Abstract
In this paper, a receptor-based virtual screening study for the identification of estrogen receptor β (ERβ) ligands was developed. Starting from a commercial database of 400 000 molecules, only six compounds resulted to be potential active ligands of ERβ. Interestingly, all the six molecules possess scaffolds that had already been reported in known ERβ ligands. Therefore, the results obtained herein confirm the reliability of our virtual screening procedure, thus encouraging the application of this protocol to larger commercial databases in order to identify new ERβ ligands.
Introduction
Estrogen receptors (ERs) are ligand-activated transcription factors that belong to the nuclear hormone receptor superfamily. Like other steroid hormone receptors, ERs act as dimers to regulate transcriptional activation through the up- and down-regulation of gene expression in target tissuesCitation1. Estrogens, the endogenous ligands of the ERs, are the main female sex steroids, which control many cellular processes, including growth, differentiation and function of the reproductive systems. Anyway, evidences of the involvement of estrogens through their interaction with the ERs in a broader range of physiological and pathological processes have been ascertained. Not only did they prove to be implicated also in the regulation of male reproductive processes, they also showed notable effects on the cardiovascular system, in bone and adipose tissues, as well as in different organs such as brain, colon and prostateCitation2. Two different ER subtypes are known: estrogen receptor α (ERα) and β (ERβ); they are transcribed from different genes located on separate chromosomes and display discrete expression patterns as well as distinct ligand specificities. Indeed, the two ER subtypes fulfill different functions that are often opposite to each other and have a different impact in the various tissues and cell types that present a different expression rate of the two receptor subtypes. In vivo studies carried out with mice lacking either ERα or ERβ showed how the estrogenic effects on the “classical” estrogen target tissues such as uterine, mammary gland and hypothalamic/pituitary gland tissues, as well as bone tissue, are mainly due to ERα activation. On the other hand, ERβ seems to have stronger effects on non-reproductive organ systems such as prostate and colon, as well as on the cardiovascular and central nervous systems. Indeed, ERβ proved to be involved in several pathological states affecting these tissuesCitation3,Citation4. The expression of ERβ in prostate cancer and colon cancer, and its role in the development and progression of these malignancies have been extensively reviewed and discussed elsewhereCitation2,Citation5. Since ERβ exerts anti-proliferative, differentiative and proapoptotic actions in these tissues, contrarily to ERα which plays important roles in promoting cell growth and proliferation, it clearly represents a promising potential target for anti-cancer therapies, and small-molecule ligands endowed with selectivity toward this receptor subtype may be used as therapeutic agents for the treatment of these malignanciesCitation2. ERβ appears to exert tumor-suppressing actions also in the development and progression of ovarian cancer. Ovarian carcinogenesis is accompanied by a disruption of the balance between proliferation and apoptosis by the down-regulation of ERβ, and a reduced expression of this receptor was also shown to be associated with a high-lymph node metastasis rateCitation6. On the contrary, an overexpression of ERβ exerted strong antitumor effects on SK-OV-3 ovarian cancer cell lines, in terms of inhibition of growth and motility, as well as induction of apoptosisCitation7. These data suggest that ERβ selective ligands could be also employed for the treatment of ovarian cancer. Regarding breast cancer, the exact implications of ERβ are still unclear: it seems to have a pro-apoptotic and anti-proliferative action when co-expressed with ERα in breast cancer cells (in the majority of cases); however, it could also promote growth and survival of ERα-negative tumors. For this reason, the role of ERβ in the development and progression of breast cancer has been defined as “bi-faceted”. Therefore, selective ERβ ligands could help in shedding light on this issue, by broadening the molecular understanding of the involvement of ERβ in this kind of tumor, but they also might represent a valid alternative to its canonic ERα-targeted therapeutic treatmentsCitation8,Citation9. Finally, very recently ERβ proved to have a deep impact in neuroinflammation, by promoting neuroprotecting effects in oligodendrocytes. Therapeutic treatment with the ERβ ligand DPN (2,3-bis-(4-hydroxyphenyl)-proprionitrile) led to remyelination and restoration of functional axon conduction in a mouse model of multiple sclerosis through the activation of the PI3K/Akt/mTOR signaling pathway, which is required for oligodendrocytes survival and for axon myelinationCitation10. Moreover, Wu and co-workers found that ERβ, but not ERα, is expressed in mouse microglia, thus revealing ERβ as the unique mediator of estrogens inhibitory effects on microglia in the production of inflammatory molecules and in the recruitment of T-cells and macrophages. They proved that ERβ ligands can down-regulate the expression of NF-kB and iNOS in both microglia and T-cells. All these results suggest ERβ selective agonists as valid therapeutic candidates for treating neurodegenerative diseases such as multiple sclerosis, amyothrophic lateral sclerosis and Parkinson's diseaseCitation11.
Due to their numerous possible therapeutic applications, the interest in the development of new ERβ-selective ligands is constantly growing. Thus far, most of the researches in the field of estrogens have been dedicated to the selective estrogen receptor modulators (SERMs), ER ligands that are able to produce a spectrum of both agonist and antagonist actions in different target tissuesCitation12. This particular behavior could be explained considering that, in order to exert their various physiological roles, ERs interact with a high number of diverse transcriptional cofactors (coactivators and corepressors) that are not functionally equivalent and present different expression levels in different tissues and cell typesCitation13. Ligands endowed with both a SERM profile and selectivity toward ERβ subtype could provide the basis for more subtle and manageable pharmaceutical therapies. At present, only a limited number of ER agonist showing a remarkable selectivity toward ERβ have been reported in the literature. This is due to the fact that the ligand binding domains of the two receptor subtypes are very similar, in spite of a 56% of sequence identity because they have only two pairs of non-conserved residues in their ligand binding pocketCitation14. Among the computational studies concerning ER ligands reported so far in the literature, only a small number of virtual screening (VS) procedures have been developed and applied for the search of novel ERβ-selective agonists/SERMs. Nonetheless, some successful examples can be found within this small set of studies, where new ligands endowed with appreciable activity for ERβ were discovered, as well as SERM-like compounds with both an ERβ-agonist and an ERα-antagonist profilesCitation15–18. In these VS studies, a structure-based approach and in particular docking methods proved to be reliable in-silico tools for the identification of new ERβ agonists/SERMs.
In the present study, we focused our attention on the analysis of the reliability of various docking packages and scoring functions in discriminating active from inactive ERβ ligands, with the aim of identifying the best docking procedure to filter ERβ ligands from a commercial database. Then molecular dynamic (MD) simulations were carried out to further refine the screening and to identify new potential ERβ ligands.
Methods
ERβ-ligand complex structures and cross-docking
A total of 18 human ERβ structures complexed with agonists, available from the Protein Data BankCitation19, were used in this study (). Hydrogen atoms were added by means of Maestro 9.0Citation20. The ligands were extracted from the X-ray complexes and then subjected to a conformational search of 1000 steps in a water environment (using the generalized Born/surface-area model) by means of MacromodelCitation21. The algorithm used was the Monte Carlo method with the MMFFs force field and a distance-dependent dielectric constant of 1.0. The ligands were then minimized using the conjugated gradient method until a convergence value of 0.05 kcal/(Å mol), using the same force field and parameters as for the conformational search. The α carbons of the ERβ structures were aligned with each other using a reference structure, then each minimized ligand was docked in turn into all the 18 available X-ray structures by means of AUTODOCK 4.0 (AD4)Citation22, DOCK 6.5Citation23, FRED 2.2.5Citation24, GLIDE 5.0Citation25, GOLD 4.1Citation26, and AUTODOCK VINA 1.0Citation27 software packages. The docking results were evaluated through a comparison between the found docked positions of the ligand and the experimental ones. As a measure of docking reliability, the root mean square deviation (RMSD) between the position of heavy atoms of the ligand in the calculated and experimental structures was taken into account. The RMSD analysis was carried out using the rms_analysis software of the GOLD suite.
Table 1. Active ERβ agonists used in the enriched dataset.
Docking procedures
For all docking analyses, only the best pose was taken into account.
AUTODOCK 4.0
The AUTODOCK Tools utilitiesCitation28 were used in order to identify the torsion angles in the ligands, add the solvent model and assign the Gasteiger atomic charges to proteins and ligands. The regions of interest used by AUTODOCKCitation22 were defined by considering the reference ligand as the central group of a grid box of 10 Å in the x, y and z directions. A grid spacing of 0.375 Å and a distance-dependent function of the dielectric constant were used for the energetic map calculations. Using the Lamarckian genetic algorithm, the docked compounds were subjected to 20 runs of the AUTODOCK search using 2 500 000 steps of energy evaluation and the default values of the other parameters.
DOCK 6.5
The molecular surface of the binding site was calculated by means of the MS programCitation23, generating the Connolly surface with a probe with a radius of 1.4 Å. The points of the surface and the vectors normal to it were used by the Sphgen program to build a set of spheres, with radii varying from 1.4 to 4 Å that describe, from a stereoelectronic point of view, the negative image of the site. The spheres within a radius of 10 Å from the reference ligand were used to represent the site. For each ligand, DOCK 6.5 calculated 500 orientations; of these, the best grid and AMBER scored were taken into consideration. The ligand charge was calculated using the AM1-BCC method, as implemented in the Antechamber suite of AMBER 11Citation29.
FRED 2.2.5
FREDCitation24 requires a set of input conformers for each ligand. The conformers were generated by OMEGA2Citation30; in order to avoid bias the ligands obtained from the conformational search using Macromodel (see above) were used as starting structures. We applied the following modifications to the default settings of OMEGA2: the energy window was set to 50.0, the maximum number of output conformers was set to 10 000, the time limit was set to 1200, and the RMSD value below which two conformations were considered to be similar was set at 0.3 Å. The region of interest for the docking studies was defined in such a manner that it contained all residues which stayed within 10 Å from the ligand in the X-ray structures. The FRED docking method roughly consisted of two steps: shape fitting and optimization. During shape fitting, the ligand was placed into the binding site using a smooth Gaussian potential. A series of three optimization filters was then processed, which consisted of refinement of the positions of the hydroxyl hydrogen atoms of the ligand, rigid body optimization and optimization of the ligand pose in the dihedral angle space. In the last optimization step, the Chemgauss2 scoring function was used. After the docking calculation, the poses were scored independently by the six available scoring functions, i.e. Chemgauss3 (CG3), Chemscore (CS), Plp (PLP), OEChemscore (OCS), Screenscore (SCR), Shapegauss (SHA) and by a combination of them (FRED_ALL). CG3 is the third version of the Chemgauss scoring function, which uses smooth Gaussian functions to represent the shape and chemistry of molecules. CS was derived empirically from a set of 82 protein–ligand complexes for which measured binding affinities were available, and it was trained by regression against measured affinity data. PLP is a knowledge-based simplified energetic model that includes intramolecular energy terms for the ligand, given by torsional and non-bonded functions, as well as intermolecular ligand–protein steric and hydrogen-bond interaction terms calculated from a piecewise linear potential summed over all protein and ligand heavy atoms. OCS is an OpenEye variant of the Chemscore. SCR derives from a combination of the PLP and FlexX score. SHA is a shape-based scoring function that uses smooth Gaussian functions to represent the shapes of molecules.
GLIDE 5.0
The binding site was defined by a rectangular box of 10 Å in the x, y and z directions centered on the ligand. The possibility of docking compounds with more than 120 atoms was activated, whereas the GLIDECitation25 defaults were used for all other parameters. The GlideScore fitness function is based on Chemscore but includes a steric-clash term, adds buried polar terms to penalize electrostatic mismatches and modifications on other secondary terms. Two docking analyses were carried out using the standard precision (SP) and extra precision (XP) methods. The XP mode is a refinement tool designated for use only on good ligand poses, the sampling is based on an anchor and refined growth strategy, and the scoring function includes a more complete treatment of some of the SP terms, such as the solvation and hydrophobic terms.
GOLD 4.1
The region of interest for the docking studies was defined in such a manner that it contained all residues which stayed within 10 Å from the ligand in the X-ray structures; the “allow early termination” command was deactivated, while the possibility for the ligand to flip ring corners was activated. For all other parameters, the GOLDCitation31 defaults were used and the ligands were subjected to 30 genetic algorithm runs. Four docking analyses were carried out. The four fitness functions implemented in GOLD, i.e. GOLDScore (GS), ChemScore (CS), Astex Statistical Potential (ASP) and ChemPLP (PLP), were used.
AUTODOCK VINA 1.0
The input files for proteins and ligands originated from the AUTODOCK Tools utilities for AUTODOCK calculations were also used for the AUTODOCK VINACitation27 calculations, including the grid box dimensions. The exhaustiveness parameter was set to 10 and the Energy_range to 1, whereas for all other parameters, the AUTODOCK VINA defaults were used. The VINA scoring function combines certain advantages of knowledge-based potentials and empirical scoring functions, extracting information from both the conformational preferences of the receptor–ligand complexes and the experimental affinity measurements.
Rescoring evaluation
A first enriched dataset constituted by 12 active ERβ ligands extracted from their X-ray complexes and 2570 molecule derived from the DUD estrogen agonists decoys databaseCitation32 was docked by means of GLIDE with the extra precision mode into the ERβ structure (1YYE PDB codeCitation33). The docking results were then scored by means of the following rescoring functions: AUTODOCK 4.0; AUTODOCK VINA 1.0; AMBER, Hawkins GB/SA (GBSA), grid, PB/SA (PBSA), Zou GB/SA (ZGBSA) from DOCK 6.5; CG3, CS, PLP, OCS, SCR, SHA, from FRED 2.1; GLIDE SP and XP; GS, CS, ASP, and PLP from GOLD 4.1. All the default parameters were applied when using these scoring functions. A second enriched ERβ dataset constituted by the 2570 molecule derived from the DUD estrogen agonists decoys database and 40 active ERβ ligands reported in literature was generated and subjected to the same docking rescoring evaluation described above.
Virtual screening evaluation
A commercial database of 400 000 constituted by a selection from different vendors was docked into the ERβ structure (1YYE PDB codeCitation33) by means of a GLIDE two steps procedure. In the first step, a constrained GLIDE SP docking evaluation was carried out, in which all the compounds that were unable to form two H-bonds with H475 and the E305-R346 network system were rejected. In the second step, an unconstrained docking calculation using GLIDE XP method was carried out. All the compounds after the docking calculation showed that the two H-bonds with H475 and the E305-R346 network system were then rescored by using the FRED CS and GLIDE SP scoring functions.
Statistical evaluation
The results were evaluated by using the enrichment factor (EF) and the area under curve (AUC) of the receiver operator characteristic (ROC) curveCitation34. The EF measures the enrichment of the method compared with random selection:
where tp is the number of high-affinity compounds not rejected (true positives); fn is the number of high-affinity compounds rejected during the VS filtering (false negatives); NCtot is the total number of molecules of the database; NC is the total number of compounds obtained by the VS protocol. The EF10% indicates the EF values retaining the 10% of the whole database, the maximum value that can be reach is EF10% = 10, therefore all the evaluated EF10% were reported as the percentage of this value. The AUC is the area under the ROC curve; when the discrimination between actives and decoys is random the AUC correspond to 0.5, whereas in the ideal performance, where all the known active ligands are ranked before all the decoys, the AUC is very close to 1.0.
Molecular dynamic simulations
All simulations were performed using AMBER 11Citation29. The complexes were solvated with a 10-Å water cap. Sodium ions were added as counterions to neutralize the system. Two steps of minimization were then carried out. In the first stage, we kept the complexes fixed with a position restraint of 500 kcal/(mol Å2) and we solely minimized the positions of the water molecules. In the second stage, we minimized the entire system through 20 000 steps of steepest descent followed by conjugate gradient until a convergence of 0.05 kcal/(mol Å2) was attained. All the α carbons of the protein were blocked with a harmonic force constant of 10 kcal/(mol Å2). Four nanoseconds of MD simulation were then carried out. The time step of the simulations was 2.0 fs with a cut-off of 10 Å for the non-bonded interaction, and SHAKE was employed to keep all bonds involving hydrogen atoms rigid. Constant-volume periodic boundary MD was carried out for 200 ps, during which the temperature was raised from 0 to 300 K. Then 3.8 ns of constant pressure periodic boundary MD was carried out at 300 K using the Langevin thermostat to maintain the temperature of our system constant. General Amber force field (GAFF) parameters were assigned to the ligands, while partial charges were calculated using the AM1-BCC method as implemented in the Antechamber software from AMBER 11.
Similarity search
The test compounds were compared with published ER ligands. This comparison was made using the Similarity Search task of SciFinderCitation35. Similarity search locates structures that are similar to the query, based on a two-dimensional small-molecule comparison using a Tanimoto similarity metric. A score of 100 means that the two structures are identical.
Results and discussion
Docking evaluation
A total of 18 X-ray structures of ERβ complexed with agonists were retrieved from the RCSB protein data bankCitation19. The reliability of various docking software packages was assessed by applying a self-docking and a cross-docking approach. In the self-docking step each ligand was docked back into its native protein structure, whereas in the cross-docking step each ligand was docked into all the 18 available structures of ERβ. In both steps, the resulting docking poses of each ligand were evaluated through the comparison with its corresponding experimental position. For this purpose, the RMSD between the positions of the heavy atoms of the ligand in the predicted and experimental structures was calculated.
Self-docking results
All the 18 ligands were extracted from the X-ray complexes and subjected to conformational search. Then, they were docked into their corresponding proteins. Six different docking software packages with a total of 18 different docking procedures (see section “Methods” for details) were tested for their ability to self-dock estrogen ligands. shows for each docking procedure the average RMSD of the position of the ligand resulting from the docking with respect to the experimental disposition. DOCK software seemed to be the most reliable as it showed RMSD values of 0.6–0.7 Å, followed by GLIDE (RMSD values of 1.0–1.1 Å).
Cross-docking results
Compared to self-docking studies, cross-docking analysis is a more reliable approach for verifying how efficiently docking studies can support lead identification and structure–activity relationship analysis, because it evaluates how efficiently a docking software is able to reproduce the experimentally determined binding pose of a ligand by docking it into a protein whose 3D structure was determined in a complex with a different ligand. For all 18 complexes, the ligand was extracted and docked in all human ERβ structures, and the obtained docking results were compared with the experimentally determined ligand dispositions (see “Methods” section for details). The cross-docking studies were carried out using all the docking procedures applied for the self-docking calculations; as a result, 5832 docking calculations were taken into account. Beyond the average, RMSD of the ligands poses resulting from the docking with respect to their experimental disposition, the number of ligands with a reliable docking pose (i.e. the number of ligands with a RMSD smaller than 2.0 Å) was used as a second parameter for comparing the reliability of the results obtained from the different docking procedures. As shown in , GLIDE_XP showed the best results with an average RMSD of 1.4 Å and about 75% of compounds with a RMSD smaller than 2.0 Å. DOCK6_GRID and GOLD_ASP also showed good results with an average RMSD of 1.8 Å and 63–68% of compounds with an RMSD smaller than 2.0 Å, whereas all the other docking procedures showed an average RMSD greater than 2 Å and less than 60% of compounds with a RMSD smaller than 2.0 Å.
Figure 2. Results of the cross-docking study. The average RMSD (A) and the number of poses with a RMSD less than 2 Å (B) is reported.
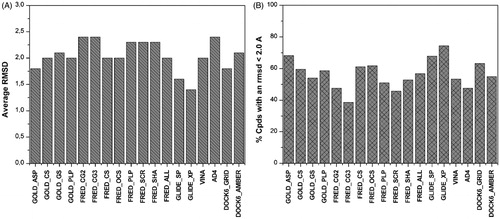
In conclusion, the docking evaluation strongly suggested that, for docking ERβ agonists, GLIDE using the extra precision mode was the best docking approach followed by DOCK using the Grid energy score.
Rescoring evaluation
In protein–ligand docking, the scoring functions are needed from both a qualitative and quantitative point of view. The first role of a scoring function is the identification of the correct pose of a particular ligand, whereas the second role is to rank the docking results according to the relative binding affinities of different ligands. This second role is fundamental for the docking-based VSCitation36. In order to investigate the reliability of scoring functions in discriminating active from inactive ERβ agonists, an enriched database was created and 20 different scoring functions were applied. The 18 ERβ agonists previously used in the cross-docking studies were clustered on the bases of their chemical properties and the resulting 12 active ligands () were added to the DUD estrogen agonists decoys database, comprising 2570 moleculesCitation32. The resulting database of 2582 molecules was docked into the ERβ structure (1YYE PDB codeCitation33) using GLIDE_XP, as this method proved to be the best one in the cross-docking studies. The employment of active ligands with a known interaction disposition, for which docking evaluation proved to be highly reliable (), should guarantee that for these compounds the rescoring process is not influenced by their erroneous disposition into the binding site.
The docked database was then rescored using 20 scoring functions (see “Methods” section for details), and the results were evaluated in terms of area under the enrichment factor curve (AUC) and enrichment factor for the top 10% of the ranked database (EF10%). The AUC is a measure of the overall performance of a method and the enrichment factor is defined as the fraction of known ligands identified in certain thresholds of the ranked database. At a fixed percentage of the database, the higher the enrichment factor, the better the performance of the method in identifying known ligands. All the evaluated EF10% were reported as the percentage of the maximum reachable EF10% value. As shown in , taking into account the AUC and EF10% factors, six different scoring functions seemed to work better than the others. In particular, DOCK software using AMBER score and ZGBSA Score, FRED and GOLD software using the CS function, FRED using the SCR score and GLIDE using the SP method showed AUC values greater than 0.8 and EF10% greater than 80%.
Figure 3. AUC (black) and EF10% (white) values of the first enriched dataset using each scoring function.
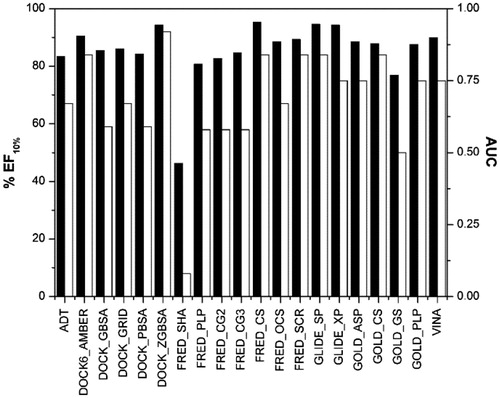
In order to further test the reliability of the rescoring functions, a second dataset of active ERβ agonists was used. The most important compounds reported in the literature as ERβ agonists that were tested in the laboratory of Prof Katzenellenbogen were analyzed and all the compounds with an ERβ Ki lower than 12.5 nM, which corresponds to a relative binding affinity (RBA) higher than 4%, were considered as active ligands. As a result, 40 ligands (see Table S1 in the Supplementary Material) were added to the DUD estrogen agonist decoys database of 2570 molecules, and the database was docked with GLIDE SP and then rescored through the previously used scoring functions. As shown in , the analysis of the AUC and EF10% confirmed some of the results obtained with the first dataset. In particular, the use of FRED with the CS function and GLIDE with the SP method confirmed their reliability, whereas the other four scoring functions that showed promising results with the first dataset (i.e. DOCK software using AMBER score and the ZGBSA score, GOLD software using the CS function and FRED using the SCR) here showed worse statistical results, in particular with EF10% values lower than 70.0%.
Virtual screening studies
The results so obtained highlighted that among the methods tested, the use of GLIDE_XP for the docking evaluation and the rescoring with FRED_CS and GLIDE_SP seemed to be the best filtering procedures. On these bases a VS workflow was developed (). A database of about 400 000 molecules was used for the VS study and was enriched with the dataset of the 40 active ERβ agonists used in the previous analysis. It is currently known that the most important ERβ agonists usually show two H-bonds with the receptor binding site, one with H475 and one with the E305-R346 network system. The analysis of the available ERβ crystal structures confirmed these data, as only one ligand (2GIU PDB codeCitation37) did not show these two H-bonds. On these bases, as a first step of the virtual screening workflow (), a constrained docking evaluation was applied.
Figure 5. Virtual screening workflow. *The compounds that after the docking calculation showed the two H-bonds with H475 and the E305-R346 network system were taken into account. **The compounds that maintained the H-bonds with H475 and the E305-R346 network system for at least the 75% of MD simulation were considered.
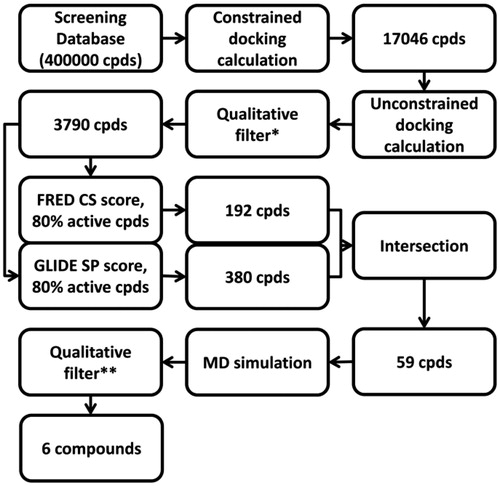
Using GLIDE with the single precision method, a constraint on the presence of the two H-bonds with H475 and the E305-R346 network system was applied. This first step led us to easily remove all the compounds for which it was not energetically possible to form the two H-bonds (due to steric, geometric or atom type problems). The filtered database was then subjected to an unconstrained docking calculation using GLIDE_XP. All the compounds that showed the two H-bonds with H475 and the E305-R346 network system after the docking calculation were then taken into account. The so-obtained 3790 compounds were then rescored using FRED with the ChemScore function and GLIDE with the simple precision method, since they showed the best results for the first and second datasets. Taking also into account the scoring results for the known active ligands, the statistical analysis of these new data confirmed the high-reliability of GLIDE and FRED (). Considering the good ability of GLIDE and FRED in recognizing the active ERβ agonists, the compounds that were suggested as active (i.e. those showing a scoring value in the range of the first 80% of the active molecules, see ) by each of these two scoring functions were filtered, and then only the 59 compounds that were considered as active by both approaches were taken into account (; Table S3 in the Supplementary Material).
Figure 6. Rescoring results for the enriched database using GLIDE (A) and FRED (B). The grey vertical line corresponds to the threshold used for filtering the compounds.
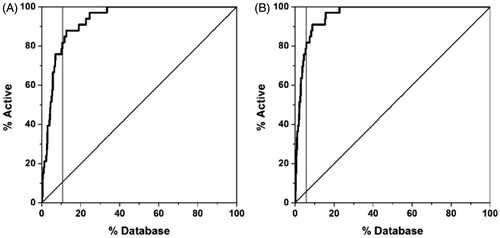
In order to setup a MD simulation protocol, the complex between 3-(3-fluoro-4-hydroxyphenyl)-7-hydroxy-1-naphthonitrile and human ERβ (1YYE PDB codeCitation33) was used as a test set. The residues beyond a threshold of 15 Å from the ligand were removed and as a result only the segment E276-K391 was considered and solvated with a 10-Å water cap (see “Methods” section for more details). The ligand–protein complex was then subjected to a total of 4 ns of MD simulation. As shown in Figure S1 in the Supplementary Material, the system reached an equilibrium after about 300 ps, since the total energy for the last 3.7 ns remained approximately constant. By analyzing the RMSD of all the heavy atoms from the X-ray structures, we observed an initial increase due to the equilibration of the system, followed, after 500 ps, by a stabilization of the RMSD value around 1.2 Å. Regarding the geometry of the ligand, we analyzed the RMSD of the position of the ligand with respect to the X-ray structures during the simulation. Figure S1 in the Supplementary Material shows that the ligand demonstrated an RMSD value around 0.2 Å. With regards to the H-bond analysis (as shown in Table S2 in the Supplementary Material), the interaction of the ligand with H475 and the E305-R346 network system appeared to be very stable as these interactions were maintained during the whole MD simulation and a water molecule was also found to mediate the interaction with R346.
The 59 compounds obtained by the previous VS steps were subjected to MD simulation using the protocol described above and all the ligands that maintained the H-bonds with H475 and the E305-R346 network system for at least 3 ns out of the total 4 ns (i.e. corresponding to the 75% of MD simulation) were further considered. Using this filter, only the six compounds showed in survived and therefore were taken into account. As shown in Figure S2 in the Supplementary Material, a comparison between the scores obtained for the known active ERβ agonists used in the enriched VS dataset and those obtained for these compounds highlighted that all the six compounds belonged to the top 40% of the active scored compounds, thus supporting the potential activity of these molecules.
Table 2. Hypothetical lead compounds.
A visual inspection of the six compounds strongly supported the possibility of clustering them into three main groups. The first cluster was constituted by compounds 1, 2 and 3, the second one by compounds 4 and 5, whereas compound 6 constituted the third cluster. As shown in , the 1,3-dihydroxyphenyl ring of compound 1 formed H-bonds with M295 and H475, the thiazole fragment occupied the middle portion of the binding site and the 4-hydroxyphenyl portion interacted with E305. The hydroxy group on the chromenone central scaffold of compound 4 () formed an H-bond with H475, the chromenone scaffold showed lipophilic interactions with L298 whereas the 3,4-dihydroxyphenyl ring formed H-bonds with E305. With regard to compound 6, the 3-hydroxybenzyl group showed an H-bond with H475, the benzimidazole ring showed lipophilic interactions with I376 and F377 and the 3-hydroxyphenyl ring showed an H-bond with E305 ().
Figure 7. Minimized average structures of compounds 1 (B), 4 (C), and 6 (D) docked into ERβ. The X-ray complex between ERβ and 3-(3-fluoro-4-hydroxyphenyl)-7-hydroxy-1-naphthonitrile (1YYE PDB code) is also reported as a reference system (A).
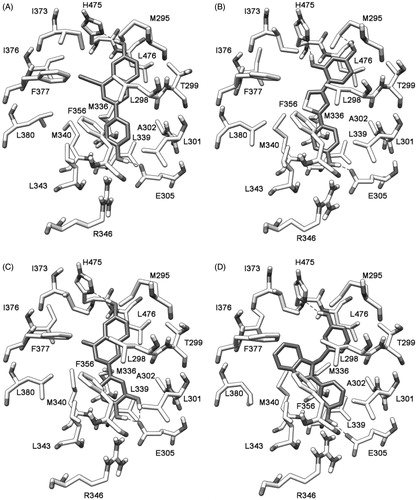
Very interestingly, a similarity search for the six compounds against the already reported ER ligands revealed that all of them were very similar to already reported ER ligands. Bey and co-workers in 2008 and 2009 reported the design, synthesis and biological evaluation of bis(hydroxyphenyl) substituted azoles, thiophenes, benzenes and aza-benzenes as potent and selective non-steroidal inhibitors of 17β-hydroxysteroid dehydrogenase type 1. Some of these compounds, very similar to compounds 1–3 (similarity score = 85–89; score = 100 means that the two compounds are identical), were also tested for their activity against ERα and ERβ and one compound showed a certain binding affinity for ERβCitation38,Citation39. Compound 4 had been already reported as an ER agonist (similarity score = 100)Citation40. In 2003, Suetsugi and co-workers using mammalian cell transfection and mammalian two-hybrid experiments revealed that this flavone behaved as an agonist of both ERα and ERβ. Finally, compound 6 was very similar to the members of the class of benzimidazoles patented by AstraZeneca AB in 2002 as selective ERβ ligands (similarity score = 90–94)Citation41.
Conclusions
In the present study, we tested the application of a docking-based method for the development of a VS study for ERβ ligands. Prior to apply the VS procedure, different docking methods and rescoring functions were evaluated for their ability to correctly predict the binding mode and the energy interactions of known ERβ ligands. The best approach for developing the VS study seemed to be achieved by combining GLIDE docking and the rescoring of docking results with the ChemScore function of FRED and the simple precision method of GLIDE. The optimized procedure was then used for filtering a collection of 400 000 commercial compounds. The obtained results strongly support the reliability of our VS procedure, as the resulting six compounds that survived the VS filtering procedure were very similar to already reported ERβ-active ligands. Taken together, these findings suggest that the techniques herein optimized may be suitable for the design of new ERβ ligands. On these bases, the obtained results urge us to apply this VS protocol to larger commercial databases in order to identify novel ERβ ligands.
Supplementary material available online
Supplementary Tables 1–3
Supplementary Figures 1 and 2
Supplemental Material.pdf
Download PDF (372 KB)Acknowledgements
Many thanks are due to Prof. Maurizio Botta for using the GLIDE program in his computational laboratory (University of Siena, Italy).
Declaration of interest
The authors report no declarations of interest.
References
- Dahlman-Wright K, Cavailles V, Fuqua SA, et al. International Union of Pharmacology. LXIV. Estrogen receptors. Pharmacol Rev 2006;58:773–81
- Dey P, Barros RP, Warner M, et al. Insight into the mechanisms of action of estrogen receptor beta in the breast, prostate, colon, and CNS. J Mol Endocrinol 2013;51:T61–74
- Harris HA. Estrogen receptor-beta: recent lessons from in vivo studies. Mol Endocrinol 2007;21:1–13
- Swedenborg E, Power KA, Cai W, et al. Regulation of estrogen receptor beta activity and implications in health and disease. Cell Mol Life Sci 2009;66:3873–94
- Hartman J, Strom A, Gustafsson JA. Current concepts and significance of estrogen receptor beta in prostate cancer. Steroids 2012;77:1262–6
- Haring J, Schuler S, Lattrich C, et al. Role of estrogen receptor beta in gynecological cancer. Gynecol Oncol 2012;127:673–6
- Treeck O, Pfeiler G, Mitter D, et al. Estrogen receptor {beta}1 exerts antitumoral effects on SK-OV-3 ovarian cancer cells. J Endocrinol 2007;193:421–33
- Leygue E, Murphy LC. A bi-faceted role of estrogen receptor beta in breast cancer. Endocr Relat Cancer 2013;20:R127–39
- Haldosen LA, Zhao C, Dahlman-Wright K. Estrogen receptor beta in breast cancer. Mol Cell Endocrinol 2014;382:665–72
- Kumar S, Patel R, Moore S, et al. Estrogen receptor beta ligand therapy activates PI3K/Akt/mTOR signaling in oligodendrocytes and promotes remyelination in a mouse model of multiple sclerosis. Neurobiol Dis 2013;56:131–44
- Wu WF, Tan XJ, Dai YB, et al. Targeting estrogen receptor beta in microglia and T cells to treat experimental autoimmune encephalomyelitis. Proc Natl Acad Sci USA 2013;110:3543–8
- Minutolo F, Bertini S, Granchi C, et al. Structural evolutions of salicylaldoximes as selective agonists for estrogen receptor beta. J Med Chem 2009;52:858–67
- McDonnell DP, Norris JD. Connections and regulation of the human estrogen receptor. Science 2002;296:1642–4
- Minutolo F, Macchia M, Katzenellenbogen BS, Katzenellenbogen JA. Estrogen receptor beta ligands: recent advances and biomedical applications. Med Res Rev 2011;31:364–442
- Zhao L, Brinton RD. Structure-based virtual screening for plant-based ERbeta-selective ligands as potential preventative therapy against age-related neurodegenerative diseases. J Med Chem 2005;48:3463–6
- Knox AJ, Meegan MJ, Sobolev V, et al. Target specific virtual screening: optimization of an estrogen receptor screening platform. J Med Chem 2007;50:5301–10
- Shen J, Tan C, Zhang Y, et al. Discovery of potent ligands for estrogen receptor beta by structure-based virtual screening. J Med Chem 2010;53:5361–5
- Cao X, Jiang J, Zhang S, et al. Discovery of natural estrogen receptor modulators with structure-based virtual screening. Bioorg Med Chem Lett 2013;23:3329–33
- Berman HM, Westbrook J, Feng Z, et al. The protein data bank. Nucleic Acids Res 2000;28:235–42
- Maestro, version 9.0. Portland (OR): Schrödinger Inc; 2009
- Macromodel, version 9.7. Portland (OR): Schrödinger Inc; 2009
- Morris GM, Huey R, Lindstrom W, et al. AutoDock4 and AutoDockTools4: automated docking with selective receptor flexibility. J Comput Chem 2009;30:2785–91
- DOCK, version 6.0. San Francisco (CA): Molecular Design Institute, University of California; 1998
- FRED, version 2.2.5. Santa Fe (NM): OpenEye Scientific Software, Inc.; 2010
- GLIDE, version 5.0. Portland (OR): Schrödinger Inc; 2009
- Verdonk ML, Mortenson PN, Hall RJ, et al. Protein-ligand docking against non-native protein conformers. J Chem Inf Model 2008;48:2214–25
- Trott O, Olson AJ. AutoDock Vina: improving the speed and accuracy of docking with a new scoring function, efficient optimization, and multithreading. J Comput Chem 2010;31:455–61
- Sanner MF. Python: a programming language for software integration and development. J Mol Graph Model 1999;17:57–61
- Case DA, Darden TA, Cheatham TE III, et al. AMBER, version 11. San Francisco (CA): University of California; 2010
- OMEGA2, version 2.4.6. Santa Fe (NM): OpenEye Scientific Software, Inc.; 2013
- Verdonk ML, Cole JC, Hartshorn MJ, et al. Improved protein-ligand docking using GOLD. Proteins 2003;52:609–23
- Huang N, Shoichet BK, Irwin JJ. Benchmarking sets for molecular docking. J Med Chem 2006;49:6789–801
- Mewshaw RE, Edsall RJ, Jr, Yang C, et al. ERbeta ligands. 3. Exploiting two binding orientations of the 2-phenylnaphthalene scaffold to achieve ERbeta selectivity. J Med Chem 2005;48:3953–79
- Poli G, Tuccinardi T, Rizzolio F, et al. Identification of new fyn kinase inhibitors using a FLAP-based approach. J Chem Inf Model 2013;53:2538–47
- SciFinder Scholar. Washington, DC: American Chemical Society; 2013
- Tuccinardi T. Docking-based virtual screening: recent developments. Comb Chem High Throughput Screen 2009;12:303–14
- Wilkening RR, Ratcliffe RW, Tynebor EC, et al. The discovery of tetrahydrofluorenones as a new class of estrogen receptor beta-subtype selective ligands. Bioorg Med Chem Lett 2006;16:3489–94
- Bey E, Marchais-Oberwinkler S, Werth R, et al. Design, synthesis, biological evaluation and pharmacokinetics of bis(hydroxyphenyl) substituted azoles, thiophenes, benzenes, and aza-benzenes as potent and selective nonsteroidal inhibitors of 17beta-hydroxysteroid dehydrogenase type 1 (17beta-HSD1). J Med Chem 2008;51:6725–39
- Bey E, Marchais-Oberwinkler S, Negri M, et al. New insights into the SAR and binding modes of bis(hydroxyphenyl)thiophenes and -benzenes: influence of additional substituents on 17beta-hydroxysteroid dehydrogenase type 1 (17beta-HSD1) inhibitory activity and selectivity. J Med Chem 2009;52:6724–43
- Suetsugi M, Su L, Karlsberg K, et al. Flavone and isoflavone phytoestrogens are agonists of estrogen-related receptors. Mol Cancer Res 2003;1:981–91
- Barlaam BD, Steven FJ. Preparation of benzimidazoles as selective estrogen receptor-b ligand. Patent WO2002046168;2002