Abstract
The histamine H4 receptor binding affinity of 2-(4-methylpiperazin-1-yl)quinoxaline derivatives has been quantitatively analyzed in terms of Dragon descriptors. The derived QSAR models have provided rationales to explain the activity of titled derivatives. The descriptors identified in CP-MLR analysis have highlighted the role of path/walk 4-Randic shape index (PW4), mean square distance (MSD) index, topological charges (GGI9, JGI2, and JGI7), atomic properties in respective lags of 2D-autocorrelations (MATS7e, GATS7e, and MATS8p), and Burden matrix (BELm1) to explain the binding affinity. Certain structural fragments (C-002 and C-027) have also shown prevalence to optimize the H4R binding affinity of titled compounds. The PLS analysis has also confirmed the dominance of information content of CP-MLR-identified descriptors for modelling the activity.
Introduction
Histamine plays a role in many physiological processes such as inflammation and vasodilatation, gastric acid secretion, cognitive processes, regulation of food intake, sleep, and wakefulness. It is a member of G-protein-coupled receptors (GPCRs) family. At present, there are four histamine receptor subtypes, namely, H1R, H2R, H3R, and H4R. The antagonists of H1 and H2 receptors are widely used for the treatment of allergic disorders such as hay feverCitation1 and gastric ulcers,Citation2 respectively. The H3R antagonists are being assessed for clinical efficiency in attention-deficit hyperactivity disorder (ADHD), dementia, and narcolepsy.Citation3 The most recently identified histamine receptor subtypeCitation4–8 H4R has a diverse pharmacological profile than H1, H2, and H3R.Citation9 It is primarily expressed in haematopoietic and immune cells such as eosinophils, mast cells, and macrophages, as well as in peripheral tissues such as spleen, thymus, and bone marrow,Citation10 and plays a role in immunological and inflammatory processes. For the treatment of various chronic inflammatory diseases, such as inflammatory bowel disease, asthma, and rheumatoid arthritis,Citation11–13 and possible role in the proliferation of colon carcinoma cells, in the modulation of angiogenesis and in mediating pruritis,Citation14,Citation15 the H4R is being considered as a potential drug target.
Recently, a series of N-methylpiperazinylquinoxaline derivatives, as a novel class of potent H4R ligands, has been reported by de Esch and colleagues.Citation16 Apart from this, Kiss et al.Citation17 have reported structure-based virtual screening of >8.7 million diverse chemical entities from different database using a ligand-supported homology model of the human histamine H4 receptor (hH4R) and identified several novel scaffolds as selective H4 ligands. Several of these hits shared aryl, heteroaryl, and functionalized unsaturated chains as part of their structures. These results furthermore highlight the N-methylpiperazinylquinoxalines as potential H4R ligands. Moreover, in view of the importance of anti-inflammatory agents in the clinical management of several disorders, a quantitative structure–activity relationship is attempted on the binding affinity of these quinoxaline derivatives. The present study is aimed at rationalizing the substituent variations of these analogues to provide insight for the future endeavours.
Materials and methods
Dataset and molecular descriptors
The 45 reported N-methylpiperazinylquinoxaline derivatives [16] have been considered as the dataset for present QSAR study. The binding affinity of these compounds were measured by displacement of [3H]histamine binding using membranes of HEK cells transiently expressing the human H4R. The structural variations and binding affinity (as pKi) are mentioned in . The descriptors, accounting molecular features, for all the reported compounds have been calculated from Dragon software.Citation18 The input for this software was the energy-minimized 3D structures of the compounds drawn in ChemDraw software.Citation19 A total number of 458 descriptors corresponding to 0D-, 1D-, and 2D-classes of Dragon software have been considered in the present modelling study. Before model development procedure, all those descriptors that are intercorrelated beyond 0.90 and showing a correlation of <0.1 with the biological endpoints (descriptor versus activity, r < 0.1) were excluded. This procedure has reduced the total descriptors from 458 to 92 as relevant ones to explain the biological actions of titled compounds. In the dataset, the initial assessment of activity with the all descriptors has suggested the compound 4 as potential outlier. An outlier to a QSAR can indicate the limits of applicability of QSAR models.
Table 1. Structures and observed experimental binding affinity of N-methylpiperazinylquinoxaline derivatives.a
In these derivatives, two-ring heterocyclic scaffolds are directly connected to the N-methylpiperazine moiety. These analogues were synthesized on the basis of flexible alignment model of VUF6884 (a tricyclic clozapine analogue) and JNJ7777120 (an indole-N-methylpiperazine derivative).Citation20 The model suggested that both the compounds had overlapping binding mode for the H4 receptor. The reported quinoxaline derivatives are having the hybrid scaffold based on the structures of clozapine analogue and indole anologue. The alignment model suggested that substitution of quinoxaline with an additional aromatic ring system could occupy the aromatic pocket, which is also occupied by one of the aromatic rings of a tricyclic clozapine analogue when it binds to the H4R. Compound 4 may be treated as an outlier as it may not fit to an aromatic pocket and/or may furnish an additional interaction with an aromatic pocket. In view of this, the modelling study has been carried out without this analogue. Inclusion of this analogue to the dataset leads to statistically insignificant results.
Development and validation of model
The combinatorial protocol in multiple linear regression (CP-MLR)Citation21–25 and partial least-squares (PLS)Citation26–28 procedures have been used in the present study for developing QSAR models. The CP-MLR is a “filter”-based variable selection procedure, which employs a combinatorial strategy with MLR to result in selected subset regressions for the extraction of diverse structure–activity models, each having unique combination of descriptors from the generated dataset of the compounds under study. The embedded filters make the variable selection process efficient and lead to unique solution. Fear of “chance correlations” exists where large descriptor pools are used in multilinear QSAR/QSPR studies.Citation29,Citation30 In view of this, to find out any chance correlations associated with the models recognized in CP-MLR, each cross-validated model has been subjected to randomization testCitation31,Citation32 by repeated randomization (100 simulation runs) of the biological responses. The datasets with randomized response vector have been reassessed by multiple regression analysis. The resulting regression equations, if any, with correlation coefficients better than or equal to the one corresponding to unscrambled response data were counted. This has been used as a measure to express the percent chance correlation of the model under scrutiny.
Validation of the derived model is necessary to test its prediction and generalization within the study domain. The dataset of the present study is randomly divided into training set for model development and test set for external prediction or validation. The compounds of test set were randomly picked using an in-house written randomization program.
For each model, derived by involving n data points, a number of statistical parameters such as r (the multiple correlation coefficient), s (the standard deviation), F (the F ratio between the variances of calculated and observed activities), and Q2LOO (the cross-validated index from leave-one-out procedure) have been obtained to access its overall statistical significance. In case of internal validation, Q2LOO is used as a criterion of both robustness and predictive ability of the model. A value greater than 0.5 of Q2 index suggests a statistically significant model. The predictive power of derived model is based on test set compounds. The model obtained from training set has a reliable predictive power if the value of the r2Test (the squared correlation coefficient between the observed and predicted values of compounds from test set) is greater than 0.5.
Applicability domain
The usefulness of a model is based on its accurate prediction ability for new congeners. A model is valid only within its training domain and new compounds must be assessed as belonging to the domain before the model is applied. The applicability domain (AD) is evaluated by the leverage values for each compound.Citation33 A Williams plot (the plot of standardized residuals versus leverage values (h)) is constructed, which can be used for a simple graphical detection of both the response outliers (Y outliers) and structurally influential chemicals (X outliers) in the model. In this plot, the AD is established inside a squared area within ±x standard deviations and a leverage threshold h*, which is generally fixed at 3(k + 1)/n (n is the number of training set compounds and k is the number of model parameters), whereas x = 2 or 3. If the compounds have a high leverage value (h > h*), then the prediction is not trustworthy. On the other hand, when the leverage value of a compound is lower than the threshold value, the probability of accordance between predicted and observed values is as high as that for the training set compounds.
Results and discussion
QSAR results
In multidescriptor class environment, exploring for best model equation(s) along the descriptor class provides an opportunity to unravel the phenomenon under investigation. In other words, the concepts embedded in the descriptor classes relate the biological actions revealed by the compounds. For the purpose of modelling study, 14 compounds have been included in the test set for the validation of the models derived from 30 training set compounds. A total number of 92 significant descriptors from 0D-, 1D-, and 2D-classes have been subjected to CP-MLR analysis with default “filters” set in it. Statistical models in two and three descriptor(s) have been derived successively to achieve the best relationship correlating H4R binding affinity. A total number of 6 and 12 models in two and three descriptors, respectively, were obtained through CP-MLR. These models (with 92 descriptors) were identified in CP-MLR by successively incrementing the filter-3 with increasing number of descriptors (per equation). For this, the optimum r-bar value of the preceding level model has been used as the new threshold of filter-3 for the next generation. The selected models in two and three descriptors are given below.
n = 30, r = 0.804, s = 0.513, F = 24.639, Q2LOO = 0.574, Q2L5O = 0.532, r2randY(sd) = 0.255(0.148), r2Test = 0.607
n = 30, r = 0.743, s = 0.578, F = 16.616, Q2LOO = 0.443, Q2L5O = 0.361, r2randY(sd) = 0.241(0.126), r2Test = 0.513
n = 30, r = 0.857, s = 0.454, F = 23.883, Q2LOO = 0.674, Q2L5O = 0.677, r2randY(sd) = 0.314(0.141), r2Test = 0.559
n = 30, r = 0.848, s = 0.466, F = 22.175, Q2LOO = 0.644, Q2L5O = 0.580, r2randY(sd) = 0.282(0.108), r2Test = 0.589
In above and all follow-up regression equations, the values given in the parentheses are the standard errors of the regression coefficients. The r2randY(sd) is the mean random-squared multiple correlation coefficient of the regressions in the activity (Y) randomization study with its standard deviation from 100 simulations. In the randomization study (100 simulations per model), none of the identified models has shown any chance correlation. The signs of the regression coefficients suggest the direction of influence of explanatory variables in the models.
The descriptor BELm1 belongs to BCUT class of Dragon descriptors. The BCUT descriptors are the first eight highest and the lowest absolute eigenvalues, BEHwk and BELwk, respectively, for the modified Burden adjacency matrix. Here, w refers to the atomic property and k to the eigenvalue rank. The ordered sequence of the highest and the lowest eigenvalues reflect upon the relevant aspects of molecular structure, useful for similarity searching. The negative contribution of descriptor BELm1 to the activity advocates that a higher value of this descriptor is detrimental to the activity.
The other participated descriptors, GGI9, PW4, MATS7e, and C-002, belong to Galvez topological charge indices (GALVEZ), topological (TOPO), 2D-autocorrelations (2D-AUTO), and atom-centred fragment (ACF) classes of Dragon descriptors, respectively. GALVEZ descriptors are the Galvez topological charge indices and have their origin in the first 10 eigenvalues of the polynomial of corrected adjacency matrix of the compounds. All the GALVEZ class descriptors belong to two categories. Of this, one category corresponds to the topological charge index of order n (GGIn) and the other to the mean topological charge index of order n (JGIn), where “n” represents the order of eigen value. The negative influence of descriptor GGI9 (topological charge index of ninth order) from this class to the activity suggested that a lower value of ninth order charge index would be beneficiary to the activity. The TOPO class descriptors are based on a graphical representation of the molecule and are numerical quantifiers of molecular topology obtained by the application of algebraic operators to matrices representing molecular graphs and whose values are independent of vertex numbering or labelling. They can be sensitive to one or more structural features of the molecule such as size, shape, symmetry, branching, and cyclicity and can also encode chemical information concerning atom type and bond multiplicity. The descriptor PW4, participated in above models, is representative of TOPO class. The descriptor PW4, path/walk ratio 4, is the Randic’s molecular shape descriptor and its value increases with increased branching in the vertices. The sign of regression coefficient of this descriptor shows positive influence on the activity. A higher value of this descriptor would be in favour of the activity.
The 2D-autocorrelations are molecular descriptors, which describe how a considered property is distributed along a topological molecular structure. The 2D-AUTO descriptors have their origin in autocorrelation of topological structure of Broto-Moreau (ATS), of Moran (MATS) and of Geary (GATS). The computation of these descriptors involves the summations of different autocorrelation functions corresponding to the different fragment lengths and leads to different autocorrelation vectors corresponding to the lengths of the structural fragments. Also a weighing component in terms of a physicochemical property has been embedded in this descriptor. As a result, these descriptors address the topology of the structure or parts thereof in association with a selected physicochemical property. In these descriptors’ nomenclature, the penultimate character, a number, indicates the number of consecutively connected edges considered in its computation and is called as the autocorrelation vector of lag n (corresponding to the number of edges in the unit fragment). The very last character of the descriptor’s nomenclature indicates the physicochemical property considered in the weighing component—m for mass or v for volume or e for Sanderson electronegativity or p for polarizability—for its computation. It is evident from the sign of regression coefficient of the participating descriptor that descriptor MATS7e from this class has contributed positively to the activity. Thus a higher positive value of descriptor MATS7e (Moran autocorrelation of lag 7 weighed by atomic Sanderson electronegativities) will be in favour of activity. ACF descriptors are simple molecular descriptors defined as the number of specific atom types in a molecule and their calculation is based on the knowledge of the molecular composition and atom connectivities. The ACF class descriptor C-002, representing a CH2R2 fragment in a molecular structure, has shown negative influence on the activity suggesting absence of such type of fragment for improved activity.
The three descriptor models could estimate nearly 72% in observed activity of the compounds. Considering the number of observation in the dataset, models with up to four descriptors were explored. It has resulted in 19 four-parameter models with test set r2 > 0.50. These models have shared 18 descriptors among them. All these 18 descriptors along with their brief meaning, average regression coefficients, and total incidence are listed in , which will serve as a measure of their estimate across these models. Following are some four-descriptor models for the activity.
Table 2. Physical meaning, average regression coefficients and the total incidences, and MLR-like coefficients from PLS model of descriptors identified from four-parameter CP-MLR models for the binding affinity of N-methylpiperazinylquinoxaline derivatives.
n = 30, r = 0.891, s = 0.406, F = 24.255, Q2LOO = 0.748, Q2L5O = 0.744, r2randY(sd) = 0.350(0.095), r2Test = 0.539
n = 30, r = 0.888, s = 0.412, F = 23.337, Q2LOO = 0.738, Q2L5O = 0.738, r2randY(sd) = 0.335(0.125), r2Test = 0.531
n = 30, r = 0.882, s = 0.423, F = 21.795, Q2LOO = 0.598, Q2L5O = 0.632, r2randY(sd) = 0.375(0.126), r2Test = 0.558
n = 30, r = 0.881, s = 0.424, F = 21.629, Q2LOO = 0.658, Q2L5O = 0.665, r2randY(sd) = 0.353(0.125), r2Test = 0.543
These models have accounted for nearly 77% variance in the observed activities. In the randomization study (100 simulations per model), none of the identified models has shown any chance correlation. The values greater than 0.5 of Q2 index is in accordance to a reasonable robust QSAR model. The pKi values of training set compounds calculated using Eqs. (5)–(8) and predicted from LOO procedure have been included in . The models (5)–(8) are validated with an external test set of 14 compounds listed in . The predictions of the test set compounds based on external validation are found to be satisfactory as reflected in the test set r2 (r2Test) values and the same is reported in . The plot showing goodness of fit between observed and calculated activities for the training and test set compounds is given in .
Table 3. Observed and modeled binding affinity of N-methylpiperazinylquinoxaline derivatives included in the training set.
Table 4. Observed, modelled, and residuala binding affinity of N-methylpiperazinylquinoxaline derivatives included in the test set.
Figure 1. Plot of observed versus calculated pKi values for the training (Δ) and test set (○) compounds. A, B, C, and D correspond, respectively, to four-parameter models (5), (6), (7), and (8) identified through CP-MLR. E corresponds to the MLR-like PLS equation from 18 CP-MLR-identified descriptors.
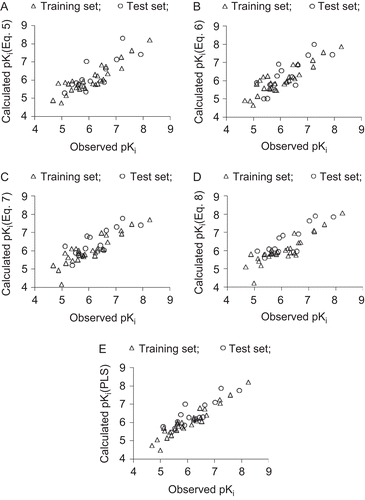
The newly appeared descriptors in above models are C-027 (an ACF class descriptor), GATS7e and MATS8p (2D-AUTO descriptors), MSD (TOPO class descriptor), JGI2 and JGI7 (both from GALVEZ class). Above equations reveal that a higher value of Balaban mean square distance (MSD) index, second order mean topological charge index (JGI2), and Moran autocorrelation of lag 8 weighed by atomic polarizabilities (MATS8p) are advantageous to enhance the activity. A higher value of seventh order mean topological charge index (JGI7) and Geary autocorrelation of lag 7 weighed by atomic Sanderson electronegativities (GATS7e) are detrimental to the activity. Counts for certain structural fragment, R-CH-X (descriptor C-027) strongly recommend the absence of such structural features favourable to activity. Thus the descriptors identified for rationalizing the activity give avenues to modulate the structure to a desirable biological endpoint.
In model Eqs. (1)–(8), the participated descriptors have indicated that the steric effect explained through molecular size, shape, and spacer (BELm1, PW4, MSD), the electronic effect (MATS7e, GATS7e, GGI9, JGI2, JGI7), the polarizability, accounting for both polarity and hydrophobicity (MATS8p), and certain structural fragments (C-002, C-027) played significant role in explaining the binding affinity of N-methylpiperazinylquinoxaline derivatives. However, the electronic and steric effects have appeared to impart dominant character in rationalizing the binding activity of titled compounds.
A partial least square (PLS) analysis has been carried out on these 18 CP-MLR identified descriptors () to facilitate the development of a “single window” structure–activity model. For the purpose of PLS, the descriptors have been autoscaled (zero mean and unit SD) to give each one of them equal weight in the analysis. In the PLS cross-validation, four components are found to be the optimum for these 18 descriptors and they explained 91.20% variance in the activity (r2 = 0.912, Q2LOO = 0.872, s = 0.265, F = 65.153, r2Test = 0.666). The MLR-like PLS coefficients of these 18 descriptors are given in . For the sake of comparison, the plot showing goodness of fit between observed and calculated activities (through PLS analysis) for the training and test set compounds is also given in . shows a plot of the fraction contribution of normalized regression coefficients of these descriptors to the activity ().
Figure 2. Plot of fraction contribution of MLR-like PLS coefficients (normalized) of the 18 descriptors () to the activity. The 10 most significant descriptors are identified by black-shaded lines. The numerals on bars are the order of the descriptors.
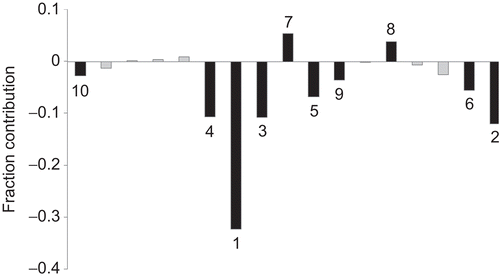
The PLS analysis has also suggested BELm1 (a BCUT class descriptor) as the most determining descriptor for modelling the activity of the compounds (descriptor S. No. 7 in ; ). The other nine significant descriptors in decreasing order of significance are C-027, GGI9, CIC5, JGI7, C-002, JGI2, MATS7e, MATS4v, and nF (descriptors S. Nos. 17, 8, 6, 10, 16, 9, 13, 11, and 1 in ; ). Of these descriptors, C-027, GGI9, JGI7, C-002, JGI2, MATS7e are part of Eqs. (1)–(8) and convey same inference in the PLS model as well. Among remaining ones, CIC5 belongs to TOPO class. The sign of regression coefficient of descriptor CIC5 (complementary information content of fifth order neighbourhood symmetry) suggests its detrimental nature to activity. The negative regression coefficient of the Moran autocorrelation of lag 4 weighed by atomic van der Waals volumes (descriptor MATS4v) advocates that a higher positive value of it is detrimental to the activity. The CONST class descriptor nF recommends a lower number or absence of fluorine atoms in a molecular structure for elevated activity. In comparison with these 10 descriptors, the remaining ones appear in lower order of significance to influence the activity of the compounds (; ). It is also observed that PLS model from the dataset devoid of 18 descriptors () is inferior in explaining the activity of the analogues.
Applicability domain
On analyzing the model AD in the Williams plot () of the model based on the whole dataset (), it has appeared that none of the compounds were identified as an obvious outlier for the H4R binding affinity if the limit of normal values for the Y outliers (response outliers) was set as 2.5 (standard deviation) units. None of the compounds was found to have leverage (h) values greater than the threshold leverages (h*). For both the training set and test set, the suggested model matches the high-quality parameters with good fitting power and the capability of assessing external data. Furthermore, almost all of the compounds was within the AD of the proposed model and were evaluated correctly.
Table 5. Resultant models for the whole dataset (n = 44) in descriptors of training set models.
Conclusions
This study has provided a rational approach for the development of new N-methylpiperazinylquinoxaline derivatives as H4 receptor ligands. The descriptors identified in CP-MLR analysis have highlighted the role of path/walk 4-Randic shape index (PW4), MSD, topological charges (GGI9, JGI2, and JGI7), atomic properties in respective lags of 2D-autocorrelations (MATS7e, GATS7e, and MATS8p), and Burden matrix (BELm1) to the activity. Certain structural fragments have also shown prevalence to optimize the H4R binding affinity of titled compounds. The PLS analysis has confirmed the dominance of information content of CP-MLR identified descriptors for modelling the activity when compared with those of leftover ones.
Acknowledgements
Authors are thankful to their Institution for providing necessary facilities to complete this study. CDRI communication no. 7960.
Declaration of interest
The authors report no conflicts of interest. The authors alone are responsible for the content and writing of the paper.
References
- Hill SJ. Distribution, properties, and functional characteristics of three classes of histamine receptor. Pharmacol Rev 1990;42:45–83.
- Black JW, Duncan WA, Durant CJ, Ganellin CR, Parsons EM. Definition and antagonism of histamine H2-receptors. Nature 1972;236:385–390.
- Celanire S, Wijtmans M, Talaga P, Leurs R, de Esch IJP. Histamine H3 receptor antagonists reach out for the clinic. Drug Discov Today 2005;23/24:1613–1627.
- Liu C, Ma X-J Jiang, X, Wilson SJ, Hofstra CL, Blevitt K, Li X, Chai W, Carruthers N, Lovenberg TW. Cloning and pharmacological characterization of a fourth histamine receptor (H4) expressed in bone marrow. Mol Pharmacol 2001;59:420–426.
- Morse KL, Behan J, Laz TM, West RE Jr, Greenfender SA, Anthes JC, Umland S, Wan Y, Hipkin RW, Gonsiorek W, Shin N, Gustafson EL, Qiao X, Wang S, Hedrick JA, Green J, Bayne M, Monsma FJ Jr. Cloning and characterization of a novel human histamine receptor. J Pharmacol Exp Ther 2001;296:1058–1066.
- Nguyen T, Shapiro DA, George SR, Setola V, Lee DK, Cheng R, Rauser L, Lee SP, Lynch KR, Roth BL, O’Dowd BF. Discovery of a novel member of the histamine receptor family. Mol Pharmacol 2001;59:427–433.
- Oda T, Morikawa N, Saito Y, Masuho Y, Matsumoto S. Molecular cloning and characterization of a novel type of histamine receptor preferentially expressed in leukocytes. J Biol Chem 2000;275:36781–36786.
- Zhu Y, Michalovich D, Wu H-L Tan, KB, Dytko GM, Mannan IJ, Boyce R, Alston J, Tierney LA, Li X, Herrity NC, Vawter L, Sarau HM, Ames RS, Davenport CM, Hieble JP, Wilson S, Bergsma DJ, Fitzgerald LR. Cloning, expression, and pharmacological characterization of a novel human histamine receptor. Mol Pharmacol 2001;59:434–444.
- de Esch IJP, Thurmond RLJA, Jongejan A, Leurs R. The histamine H4 receptor as a new therapeutic target for inflammation. Trends Pharmacol Sci 2005;26:462–469.
- Gantner F, Sakai K, Tusche MW, Cruikshank WW, Center DM, Bacon KB. Histamine H4 and H2 receptors control histamine induced interleukin-16 release from human CD8+ T cells. J Pharmacol Exp Ther 2002;303:300–307.
- Varga C, Horvath K, Berko A, Thurmond RL, Dunford PJ, Whittle BJ. Inhibitory effects of histamine H4 receptor antagonists on experimental colitis in the rat. Eur J Pharmacol 2005;522:130–138.
- Thurmond RL, Desai PJ, Dunford PJ, Fung-Leung WP, Hofstra CL, Jiang W, Nguyen S, Riley JP, Sun S, Williams KN, Edwards JP, Karlsson L. A potent and selective histamine H4 receptor antagonist with anti-inflammatory properties. J Pharmacol Exp Ther 2004;309:404–413.
- Ikawa Y, Suzuki M, Shiono S, Ohki E, Moriya H, Negishi E, Ueno K. Histamine H4 receptor expression in human synovial cells obtained from patients suffering from rheumatoid arthritis. Biol Pharm Bull 2005;10:2016–2018.
- Bell JK, McQueen DS, Rees JL. Involvement of histamine H4 and H1 receptors in scratching induced by histamine receptor agonists in BalbC mice. Br J Pharmacol 2004;142:374–380.
- Cianchi F, Cortesini C, Schiavone N, Perna F, Magnelli L, Fanti E, Bani D, Messerini L, Fabbroni V, Perigli G, Capaccioli S, Masini E. The role of cyclooxygenase-2 in mediating the effects of histamine on cell proliferation and vascular endothelial growth factor production in colorectal cancer. Clin Cancer Res 2005;19(Pt 1):6807–6815.
- Smits RA, Lim HD, Hanzer A, Zuiderveld OP, Guaita E, Adami M, Coruzzi G, Leurs R, de Esch IJP. Fragment based design of new H4 receptor-ligands with anti-inflammatory properties in vivo. J Med Chem 2008;51:2457–2467.
- Kiss R, Kiss B, Könczöl Á, Szalai F, Jelinek I, László V, Noszál B, Falus A, Keserű GM. Discovery of novel human histamine H4 receptor ligands by large-scale structure-based virtual screening. J Med Chem 2008;51:3145–3153.
- Todeschini R, Consonni V. Dragon software (version 1.11-2001). Milano, Italy.
- Chemdraw Ultra 6.0 and Chem3D Ultra. Cambridge Soft Corporation, Cambridge, USA.
- Smits RA, Lim HD, Stegink B, Bakker RA, de Esch IJP, Leurs R. Characterization of the histamine H4 receptor binding site: Part I. Synthesis and pharmacological evaluation of dibenzodiazepine derivatives. J Med Chem 2006;49:4512–4516.
- Prabhakar YS. A combinatorial approach to the variable selection in multiple linear regression: analysis of Selwood et al. data set—a case study. QSAR Comb Sci 2003;22:583–595.
- Sharma BK, Pilania P, Singh P. Modeling of cyclooxygnase-2 and 5-lipooxygenase inhibitory activity of apoptosis inducing agents potentially useful in prostate cancer chemotherapy: the derivatives of diarylpyrazole. J Enz Inhib Med Chem 2009;24:607–615.
- Sharma S, Sharma BK, Pilania P, Singh P, Prabhakar YS. Modeling of the growth hormone secretagogue receptor antagonistic activity using chemometric tools. J Enz Inhib Med Chem 2009;24:1024–1033.
- Sharma BK, Pilania P, Singh P, Prabhakar YS. Combinatorial protocol in multiple linear regression/partial least-squares directed rationale for the caspase-3 inhibition activity of isoquinoline-1,3,4-trione derivatives. SAR QSAR Environ Res 2010;21:169–185.
- Sharma BK, Pilania P, Sarbhai K, Singh P, Prabhakar YS. Chemometric descriptors in modeling the carbonic anhydrase inhibition activity of sulfonamide and sulfamate derivatives. Mol Divers 2010;14:371–384.
- Wold S. Cross-validatory estimation of the number of components in factor and principal components models. Technometrics 1978;20:397–405.
- Kettaneh N, Berglund A, Wold S. PCA and PLS with very large data sets. Comput Stat Data Anal 2005;48:69–85.
- Stahle L, Wold S. Multivariate data analysis and experimental design. In: Ellis GP, West WB. Eds., Biomedical research. Progress in medicinal chemistry. Elsevier Science Publishers, BV, Amsterdam. 1988;25:291–338.
- Topliss JG, Edwards RP. Chance factors in studies of quantitative structure–activity relationships. J Med Chem 1979;22:1238–1244.
- Katritzky AR, Dobchev DA, Slavov S, Karelson M. Legitimate utilization of large descriptor pools for QSAR/QSPR models. J Chem Inf Model 2008;48:2207–2213.
- So S-S, Karplus M. Three-dimensional quantitative structure–activity relationship from molecular similarity matrices and genetic neural networks. 1. Method and validation. J Med Chem 1997;40:4347–4359.
- Prabhakar YS, Solomon VR, Rawal RK, Gupta MK, Katti SB. CP-MLR/PLS directed structure–activity modeling of the HIV-1 RT inhibitory activity of 2,3-diaryl-1,3-thiazolidin-4-ones. QSAR Comb Sci 2004;23:234–244.
- Gramatica P. Principles of QSAR models validation: internal and external. QSAR Comb Sci 2007;26:694–701.