) results in a POD of approximately 47 mg/kg/day.
Table 25. BMD modeling results for considering day of preputial opening in male Wistar rats () as a dichotomous endpoint as reported by Andrade et al. (Citation2006c) and using external maternal dose (mg/kg/day) as the relevant dose metric.
Smoothing of the dose–response curve was also attempted using decreased sperm production (Andrade et al., Citation2006a). Numerous combinations of dose groups were modeled, but in the case of this endpoint an acceptable fit of the model could not be achieved ().
Table 26. BMD Modeling results for changes in sperm production in Wistar rats () from Andrade et al. (Citation2006a) using external maternal dose (mg/kg/day) as the relevant dose metric.
Summary
With the phthalates and in particular DEHP, observations at high doses may not be predictive of effects/changes at low doses. What is most striking about the Andrade and coworkers studies is that while they provide some evidence that a particular window of exposure, in utero/lactational, may be the most sensitive period, those endpoints that appear to be most sensitive lack a conventional monotonic dose–response. Application of BMD modeling to these datasets, while beneficial for many reasons, is not adequate to address the changes in endpoints measured in these reproductive/developmental studies. Even using nonconventional methods, such as dropping dose groups and smoothing of dose response information, while successful in selected cases, was largely unsuccessful in providing consistent results or resulting in acceptable fits. For many of the endpoints measured in these studies two observations were noted: 1) there was considerable variability in response (given the way the data are presented in figures and tables in these studies); and 2) for most of the endpoints, there were some sporadic changes in the low dose region, but then statistical significance achieved in more of the responses in the high dose region, without monotonic changes in the measure of that response beyond that point of inflection. This exercise provided evidence that in the case of the complex endpoints observed in reproductive/developmental studies, the application of mathematical modeling may not be adequate to provide an acceptable estimate of a POD. In addition, the lack of the fit of a mathematical model to critical toxicity data does not provide the risk assessor with a reason to reject the importance of a particular endpoint and eliminate it from consideration in making the determination of a potential acceptable level of a chemical. Instead it should challenge the risk assessor to investigate further the biological implications of the effects observed to better determine the potential threshold for reproductive/developmental effects following low-dose oral exposure.
Determination of an inhalation POD for noncancer
As mentioned previously, limited information was available to address the potential reproductive/developmental effects of DEHP following inhalation exposure. In both the Ma et al. (Citation2006) and the Kurahashi et al. (Kurahashi et al., Citation2005) studies no NOAEC was identified, with statistically significant effects (i.e. age at vaginal opening) at the lowest concentration tested. In this case, the application of BMD modeling is beneficial because it can estimate a NOAEC equivalent concentration for the POD.
Application of the continuous BMD models to the age of vaginal opening in female rats following exposure for PND 22–41 (Experiment 2) to those estimated following PND 22–84 (Experiment 1) (Ma et al., Citation2006), resulted in acceptable fits for all of the models, except the Hill model (). Results from one control group and two treated groups did not provide an adequate number of data points for the Hill model to be applied. In comparing the PODs estimated for Experiment 1 versus Experiment 2, lower PODs were estimated for Experiment 1 (13.1 mg/m3) than for Experiment 2 (20.1 mg/m3). It is likely that this would reflect the difference in the dose–response curves for different exposure durations, with the longer duration resulting in the lower POD.
Table 27. BMD modeling results from Ma et al. (Citation2006) () and Kurahashi et al. (Citation2005) () using external inhalation concentration (mg/m3) as the relevant dose metric.
Because statistically significant effects were observed at the lowest concentration tested in Ma et al. (Citation2006) (5 mg/m3), it might be expected that the estimated POD using the BMD approach would be lower than this concentration. However, in this particular case, the estimated POD is driven by the shape of the dose–response curve (). Because of the limited number of data points and the sharp drop in response in the low-concentration group compared to controls, many of the available models have difficulty fitting this type of curve. As shown for these two datasets, all of the models have reduced to a linear model. While the fit is acceptable and the model is within the variability of the response, the curve does not drop as rapidly as the data demonstrate and is on the upper end of the variability in response for the low concentration group. The result is a higher estimate of the BMD than would be estimated if the model-predicted curve dropped in a manner closer to the shape the data suggests.
Figure 4a. BMD modeling of the age of vaginal opening in female Wistar rats following inhalation exposure to DEHP as reported by Ma et al. (Citation2006) (Experiment 1− exposure PND 22–84).
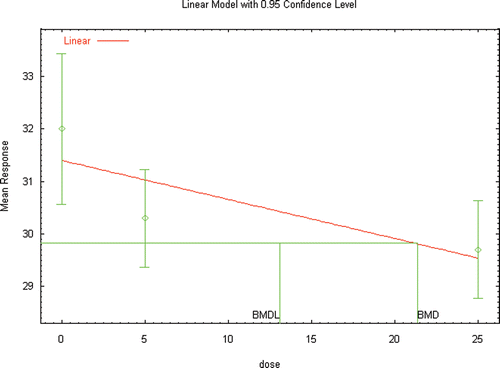
Figure 4b. BMD modeling of the age of vaginal opening in female Wistar rats following inhalation exposure to DEHP as reported by Ma et al. (Citation2006) (Experiment 2− exposure PND 22–41).
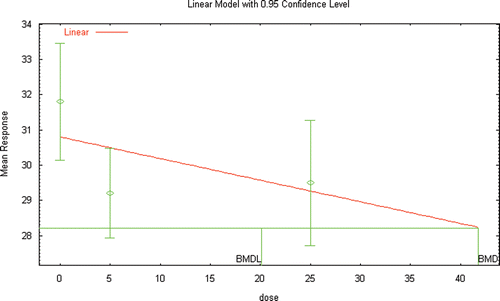
As with many of the datasets evaluated previously, acceptable fits of the models could not be achieved using the data for increases in testosterone or seminal vesicle weight observed in male rats (). As with the dataset in the female rat, the models could not adequately describe the shape of the dose–response curve in the low-dose region (). The responses reported in male and female rats by Ma et al. (Citation2006) and Kurahashi et al. (Citation2005) suggest that the dose–response curve has a “hockey-stick” shape which can only be approximated by allowing the available models to be unconstrained. This is not a standard approach for multiple reasons including the instability of the models and it allows for curve shapes that are not expected to be biologically plausible.
Figure 5a. BMD modeling of the changes in plasma testosterone reported in male Wister rats following inhalation exposure to DEHP (Kurahashi et al. Citation2005).
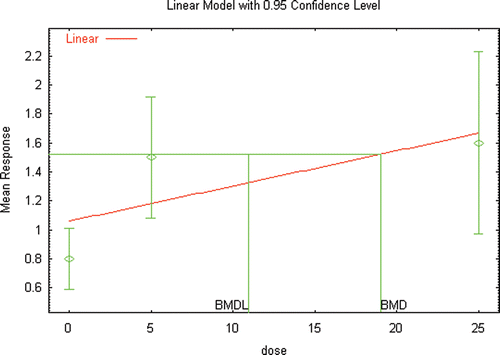
Figure 5b. BMD modeling of the changes in seminal vesicle weight reported in male Wister rats following inhalation exposure to DEHP (Kurahashi et al. Citation2005).
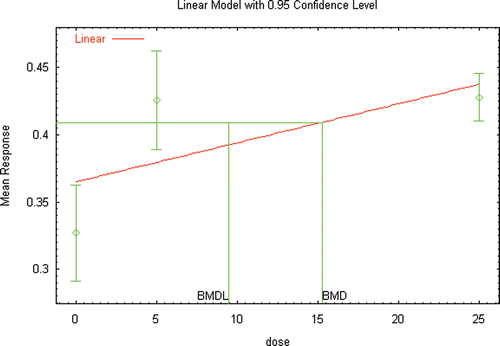
For example, if unconstrained models are applied to the two significantly changed endpoints (plasma testosterone and seminal vesicle weight) reported by Kurahashi et al. (Citation2005) in male rats an acceptable fit can be obtained, and BMDL10s of 0.65 to 0.85 mg/m3 determined. However, as illustrated in and , in order to achieve these acceptable fits, the model derived dose–response curve has an unusual shape, with a large increase followed by a decrease between the two available datapoints from exposed animals. This type of unusual model behavior is typically why models are always constrained, which restricts the model predictions to a single direction (either increasing or decreasing).
Figure 6a. BMD modeling (unconstrained) of the changes in plasma testosterone reported in male Wister rats following inhalation exposure to DEHP (Kurahashi et al. Citation2005).
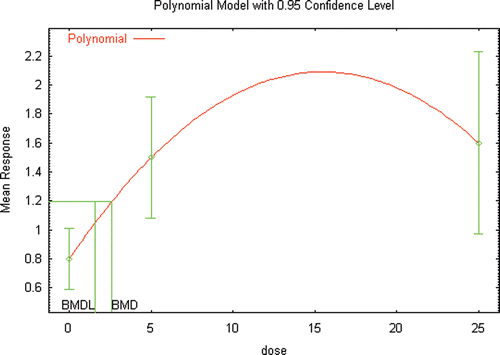
Figure 6b. BMD modeling (unconstrained) of the changes in seminal vesicle weight reported in male Wister rats following inhalation exposure to DEHP (Kurahashi et al. Citation2005).
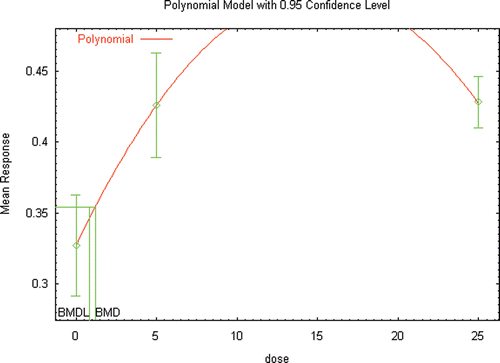
As with the oral data, the available inhalation datasets appear to present challenges in the determination of the dose–response curve in the low-dose region. More information is needed to better characterize the biological pathways involved in the responses observed.
Mathematical modeling for cancer endpoints
Traditionally, based on the original guidelines for cancer risk assessment (USEPA, Citation1986) cancer dose–response modeling was intended to be a statistical exercise to estimate a CSF or UR. This value represents the upper bound of the risk of cancer per unit dose (mg/kg/day) or concentration (mg/m3) and was typically estimated by applying the Multistage model to the dataset. The intent was initially to quantify the risk associated with the most sensitive effect in the most sensitive species. Also because the processes by which cancer developed were assumed not to have a threshold, the shape of the dose–response curve in the low-dose region was assumed to be linear. In addition, no consideration at that time was given to the potential human relevance of an endpoint observed in animals and the intent was to conduct the chronic bioassays with dosing to achieve a Maximum Tolerated Dose (MTD).
About a decade ago, revisions in the guidelines for dose–response modeling for cancer endpoints were initiated, beginning with draft recommendations in 1996 and the guidelines finalized in 2005 (USEPA, Citation2005). The most significant changes in the guidelines were:
The definition of default approaches were considered as “no-data” options, the use of which must be justified based on the lack of sufficient information to support a more chemical-specific approach;
Explicit support for biologically-based modeling as the preferred method for dose–response assessment;
Definition of multiple low-dose extrapolation defaults: linear versus nonlinear; and,
Consideration of the mode of action (carcinogenic mechanism) of the chemical both for determining the conditions under which the chemical should be considered a human carcinogen, and for determining the appropriate low-dose extrapolation approach.
For those endpoints determined to be relevant to human health, mathematical models are applied to estimate a POD, similarly to noncancer dose–response assessments. The POD is the lower bound on the dose at a specified level of risk or increase in response, typically the 10% level, i.e. the LED10 which is analogous to the BMDL10. The same mathematical models as used in the noncancer assessment are now applied to cancer dose–response assessment rather than restricting the analysis to the use of the Multistage model, under the assumption that most models would give similar answers in the observable range of doses. For chemicals assumed to act by a linear, non-threshold mode of action, largely based on positive genotoxicity data, a linear extrapolation to lower risk levels was recommended. For chemicals for which the mode of action was determined to have a threshold or be non-linear at low doses, the POD was then treated as the starting point for the application of UFs in the same manner as derivation of the RfD or RfC.
Weight of evidence for DEHP
As discussed previously, there are at least four studies available in the literature that can be used to characterize the carcinogenic potential of DEHP. These studies have been conducted in two species (mice and rats) and multiple strains of rats (Sprague Dawley, Fischer 344, Long Evans). The main response observed in both species is hepatocellular adenomas and carcinomas, with pancreatic acinar cell adenomas and Leydig cell tumors also observed in rats.
Under the older cancer guidelines, the weight of evidence would have been conducted in a stepwise fashion and included:
Characterization of the evidence from human studies and from animal studies individually,
Combination of the characterizations of these two types of data into an indication of the overall weight of evidence for human carcinogenicity, and
Evaluation of all supporting information to determine if the overall weight of evidence should be modified.
Under the new cancer guidelines (USEPA, Citation2005), emphasis is placed on the importance of weighing all of the evidence in a single integrative step after assessing all of the individual lines of evidence, which is in contrast to the step-wise approach in the 1986 cancer guidelines. Evidence considered can include tumor findings (in humans and animals), chemical and physical properties, structure-activity relationships (SARs), studies addressing potential carcinogenic processes and MOA. In the overall characterization of potential carcinogenicity, data from epidemiologic studies are generally preferred. However, the integration of all of the information discussed above can provide insight into the possible MOAs and the relevance of the observations observed, particularly in animals studies, to human cancer. The revisions to the cancer guidelines were driven by the growing sophistication of research methods, particularly in the growing capability to evaluate effects at the cellular and subcellular levels, as well as increasing knowledge of pharmacokinetic processes.
The major change to the weight of evidence assessment is to now create a hazard narrative to describe the potential carcinogenicity of the chemical using descriptive phrases, such as “likely or not likely to cause cancer in humans under these specified conditions”, rather than the previous “letter” designation, e.g. A, B1, or B2Footnote3 (). The available USEPA IRIS cancer assessment for DEHP, last updated in 1993, classifies DEHP as a B2, probable human carcinogen. This is based on inadequate information in the human and the significant dose-related increases in liver tumor responses in rats and mice of both sexes reported by NTP (NTP, Citation1982).
Table 28. Illustrative Categorization of Evidence Based on Animal and Human Data1
Since this USEPA assessment, several other chronic bioassays have been conducted (David et al., Citation1999; David et al., Citation2000a; David et al., Citation2000b; Voss et al., Citation2005), with the evidence of the carcinogenic potential for DEHP still limited to animal data and based largely on the observation of liver tumors in rodents. A large body of data supports the hypothesis that peroxisome proliferator-induced hepatic peroxisome proliferation, hyperplasia, and neoplasia are responses unique to, or are much more significant in rats and mice. Overall, the rank order of responsiveness appears to be rat ≈ mouse > hamster >> guinea pig > monkey ≈ human (reviewed by Bentley et al., Citation1993). Numerous reviews have been written in the past several years that provide detailed discussions of the responses of rats and mice to PPARα agonists and their general lack of relevance to humans and other primates (Ashby et al., Citation1994; Cattley et al., Citation1998; Doull et al., Citation1999; Klaunig et al., Citation2003; Lai, Citation2004; Rusyn et al., Citation2006; USEPA, Citation2003; Woodyatt et al., Citation1999). As described in these reviews, although humans possess functional PPARα and the human receptor can be activated by peroxisome proliferators, humans and other primates are generally refractory to the key events associated with the induction of liver tumors by PPARα agonists.
Other than liver tumors, the only remaining statistically significant changes reported were increases in the incidence of pancreatic acinar cell adenomas in male rats reported by both David et al. (Citation1999; Citation2000a; Citation2000b) and Voss et al. (Citation2005) and the incidence of Leydig cell tumors in male rats reported by Voss et al. (Citation2005). This “triad” of tumors (liver, pancreas and Leydig cell) all have proposed MOAs that suggest a possible downstream effect resulting from PPARα activation (Klaunig et al., Citation2003). While the available data provide strong evidence that rodent liver tumors following administration of PPARα agonists are not relevant to human health, the justification for the lack of human relevance of the observed Leydig cell and pancreas acinar cell tumors is still questionable.
Based on the available data and applying the new USEPA Cancer Guidelines (USEPA, Citation2005), DEHP could be classified in two ways depending upon which tumors could be justified to be relevant to human health. These include 1) “likely to be carcinogenic to humans” based on the observation of pancreatic acinar cell tumors in multiple strains of rats and/or Leydig cell tumors in one strain of rats or 2) “not likely to be carcinogenic” based on convincing experimental evidence that the only carcinogenic effects observed in animals are not relevant to humans.
The assessment conducted here will proceed assuming the classification is “likely to be carcinogenic to humans” based on the incidence of pancreatic acinar cell and Leydig cell tumors. Mathematical modeling of the incidence of liver tumors will also be provided for comparative purposes, because existing risk assessments in the literature still rely on these endpoints. Therefore, the impact of the application of various guidelines and approaches can be demonstrated.
Dose–response modeling for DEHP cancer endpoints
Without consideration of MOA, dose–response modeling, using the models available in BMDS, was conducted for the tumor incidences that were reported to be statistically significantly increased in the four chronic bioassays, including rodent liver tumors. These included the results reported by NTP (Citation1982) (), David et al. (Citation1999) (), David et al. (Citation2000a; Citation2000b) (), and Voss et al. (Citation2005) (). Using the traditional statistical approach from the 1986 guidelines (USEPA, Citation1986), the lowest animal POD (51.66 mg/kg/day) associated with the best model fit (p = 0.668− Multistage model) was estimated using liver adenomas and carcinomas in female mice from the David et al. (Citation1999) ().
Table 29. BMD modeling results for liver tumors in mice and rats reported by NTP (1982) () using external dose (mg/kg/day) as the relevant dose metric.
Table 30. BMD modeling results for liver tumors as reported by David et al., (1999) () using external dose (mg/kg/day) as the relevant dose metric.
Table 31. BMD modeling results for pancreatic acinar cell and hepatic lesions reported by David et al. (2000a) () using external doses (mg/kg/day) as the relevant dose metric.
Table 32. BMD Modeling results for pancreatic, liver, and Leydig cell lesions reported by Voss et al., (2005) () using external doses (mg/kg/day) as the relevant dose metric.
In the absence of the application of a PBPK model, animal-to-human extrapolation was accomplished by applying the default scaling value of body weight3/4. The justification for this extrapolation approach is provided in USEPA (USEPA, Citation2005):
For oral exposures, administered doses should be scaled from animals to humans on the basis of equivalence of mg/kg3/4-d (milligrams of the agent normalized by the 3/4 power of body weight per day) (USEPA, Citation1992). The 3/4 power is consistent with current science, including empirical data that allow comparison of potencies in humans and animals, and it is also supported by analysis of the allometric variation of key physiological parameters across mammalian species. It is generally more appropriate at low doses, where sources of nonlinearity such as saturation of enzyme activity are less likely to occur. This scaling is intended as an unbiased estimate rather than a conservative one. Equating exposure concentrations in food or water is an alternative version of the same approach, because daily intakes of food or water are approximately proportional to the 3/4 power of body weight. The aim of these cross-species scaling procedures is to estimate administered doses in animals and humans that result in equal lifetime risks.
The resulting human equivalent POD using the dataset of liver adenomas and carcinomas in female mice from the David et al. (Citation1999) is approximately 7.5 mg/kg/day. The corresponding CSF, assuming a linear dose–response curve, can be calculated using the equation 0.1/human equivalent LED10. This step would only be needed if the risk assessor was conducting an assessment that relied upon dose–response assessments provided under older guidelines. Many of the chemical dose–response assessments provided in the Integrated Risk Information System (IRIS) database were conducted prior to the recent revisions to the cancer guidelines and provide results as a CSF or UR, rather than providing a POD.
Consideration of the changes in the new cancer guidelines (USEPA, Citation2005) and the human relevance framework would suggest that liver tumors in rodents should not be used to estimate the potential cancer risk in humans. As discussed previously, it is generally accepted that these tumors in rodents are caused by a PPARα-mediated process (Foster, Citation2006; Klaunig et al., Citation2003). Activation of the PPARα receptor is a well-characterized, nongenotoxic mode of action causally related to the induction of rodent liver tumors and thought to be specific to rodent livers (Ashby et al., Citation1994; Bentley et al., Citation1993; Cattley et al., Citation1998; Chevalier and Roberts, Citation1998; Klaunig et al., Citation2003; USEPA, Citation2003; USEPA, Citation2005). If this information is factored into the cancer dose–response assessment for DEHP, limited evidence of carcinogenic potential is available.
The lowest animal POD estimated for the remaining endpoints (pancreatic acinar cell adenomas and Leydig cell tumors) observed in male rats is approximately 97 mg/kg/day, based on the application of the log-logistic model to the incidence of all Leydig cell tumors from Voss et al.(Citation2005). The PODs estimated based on the incidence of pancreatic acinar cell tumors from the same study was about a factor of two higher (approximately 195 mg/kg/day− Multistage model). While the finding of Leydig cell tumors in Voss et al. (Citation2005) is new, it is not surprising. As discussed previously, a number of other peroxisome proliferators have been reported to induce testicular tumors as well (Klaunig et al., Citation2003). The mechanism by which Leydig cell tumors are induced is different from that in hepatocytes because steroid hormone effects are involved. PPAR agonists are reported to induce Leydig cell tumors by inhibiting testosterone biosynthesis and/or inducing aromatase that in turn increases estradiol levels (Klaunig et al., Citation2003). These results are also in line with changes in LH and testosterone levels and Leydig cell hyperplasia noted in other shorter duration studies (Akingbemi et al., Citation2004; Akingbemi et al., Citation2001; Andrade et al., Citation2006c; Andrade et al., Citation2006b; Andrade et al., Citation2006a; Ge et al., Citation2007a).
Consideration of dosimetry in the dose–response assessment
In the latest cancer guidelines (USEPA, Citation2005), toxicokinetic or physiologically based pharmacokinetic (PBPK) modeling is now the preferred approach for estimating dose to be used in dose–response modeling. Physiologically based pharmacokinetic (PBPK) models have proven to be useful tools for integrating toxicological and pharmacokinetic data and are valuable in that they provide an alternative to use of either an exposure concentration (inhalation) or administered amount (oral) to estimate acceptable levels by incorporating internal dosimetry or target tissue dose metrics to characterize exposure (Barton and Clewell, Citation2000; Clewell and Andersen, Citation1985; Clewell et al., Citation1995).
To the extent to which the structure of a PBPK model includes the important determinants of the kinetics of the chemical, a properly validated PBPK model can be used to perform high-to-low dose, dose-route, and interspecies extrapolations necessary for estimating human equivalent doses based on animal toxicology studies (Andersen et al., Citation1991; Andersen et al., Citation1987; Barton and Clewell, Citation2000; CitationClewell and Andersen, 1985; Clewell et al., Citation2000; Clewell et al., Citation2001a; Clewell and Clewell, Citation2008; Clewell et al., Citation2001b; Corley, Citation1996; Corley et al., Citation1994; Corley et al., Citation1990; el-Masri et al., Citation1995; Fisher, Citation2000; Gerrity and Henry, Citation1990; Johanson and Filser, Citation1993; Mann et al., Citation1996a; Mann et al., Citation1996b; O’Flaherty, Citation1989; Reitz et al., Citation1990). A PBPK model is also useful for examining the effects of changing physiology with age on target tissue dosimetry, as in the case of early life exposure (Barton, Citation2005; Clewell et al., Citation2004; Clewell et al., Citation2001a; Clewell et al., Citation2007; Clewell et al., Citation2001b; Corley et al., Citation2003; Fisher et al., Citation1991; Fisher et al., Citation1989; Gentry et al., Citation2004; Gentry et al., Citation2003; O’Flaherty, Citation1995; Sarangapani et al., Citation2003).
The results of the PBPK model will typically be expressed in units relevant to the target tissue dose, e.g., concentration of chemical per unit of tissue rather than the original exposure concentration or administered dose. To determine the equivalent exposure concentration or administered dose in the human that would result in the estimated target tissue dose, the pharmacokinetic model must be run “backwards”; that is, the model must be run repeatedly, varying the exposure concentration or administered dose until the desired dose metric dose at the target tissue is obtained. The use of PBPK modeling to improve estimation of the POD expressed in human equivalent doses, e.g., mg/kg/day, has been explored with DEHP data, as discussed in the following sections.
The ultimate aim of using PBPK modeling is to provide a measure of dose that better represents the “biologically effective dose” or the dose that causally relates to the adverse outcome. As a result, target tissue dosimetry provided by PBPK modeling can also be an essential component in models of pharmacodynamics, as well as in biologically based dose–response models of cancer (Clewell and Andersen, Citation1989).
Description of the DEHP PBPK models
Several PBPK models have been developed for selected phthalates, including DEHP (Cahill et al., Citation2003; Clewell et al., Citation2008; Keys et al., Citation1999; Keys et al., Citation2000). The most recent phthalate model, developed by Clewell et al. (Citation2008) for DBP, has been extended to the human. The original DBP model by Clewell et al. (Citation2008) described serum, urine, bile, and fecal time-course data for DBP, monobutyl phthalate (MBP), and the glucuronide and oxidative metabolites of MBP across a wide range of doses administered via intravenous injection or oral gavage, and with single or repeated dosing. In order to apply this model to DEHP, very few changes were made to the original model structure. In particular, a more detailed kinetic description of the combined oxidative metabolites of the monoester was required to fit the metabolite excretion data for DEHP.
The final DEHP model contains four inter-connected sub-models, each with the necessary amount of detail to adequately describe the chemical species: diester, monoester, monoester-glucuronide, and the combined oxidative metabolites. The individual sub-models interact at sites of metabolism (hydrolysis of the diester, glucuronidation, hydrolysis of the glucuronide, and oxidation). In addition, each PBPK model requires data on species-specific physiological parameters, such as body weight or organ volumes and blood flow, and species-specific kinetic parameters (Appendix B). The data used to develop the PBPK models for each chemical species in the adult rat and modifications made to describe gestation and lactation are described in detail in Appendix B. The final model structure used to describe disposition of DEHP in the pregnant rat, the more complex of the PBPK models, is shown in .
Figure 7. Model structure for DEHP/DBP kinetics in the pregnant rat. Adult male rat model is identical with the exception of the placenta/fetus compartments. Lactation model has two added compartments the diester and free monoester in the mammary gland and milk. Dashed arrows indicate first order processes. Bold dashed arrows represent saturable processes. Solid arrows represent blood flows to the tissue compartments and are diffusion limited.
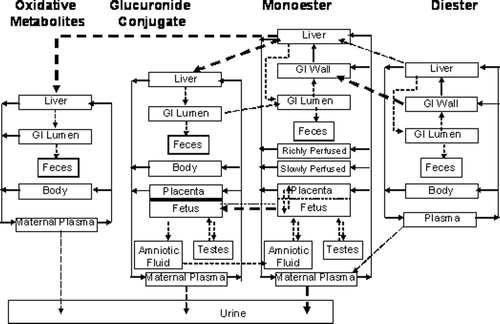
The rodent model was extended to human with known values for the required physiological and kinetic parameters or scaling of the rodent values to estimate human equivalent values. Parameters for adult females are shown in . Additional parameters needed for gestation and lactation are shown in and , respectively. The data used for the key parameters in the human gestation and lactation model ( and , respectively) were derived from the following sources. Equations describing the growth rates and physiological changes in the human maternal compartments during pregnancy were based on the Clewell et al., (Citation1999) PBPK model for methylmercury. For amniotic fluid volume, a Gompertz equation was fit to the measured values published by Beall et al., (Citation2007). The volume of the fetal testes was fit to data published in ICRP (Citation2002) and Payne and Jaffe (Citation1972). Two changes were made to fit the differences in clearance of MEHP between rodents and humans. The urinary excretion of MEHP was changed to clearance (L/h) based on the ratio of the Vmaxc to the Km and the glucuronide conjugation of MEHP was increased to describe the oral ( and ) human data published by Koch et al., (2004; 2005). Hydrolysis of DEHP in the gut and liver and phase I and II metabolism in the liver of the neonate/infant were set to 60% of the adult value as the ontogeny of the metabolic pathways for MEHP are not well characterized. The lactation model was based on the Clewell and Gearhart (Citation2002). The rate of milk production, the residual milk volume in the mammary gland and the volume of milk ingested by the growing infant were set to the average values reported for the nursing mothers and infants up to 1 year of age (USEPA, Citation2002). The volume of the mammary gland was simplified by setting the fraction of body weight to the final fraction as this volume was reached within the first 15 days and did not impact the steady state kinetics when averaged over a 1 year period. In all human PBPK models, DEHP concentration in the fat compartment was set to the arterial concentration of DEHP times the partition coefficient for fat in order to reduce the computational time necessary for the model to reach steady state. to also include values for each of these parameters describing the distribution of values that were subsequently used in a Monte Carlo analysis to determine a distribution of target tissues doses and subsequently, a distribution of estimated external exposure that would correspond to each target tissue dose.
Figure 8. Excretion of DEHP metabolites in urine after a single oral dose in one randomly chosen platelet donor reported by Koch et al. (Citation2005). The estimated dose was approximately 0.048 mg/kg over a 45 min infusion.
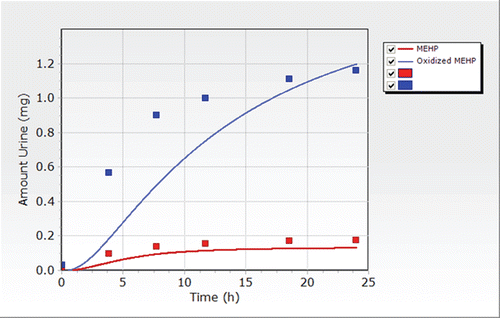
Figure 9. Total amount of MEHP excreted in urine after a single oral dose of 38 mg on buttered toast to a single volunteer (Koch, Citation2004).
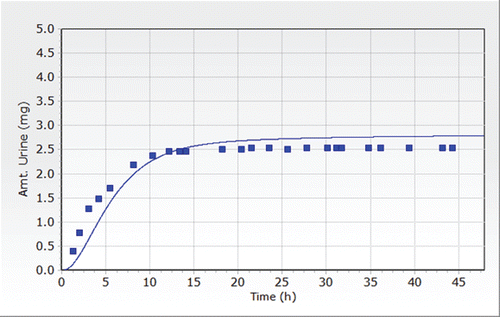
Table 33. Model parameters and distributions used for the DEHP human PBPK model Monte Carlo Analysis.
Table 34. Additional model parameters and distributions used for the DEHP human pregnancy PBPK model Monte Carlo Analysis.
Table 35. Additional model parameters and distributions used for the DEHP human lactation PBPK model Monte Carlo Analysis.
Selection of a dose metric for use in dose–response modeling
An important consideration in describing the relationship between chemical concentration and tissue response is the need to determine the toxicologically active form of the chemical. The selection of the dose metric, that is, the active chemical form for which tissue exposure should be determined and the nature of the measure to be used (e.g., peak concentration (CMAX) or area under the concentration-time profile (AUC)) is the most important step in applying pharmacokinetics in dose–response assessment and should be determined for each critical endpoint for DEHP prior to the initiation of dose–response modeling. For each endpoint, priority should be given to the dose metric that, on the basis of the available evidence, appears to provide most plausible basis for estimating the biologically effective dose. The plausibility of a given dose metric will be determined primarily by two factors: (1) its consistency with available information on the MOA, and (2) the consistency of its dose–response with that of the endpoint of concern.
The specific nature of the relationship between target tissue dose and response should be determined based on the proposed MOA. Many short-term, rapidly-reversible toxic effects, such as acute skin irritation or acute neurological effects may result primarily from the current concentration of the chemical in the tissue. In such cases, the likelihood of toxicity from a particular exposur e scenario can be conservatively estimated by the maximum concentration (CMAX) achieved in the target tissue. On the other hand, the acute toxicity of highly reactive chemicals, as well as many longer-term toxic effects, such as tissue necrosis and cancer, may be cumulative in nature, depending on both the concentration and duration of the exposure. A simple metric for such cases is the area under the concentration curve (AUC) in the tissue, which is defined mathematically as the integral of the concentration over time. This mathematical form implicitly assumes that the effect of the chemical on the tissue is linear over both concentration and time. The use of the AUC represents an extension of “Haber’s Law”, a concept developed from observations of the effects of chemical warfare gases (Haber, Citation1924) that toxicity is proportional to the product of the concentration and time of exposure (C x T). For developmental effects, the chemical time course may also have to be convoluted with the window of susceptibility for a particular gestational event.
In the case of DEHP, evidence suggests that the noncancer effects may be associated with the metabolite MEHP (ATSDR, Citation2002) or some of MEHP’s downstream oxidative metabolites (Koch et al., Citation2005). Koch et al. (Citation2005) demonstrated that secondary metabolites other than DEHP, MEHP may be involved in the observed testicular effects, specifically 5-hydroxy MEHP. However, because of the complex nature of the metabolism of DEHP, estimation and validation of concentrations of metabolites other than MEHP cannot be achieved with the data that are currently available.
For purposes of this assessment and to demonstrate the application of PBPK modeling in risk assessment, one dose metric will be determined and applied. The dose metric selected was the AUC for MEHP, which is representative of the average target tissue dose over the period being considered. Although it is possible the peak concentration could be a relevant dose metric for selected endpoints, use of peak concentration as the dose metric is very sensitive to changes in exposure, requiring more specific information about intake for routes of exposure, such as diet or drinking water. In general, the use of the AUC results in more conservative estimates of acceptable intake and therefore will be used to demonstrate applications of PBPK modeling for this assessment.
Estimation of internal dose metrics for DEHP
The rat model was used to calculate the AUC MEHP corresponding to exposure at different lifestages that were the focus of the toxicity/carcinogenicity studies (). For each dosing scenario from the noncancer reproductive/developmental studies, the AUC for MEHP was estimated for multiple lifestages. These included maternal dosing (), gestation and lactation dosing separate and combined (), postnatal dosing (), and multigenerational dosing (). These dose metrics were estimated to investigate whether the observed adverse effects may be attributed to exposure during various windows of development. The model was run using a daily dosing regimen for the amount of time described in the study and a total area under the curve (AUC) for MEHP was estimated. The AUC was then divided by the total amount of time for which the simulation was run to obtain an average daily concentration in the non-pregnant, pregnant, lactating, fetal or neonatal rat. For dosing scenarios that included both gestation and lactation, the gestation model was run to the time of birth and final maternal and fetal tissue concentrations were tabulated and used as starting tissue concentrations in the maternal and neonatal models. AUC for combined exposures across lifestages (i.e., gestation, lactation) were estimated by adding the gestation AUC and lactation AUC and dividing by the total exposure time. For cancer effects, the AUC MEHP was estimated over the lifetime of the rat ().
Table 36. Pregnancy and lactation dose metrics for the rat estimated applying the PBPK model.
Table 37. Postnatal dose metrics in the rat estimated applying the PBPK model.
Table 38. Dose metrics estimated for the multigenerational study conducted by rats by NTP (Citation2004).
Table 39. Dose metrics for lifetime dietary administration of DEHP in rats estimated using the PBPK model.
Based on the oral gavage studies reported by Andrade and coworkers (), the model estimates of the AUC for MEHP, comparing fetal blood AUC to pup blood AUC, suggested that exposure to the fetus during gestation is much greater than that resulting from lactation alone. The postnatal gavage doses tested (1 to 750 mg/kg/day) over various windows of development resulted in AUCs () that were comparable to those estimated during gestation.
Use of a dose metrics in noncancer dose–response assessment
PBPK modeling is typically used to develop internal dose metrics to be used in the dose–response modeling assessment, replacing the use of external exposure concentrations or administered doses. The main goal in applying these types of models is to adjust for species differences in pharmacokinetics that can impact the delivery of the ultimate active chemical to the target tissue. The application of these models in dose–response assessment replaces the application of a portion of the UF associated with the pharmacokinetic differences in animal-to-human extrapolation. However, as demonstrated in the following sections, the results from this type of modeling can be used in numerous ways to provide a better understanding of the contribution of target tissue dose delivery in understanding the potential MOA for an endpoint of concern.
For purposes of estimating a POD for DEHP, the use of the AUC for MEHP from the rat models were incorporated into the dose–response assessment as the “dose metric”, replacing the use of administered gavage or dietary exposure doses. The same dose–response models described previously were used. Typically, if the observed response is closely related to the internal dose of a parent compound or a specific metabolite, the use of this dose metric in the application of benchmark models can result in a better fit. However, in the case of DEHP, the use of AUC for MEHP as the dose metric improved the fit of the dose response models to the data for some data sets, but for many datasets, the fit was still unacceptable (). Because of the lack of improvement in the fit of the dose–response models to the the data when PBPK-derived doses from the animal model were used, it was assumed that improvement of the fit using the human model would be unlikely.
Table 40. BMD modeling results for selected noncancer endpoints incorporated dose metrics estimated using the PBPK model.
These results for DEHP, that is, the fit of the dose response models to the noncancer data sets and the lack of appreciable change in estimates of BMDs and BMDLS suggested that while kinetics may contribute somewhat to the observed dose–response behavior, the observed reproductive/developmental effects are likely more closely related to pharmacodynamics and to exposure to the animals during critical windows of development.
Use of a dose metrics in cancer risk assessment
As was conducted with the noncancer endpoints, internal dose metrics can be incorporated into the dose–response assessment for cancer endpoints. Using the Voss et al. (Citation2005), BMD modeling was conducted using AUC MEHP as the dose metric in conjunction with all three cancer endpoints of interest (liver, pancreatic acinar cell and Leydig cell tumors) (). Unlike many of the noncancer endpoints, adequate fits of multiple dose–response models were achieved for most of the cancer endpoints using external dietary concentrations expressed in mg/kg/day as the dose metric for BMD modeling.
Table 41. BMD modeling results for cancer endpoints from Voss et al., (2005) incorporating dose metrics estimated using a PBPK model.
In comparing the fit of the data in the dose response models using the experimentally administered dose to estimate the human external dose, e.g., dietary dose, to that derived using the human PBPK model, it does not appear that consideration of the pharmacokinetics of DEHP significantly impacted the fit of the dose–response models to the cancer data. For example, the animal POD based on the application of the log-logistic model to the incidence of all Leydig cell tumors from Voss et al. (Citation2005) (97 mg/kg/day) (), results in a human equivalent POD approximately 26 mg/kg/day by applying a body weight3/4 animal-to-human extrapolation factor. The incorporation of the dose-metric AUC MEHP did not significantly affect fit of the dose response model (p = 0.948 using external concentrations versus p = 0.884 using AUC MEHP). Application of the human PBPK model to estimate the external dose associated with the POD of approximately 2 mg/L blood of MEHP () resulted in an external concentration of approximately 22 mg/kg/day, assuming chronic exposure in the human. Comparison of these human equivalent PODs (26 versus 22 mg/kg/day), which are less than a factor of 2 different, suggested that the application of default approaches to account for animal-to-human differences in pharmacokinetics and pharmacodynamics are appropriate in the case of DEHP. This may not be applicable to all compounds, but could be investigated by the risk assessor, if the appropriate models and data are available.
Route-to-route comparison
Incorporation of PBPK modeling into the dose–response assessment not only allows for conducting dose–response modeling with an internal dose metric, rather than external exposure, but also allows comparison from route-to-route, based on an internal target tissue concentration or a surrogate, such as blood concentration of parent or metabolite. In the case of DEHP, limited inhalation data were available. The studies conducted by Ma et al. (Citation2006) and Kurahashi et al. (Citation2005) provided data for route-to-route comparisons in the animal.
Application of a multi-route PBPK model allows the risk assessor to compare the internal dose metrics across routes of exposure and estimate external concentrations in like units (i.e., express external concentrations for an inhalation study in mg/kg/day oral dose), rather than applying standard unit conversions. For example, comparison of dose metrics predicted for inhalation and oral gavage studies in which animals were exposed postnatally (), with comparable periods of exposure (PND 21–41 or 22–84), suggested that blood concentrations following administration of 5 mg/m3 in air, using the exposure protocols applied by Ma et al. (Citation2006) and Kurahashi et al. (Citation2005), are comparable to concentrations following administration of approximately 1 mg/kg/day via gavage, as administered by Akingbemi et al., (Citation2001). These modeling results also suggested that exposure to an air concentration of 25 mg/m3 would be comparable to 10 mg/kg/day using these same exposure protocols in the animal. These types of comparisons across routes may be important to the risk assessor to integrate data related to a potential MOA, especially if information across routes may be used to fill data gaps.
Integration of Data
As discussed previously, traditional risk assessment has relied mainly on a single endpoint in a single species to define the potential for adverse events in a population of interest. However, as guidelines have evolved, a new “vision” of risk assessment is emerging that suggests not only an expansion of the types of data to be considered when making risk assessment decisions, but also is moving towards harmonizing cancer and noncancer assessments (USEPA, Citation2005). The proposed approach focuses on an understanding of background disease processes and chemical exposures (NAS, Citation2007) and the MOAs that may affect a chemical’s dose–response relationship in humans (NAS, Citation2009). The intent is to move away from whole animal testing, focusing on cellular events in the human and to the potential impact of perturbations at the cellular level on the development of disease (NAS, Citation2009). Therefore, the identification of dose-related transitions at the cellular levels and concentrations that result in biological perturbations that may lead to adverse effects becomes a critical component in making decisions in risk assessment.
This integration of data has not only been outlined in guidelines as it related to a single compound, but also for multiple compounds that may have similar adverse effects. In the recent report by NAS (Citation2008) on Phthalates and Cumulative Risk Assessment, the committee strongly recommended chemicals that cause common adverse outcomes be grouped for consideration and not focused exclusively on structural similarity or on similar mechanisms of action.
With these proposed changes in how risk assessment is approached by the assessor, it becomes increasingly important to integrate the information provided by the available data, rather than focusing on individual endpoints from individual studies. One approach to integrate the available noncancer toxicological data for DEHP would be to consider the combined results from multiple reproductive/developmental studies.
When considering reproductive/developmental effects, there are multiple windows of development that may provide opportunities for a chemical to elicit adverse effects in the offspring. In the case of DEHP, recent studies have focused on the evaluation of three potential windows of exposure: prenatal, postnatal and multigenerational. These studies provide a wealth of information on a variety of endpoints across a wide range of exposure concentrations and multiple routes of exposure. While quantitative approaches with increasing complexity can be incorporated into a risk assessment, when sufficient data are available, other approaches can also be applied, which appear simplistic in nature, but can allow the risk assessor to readily see patterns within and across studies that can inform potential MOA, as well as the shape of the dose–response curve. A more simplistic approach may be preferable, especially for a database, such as that for DEHP with multiple exposure periods, time points for measurement of change, and a large number of potentially interrelated biological endpoints.
As an example, while the results of the available studies suggest that the prenatal period may be the most sensitive, based on effects in male rats, it could be difficult to select a NOAEL/LOAEL or apply the BMD method to an individual endpoint. Especially when the shape of the dose–response curves are nonmonotonic, as is demonstrated with some of the endpoints considered for DEHP as part of this evaluation.
The studies conducted during the prenatal period through PD20 (Andrade et al., Citation2006c; Andrade et al., Citation2006b; Andrade et al., Citation2006a; Grande et al., Citation2006; Grande et al., Citation2007), allow evaluation of the results for multiple endpoints at multiple time points in both male and female rats following gavage administration of a wide range of DEHP doses (0.015−405 mg/kg/day). When evaluating the changes across endpoints, a pattern emerges of transitional changes in multiple endpoints in the male rat from the lowest dose tested (0.015 mg/kg/day gavage) to doses greater than 500 mg/kg/day (). When focusing on doses of 10 mg/kg/day and lower, the endpoints (i.e., increased anogenital distance, decreased sperm production, increased testes weight) observed in the studies in which DEHP was administered by gavage are consistent with an increase in testosterone (Andrade et al. Citation2006c; Ge et al. Citation2007a). However, as dose increases a transition from effects that were consistent with an increase in testosterone to effects that were consistent with a decrease in testosterone is observed.
Table 42. Integration of noncancer effects by administered dose of DEHP.
In the studies in which DEHP was administered postnatally either via gavage (Akingbemi et al., Citation2004; Akingbemi et al., Citation2001; Ge et al., Citation2007a) or via inhalation (Kurahashi et al., Citation2005; Ma et al., Citation2006), a similar pattern in dose-dependent transitions was observed. Ge et al., (Citation2007b) have suggested a “biphasic” response following gavage administration of DEHP (an increase or a decrease depending upon duration of exposure). However, Noriega et al. (Citation2009) recently investigated the potential non-monotonic nature of the shape of the dose–response curve for DEHP and effects in prepubertal male rats following administration of gavage doses from 10 to 900 mg/kg/day. The results of Noriega et al. (Citation2009) study, conducted in both male Sprague-Dawley and Long-Evans rats did not demonstrate a “biphasic” dose–response curve. What becomes important for the risk assessor in evaluating data when there are conflicting data and interpretations, e.g., whether or not there is a “biphasic” effect, is to focus on the relevance of the various endpoints and exposure methods to humans. An important observation, from a risk assessment perspective, emerges from this exercise of integrating the available noncancer data for DEHP. That is, while there is evidence of a dose-dependent transition in effects associated with changes in testosterone following both gavage and inhalation exposure to DEHP, no similar pattern in responses is observed in the Noriega et al. (Citation2009) or multigenerational study conducted by NTP (Citation2004). The multigenerational study is the only reproductive/developmental study considered as part of this case study in which DEHP was administered in the diet over the entire life cycle, the most relevant route of exposure for humans. It would be expected that the risk assessor would have more confidence in the results of the dietary study than those studies in which DEHP was administered by gavage, because it is the more relevant route of exposure in humans.
Another approach that could be used to integrate the available noncancer data would be to compare the PODs identified from the data by incorporating the confidence in the POD, based on both the study design and relevance to human health. This approach allows the risk assessor to compare the PODs across endpoints within the observable range of the data without the application of default UFs. In the case of the studies considered for DEHP, because of the inability of dose–response models typically applied in risk assessment (i.e., Multistage, Log-Logistic) to adequately fit the dose–response data for many of the noncancer endpoints for DEHP, the NOAELs and LOAELs can be compared visually by plotting on a graph (). The bolder lines in are the NOAELs and LOAELs associated with the NTP (Citation2004) multigenerational study, which based on route of administration and duration of exposure for this case study would be most relevant to human health. The dashed lines in the lower dose region of are the NOAELs and LOAELs based on statistical analyses of the Andrade et al., (Andrade et al., Citation2006c; Andrade et al., Citation2006b; Andrade et al., Citation2006a) studies, but have questionable relevance to human health (IPCS Citation2005); therefore, the risk assessor is unlikely to place high confidence in these endpoints. The medium weight lines are the NOAELs/LOAELS reported in the postnatal studies. While these endpoints have potentially more relevance to human health, the confidence would not be considered as high as the results from NTP (Citation2004) when comparing gavage versus dietary exposure, as well as comparison of chronic multigenerational to bolus gavage dosing during potential critical windows of exposure.
This type of approach can also be applied to the PODs for cancer endpoints (). In , the dashed lines represent the PODs associated with liver tumors, which are the most questionable for predicting human health. Because the relevance of pancreatic acinar cell and Leydig cell tumors (all benign) to human health is still uncertain but currently considered more relevant than rodent liver tumors, the lines associated with the PODs for these tumors is a line, rather than a dashed line.
This approach then allows the risk assessor to integrate the PODs for cancer and noncancer by visually inspecting the relationship among the confidence lines placed on the individual studies and endpoints within a study (). In the case of DEHP, the lowest POD, considering both noncancer and cancer endpoints, is a NOAEL for 50 mg/kg/day associated with the multigenerational study conducted by NTP (Citation2004). The next POD is associated with the BMDL of 97 mg/kg/day is estimated based on the incidence of Leydig cell tumors in the male rat, reported by Voss et al. (Citation2005). This type of graphical integration allows the risk assessor to easily see the relationship between noncancer and cancer PODs. Considering MOA, in combination with the relationship between PODs, would assist the risk assessor in making decisions as to whether a single POD could be relied upon in the determination of a Reference Dose or Concentration that would be protective for both noncancer and cancer endpoints.
As discussed previously, there are several MOAs presented for the noncancer and cancer endpoints observed in animals following exposure to DEHP. However, there are key events in common, such as receptor-mediated events (PPARα activation) that could be initiated events in the development of both noncancer and cancer endpoints. This type of evidence may be relied upon to justify the harmonization of endpoints, allowing the risk assessor to select one POD that could be used in decision-making related to the potential for human health effects.
These approaches for combining information demonstrate that the integration of all the available toxicological data is critical to understanding the potential relationship between responses and exposure and predictions of human health outcomes, and aid in combining what is known regarding the biological processes that may be impacted following chemical exposure. The traditional method of reviewing studies and individual datasets within studies in isolation can be misleading and result in reliance upon an endpoint that may not be relevant to human health or may not be as protective as necessary
While these approaches do not include application of complex quantitative models, this type of integration across studies can provide a form of “biologically based dose–response” assessment that is highly informative to a risk assessor, without required knowledge of complex mathematical approaches. It also points out that consideration of those routes of exposure relevant to human populations must be given highest priority.
Exposure Assessment/Biological Monitoring
A standard exposure assessment is typically an attempt to quantify the amount of chemical to which individuals may be exposed under specific conditions by defining the intensity, frequency and duration of that exposure. In the case of DEHP, the availability of human monitoring data from the NHANES (CDC, Citation2005) study, in combination with availability of a PBPK model, provides the risk assessor a unique opportunity to compare a POD to an external concentration associated with measured urine concentrations in the general population. This comparison could then be used instead of a standard exposure assessment that often contains numerous assumptions, and therefore uncertainties, related to characterizing exposure in the general population. Understanding human biomonitoring results in the context of risk is necessary for risk-based decision making. Without a risk context, biomonitoring results can cause alarm, foster precautionary actions, product de-selection and calls for legislative/regulatory actions. In particular, identification of chemicals in the blood of adults can raise concern for effects of early life exposure, but biomonitoring data is seldom available for this period.
NHANES II and III (CDC, Citation2005) reported levels of MEHP as well as mono-(2-ethyl-5-oxohexyl) phthalate (MEOHP) and mono-(2-ethyl-5-hydroxyhexyl) phthalate (MEHHP) in urine. For the primary metabolite, MEHP, levels in urine for NHANES III were 4.1 ng/ml (median) with a 95th percentile of 38.9 ng/ml for all subjects aged 6 and older (n = 2782). These values were slightly higher than the values reported in NHANES II, which were 3.2 ng/ml (median) with a 95th percentile of 23.8 ng/ml. For MEOHP and MEHHP, both secondary metabolites of DEHP (measured for NHANES III only), the levels were somewhat higher than MEHP with a median of 20.1 ng/ml and 14.0 ng/ml and 95th percentiles of 192 and 120 ng/ml, respectively. Similar urine levels of MEHP were reported in a small sample of Germans (Fromme et al., Citation2007) where median levels of 4.0 and 6.4 ng/ml were reported for male (n = 23) and female (n = 27) subjects. The 95th percentiles were 12.8 and 9.1 ng/ml for males and females, respectively. Koch et al. (Citation2003) reported the concentration of several DEHP metabolites in the first urine void of the day, including MEHP, MEOHP and MEHHP, in 85 German subjects. The median concentrations were somewhat higher in first voids with MEHP being 10.3 ng/ml, MEOHP being 36.5 ng/ml and MEHHP being 46.8 ng/ml. The 95th percentiles were 37.9, 224 and 156 ng/ml, respectively, for MEHP, MEOHP and MEHHP. A retrospective study of phthalate biomonitoring data collected over the last two decades (Wittassek et al., Citation2007) showed nearly 50% decline in urinary MEHP, MEOHP and MEHHP concentrations from 1988 to 2003 with median MEHP concentrations falling from 9.4 ng/ml to 4.6 ng/ml.
To provide an estimate of the distribution of ingestion rates in a population generated using the PBPK adult model that could be associated with the published NHANES biomonitoring data, probability distributions for each model parameter were determined from the literature and used in a Monte Carlo analysis. The parameter distributions used in this Monte Carlo analysis for DEHP, expressed as means and coefficients of variation (CV ± standard deviation/mean), are defined in . The standard deviations for the physiological parameters were based on the previously published Clewell model for methylmercury (Clewell et al., Citation1999). In most cases, the means of the distributions were the parameter values identified during the development and validation of the model described above. Normal distributions were used for plasma flows and tissue volumes, while lognormal distributions were used for partition coefficients and kinetic parameters. To avoid physiologically implausible values, the distributions were truncated at three standard deviations of the mean.
To perform the Monte Carlo simulation for reverse dosimetry based on the population data published in NHANES III, the human model was employed without pregnancy models. The probability distributions for each of the PBPK model parameters were repeatedly sampled, and the PBPK model was run using each chosen set of parameter values. Random sampling was performed in acslX (ver. 2.4, Aegis Technologies). It was determined that 1000 iterations were adequate to ensure the reproducibility of the mean and standard deviation of the output distributions as well as the 50th through 95th percentiles. The output of the Monte Carlo simulation was a distribution of urine MEHP concentrations (average total MEHP including free and conjugated). Coupled with the Monte Carlo analysis, reverse dosimetry was employed to estimate the distribution in exposures associated with the urinary MEHP biomonitoring data for women reported in NHANES III (CDC, Citation2005) based on the methodology previously published by Tan et al. (Citation2007; Citation2006). Briefly, to estimate external exposures consistent with the measured biomonitoring data, the Monte Carlo version of the PBPK model was used to predict the distribution of urinary MEHP concentrations given a series of continuous time-weighted average exposures. The output distribution was then inverted to obtain a distribution of an ‘‘exposure conversion factor’’ (ECF) in units of (µg/kg/day DEHP intake) per (µg/L MEHP in urine). The distribution of ECFs can then be multiplied by the distribution in the measured biomonitoring data to estimate a distribution of DEHP intakes per day. Exposures ranging from 0.001 to 91.2 µg/kg/day DEHP intake in increments of 100.13 were necessary to reproduce the reported distribution of MEHP concentrations in urine including the 50th and 95 percentiles. Simulations were run for 1000 h to ensure steady state conditions and then the average concentration in urine was calculated for another 336 h.
The results from the reverse dosimetry with the human DEHP PBPK Monte Carlo simulations are shown in and . The estimated median exposure was 1.45 µg/kg/day, with 5th and 95th percentiles of 0.13 and 20.4 µg/kg/day.
Figure 13. Cumulative distribution of exposure to DEHP in females aged 7 to 75 based on reverse dosimetry of the biomonitoring data published in NHANES III.
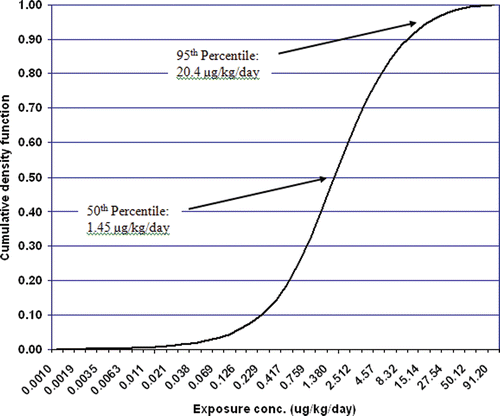
Table 43. Exposure distribution for females aged 7 to 75 as determined from reverse dosimetry using the DEHP PBPK model.
Risk Characterization/Margin of Exposure
The risk characterization step of a standard risk assessment combines the exposure and dose–response assessments and estimates the potential for an adverse health effect under various conditions of human exposure described in the exposure assessment. The overall risk characterization lets the risk manager and others know why an agency or individual assessed the risk in a particular way (USEPA, Citation2000b), Particularly in terms of the available data and its analysis, uncertainties, alternative analyses, and the choices made. A good risk characterization will restate the scope of the assessment, express results clearly, articulate major assumptions and uncertainties, identify reasonable alternative interpretations, and separate scientific conclusions from policy judgments.
USEPA (Citation2000b) guidelines indicate that, while it is generally preferred that quantitative uncertainty analyses are used in each risk characterization, there is no single recognized guidance that currently exists on how to conduct an uncertainty analysis. Nonetheless, risk assessors should perform an uncertainty analysis. Even if the results are arrived at subjectively, they will still be of great value to a risk manager. The uncertainty analysis should, in theory, address all aspects of a human health risk assessment, including hazard identification, dose–response assessment, and exposure assessment. Uncertainty analysis should not be restricted to discussions of precision and accuracy, but should include such issues as data gaps and models. One purpose is to identify those uncertainties that if reduced (e.g., about whether or not we know if the agent causes cancer, about whether or not we know what happens at low doses, that we know the exposure only occurs in certain specific locations) or the policy choices and management decisions that if changed would make a real impact on the risk assessment.
Traditionally, exposure assessments are based on the concentration of a chemical in a particular media, such as drinking water or food. These concentrations are adjusted by exposure patterns and durations and compared to an acceptable concentration, such as the RfC, for the risk characterization portion of a risk/hazard assessment. However, in the case of DEHP, biomonitoring studies have been conducted in which DEHP was measured in human urine (CDC, Citation2005). This, in combination with the application of a PBPK model, allowed for comparison of external concentrations associated with the distributions of urinary concentrations to the PODs that have been determined as part of the standard dose–response modeling. The application of a Monte Carlo analysis in conjunction with the PBPK model to estimate external concentrations allows the risk assessor to consider the potential variability in intake values that may be associated with the distribution of concentrations in biological media.
When evaluating the integration of PODs for noncancer and cancer for which the risk assessor would have high confidence (), the lowest POD (50 mg/kg/day) was associated with the NOAEL for changes in age at preputial separation reported by NTP (Citation2004) following multigenerational dietary exposure to DEHP. The next highest POD was 97 mg/kg/day, which is the BMDL associated with the incidence of Leydig cell tumors reported by Voss et al. (Citation2005), also following dietary exposure to DEHP.
The results of the reverse dosimetry of the NHANES (CDC, Citation2005) study suggest that exposures to DEHP in the general population would have a median value of 1.45 µg/kg/day, with 5th and 95th percentiles of 0.13 and 20.4 µg/kg/day. When compared to the lowest noncancer POD (50 mg/kg/day) a Margin of Exposure (MOE) would be approximately 27000 at the median and approximately 2500 at the 95th percentile. For the lowest cancer POD (97 mg/kg/day), a MOE of approximately 52000 at the median and approximately 4800 at the 95th percentile could be estimated.
Knowledge of these MOEs, outside of the application of UFs, can help a risk assessor in making decisions related to the relationship between concentrations that are known in animals to result in adverse events and those concentrations representative of potential human exposure, as well as the need for and the magnitude of UFs. It provides insights on whether the application of the typical default UF for noncancer effects or the application of low-dose extrapolation for cancer effects would suggest a significant portion of the population would be expected to have a potential for adverse events, depending upon the MOA of the compound of interest. The MOEs developed as part of this assessment suggest a decreased concern for effects due to the magnitude of the MOEs.
8.0 Discussion
Over the past decades, advances have been made in the scientific methods and approaches now considered in the field of human health risk assessment. Research technologies have progressed to provide more accurate and reliable information that can be considered in risk assessment. This, in combination with significant revisions to the standard guidelines for both noncancer and cancer risk assessment, allow for the integration of more biological information into the risk assessment process than in the “traditional” risk assessment approaches.
The objective of this case study for DEHP was to incorporate innovative approaches into each step of the risk assessment process. DEHP is a “data rich” compound in the class of phthalate compounds. It has been well studied in animals following oral exposure; however, there is limited information in humans. DEHP was selected as the demonstration compound for this case study because the knowledge base on what effects result from exposure to this compound is well characterized and the potential modes of action for some endpoints have been hypothesized. The selection of a chemical with this type of database would allow for the incorporation of advanced methods into the risk assessment paradigm without significantly increasing uncertainty.
The initial expectations were that the available data for DEHP would lend themselves to incorporation of new quantitative approaches that would improve on the NOAEL approach and even the BMD approach, especially consideration of PBPK modeling in defining target tissue doses and corresponding external doses that could be used as PODs.
As this case study proceeded, several challenges were encountered that did not advance the analyses in the manner expected, in particular, the application of certain mathematical modeling approaches that are becoming a standard approach in current risk assessments: BMD models and PBPK models. Several lessons can be learned from this case study regarding current guidelines for risk assessment and the challenges that risk assessors face.
First, it is generally considered that the incorporation of mathematical models, with increasing complexity, is often needed to adequately characterize dose–response relationships. This case study demonstrated that statistical dose–response and complex PBPK models do not always improve dose–response assessments above the older NOAEL approach when the experimental data is also complex, presents conflicting or unexplained results, e.g., the biphasic responses for reproductive/developmental milestones, or the MOA is not or only partially understood.
This case study further demonstrated that simplistic approaches (e.g. –) that allow for integration of all the available toxicological data can be critical to understanding the biological processes being impacted. In particular, these types of approaches may highlight the interplay between events that may be related to a proposed MOA for the effects observed following chemical exposure. This type of integration of data can assist in the identification of dose-dependent transitions in effects and provide a form of “biologically based dose–response” assessment without the incorporation of complex mathematical approaches. This is not to suggest that mathematical approaches, especially incorporation of pharmacokinetic processes through the use of PBPK modeling, are not valuable; however, differences in pharmacokinetics are not always the critical factor for the use of animal data to be relevant and predictive of human health outcomes from exposure to the specific chemical.
This case study also highlighted issues that may develop if researchers focus on endpoints that may not be relevant to human health (i.e., the effects observed following gavage administration of DEHP) or if too much emphasis is placed on the review of individual studies and individual datasets (i.e., trying to select a POD based on the available NOAELs in isolation). This is reflected in the question a risk assessor would face with DEHP of whether or not biphasic effects are observed in animal studies following gavage dosing or inhalation exposure. While there are multiple studies demonstrating this effect (Andrade et al., Citation2006a; Ge et al., Citation2007a; Kurahashi et al., Citation2005; Ma et al., Citation2006) and others that do not (Noriega et al., Citation2009), the study that is probably most relevant to human health, a multigenerational dietary study in rats, shows no evidence of such an effect. Therefore, consideration of those routes of exposure most relevant to human populations and for those endpoints that are biologically relevant to human health must be given highest priority when interpreting toxicological data for risk assessment purposes.
This case study encountered some unusual dose–response relationships that were difficult for standard dose–response models to capture. It is likely that the issues encountered as part of this exercise are not limited to DEHP, but are also important for other receptor-mediated compounds or compounds that “perturb” biological pathways, such as endocrine active compounds. What is significant about several of the approaches applied in this case study to integrate data is that they do not necessarily require the identification of a single or specific MOA to justify the shape of the low-dose region or a threshold, but integrate the data in a manner that the shape of the dose–response curve is described by all of the available data. While in most cases the MOA cannot be determined with absolute certainty, the weight of the evidence must be considered.
With the new NAS vision (NAS, Citation2007; NAS, Citation2009) and the guidance towards incorporating more biological considerations in the risk assessment process, as demonstrated by the cumulative risk guidelines and proposed new toxicity testing (i.e., genomics, biological perturbations), the paradigm for risk assessment and toxicity testing is shifting from a “top-down” approach to a “bottom-up” approach. This approach demands that the risk assessment not only understand the tools typically applied in risk assessment, but also the underlying biological processes involved in the endpoint of interest.
As demonstrated in the case of DEHP, data supporting the initiating event involved in the endpoints of interest is needed to enable the risk assessor not only to integrate the toxicological data, both noncancer and cancer, but also understand the relationships among endpoints and the possible shape of the dose–response curve in the low dose region of interest for human exposures. In the case of DEHP, the available data suggested a relationship between the observed responses in animals, both cancer and noncancer, and changes in testosterone for both noncancer and cancer endpoints. However, it is also evident that there are significant data gaps that prevent the risk assessor from drawing firm conclusions.
DEHP is a well-studied compound with a rich data base of in vivo studies conducted in animals. It presents a selected set of challenges often faced by risk assessors in applying the “top down” approach. However, with the move towards the “bottom up” approach, which focuses more on changes at the cellular or gene level, risk assessors will face a new set of challenges. The traditional approaches that have been applied in risk assessment are not easily applied to a wealth of information that is currently being generated for rapid screening or high throughput.
The scientific and risk assessment community has embraced the integration of MOA information into the risk assessment process, as is evident in the latest USEPA cancer guidelines (USEPA, Citation2005) and the IPCS (Citation2005) guidelines, as well as recent risk assessments conducted by the Office of Research and Development (e.g. EGME) and the Office of Water and the Office of Pesticide Programs (e.g. DMA) within the USEPA. However, the consideration of MOA remains mostly a decision point in selecting a method for low-dose extrapolation (i.e., linear versus nonlinear). The incorporation of biological information into the risk assessment process continues to be difficult due to the rigidity of the methods currently embraced by regulatory agencies.
Value of information is missing from most of the current risk assessments being conducted. While most risk assessments point out the uncertainties or concerns in the use of the available data, little thought is given to the key data that would change the risk assessment and reduce uncertainty. Identifying those data that would result in a different “number” is critical, especially if those data refine an understanding of the underlying biological processes and, therefore, decrease the uncertainty in the risk assessment outcome. While scientists engaged in research can certainly make recommendations regarding data generation, these scientists are often too engaged in a specific area or experimental method. It becomes the duty of the risk assessor to provide the recommendations on what data are truly needed to improve the risk assessment for a compound of interest. Communication between the risk assessor and the research community becomes a critical component of the risk assessment process if research is truly conducted to improve our understanding of the potential for adverse events in both the general population and sensitive subpopulations.
The challenges, such as those demonstrated by the DEHP case study, should encourage communication between risk assessors and researchers to answer the questions,
What are the residual concerns?
What are the major data gaps?
What studies are needed?
What research will best inform the next risk assessment conducted for this compound?
The answers to these types of questions will move the risk assessment for individual chemicals, as well as the risk assessment paradigm, forward towards realizing the NAS vision.
Declaration of interest
This paper was prepared with financial support to ENVIRON International Corporation, a private consulting firm, and The Hamner Institutes for Health Sciences by the American Chemistry Council Long-Term Research Initiative. The work reported in the paper was conducted during the normal course of employment. The authors have the sole responsibility for the writing and contents of this paper.
Appendix A
Continuous Models
All the continuous models were run using a BMR of one standard deviation.
Hill model
Parameters:
μ(d) is the mean value of the response at dose d,
γ is the background response value,
k is the slope,
n is the power (restricted to >= 1), and
ν indicates the sign or direction of the change in response with increased dose.
Linear Model
Parameters:
μ(d) is the mean value of the response at dose d,
β0 is the background response value, and
β1 is the linear coefficient or slope.
Polynomial Model
Parameters:
μ(d) is the mean value of the response at dose d,
β0 is the background response value,
β1…βk are the polynomial coefficients, and
k is the polynomial degree.
Note that the coefficients are for the polynomial are restricted to all non-negative or all non-positive (depending on the direction of change of the response with increasing dose) to prevent wavy dose response curves.
Power Model
Parameters:
μ(d) is the mean value of the response at dose d,
γ is the background response value, and
β is the slope, and
α is the power term (restricted to > = 1 to prevent supralinear curves where the dose–response curve has an infinite slope as the dose approaches zero).
Dichotomous (Quantal) Models
All dichotomous models were run using a BMR of 0.1 (10%) extra risk.
Gamma Model
Parameters:
P(d) is the probability of the response occurring at dose d,
γ is the background probability value,
β is the slope, and
α is the power term (restricted to >= 1).
Logistic model
Parameters:
P(d) is the probability of the response occurring at dose d,
γ is the background probability value, and
β is the slope.
Logistic model
Parameters:
P(d) is the probability of the response occurring at dose d,
is the background probability value, and
β is the slope.
Log-Logistic model
Parameters:
P(d) is the probability of the response occurring at dose d,
γ is the background probability value, and
β is the slope.
Multistage Model
Parameters:
P(d) is the probability of the response occurring at dose d,
γ is the background response value,
β1…βk are the polynomial coefficients (restricted to be non-negative), and
k is the polynomial degree.
Probit model
Parameters:
P(d) is the probability of the response occurring at dose d,
Φ is the normal distribution function,
Φ (α) is the background probability value, and
β is the slope.
Log-Probit model
Parameters:
P(d) is the probability of the response occurring at dose d,
Φ is the normal distribution function,
γ is the background probability value,
α is the intercept, and
β is the slope (restricted to >= 1).
Weibull Model
Parameters:
P(d) is the probability of the response occurring at dose d,
γ is the background response value,
β is the slope, and α is the power term (restricted to > = 1).
Appendix B: Model Validation and Parameterization of the Rat Gestation and Lactational Model
The final DEHP model contains four inter-connected sub-models, each with the necessary amount of detail to adequately describe the chemical species: diester, monoester, monoester-glucuronide, and the combined oxidative metabolites. The individual sub-models interact at sites of metabolism (hydrolysis of the diester, glucuronidation, hydrolysis of the glucuronide, and oxidation). The models for each chemical species in the adult rat are described below, followed by the modifications made to describe gestation and lactation.
Intact diester. Enzymes responsible for the hydrolysis of the diesters are present in the intestinal mucosa, blood and liver (Rowland et al., Citation1977; Tanaka et al., Citation1978). Hydrolysis in the blood and liver are described as a first order rates as none of the tested doses were sufficient to overwhelm hydrolysis. Hydrolysis in the upper GI (stomach + small intestine; GC1), on the other hand, is described as a saturable process based on the in vitro data of Rowland et al. (Citation1977) and the apparent saturation of oral uptake at the highest doses (>500 mg/kg) (Kessler et al., Citation2004). Some diester may also enter circulation intact via oral absorption or be passed into the lower intestine (GC2), where it is excreted in the feces. Oral absorption is described as a first order process. Movement through the intestine and fecal excretion are described as clearance rates (L/hr). Diester that is taken up into the gut wall is passed to the liver via the portal blood where it is hydrolyzed, released into systemic circulation or excreted into the bile. Biliary diester is excreted into the upper intestine.
Free monoester. Oral absorption of the monoesters is described as a first order process. Movement through the GI and fecal excretion are clearance rates. Unlike the diesters, their monoester metabolites MEHP and MBP are readily absorbed in the gut. Glucuronidation and oxidation of the monoester in the liver are described using saturable kinetics. The free monoesters may be excreted into the bile (recirculated to small intestine) or released into systemic circulation. Transport of monoester into the tissues is modeled using diffusion-limitation. Secretion into the urine is a saturable process, based on non-linear behavior of MBP excretion data at low doses (Payan et al., Citation2001).
Monoester-glucuronide conjugates. Because MEHP is not glucuronidated in vivo, this section of the model only applies to DBP.
Oxidative Metabolites. Metabolites of MEHP and MBP formed by P450 metabolism in the liver are released into the body via the venous blood. In the original DBP model, a one-compartment volume of distribution model was used to describe the combined oxidative metabolites. This description was sufficient for DBP, due the fact the majority of the dose exists as free MBP or MBP-G in the rat. However, in the case of DEHP, the metabolite profile is quite different. In fact, the majority of the dose (>90%) undergoes oxidation, free MEHP is only a minor metabolite, and the glucuronide conjugate does not exist at detectable levels. Thus the description of the oxidative metabolites was expanded to better describe DEHP kinetics.
Oxidative metabolism is described in the liver using a saturable Michealis-Menten description. The oxidized monoesters are then excreted into the bile or released into systemic circulation. Biliary metabolites are released into the upper intestine (GC1), where they may be reabsorbed (described as a first order rate) or passed in the feces (described as a first order clearance rate). A three compartment model is used to describe the oxidative metabolites in the blood, liver and other tissues. Distribution of into the tissues is modeled using flow-limitation, assuming distribution with body water. Urinary excretion is modeled using a first order clearance rate from the plasma compartment.
Gestation Kinetics
During gestation, all of the chemical species are allowed to move freely between the arterial and placental blood. However, only the free monoester was allowed to cross the placenta based on the previous DBP model and metabolite data in the fetal rat. While MBP-G was found in the fetal blood, the kinetic behavior suggests that it is formed in the fetus rather than maternally. Transfer of MEHP and MBP between the placental blood and the fetal blood are described as diffusion-limited processes (Gentry et al., Citation2003). Based on fetal MBP kinetic data, as well as published data on UDPGT and β-glucuronidase activities in fetal tissues (Lucier and McDaniel, Citation1977; Lucier et al., Citation1975; Wishart, Citation1978), glucuronidation and hydrolysis of the glucuronide conjugates were included in both dam and fetus. P450 activity, however, is negligible in the fetal liver (Neale and Parke, Citation1973) and was therefore excluded from the fetal model. Metabolite transfer between the fetus and amniotic fluid were described as first-order processes. Transfer between the fetal plasma and testes tissue is described using flow-limited transport. Postnatal Kinetics
The structure of neonatal model is identical to that of the adult non-pregnant rat. In vitro data suggests that the enzymes responsible for both glucuronidation and oxidative metabolism are present and increasing in concentration during the postnatal period. Thus, both metabolic processes were included in the neonatal model. Uptake of the diesters and monoesters are described as flow-limited. Transfer in the milk is described using first order clearance rates based on the milk concentrations and published suckling rates (Stolc et al., Citation1966).
Model Parameterization:
Physiological Parameters:
Physiological parameters were obtained from measured values in the literature as described in Clewell et al. (Citation2008). Adult male rat, body weight, cardiac output, and fractional tissue volumes and blood flows were available from Brown et al. (Citation1997). Fractional tissue volumes were scaled by BW and blood flows were scaled by BW0.75.
Gestation. During gestation, mammary gland (VM) and fat (VF) tissue growth were described as a linear processes based on the data of Hanwell and Linzell, (1973) and Andrade et al. (Citation2006b), Knight and Peaker (Citation1982), and Naismith et al., (Citation1982), as described in Clewell et al. (Citation2003). Placental volume (VPl) was described as the sum of the yolk sac and chorioallantoic placenta based on the model of O’Flaherty et al. (Citation1992). Growth equations were available in the cited papers. The total body weight of the dam was made equal to the initial body weight plus the change in volume of the uterus, fat, mammary gland, placenta, and fetus. Fetal volume (Vfet) was described using the equations of O’Flaherty et al. (Citation1992). Growth of fetal testes is proportional to the total body weight, accounting for approximately 0.1% of the total fetal volume from GD16 through the end of gestation (LaBorde et al., Citation1992; Naessany and Picon, Citation1982; Parks et al., Citation2000). Changes in amniotic fluid volume were described using a Table function in the simulation software, by linear interpolation between data points (Park and Shepard, Citation1994; Wykoff, Citation1971).
Maternal cardiac output was described as the sum of initial cardiac output (Brown et al., Citation1997) and the change in blood flow to the placenta, mammary and fat tissues, per the approach of O’Flaherty et al. (Citation1992). Changes in the fractional cardiac output to the mammary gland, fat and yolk sac were assumed to be proportional to changes in tissue volumes, with the exception of the chorioallantoic placenta which increased more rapidly than the tissue volume. Chemical transport within the fetus was modeled using diffusion, rather than blood-flow limitation. Thus, no assumptions were made as to proportional blood flows to fetal tissues.
Lactation. The physiological description of maternal and neonatal rats during lactation is based on the work of Clewell et al. (Citation2003). Maternal body weight increases by 12% between PND 1 and 10 (Clewell et al., Citation2003). The relative volume of the mammary tissue increased from 4.4% on PND 2 to 5.6, 6.3 and 6.6% of the maternal body weight on PND 7, 14 and 21, respectively (Knight et al., Citation1984). Maternal body fat increased from 12.4 to 15.2% of the body weight between parturition and PND 2, with a subsequent decrease to 6.9% of the body weight from PND 2 to 16 (Naismith et al., Citation1982). The rate of milk production was assumed to be equal to the suckling rate in Stolc et al. (Citation1966). Values for neonatal body fat increase from 2.7 to 11% BW between PND 2 and 16 and a subsequent decrease to the adult value of 4.61(Brown et al., Citation1997; Naismith et al., Citation1982). Changes in neonatal body weight and relative tissue volumes have been described previously (Clewell et al., Citation2003).
Changing maternal cardiac output and fractional blood flow to the mammary tissue throughout lactation are described according to the data of Hanwell and Linzell (Citation1973). Neonatal cardiac output, hematocrit and regional blood flows are based on the data of Rakusan and Marcinek (Citation1973).
Kinetic Parameters:
Kinetic parameters were scaled allometrically as is typical for intra- and inter-species extrapolation (Dedrick, Citation1973). PA, Vmax, and clearance constants were scaled by BW0.75. Whenever possible parameters were taken from published values or calculated from in vitro studies. However, the lack of specific tissue and metabolism data required that some model parameters be fitted to in vivo kinetic data. A detailed description of the process used to determine the original DBP model parameters is available elsewhere (Clewell et al., Citation2008).
While an attempt was made to keep the model parameters as similar as possible between the DEHP and DBP models, it was necessary to refine a number of the kinetic parameters in order to recapitulate the DEHP kinetic data. In fact, when the DBP model was run “as is” against the MEHP iv and DEHP po data, the model over-predicted serum MEHP levels by an order of magnitude (not shown). Several observations can be made about the kinetic differences in the two phthalates based on available in vitro and in vivo data. 1) DEHP is not hydrolyzed as efficiently as DBP in the gut (Rowland et al., Citation1977), which leads to a greater loss of unmetabolized diester in the feces as well as some circulation of DEHP in the blood. 2) Both DEHP and MEHP also are more poorly absorbed in the gut than DBP and MBP. 3) The high lipophilicity of DEHP and the reduced hydrolysis in the blood (when compared to DBP) also results in reduced clearance of the diester from the blood after iv dosing. Iv doses of DBP, on the other hand are metabolized within minutes of dosing. 4) Metabolism of the monoester is also quite different for the two phthalates. A large portion of MBP is removed via glucuronidation and oxidation is only important at low doses (Payan et al., Citation2001). In contrast, oxidation, not glucuronidation, is the dominant metabolic pathway for MEHP. Oxidative metabolites make up >90% of the total urinary metabolites, while glucuronide conjugates of MEHP have not been detected in the urine or plasma of rats after DEHP dosing (Albro and Moore, Citation1974).
Based on these observations, UGT activity (VmaxLc) was turned off in the DEHP model. The Vmax for oxidative metabolism (VmaxOc = 28 mg/hr-kg BW) was adjusted by visually fitting the model simulations to MEHP iv data in the adult male rat (Pollack et al., Citation1985). The rate of oral absorption of MEHP (kam = 0.4 hr−1) was adjusted based on the fit of the model to MEHP oral gavage studies (Teirlynck and Belpaire, Citation1985). The maximum capacity for hydrolysis in the gut (VmaxGc = 80 mg/hr-kg BW), oral absorption of DEHP (kad = 0.015 hr−1), and intestinal clearance of DEHP (kgic1 = 0.1L /hr) and the other metabolites (kgic2 = 0.05 L/hr), and the urinary excretion rate of MEHP (VmxUc = 1 mg/hr-kg BW, KmU = 450 mg/L) were fit to DEHP oral gavage data (blood, feces, urine) in the non-pregnant female rat (Kessler et al., Citation2004). With the exception of those parameters listed above, the kinetic parameters were identical to those used in the DBP model and are available elsewhere (Clewell et al., Citation2008). Final model simulations are shown versus various data sets after iv and po doses of MEHP or DEHP in the non-pregnant rat in Figures B1− B3.
Figure B1. Free MEHP in the blood of the adult male rat after an iv dose of 20 or 50 mg/kg MEHP. Lines indicate model simulations. Points represent measurements from individual animals administered (•) 20 mg/kg MEHP (Sjoberg et al., Citation1985), (▾) 50 mg/kg MEHP (Pollack et al., Citation1985), or (Δ) 50 mg/kg MEHP (Kessler et al., Citation2004).
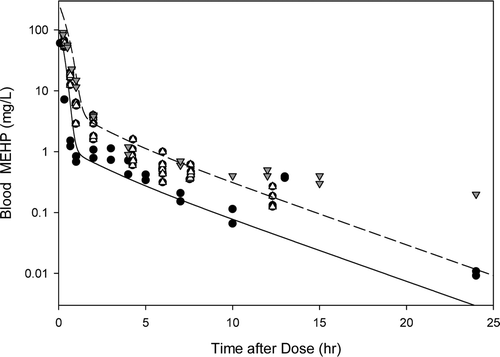
Figure B2. Free MEHP in the (A) blood of adult male rat after an oral dose of 70, 100, or 400 mg/kg MEHP or (B) excreta of adult male rat after an oral dose of 70 mg/kg MEHP. (A) Lines indicate model simulations. Points represent measurements from individual animals administered (o) 70 mg/kg MEHP (Chu et al., Citation1978), (▪) 100 mg/kg MEHP (Pollack et al., Citation1985), or (•) 400 mg/kg MEHP (Teirlynck and Belpaire, Citation1985). (B) Bars represent model simulations (gray) or mean + SD of measured (black) MEHP in the urine and feces rats administered 70 mg/kg MEHP (Chu et al., Citation1978).
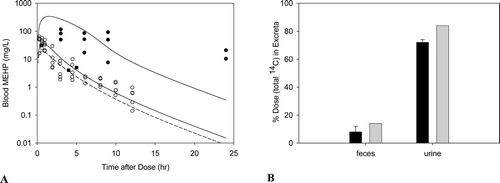
Figure B3. Free MEHP in the blood of adult rats after an (A)iv dose of 100 mg/kg DEHP, or (B) oral dose of 30, 300, or 500 mg/kg DEHP. Line indicates model simulation. Points represent measurements from individual animals administered (A) 100 mg/kg DEHP iv (Kessler et al., Citation2004) or (B) (o) 30 mg/kg, (Δ) 300 mg/kg, or (•) 500 mg/kg DEHP po (Kessler et al., Citation2004).
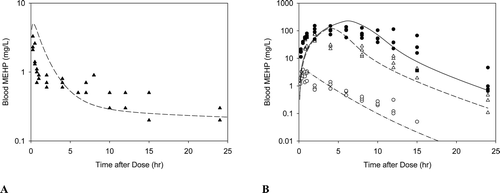
Gestation. Similar to DBP (Clewell et al., Citation2008), only one kinetic parameter was adjusted before using the model in the pregnant dam based on published in vitro studies: VmaxOc. Because glucuronidation is set to zero in the DEHP model, it was not necessary to adjust that parameter for the reduced capacity for glucuronide conjugation during gestation (Lucier et al., Citation1975; Luquita et al., Citation2001). However, similar studies with microsomes obtained from the liver of pregnant and non-pregnant rats showed approximately 40% reduction in the capacity of oxidative metabolism in the pregnant rat liver regardless of the substrate used (Neale and Parke, Citation1973). Thus, the maximum capacity for oxidative metabolism of MEHP (VmaxOc) was reduced by 40% when applied to the pregnant rat. All other maternal parameters were scaled allometrically from the adult male rat. Since MEHP is not significantly glucuronidated and oxidative metabolism is negligible in the rat fetus, both VmaxGcf and VmaxOcf were set to zero in the fetus. Transfer of MEHP between the maternal and fetal blood, and between the fetal blood and amniotic fluid were assumed to be the same as MBP. Model simulations are shown with data collected in the pregnant and fetal rat after oral administration of DEHP (Figure B4).
Figure B4. Free MEHP in the (A) maternal blood or (B) placenta and fetal tissues after the last oral dose of 30 or 500 mg/kg/day administered from GD 14 - 19. (A) Lines indicate model simulations. Points represent measurements from individual animals administered (•) 30 mg/kg/day or (o) 500 mg/kg/day DEHP po (Kessler et al., Citation2004). (B) Bars represent model simulations (black) or mean + SD of measured concentrations (gray) in the placenta and fetal tissues 2 hrs after the last daily dose of 500 mg/kg/day (Kessler et al., Citation2004).
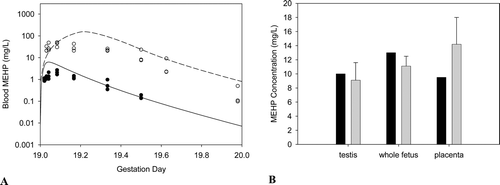
Figure B5. (A) DEHP and MEHP in the milk and MEHP in the blood of the lactating dam, and (B) MEHP in the suckling rat after the last oral dose of 2000 mg/kg/day administered on days 15− 17 of lactation. Bars represent model simulations (black) or mean + SD of measured concentrations (gray) in the milk, maternal blood and pup blood 3-6 hrs after the last dose of 2000 mg/kg/day DEHP (Dostal et al., Citation1987).
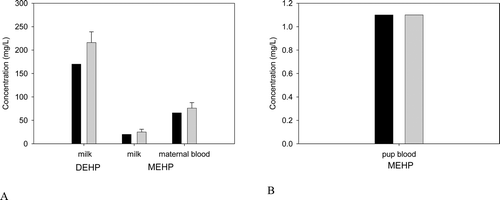
Lactation. During lactation, both DEHP and MEHP were assumed to move freely between the blood and the mammary gland. Transfer between the mammary gland and milk was described using diffusion-limited uptake. In the absence of rat data, partition coefficients for DEHP (pmk = 200) and MEHP (pmmk: 0.3) were obtained from human blood:milk measurements (Hogberg et al., Citation2008). Development of oxidative metabolism (VmaxOn) in the neonate was estimated using in vitro data for total P450 activity using the same method described for fetal UGT development in the DBP model (Equation B1; (Clewell et al., Citation2008). All other neonatal parameters were scaled allometrically from the adult male rat values.
VmaxOn = VmaxO × RAnL × MPC × BWn × numpups (Equation B1)
where VmaxO is the maximum capacity for oxidative metabolism in the adult rat (after scaling for BW), RAnL is the in vitro relative activity expressed as the ratio of neonatal to maternal activity per mg microsomal protein, MPC is the ratio of the microsomal protein content of the fetal liver to maternal liver, LW is the ratio of the fetal: maternal liver weight, and numpups is the number of pups per litter. Model simulations are shown with data collected in the lactating and suckling rat after oral administration of DEHP to the dam are shown in Figure B5.
Notes
1When considering cancer endpoints, the use of a species scaling factor based on comparative surface area still forms the basis for the extrapolation across species for the vast majority of chemicals listed in IRIS. This is the case because the original assessment of the carcinogenic potency of these chemicals was derived prior to the recommended and subsequently implemented change to a comparative body weight scaling USEPA. (1996). Proposed Guidelines for Carcinogen Risk Assessment. EPA/600/P-92/003C. Office of Research and Development, Washington, DC. April, 1996.. Further, not all regulatory bodies, such as the State of California’s Prop 65 program, have adopted the newest species scaling approach of body weight3/4.
2A “point of departure” (POD) marks the beginning of extrapolation to lower doses. The POD is an estimated dose (usually expressed in human-equivalent terms) near the lower end of the observed range, without significant extrapolation to lower doses.
3This classification scheme is still in use and reported in the USEPA’s IRIS for the vast majority of chemicals that have not been re-evaluated using the newest guidelines.
References
- Akaike H. 1974. A new look at the statistical model identification. IEEE Transactions on Automatic Control 19(6):716–723.
- Akingbemi BT, Ge R, Klinefelter GR, Zirkin BR, Hardy MP. 2004. Phthalate-induced Leydig cell hyperplasia is associated with multiple endocrine disturbances. Proc Natl Acad Sci U S A 101:775–780.
- Akingbemi BT, Youker RT, Sottas CM, Ge R, Katz E, Klinefelter GR, Zirkin BR, Hardy MP. 2001. Modulation of rat Leydig cell steroidogenic function by di(2-ethylhexyl)phthalate. Biol Reprod 65:1252–1259.
- Albro PW. 1986. Absorption, metabolism, and excretion of di(2-ethylhexyl) phthalate by rats and mice. Environ Health Perspect 65:293–298.
- Albro PW, Hass JR, Peck CC, Jordan ST, Corbett JT, Schroeder J. 1982. Applications of isotope differentiation for metabolic studies with di-(2-ethylhexyl) phthalate. J Environ Sci Health B 17:701–714.
- Albro PW, Moore B. 1974. Identification of the metabolites of simple phthalate diesters in rat urine. J Chromatogr 94:209–218.
- Alexander B. 2001. Mortality study of workers employed at the 3M Cottage Grove facility. Final Report. Division of Environmental and Occupational Health, School of Public Health, University of Minnesota.
- Alexander BH, Olsen GW, Burris JM, Mandel JH, Mandel JS. 2003. Mortality of employees of a perfluorooctanesulphonyl fluoride manufacturing facility. Occup Environ Med 60:722–729.
- Andersen ME, Clewell HJ, 3rd Gargas, ML, MacNaughton MG, Reitz RH, Nolan RJ, McKenna MJ. 1991. Physiologically based pharmacokinetic modeling with dichloromethane, its metabolite, carbon monoxide, and blood carboxyhemoglobin in rats and humans. Toxicol Appl Pharmacol 108:14–27.
- Andersen ME, MacNaughton MG, Clewell HJ, 3rd Paustenbach, DJ. 1987. Adjusting exposure limits for long and short exposure periods using a physiological pharmacokinetic model. Am Ind Hyg Assoc J 48:335–343.
- Andrade AJ, Grande SW, Talsness CE, Gericke C, Grote K, Golombiewski A, Sterner-Kock A, Chahoud I. 2006c. A dose response study following in utero and lactational exposure to di-(2-ethylhexyl) phthalate (DEHP): reproductive effects on adult male offspring rats. Toxicology 228:85–97.
- Andrade AJ, Grande SW, Talsness CE, Grote K, Chahoud I. 2006b. A dose–response study following in utero and lactational exposure to di-(2-ethylhexyl)-phthalate (DEHP): non-monotonic dose–response and low dose effects on rat brain aromatase activity. Toxicology 227:185–192.
- Andrade AJ, Grande SW, Talsness CE, Grote K, Golombiewski A, Sterner-Kock A, Chahoud I. 2006a. A dose–response study following in utero and lactational exposure to di-(2-ethylhexyl) phthalate (DEHP): effects on androgenic status, developmental landmarks and testicular histology in male offspring rats. Toxicology 225:64–74.
- Ashby J, Brady A, Elcombe CR, Elliott BM, Ishmael J, Odum J, Tugwood JD, Kettle S, Purchase IF. 1994. Mechanistically-based human hazard assessment of peroxisome proliferator-induced hepatocarcinogenesis. Hum Exp Toxicol 13 Suppl 2:S1–117
- Atanassova NN, Walker M, McKinnell C, Fisher JS, Sharpe RM. 2005. Evidence that androgens and oestrogens, as well as follicle-stimulating hormone, can alter Sertoli cell number in the neonatal rat. J Endocrinol 184:107–117.
- ATSDR (Agency for Toxic Substances and Disease Registry). 2002. Toxicological profile for Di(2-ethylhexyl)phthalate. U.S. Department of Health and Human Services, Public Health Service, Agency for Toxic Substances and Disease Registry.
- Barnes DG, Daston GP, Evans JS, Jarabek AM, Kavlock RJ, Kimmel CA, Park C, Spitzer HL. 1995. Benchmark Dose Workshop: criteria for use of a benchmark dose to estimate a reference dose. Regul Toxicol Pharmacol 21:296–306.
- Barton HA. 2005. Computational pharmacokinetics during developmental windows of susceptibility. J Toxicol Environ Health A 68:889–900.
- Barton HA, Clewell HJ 3rd. 2000. Evaluating noncancer effects of trichloroethylene: dosimetry, mode of action, and risk assessment. Environ Health Perspect 108 Suppl 2:323–334.
- Beall MH, van den Wijngaard JP, van Gemert MJ, Ross MG. 2007. Amniotic fluid water dynamics. Placenta 28:816–823.
- Bentley P, Calder I, Elcombe C, Grasso P, Stringer D, Wiegand HJ. 1993. Hepatic peroxisome proliferation in rodents and its significance for humans. Food Chem Toxicol 31:857–907.
- Biegel LB, Hurtt ME, Frame SR, O’Connor JC, Cook JC. 2001. Mechanisms of extrahepatic tumor induction by peroxisome proliferators in male CD rats. Toxicol Sci 60:44–55.
- Biegel LB, Liu RC, Hurtt ME, Cook JC. 1995. Effects of ammonium perfluorooctanoate on Leydig cell function: in vitro, in vivo, and ex vivo studies. Toxicol Appl Pharmacol 134:18–25.
- Boobis AR, Cohen SM, Dellarco V, McGregor D, Meek ME, Vickers C, Willcocks D, Farland W. 2006. IPCS framework for analyzing the relevance of a cancer mode of action for humans. Crit Rev Toxicol 36:781–792.
- Boobis AR, Doe JE, Heinrich-Hirsch B, Meek ME, Munn S, Ruchirawat M, Schlatter J, Seed J, Vickers C. 2008. IPCS framework for analyzing the relevance of a noncancer mode of action for humans. Crit Rev Toxicol 38:87–96.
- Borch J, Metzdorff SB, Vinggaard AM, Brokken L, Dalgaard M. 2006. Mechanisms underlying the anti-androgenic effects of diethylhexyl phthalate in fetal rat testis. Toxicology 223:144–155.
- Bosland MC. 1996. Hormonal factors in carcinogenesis of the prostate and testis in humans and in animal models. Prog Clin Biol Res 394:309–352.
- Brown RP, Delp MD, Lindstedt SL, Rhomberg LR, Beliles RP. 1997. Physiological parameter values for physiologically based pharmacokinetic models. Toxicol Ind Health 13:407–484.
- Butenhoff J, Costa G, Elcombe C, Farrar D, Hansen K, Iwai H, Jung R, Kennedy G, Jr., Lieder P, Olsen G, and others. 2002. Toxicity of ammonium perfluorooctanoate in male cynomolgus monkeys after oral dosing for 6 months. Toxicol Sci 69:244–257.
- Cahill TM, Cousins I, Mackay D. 2003. Development and application of a generalized physiologically based pharmacokinetic model for multiple environmental contaminants. Environ Toxicol Chem 22:26–34.
- Calafat AM, Brock JW, Silva MJ, Gray LE, Jr., Reidy JA, Barr DB, Needham LL. 2006. Urinary and amniotic fluid levels of phthalate monoesters in rats after the oral administration of di(2-ethylhexyl) phthalate and di-n-butyl phthalate. Toxicology 217:22–30.
- CALEPA (California Environmental Protection Agency). 1997. Public Health Goal for Di(2-Ethylhexyl)phthalate (DEHP) in drinking water. Pesticide and Environmental Toxicology Section, Office of Environmental Health Hazard Assessment, California Environmental Proection Agency.
- Cattley RC. 2004. Peroxisome proliferators and receptor-mediated hepatic carcinogenesis. Toxicol Pathol 32 Suppl 2:6–11.
- Cattley RC, DeLuca J, Elcombe C, Fenner-Crisp P, Lake BG, Marsman DS, Pastoor TA, Popp JA, Robinson DE, Schwetz B, and others. 1998. Do peroxisome proliferating compounds pose a hepatocarcinogenic hazard to humans? Regul Toxicol Pharmacol 27:47–60.
- CDC (Centers for Disease Control and Prevention). 2005. D.O.H.H.S (ed.) Third National Report on Human Exposure to Environmental Chemicals, National Center for Environmental Health, Centers for Disease Control and Prevention, Atlanta, 2005.
- Chevalier S, Roberts RA. 1998. Perturbation of rodent hepatocyte growth control by nongenotoxic hepatocarcinogens: mechanisms and lack of relevance for human health (review). Oncol Rep 5:1319–1327.
- Chu I, Villeneuve DC, Secours V, Franklin C, Rock G, Viau A. 1978. Metabolism and tissue distribution of mono-2-ethylhexyl phthalate in the rat. Drug Metab Dispos 6:146–149.
- Clegg ED, Cook JC, Chapin RE, Foster PM, Daston GP. 1997. Leydig cell hyperplasia and adenoma formation: mechanisms and relevance to humans. Reprod Toxicol 11:107–121.
- Clewell HJ, 3rd Andersen, ME. 1985. Risk assessment extrapolations and physiological modeling. Toxicol Ind Health 1:111–131.
- Clewell HJ, 3rd Andersen, ME. 1989. Biologically motivated models for chemical risk assessment. Health Phys 57 Suppl 1:129–137.
- Clewell HJ, 3rd Gentry, PR, Covington TR, Gearhart JM. 2000. Development of a physiologically based pharmacokinetic model of trichloroethylene and its metabolites for use in risk assessment. Environ Health Perspect 108 Suppl 2:283–305.
- Clewell HJ, Gearhart JM, Gentry PR, Covington TR, VanLandingham CB, Crump KS, Shipp AM. 1999. Evaluation of the uncertainty in an oral reference dose for methylmercury due to interindividual variability in pharmacokinetics. Risk Anal 19:547–558.
- Clewell HJ, Gentry PR, Covington TR, Sarangapani R, Teeguarden JG. 2004. Evaluation of the potential impact of age- and gender-specific pharmacokinetic differences on tissue dosimetry. Toxicol Sci 79:381–393.
- Clewell HJ, Gentry PR, Gearhart JM, Allen BC, Andersen ME. 1995. Considering pharmacokinetic and mechanistic information in cancer risk assessments for environmental contaminants: examples with vinyl chloride and trichloroethylene. Chemosphere 31:2561–2578.
- Clewell HJ, Gentry PR, Gearhart JM, Allen BC, Andersen ME. 2001a. Comparison of cancer risk estimates for vinyl chloride using animal and human data with a PBPK model. Sci Total Environ 274:37–66.
- Clewell RA, Clewell HJ 3rd. 2008. Development and specification of physiologically based pharmacokinetic models for use in risk assessment. Regul Toxicol Pharmacol 50:129–143.
- Clewell RA, Gearhart JM. 2002. Pharmacokinetics of toxic chemicals in breast milk: use of PBPK models to predict infant exposure. Environ Health Perspect 110:A333–A337
- Clewell RA, Kremer JJ, Williams CC, Campbell JL, Jr., Andersen ME, Borghoff SJ. 2008. Tissue exposures to free and glucuronidated monobutylyphthalate in the pregnant and fetal rat following exposure to di-n-butylphthalate: evaluation with a PBPK model. Toxicol Sci 103:241–259.
- Clewell RA, Merrill EA, Gearhart JM, Robinson PJ, Sterner TR, Mattie DR, Clewell HJ 3rd. 2007. Perchlorate and radioiodide kinetics across life stages in the human: using PBPK models to predict dosimetry and thyroid inhibition and sensitive subpopulations based on developmental stage. J Toxicol Environ Health A 70:408–428.
- Clewell RA, Merrill EA, Robinson PJ. 2001b. The use of physiologically based models to integrate diverse data sets and reduce uncertainty in the prediction of perchlorate and iodide kinetics across life stages and species. Toxicol Ind Health 17:210–222.
- Clewell RA, Merrill EA, Yu KO, Mahle DA, Sterner TR, Mattie DR, Robinson PJ, Fisher JW, Gearhart JM. 2003. Predicting fetal perchlorate dose and inhibition of iodide kinetics during gestation: a physiologically-based pharmacokinetic analysis of perchlorate and iodide kinetics in the rat. Toxicol Sci 73:235–255.
- Cohen SM. 2004. Human carcinogenic risk evaluation: an alternative approach to the two-year rodent bioassay. Toxicol Sci 80:225–229.
- Cohen SM, Meek ME, Klaunig JE, Patton DE, Fenner-Crisp PA. 2003. The human relevance of information on carcinogenic modes of action: overview. Crit Rev Toxicol 33:581–589.
- Cook JC, Klinefelter GR, Hardisty JF, Sharpe RM, Foster PM. 1999. Rodent Leydig cell tumorigenesis: a review of the physiology, pathology, mechanisms, and relevance to humans. Crit Rev Toxicol 29:169–261.
- Cook JC, Murray SM, Frame SR, Hurtt ME. 1992. Induction of Leydig cell adenomas by ammonium perfluorooctanoate: a possible endocrine-related mechanism. Toxicol Appl Pharmacol 113:209–217.
- Corley R. 1996. Assessing the risk of hemolysis in humans exposed to 2-butoxyethanol using a physiologically-based pharmacokinetic model. Occupational Hygiene 2:45–55.
- Corley RA, Bormett GA, Ghanayem BI. 1994. Physiologically based pharmacokinetics of 2-butoxyethanol and its major metabolite, 2-butoxyacetic acid, in rats and humans. Toxicol Appl Pharmacol 129:61–79.
- Corley RA, Mast TJ, Carney EW, Rogers JM, Daston GP. 2003. Evaluation of physiologically based models of pregnancy and lactation for their application in children’s health risk assessments. Crit Rev Toxicol 33:137–211.
- Corley RA, Mendrala AL, Smith FA, Staats DA, Gargas ML, Conolly RB, Andersen ME, Reitz RH. 1990. Development of a physiologically based pharmacokinetic model for chloroform. Toxicol Appl Pharmacol 103:512–527.
- Corton JC, Lapinskas PJ. 2005. Peroxisome proliferator-activated receptors: mediators of phthalate ester-induced effects in the male reproductive tract? Toxicol Sci 83:4–17.
- David RM. 2006. Proposed mode of action for in utero effects of some phthalate esters on the developing male reproductive tract. Toxicol Pathol 34:209–219.
- David RM, Moore MR, Cifone MA, Finney DC, Guest D. 1999. Chronic peroxisome proliferation and hepatomegaly associated with the hepatocellular tumorigenesis of di(2-ethylhexyl)phthalate and the effects of recovery. Toxicol Sci 50:195–205.
- David RM, Moore MR, Finney DC, Guest D. 2000a. Chronic toxicity of di(2-ethylhexyl)phthalate in rats. Toxicol Sci 55:433–443.
- David RM, Moore MR, Finney DC, Guest D. 2000b. Chronic toxicity of di(2-ethylhexyl)phthalate in mice. Toxicol Sci 58:377–385.
- Dedrick RL. 1973. Animal scale-up. J Pharmacokinet Biopharm 1:435–461.
- DeLuca JG, Doebber TW, Kelly LJ, Kemp RK, Molon-Noblot S, Sahoo SP, Ventre J, Wu MS, Peters JM, Gonzalez FJ, and others. 2000. Evidence for peroxisome proliferator-activated receptor (PPAR)alpha-independent peroxisome proliferation: effects of PPARgamma/delta-specific agonists in PPARalpha-null mice. Mol Pharmacol 58:470–476.
- Dostal LA, Weaver RP, Schwetz BA. 1987. Transfer of di(2-ethylhexyl) phthalate through rat milk and effects on milk composition and the mammary gland. Toxicol Appl Pharmacol 91:315–325.
- Doull J, Cattley R, Elcombe C, Lake BG, Swenberg J, Wilkinson C, Williams G, van Gemert M. 1999. A cancer risk assessment of di(2-ethylhexyl)phthalate: application of the new U.S. EPA Risk Assessment Guidelines. Regul Toxicol Pharmacol 29:327–357.
- el-Masri HA, Thomas RS, Benjamin SA, Yang RS. 1995. Physiologically based pharmacokinetic/pharmacodynamic modeling of chemical mixtures and possible applications in risk assessment. Toxicology 105:275–282.
- Fennell TR, Krol WL, Sumner SC, Snyder RW. 2004. Pharmacokinetics of dibutylphthalate in pregnant rats. Toxicol Sci 82:407–418.
- Fisher JS, Macpherson S, Marchetti N, Sharpe RM. 2003. Human ‘testicular dysgenesis syndrome’: a possible model using in-utero exposure of the rat to dibutyl phthalate. Hum Reprod 18:1383–1394.
- Fisher JW. 2000. Physiologically based pharmacokinetic models for trichloroethylene and its oxidative metabolites. Environ Health Perspect 108 Suppl 2:265–273.
- Fisher JW, Gargas ML, Allen BC, Andersen ME. 1991. Physiologically based pharmacokinetic modeling with trichloroethylene and its metabolite, trichloroacetic acid, in the rat and mouse. Toxicol Appl Pharmacol 109:183–195.
- Fisher JW, Whittaker TA, Taylor DH, Clewell HJ, 3rd Andersen, ME. 1989. Physiologically based pharmacokinetic modeling of the pregnant rat: a multiroute exposure model for trichloroethylene and its metabolite, trichloroacetic acid. Toxicol Appl Pharmacol 99:395–414.
- Foster PM. 2006. Disruption of reproductive development in male rat offspring following in utero exposure to phthalate esters. Int J Androl 29:140–147; discussion 181–185.
- Foster PM, Mylchreest E, Gaido KW, Sar M. 2001. Effects of phthalate esters on the developing reproductive tract of male rats. Hum Reprod Update 7:231–235.
- Fromme H, Bolte G, Koch HM, Angerer J, Boehmer S, Drexler H, Mayer R, Liebl B. 2007. Occurrence and daily variation of phthalate metabolites in the urine of an adult population. Int J Hyg Environ Health 210:21–33.
- Gazouli M, Yao ZX, Boujrad N, Corton JC, Culty M, Papadopoulos V. 2002. Effect of peroxisome proliferators on Leydig cell peripheral-type benzodiazepine receptor gene expression, hormone-stimulated cholesterol transport, and steroidogenesis: role of the peroxisome proliferator-activator receptor alpha. Endocrinology 143:2571–2583.
- Ge RS, Chen GR, Dong Q, Akingbemi B, Sottas CM, Santos M, Sealfon SC, Bernard DJ, Hardy MP. 2007a. Biphasic effects of postnatal exposure to diethylhexylphthalate on the timing of puberty in male rats. J Androl 28:513–520.
- Ge RS, Chen GR, Tanrikut C, Hardy MP. 2007b. Phthalate ester toxicity in Leydig cells: developmental timing and dosage considerations. Reprod Toxicol 23:366–373.
- Gentry P, Haber L, McDonald T, Zhao Q, Covington T, Nance P, Clewell III H, Lipscomb J, Barton H. 2004. Data for physiologically based pharmacokinetic modeling in neonatal animals: physiological parameters in mice and Sprague–Dawley rats. J Children Health 2:363–411.
- Gentry PR, Covington TR, Clewell HJ 3rd. 2003. Evaluation of the potential impact of pharmacokinetic differences on tissue dosimetry in offspring during pregnancy and lactation. Regul Toxicol Pharmacol 38:1–16.
- Gerrity T, Henry C. 1990. Principles of route-to-route extrapolation for risk assessment. New York, NY: Elsevier. p. 1–12.
- Gilliland FD, Mandel JS. 1993. Mortality among employees of a perfluorooctanoic acid production plant. J Occup Med 35:950–954.
- Goldenthal E, Jessup D, Geil R, Mehring J. 1978. Fluorad® Fluorochemical FC-143 Ninety Day Subacute Rhesus Monkey Toxicity Study. 137-090. International Research and Development Corporation.
- Grande SW, Andrade AJ, Talsness CE, Grote K, Chahoud I. 2006. A dose–response study following in utero and lactational exposure to di(2-ethylhexyl)phthalate: effects on female rat reproductive development. Toxicol Sci 91:247–254.
- Grande SW, Andrade AJ, Talsness CE, Grote K, Golombiewski A, Sterner-Kock A, Chahoud I. 2007. A dose–response study following in utero and lactational exposure to di-(2-ethylhexyl) phthalate (DEHP): reproductive effects on adult female offspring rats. Toxicology 229:114–122.
- Guyton KZ, Chiu WA, Bateson TF, Jinot J, Scott CS, Brown RC, Caldwell JC. 2009. A reexamination of the PPAR-alpha activation mode of action as a basis for assessing human cancer risks of environmental contaminants. Environ Health Perspect 117:1664–1672.
- Haber F. 1924. Zur Geschichte des Gaskrieges (1924). On the history of gas warfare. In: Funf Vortrage aus den Jahren 1920–1923 (Five Lectures from the years 1920–1923). Berlin: Springer. p 76–92.
- Hanefeld M, Kemmer C, Kadner E. 1983. Relationship between morphological changes and lipid-lowering action of p-chlorphenoxyisobutyric acid (CPIB) on hepatic mitochondria and peroxisomes in man. Atherosclerosis 46:239–246.
- Hanwell A, Linzell JL. 1973. The time course of cardiovascular changes in lactation in the rat. J Physiol 233:93–109.
- Herath CB, Watanabe G, Wanzhu J, Noguchi J, Akiyama K, Kuramoto K, Groome NP, Taya K. 2001. Elevated levels of inhibin-A and immunoreactive inhibin in aged male Wistar rats with testicular Leydig cell tumor. J Androl 22:838–846.
- Hinton RH, Mitchell FE, Mann A, Chescoe D, Price SC, Nunn A, Grasso P, Bridges JW. 1986. Effects of phthalic acid esters on the liver and thyroid. Environ Health Perspect 70:195–210.
- Hogberg J, Hanberg A, Berglund M, Skerfving S, Remberger M, Calafat AM, Filipsson AF, Jansson B, Johansson N, Appelgren M, Hakansson H 2008. Phthalate Diesters and Their Metabolites in Human Breast Milk, Blood or Serum, and Urine as Biomarkers of Exposure in Vulnerable Populations. Environmental Health Perspectives 116(3)::334–339.
- Howdeshell KL, Rider CV, Wilson VS, Gray LE Jr. 2008. Mechanisms of action of phthalate esters, individually and in combination, to induce abnormal reproductive development in male laboratory rats. Environ Res 108:168–176.
- Hunt MC, Lindquist PJ, Peters JM, Gonzalez FJ, Diczfalusy U, Alexson SE. 2000. Involvement of the peroxisome proliferator-activated receptor alpha in regulating long-chain acyl-CoA thioesterases. J Lipid Res 41:814–823.
- IARC (International Agency for Research on Cancer). 1995. IARC Technical Report No. 24. Peroxisome Proliferation and Its Role in Carcinogenesis. World Health Organization: International Agency for Research on Cancer. Lyon, France: IARC Press.
- ICRP (International Commission on Radiological Protection). 2002. Basic Anatomical and Physiological Data for Use in Radiological Protection: Reference Values: Ed. J. Valentin. ICRP Publication 89, Ann. ICRP 32. p. 1–277.
- IPCS (International Programme on Chemical Safety). 2005. IPCS framework for analyzing the relevance of a cancer mode of action for humans. IPCS Workshop 1–29.
- Ito Y, Yamanoshita O, Asaeda N, Tagawa Y, Lee CH, Aoyama T, Ichihara G, Furuhashi K, Kamijima M, Gonzalez FJ, and others. 2007. Di(2-ethylhexyl)phthalate induces hepatic tumorigenesis through a peroxisome proliferator-activated receptor alpha-independent pathway. J Occup Health 49:172–182.
- Ji B, Bi Y, Simeone D, Mortensen RM, Logsdon CD. 2001. Human pancreatic acinar cells lack functional responses to cholecystokinin and gastrin. Gastroenterology 121:1380–1390.
- Ji B, Bi Y, Simeone D, Mortensen RM, Logsdon CD. 2002. Human pancreatic acinar cells do not respond to cholecystokinin. Pharmacol Toxicol 91:327–332.
- Johanson G, Filser JG. 1993. A physiologically based pharmacokinetic model for butadiene and its metabolite butadiene monoxide in rat and mouse and its significance for risk extrapolation. Arch Toxicol 67:151–163.
- Kang KS, Lee YS, Kim HS, Kim SH. 2002. DI-(2-ethylhexyl) phthalate-induced cell proliferation is involved in the inhibition of gap junctional intercellular communication and blockage of apoptosis in mouse Sertoli cells. J Toxicol Environ Health A 65:447–459.
- Kavlock R, Barr D, Boekelheide K, Breslin W, Breysse P, Chapin R, Gaido K, Hodgson E, Marcus M, Shea K, and others. 2006. NTP-CERHR Expert Panel Update on the Reproductive and Developmental Toxicity of di(2-ethylhexyl) phthalate. Reprod Toxicol 22:291–399.
- Kessler W, Numtip W, Grote K, Csanady GA, Chahoud I, Filser JG. 2004. Blood burden of di(2-ethylhexyl) phthalate and its primary metabolite mono(2-ethylhexyl) phthalate in pregnant and nonpregnant rats and marmosets. Toxicol Appl Pharmacol 195:142–53.
- Keys DA, Wallace DG, Kepler TB, Conolly RB. 1999. Quantitative evaluation of alternative mechanisms of blood and testes disposition of di(2-ethylhexyl) phthalate and mono(2-ethylhexyl) phthalate in rats. Toxicol Sci 49:172–185.
- Keys DA, Wallace DG, Kepler TB, Conolly RB. 2000. Quantitative evaluation of alternative mechanisms of blood disposition of di(n-butyl) phthalate and mono(n-butyl) phthalate in rats. Toxicol Sci 53:173–184.
- Klaunig JE, Babich MA, Baetcke KP, Cook JC, Corton JC, David RM, DeLuca JG, Lai DY, McKee RH, Peters JM, and others. 2003. PPARalpha agonist-induced rodent tumors: modes of action and human relevance. Crit Rev Toxicol 33:655–780.
- Kleymenova E, Swanson C, Boekelheide K, Gaido KW. 2005. Exposure in utero to di(n-butyl) phthalate alters the vimentin cytoskeleton of fetal rat Sertoli cells and disrupts Sertoli cell-gonocyte contact. Biol Reprod 73:482–490.
- Kluwe WM. 1982. Overview of phthalate ester pharmacokinetics in mammalian species. Environ Health Perspect 45:3–9.
- Knight CH, Docherty AH, Peaker M. 1984. Milk yield in rats in relation to activity and size of the mammary secretory cell population. J Dairy Res 51:29–35.
- Knight CH, Peaker M. 1982. Mammary cell proliferation in mice during pregnancy and lactation in relation to milk yield. Q J Exp Physiol 67:165–177.
- Koch HM, Bolt HM, Angerer J. 2004. Di(2-ethylhexyl)phthalate (DEHP) metabolites in human urine and serum after a single oral dose of deuterium-labelled DEHP. Arch Toxicol 78:123–130.
- Koch HM, Bolt HM, Preuss R, Angerer J. 2005. New metabolites of di(2-ethylhexyl)phthalate (DEHP) in human urine and serum after single oral doses of deuterium-labelled DEHP. Arch Toxicol 79:367–376.
- Koch HM, Rossbach B, Drexler H, Angerer J. 2003. Internal exposure of the general population to DEHP and other phthalates–determination of secondary and primary phthalate monoester metabolites in urine. Environ Res 93:177–185.
- Kurahashi N, Kondo T, Omura M, Umemura T, Ma M, Kishi R. 2005. The effects of subacute inhalation of di (2-ethylhexyl) phthalate (DEHP) on the testes of prepubertal Wistar rats. J Occup Health 47:437–444.
- LaBorde JB, Hansen DK, Young JF, Sheehan DM, Holson RR. 1992. Prenatal dexamethasone exposure in rats: effects of dose, age at exposure, and drug-induced hypophagia on malformations and fetal organ weights. Fundam Appl Toxicol 19:545–554.
- Lai DY. 2004. Rodent carcinogenicity of peroxisome proliferators and issues on human relevance. J Environ Sci Health C Environ Carcinog Ecotoxicol Rev 22:37–55.
- Li LH, Jester WF, Jr., Laslett AL, Orth JM. 2000. A single dose of Di-(2-ethylhexyl) phthalate in neonatal rats alters gonocytes, reduces sertoli cell proliferation, and decreases cyclin D2 expression. Toxicol Appl Pharmacol 166:222–229.
- Li Y, Hao Y, Owyang C. 1995. Evidence for autoregulation of cholecystokinin secretion during diversion of bile pancreatic juice in rats. Gastroenterology 109:231–238.
- Liu K, Lehman KP, Sar M, Young SS, Gaido K. 2004. Gene expression profiling following in utero exposure to phthalate esters reveals new gene targets in the etiology of testicular dysgenesis. Biol Reprod 73(1):180–192.
- Liu RC, Hahn C, Hurtt ME. 1996. The direct effect of hepatic peroxisome proliferators on rat Leydig cell function in vitro. Fundam Appl Toxicol 30:102–108.
- Longnecker DS. 1987. Interface between adaptive and neoplastic growth in the pancreas. Gut 28 Suppl:253–258.
- Louie DS, May D, Miller P, Owyang C. 1986. Cholecystokinin mediates feedback regulation of pancreatic enzyme secretion in rats. Am J Physiol 250:G252–259
- Lovekamp-Swan T, Davis BJ. 2003. Mechanisms of phthalate ester toxicity in the female reproductive system. Environ Health Perspect 111:139–145.
- Lucier GW, McDaniel OS. 1977. Steroid and non-steroid UDP glucuronyltransferase: glucuronidation of synthetic estrogens as steroids. J Steroid Biochem 8:867–872.
- Lucier GW, Sonawane BR, McDaniel OS, Hook GE. 1975. Postnatal stimulation of hepatic microsomal enzymes following administration of TCDD to pregnant rats. Chem Biol Interact 11:15–26.
- Luquita MG, Catania VA, Pozzi EJ, Veggi LM, Hoffman T, Pellegrino JM, Ikushiro S, Emi Y, Iyanagi T, Vore M, and others. 2001. Molecular basis of perinatal changes in UDP-glucuronosyltransferase activity in maternal rat liver. J Pharmacol Exp Ther 298:49–56.
- Ma M, Kondo T, Ban S, Umemura T, Kurahashi N, Takeda M, Kishi R. 2006. Exposure of prepubertal female rats to inhaled di(2-ethylhexyl)phthalate affects the onset of puberty and postpubertal reproductive functions. Toxicol Sci 93:164–171.
- Mann S, Droz PO, Vahter M. 1996a. A physiologically based pharmacokinetic model for arsenic exposure. II. Validation and application in humans. Toxicol Appl Pharmacol 140:471–486.
- Mann S, Droz PO, Vahter M. 1996b. A physiologically based pharmacokinetic model for arsenic exposure. I. Development in hamsters and rabbits. Toxicol Appl Pharmacol 137:8–22.
- Martino-Andrade AJ, Chahoud I. 2010. Reproductive toxicity of phthalate esters. Mol Nutr Food Res 54:148–157.
- Meachem S, von Schonfeldt V, Schlatt S. 2001. Spermatogonia: stem cells with a great perspective. Reproduction 121:825–834.
- Meek ME, Bucher JR, Cohen SM, Dellarco V, Hill RN, Lehman-McKeeman LD, Longfellow DG, Pastoor T, Seed J, Patton DE. 2003. A framework for human relevance analysis of information on carcinogenic modes of action. Crit Rev Toxicol 33:591–653.
- Mennear J. 1988. Toxicology and Carcinogenesis Studies of Trichloroethylene in Four Strains of Rat. NTP Technical Report 273 (NIH Publication NO 88–2529).
- Mes J, Coffin DE, Campbell DS. 1974. Di-n-butyl-and di-2-ethylhexyl phthalate in human adipose tissue. Bull Environ Contam Toxicol 12:721–725.
- Mylchreest E, Sar M, Cattley RC, Foster PM. 1999. Disruption of androgen-regulated male reproductive development by di(n-butyl) phthalate during late gestation in rats is different from flutamide. Toxicol Appl Pharmacol 156:81–95.
- Mylchreest E, Sar M, Wallace DG, Foster PM. 2002. Fetal testosterone insufficiency and abnormal proliferation of Leydig cells and gonocytes in rats exposed to di(n-butyl) phthalate. Reprod Toxicol 16:19–28.
- Naessany S, Picon R. 1982. Onset of a feedback inhibition by testosterone in male rat fetuses. Biol Neonate 41:234–239.
- Naismith DJ, Richardson DP, Pritchard AE. 1982. The utilization of protein and energy during lactation in the rat, with particular regard to the use of fat accumulated in pregnancy. Br J Nutr 48:433–441.
- NAS (National Academy of Sciences). 1983. Risk Assessment in the Federal Government: Managing the Process. Washington, DC: National Academy Press.
- NAS (National Academy of Sciences). 2007. Toxicity Testing in the 21st century: A Vision and a Strategy. Washington, DC: The National Academy Press.
- NAS (National Academy of Sciences). 2008. Phthalates and cumulative risk assessment. Washington, DC: The National Acdemy Press.
- NAS (National Academy of Sciences). 2009. Science and Decisions: Advancing Risk Assessment. Washington, DC: National Academy Press.
- Neale MG, Parke DV. 1973. Effects of pregnancy on the metabolism of drugs in the rat and rabbit. Biochem Pharmacol 22:1451–1461.
- Ng KM, Chu J, Bronaugh RL, Franklin CA, Somers DA 1992. Percutaneous absorption and metabolism of pyrene, benzo[a]pyrene, and di(2-ethylhexyl)phthalate: comparison of in vitro and in vivo results in the hairless guinea pig. Toxicology and Applied Pharmacology 115(2):216–223.
- Noriega NC, Howdeshell KL, Furr J, Lambright CR, Wilson VS, Gray LE Jr. 2009. Pubertal administration of DEHP delays puberty, suppresses testosterone production, and inhibits reproductive tract development in male Sprague-Dawley and Long-Evans rats. Toxicol Sci 111:163–178.
- NTP (National Toxicology Program). 1982. Carcinogenesis bioassay of di(2-ethylhexyl)phthalate (CAS No. 117-81-7) in F344 rats and B6C3F1 mice (feed study). Research Triangle Park, NC: U.S. Department of Health and Human Services, Public Health Services, National Institute of Health. NTP publication no. 217.
- NTP (National Toxicology Program). 2004. Diethylhexylphthalate: Multigenerational Reproductive Assessment by Continuous Breeding When Administered to Sprague-Dawley Rats in the Diet. TRC Study No7244-200, NTP-RACB-98-004.
- O’Flaherty E. 1989. Interspecies conversion of kinetically equivalent doses. Risk Analysis 9:587–598.
- O’Flaherty EJ. 1995. Physiologically based models for bone-seeking elements. V. Lead absorption and disposition in childhood. Toxicol Appl Pharmacol 131:297–308.
- O’Flaherty EJ, Scott W, Schreiner C, Beliles RP. 1992. A physiologically based kinetic model of rat and mouse gestation: disposition of a weak acid. Toxicol Appl Pharmacol 112:245–256.
- Obourn JD, Frame SR, Bell RH, Jr., Longnecker DS, Elliott GS, Cook JC. 1997a. Mechanisms for the pancreatic oncogenic effects of the peroxisome proliferator Wyeth-14,643. Toxicol Appl Pharmacol 145:425–436.
- Obourn JD, Frame SR, Chiu T, Solomon TE, Cook JC. 1997b. Evidence that A8947 enhances pancreas growth via a trypsin inhibitor mechanism. Toxicol Appl Pharmacol 146:116–126.
- Olsen GW, Burris JM, Burlew MM, Mandel JH. 2000. Plasma cholecystokinin and hepatic enzymes, cholesterol and lipoproteins in ammonium perfluorooctanoate production workers. Drug Chem Toxicol 23:603–620.
- Olsen GW, Gilliland FD, Burlew MM, Burris JM, Mandel JS, Mandel JH. 1998. An epidemiologic investigation of reproductive hormones in men with occupational exposure to perfluorooctanoic acid. J Occup Environ Med 40:614–622.
- Overturf ML, Druilhet RE, Liehr JG, Kirkendall WM, Caprioli RM. 1979. Phthalate esters in normal and pathological human kidneys. Bull Environ Contam Toxicol 22:536–542.
- Park HW, Shepard TH. 1994. Volume and glucose concentration of rat amniotic fluid: effects on embryo nutrition and axis rotation. Teratology 49:465–469.
- Parks LG, Ostby JS, Lambright CR, Abbott BD, Klinefelter GR, Barlow NJ, Gray LE Jr. 2000. The plasticizer diethylhexyl phthalate induces malformations by decreasing fetal testosterone synthesis during sexual differentiation in the male rat. Toxicol Sci 58:339–349.
- Payan JP, Marty JP, Fabry JP, Beydon D, Boudry I, Ferrari E, Canel F, Grandclaude MC, Vincent CM. 2001. In vivo and in vitro percutaneous absorption of [(14)C]di-N-butylphthalate in rat. Drug Metab Dispos 29:843–854.
- Payne AH, Jaffe RB. 1972. Comparison of androgen synthesis in human fetal testis and adrenal: 3 -hydroxysteroid dehydrogenase-isomerase and 17 -steroid dehydrognase activities. Biochim Biophys Acta 279:202–207.
- Pollack GM, Li RC, Ermer JC, Shen DD. 1985. Effects of route of administration and repetitive dosing on the disposition kinetics of di(2-ethylhexyl) phthalate and its mono-de-esterified metabolite in rats. Toxicol Appl Pharmacol 79:246–256.
- Post SM, Duez H, Gervois PP, Staels B, Kuipers F, Princen HM. 2001. Fibrates suppress bile acid synthesis via peroxisome proliferator-activated receptor-alpha-mediated downregulation of cholesterol 7alpha-hydroxylase and sterol 27-hydroxylase expression. Arterioscler Thromb Vasc Biol 21:1840–1845.
- Rakusan K, Marcinek H. 1973. Postnatal development of the cardiac output distribution in rat. Biol Neonate 22:58–63.
- Rao MS, Subbarao V. 1995. Incidence of pancreatic and testicular tumors in rats treated with ciprofibrate, a peroxisome proliferator. Cancer Lett 97:185–188.
- Reitz RH, Mendrala AL, Corley RA, Quast JF, Gargas ML, Andersen ME, Staats DA, Conolly RB. 1990. Estimating the risk of liver cancer associated with human exposures to chloroform using physiologically based pharmacokinetic modeling. Toxicol Appl Pharmacol 105:443–459.
- Rider CV, Wilson VS, Howdeshell KL, Hotchkiss AK, Furr JR, Lambright CR, Gray LE Jr. 2009. Cumulative effects of in utero administration of mixtures of “antiandrogens” on male rat reproductive development. Toxicol Pathol 37:100–113.
- Rodricks JV, Swenberg JA, Borzelleca JF, Maronpot RR, Shipp AM. 2010. Triclosan: a critical review of the experimental data and development of margins of safety for consumer products. Crit Rev Toxicol 40:422–484.
- Rowland IR, Cottrell RC, Phillips JC. 1977. Hydrolysis of phthalate esters by the gastro-intestinal contents of the rat. Food Cosmet Toxicol 15:17–21.
- Rusyn I, Peters JM, Cunningham ML. 2006. Modes of action and species-specific effects of di-(2-ethylhexyl)phthalate in the liver. Crit Rev Toxicol 36:459–479.
- Rusyn I, Rose ML, Bojes HK, Thurman RG. 2000. Novel role of oxidants in the molecular mechanism of action of peroxisome proliferators. Antioxid Redox Signal 2:607–621.
- Sarangapani R, Gentry PR, Covington TR, Teeguarden JG, Clewell HJ 3rd. 2003. Evaluation of the potential impact of age- and gender-specific lung morphology and ventilation rate on the dosimetry of vapors. Inhal Toxicol 15:987–1016.
- Seed J, Carney EW, Corley RA, Crofton KM, DeSesso JM, Foster PM, Kavlock R, Kimmel G, Klaunig J, Meek ME, and others. 2005. Overview: Using mode of action and life stage information to evaluate the human relevance of animal toxicity data. Crit Rev Toxicol 35:664–672.
- Seo KW, Kim KB, Kim YJ, Choi JY, Lee KT, Choi KS. 2004. Comparison of oxidative stress and changes of xenobiotic metabolizing enzymes induced by phthalates in rats. Food Chem Toxicol 42:107–114.
- Shaban Z, Soliman M, El-Shazly S, El-Bohi K, Abdelazeez A, Kehelo K, Kim HS, Muzandu K, Ishizuka M, Kazusaka A, and others. 2005. AhR and PPARalpha: antagonistic effects on CYP2B and CYP3A, and additive inhibitory effects on CYP2C11. Xenobiotica 35:51–68.
- Short RD, Robinson EC, Lington AW, Chin AE. 1987. Metabolic and peroxisome proliferation studies with di(2-ethylhexyl)phthalate in rats and monkeys. Toxicol Ind Health 3:185–195.
- Sibinski L. 1988. Two-Year Oral (Diet) Toxicity/Carcinogenicity Study of Fluorochemical FC-143 in Rats (Riker Experiment No. 0281CR0012). 0281CR0012. Riker Laboratories, Inc. St. Paul, MN.
- Sjoberg P, Bondesson U, Hammarlund M. 1985. Non-linearities in the pharmacokinetics of di-(2-ethylhexyl) phthalate and metabolites in male rats. Arch Toxicol 58:72–77.
- Stolc V, Knopp J, Stolcova E. 1966. Iodine, solid diet, water and milk intake by lactating rats and their offsprings. Physiol Bohemoslov 15:219–225.
- Swan SH, Main KM, Liu F, Stewart SL, Kruse RL, Calafat AM, Mao CS, Redmon JB, Ternand CL, Sullivan S, and others. 2005. Decrease in anogenital distance among male infants with prenatal phthalate exposure. Environ Health Perspect 113:1056–1061.
- Tan YM, Liao KH, Clewell HJ 3rd. 2007. Reverse dosimetry: interpreting trihalomethanes biomonitoring data using physiologically based pharmacokinetic modeling. J Expo Sci Environ Epidemiol 17:591–603.
- Tan YM, Liao KH, Conolly RB, Blount BC, Mason AM, Clewell HJ. 2006. Use of a physiologically based pharmacokinetic model to identify exposures consistent with human biomonitoring data for chloroform. J Toxicol Environ Health A 69:1727–1756.
- Tanaka A, Matsumoto A, Yamaha T. 1978. Biochemical studies on phthalic esters. III. Metabolism of dibutyl phthalate (DBP) in animals. Toxicology 9:109–123.
- Tangoku A, Doi R, Chowdhury P, Blevins GT, Jr., Pasley JN, Rayford PL. 1993. Exogenous administration of estradiol and cholecystokinin alters exocrine pancreatic function in rats. Int J Pancreatol 13:81–86.
- Teirlynck OA, Belpaire F. 1985. Disposition of orally administered di-(2-ethylhexyl) phthalate and mono-(2-ethylhexyl) phthalate in the rat. Arch Toxicol 57:226–230.
- Thomford P. 2000. 4-Week Capsule Toxicity Study with Ammonium Perfluorooctanoate (APFO) in Cynomolgus Monkeys (Draft Report). 6329-230. Covance Laboratories, Inc. Madison, WI.
- Tucker M, Orton T. 1995. Comparative toxicology of hypolipidemic fibrates. Britol, PA: Taylor and Francis. p.
- Turek FW, Desjardins C. 1979. Development of Leydig cell tumors and onset of changes in the reproductive and endocrine systems of aging F344 rats. J Natl Cancer Inst 63:969–975.
- Ubel FA, Sorenson SD, Roach DE. 1980. Health status of plant workers exposed to fluorochemicals–a preliminary report. Am Ind Hyg Assoc J 41:584–589.
- USEPA (United States Environmental Protection Agency). 1986. Guidelines for Carcinogen Risk Assessment. Risk Assessment Forum. Washington, DC: U.S. Environmental Protection Agency. EPA/630/R-00/004.
- USEPA (United States Environmental Protection Agency). 1992. Draft report: a cross-species scaling factor for carcinogen risk assessment based on equivalence of mg/kg3/4/day. Federal Register 57(109):24152–24173.
- USEPA (United States Environmental Protection Agency). Guidelines for Carcinogen Risk Assessment. Review Draft. NCEA-F-0644. U.S. Environmental Protection Agency. Washington, D.C.
- USEPA. 1999. Guidelines for Carcinogen Risk Assessment. Risk Assessment Forum. Review Draft. July 1999, NCEA-F-0644.
- USEPA (United States Environmental Protection Agency). 2000a. Benchmark Dose Technical Guidance Document (External Review Draft). U.S. Environmental Protection Agency. Washington, DC. EPA/630/R-00/001.
- USEPA (United States Environmental Protection Agency). 2000b. Science Policy Handbook. Risk Characterization. Office of Research and Development, U.S. Environmental Protection Agency, Washington, DC. EPA 100-B-00-002.
- USEPA (United States Environmental Protection Agency). 2002. Child-Specific Exposure Factors Handbook. EPA Publication: EPA-600-P-00-002B.
- USEPA (United States Environmental Protection Agency). 2003. Proposed OPPTS Science Policy: PPARalpha-Mediated Hepatocarcinogenesis in Rodents and Relevance to Human health risk Assessments. U.S. Environmental Protection Agency, P. T. S. Office of Prevention.
- USEPA (United States Environmental Protection Agency). 2005. Guidelines for Carcinogen Risk Assessment Risk Assessment Forum. Washington, DC: U.S. Environmental Protection Agency. EPA/630/P-03/001b.
- Voss C, Zerban H, Bannasch P, Berger MR. 2005. Lifelong exposure to di-(2-ethylhexyl)-phthalate induces tumors in liver and testes of Sprague-Dawley rats. Toxicology 206:359–71.
- Walrath J. 1996. Final DuPont epidemiology surveillance report: Mortality and cancer incidence surveillance at Washington Works.
- Ward JM, Peters JM, Perella CM, Gonzalez FJ. 1998. Receptor and nonreceptor-mediated organ-specific toxicity of di(2-ethylhexyl)phthalate (DEHP) in peroxisome proliferator-activated receptor alpha-null mice. Toxicol Pathol 26:240–6.
- Wishart GJ. 1978. Functional heterogeneity of UDP-glucuronosyltransferase as indicated by its differential development and inducibility by glucocorticoids. Demonstration of two groups within the enzyme’s activity towards twelve substrates. Biochem J 174:485–489.
- Wittassek M, Wiesmuller GA, Koch HM, Eckard R, Dobler L, Muller J, Angerer J, Schluter C. 2007. Internal phthalate exposure over the last two decades–a retrospective human biomonitoring study. Int J Hyg Environ Health 210:319–333.
- Woodyatt NJ, Lambe KG, Myers KA, Tugwood JD, Roberts RA. 1999. The peroxisome proliferator (PP) response element upstream of the human acyl CoA oxidase gene is inactive among a sample human population: significance for species differences in response to PPs. Carcinogenesis 20:369–72.
- Wykoff MH. 1971. Weight changes of the developing rat conceptus. Am J Vet Res 32:1633–1635.